Receive a weekly summary and discussion of the top papers of the week by leading researchers in the field.
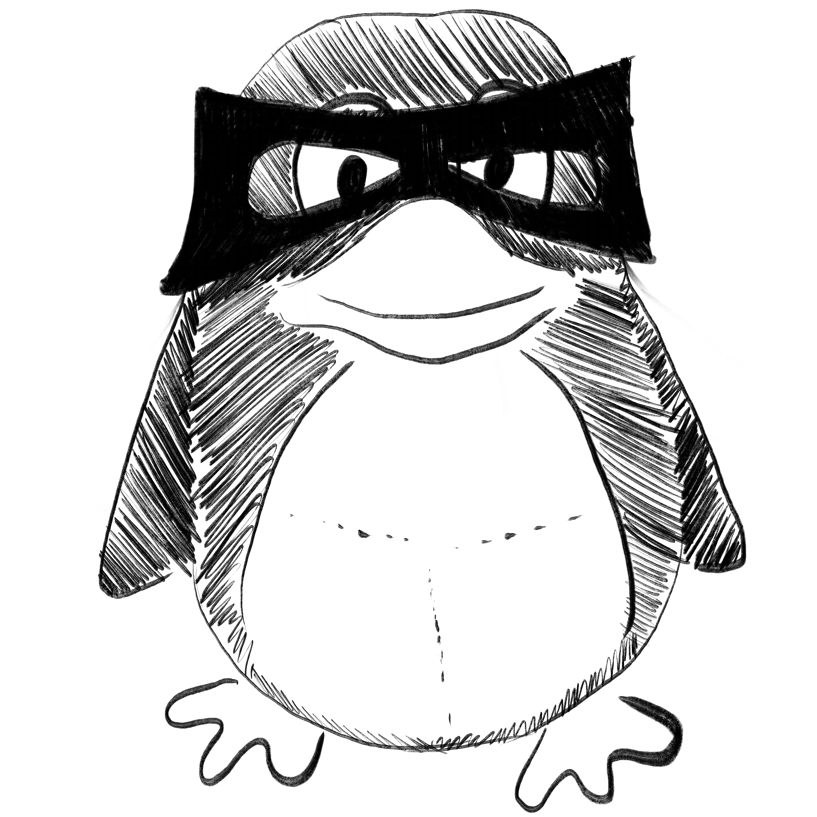
Modeling structure-activity relationships with machine learning to identify GSK3-targeted small molecules as potential COVID-19 therapeutics.
In Frontiers in endocrinology ; h5-index 55.0
Pirzada Rameez Hassan, Ahmad Bilal, Qayyum Naila, Choi Sangdun
2023
GSK3, QSAR, coronaviruses, machine learning, molecular descriptors
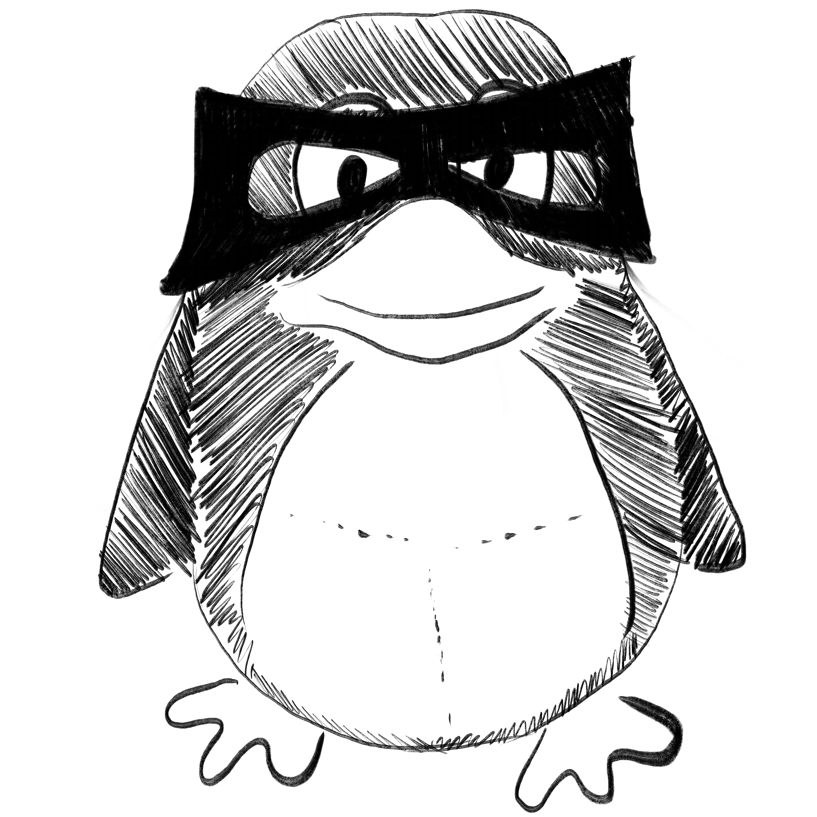
Deep Survival Analysis With Clinical Variables for COVID-19.
In IEEE journal of translational engineering in health and medicine
OBJECTIVE :
METHODS AND PROCEDURES :
RESULTS :
CONCLUSION :
CLINICAL IMPACT :
Chaddad Ahmad, Hassan Lama, Katib Yousef, Bouridane Ahmed
2023
CNN, COVID-19, clinical variables
COVID-19Base v3: Update of the knowledgebase for drugs and biomedical entities linked to COVID-19.
In Frontiers in public health
Basit Syed Abdullah, Qureshi Rizwan, Musleh Saleh, Guler Reto, Rahman M Sohel, Biswas Kabir H, Alam Tanvir
2023
CORD-19, COVID-19, SARS-CoV-2, deep learning, machine learning
Blood Inflammatory Biomarkers Differentiate Inpatient and Outpatient Coronavirus Disease 2019 From Influenza.
In Open forum infectious diseases
BACKGROUND :
METHODS :
RESULTS :
CONCLUSIONS :
Luciani Lauren L, Miller Leigh M, Zhai Bo, Clarke Karen, Hughes Kramer Kailey, Schratz Lucas J, Balasubramani G K, Dauer Klancie, Nowalk M Patricia, Zimmerman Richard K, Shoemaker Jason E, Alcorn John F
2023-Mar
SARS-CoV-2, cytokine, human, machine learning, pneumonia
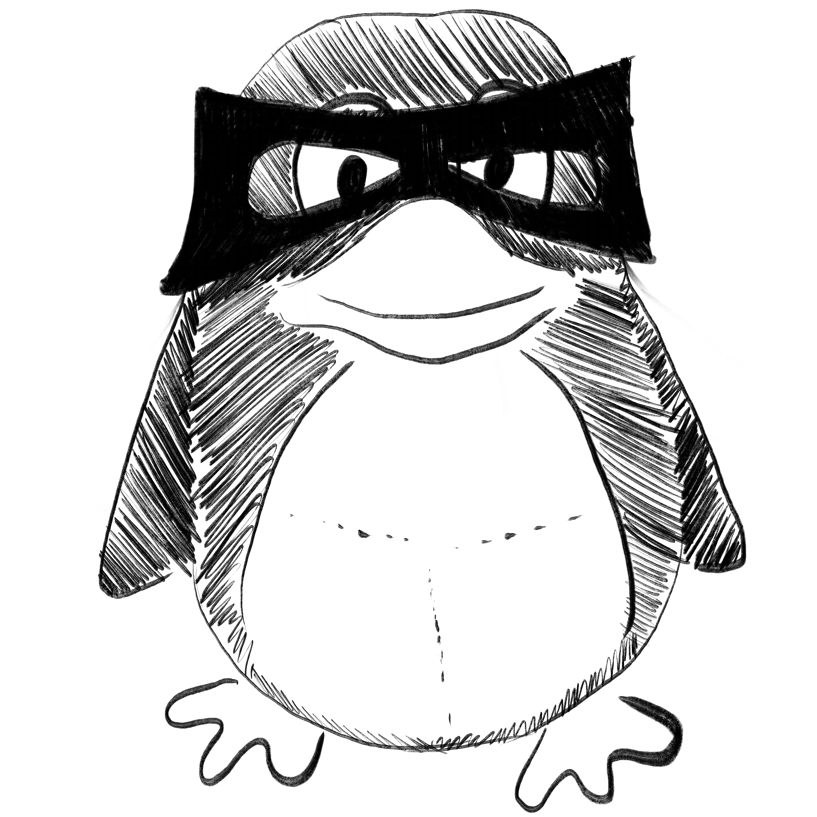
SARS-CoV-2 related adaptation mechanisms of rehabilitation clinics affecting patient-centred care: Qualitative study of online patient reports.
In JMIR rehabilitation and assistive technologies
BACKGROUND :
OBJECTIVE :
METHODS :
RESULTS :
CONCLUSIONS :
CLINICALTRIAL :
Kühn Lukas, Lindert Lara, Kuper Paulina, Choi Kyung-Eun Anna
2023-Mar-05
Predictors of Cyberchondria during the COVID-19 pandemic: A cross-sectional study using supervised machine learning.
In JMIR formative research
BACKGROUND :
OBJECTIVE :
METHODS :
RESULTS :
CONCLUSIONS :
Infanti Alexandre, Starcevic Vladan, Schimmenti Adriano, Khazaal Yasser, Karila Laurent, Giardina Alessandro, Flayelle Maèva, Hedayatzadeh Razavi Seyedeh Boshra, Baggio Stéphanie, Vögele Claus, Billieux Joël
2023-Mar-09
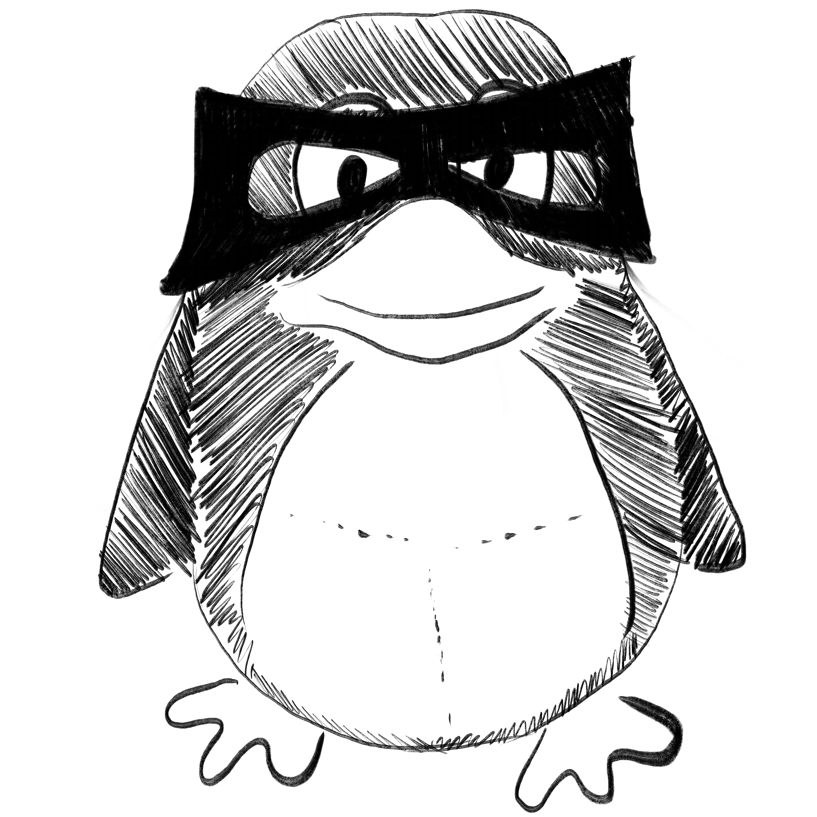
Understanding Prospective Physicians' Intention to Use Artificial Intelligence in Their Future Medical Practice: Configurational Analysis.
In JMIR medical education
BACKGROUND :
OBJECTIVE :
METHODS :
RESULTS :
CONCLUSIONS :
Wagner Gerit, Raymond Louis, Paré Guy
2023-Mar-22
artificial intelligence, attitudes and beliefs, behavioral intentions, fsQCA, fuzzy-set qualitative comparative analysis, knowledge and experience, medical education
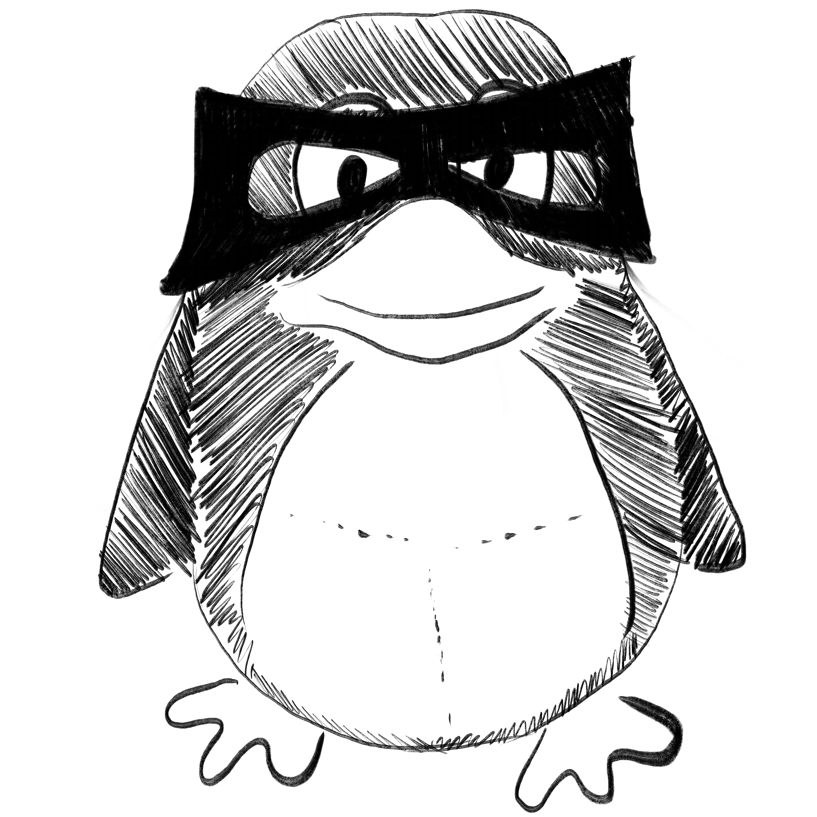
Risk Factors and Predictive Modeling for Post-Acute Sequelae of SARS-CoV-2 Infection: Findings from EHR Cohorts of the RECOVER Initiative.
In Research square
Zang Chengxi, Hou Yu, Schenck Edward, Xu Zhenxing, Zhang Yongkang, Xu Jie, Bian Jiang, Morozyuk Dmitry, Khullar Dhruv, Nordvig Anna, Shenkman Elizabeth, Rothman Russel, Block Jason, Lyman Kristin, Zhang Yiye, Varma Jay, Weiner Mark, Carton Thomas, Wang Fei, Kaushal Rainu, Consortium The Recover
2023-Mar-08
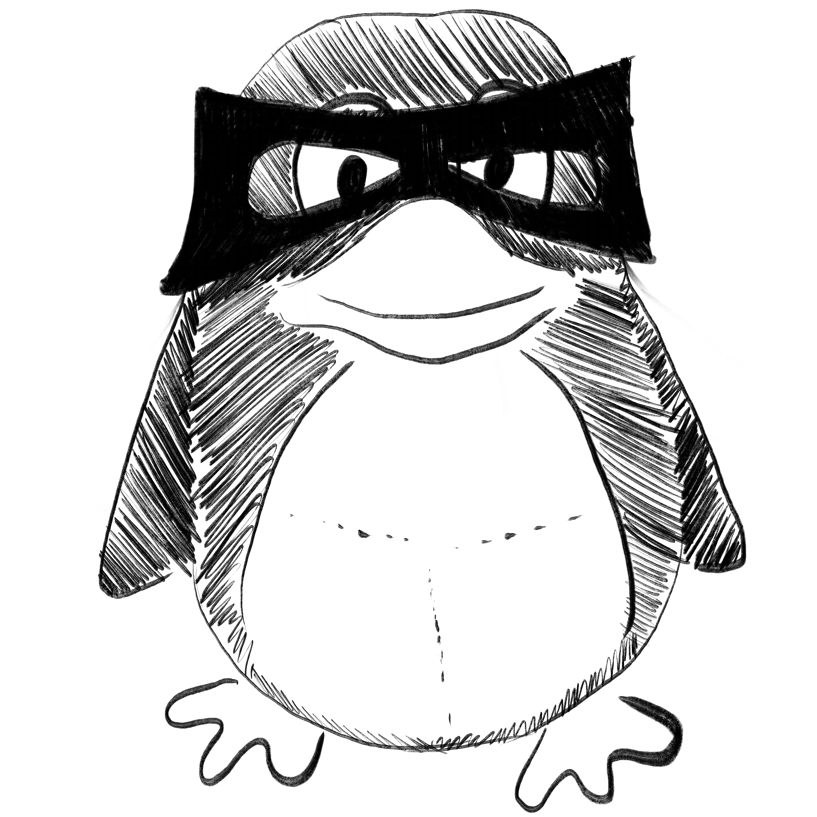
A ventilation early warning system (VEWS) for diaphanous workspaces considering COVID-19 and future pandemics scenarios.
In Heliyon
Costa Gonçal, Arroyo Oriol, Rueda Pablo, Briones Alan
2023-Mar
BIM, Building digital twin, COVID-19, Facilities management, IoT, Simulation, Smart building
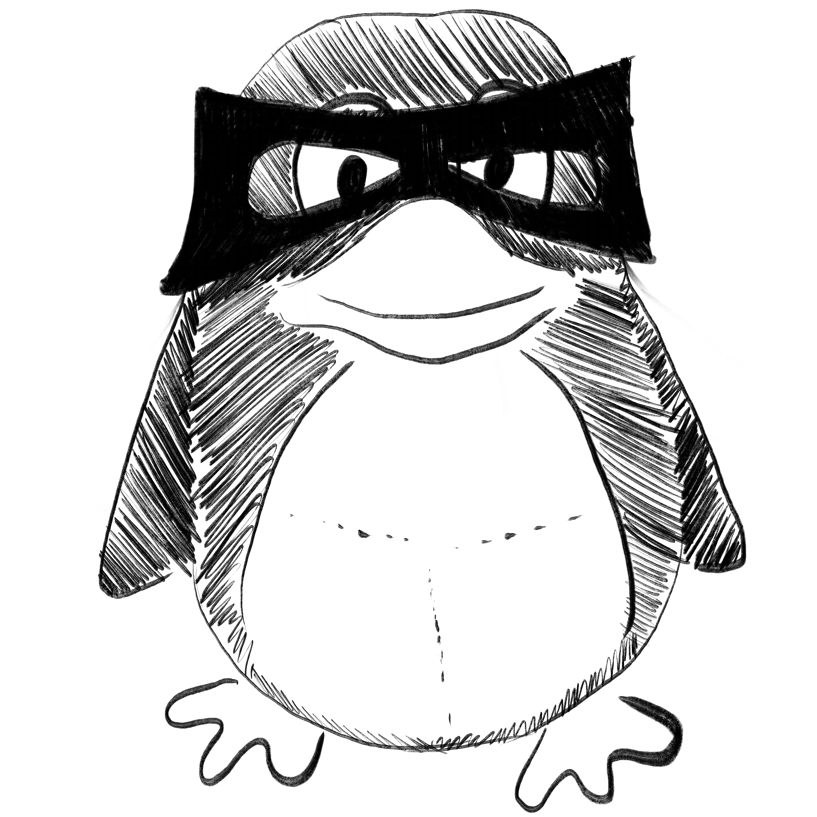
COVID-19 diagnosis: A comprehensive review of pre-trained deep learning models based on feature extraction algorithm.
In Results in engineering
Poola Rahul Gowtham, Pl Lahari, Y Siva Sankar
2023-Jun
Boundary tracing, Covid diagnosis, Deep transfer-learning, Medical imaging, Neural network models and classifiers
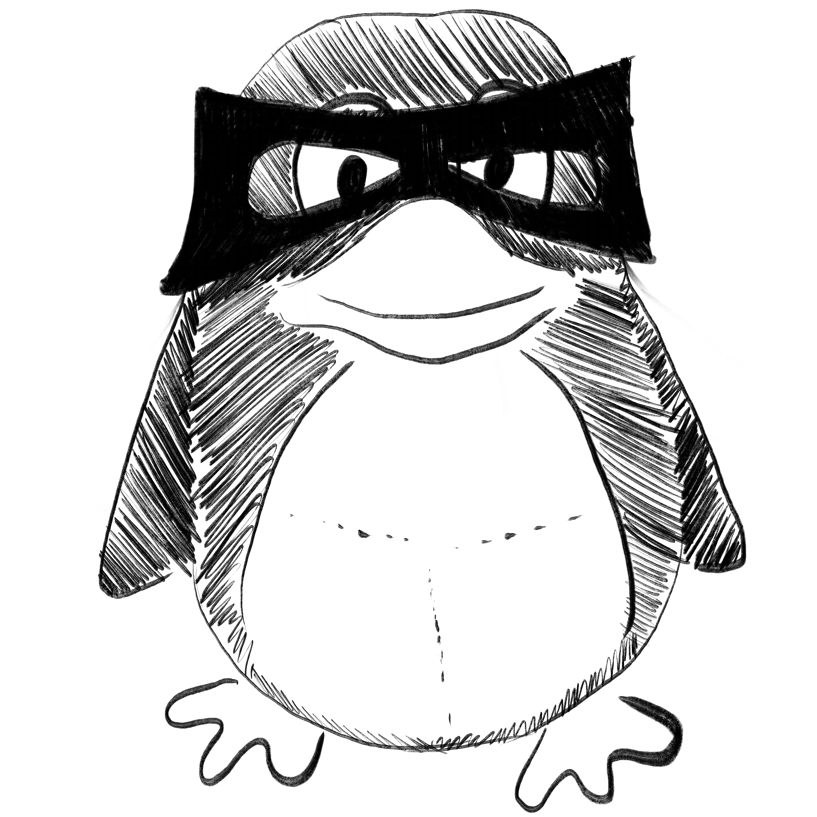
Human Behavior in the Time of COVID-19: Learning from Big Data
ArXiv Preprint
Hanjia Lyu, Arsal Imtiaz, Yufei Zhao, Jiebo Luo
2023-03-23
Impact of inactivated COVID-19 vaccines on lung injury in B.1.617.2 (Delta) variant-infected patients.
In Annals of clinical microbiology and antimicrobials
BACKGROUND :
METHODS :
RESULTS :
CONCLUSION :
Lai Miao, Wang Kai, Ding Chengyuan, Yin Yi, Lin Xiaoling, Xu Chuanjun, Hu Zhiliang, Peng Zhihang
2023-Mar-21
Artificial intelligence (AI), COVID-19, COVID-19 vaccines, Chest CT, Lung injury
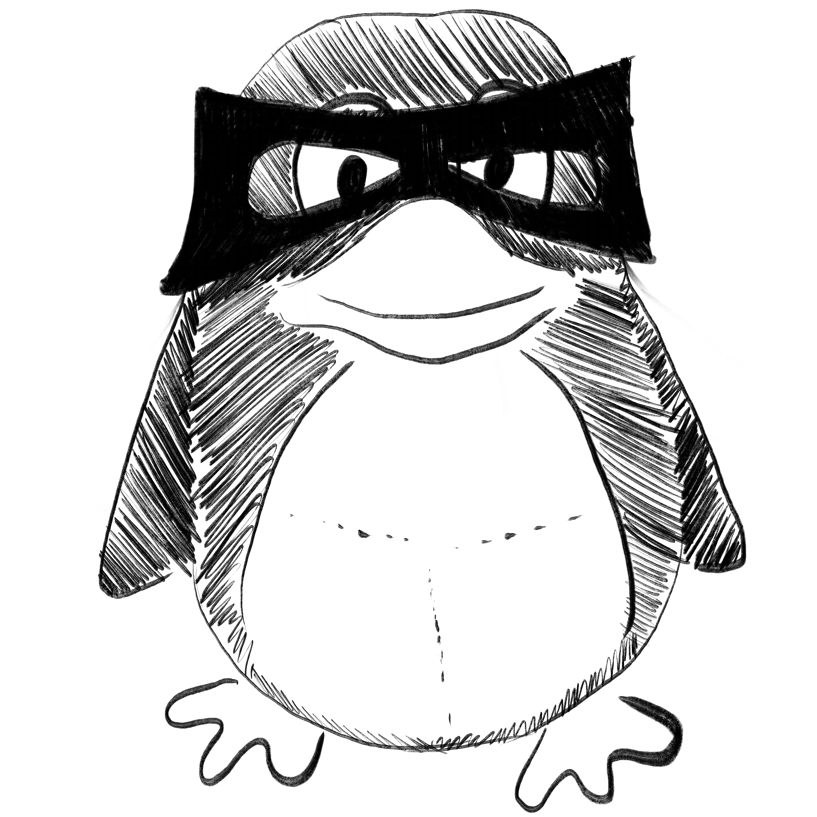
Fault Prognosis of Turbofan Engines: Eventual Failure Prediction and Remaining Useful Life Estimation
ArXiv Preprint
Joseph Cohen, Xun Huan, Jun Ni
2023-03-23
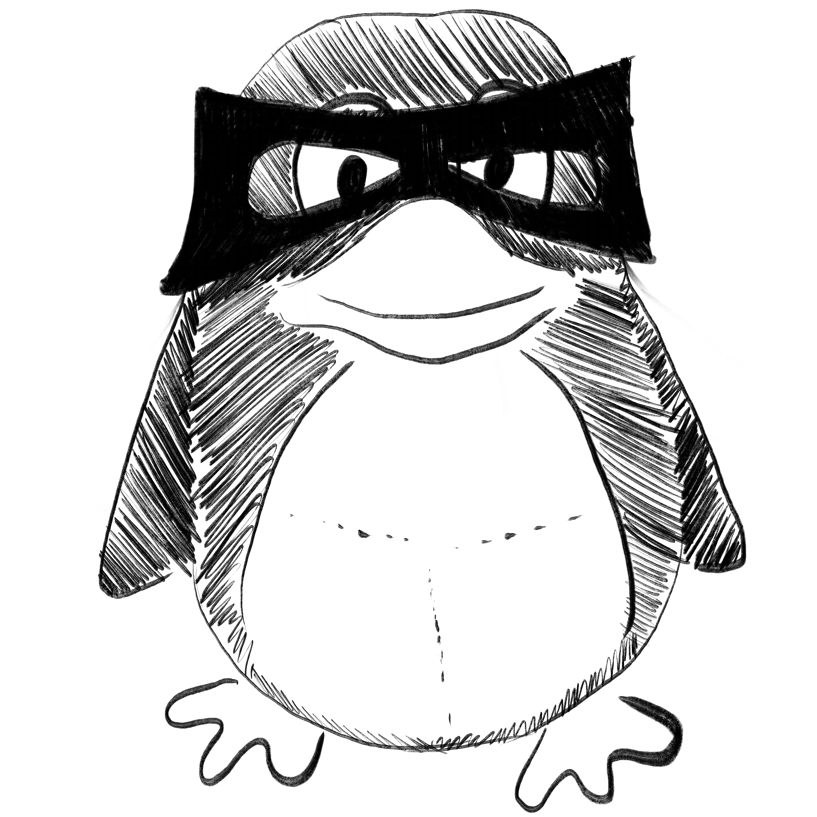
Using artificial intelligence to support rapid, mixed-methods analysis: Developing an automated qualitative assistant (AQUA).
In Annals of family medicine
Lennon Robert, Calo William, Miller Erin, Zgierska Aleksandra, Van Scoy Lauren, Fraleigh Robert
2022-Apr-01
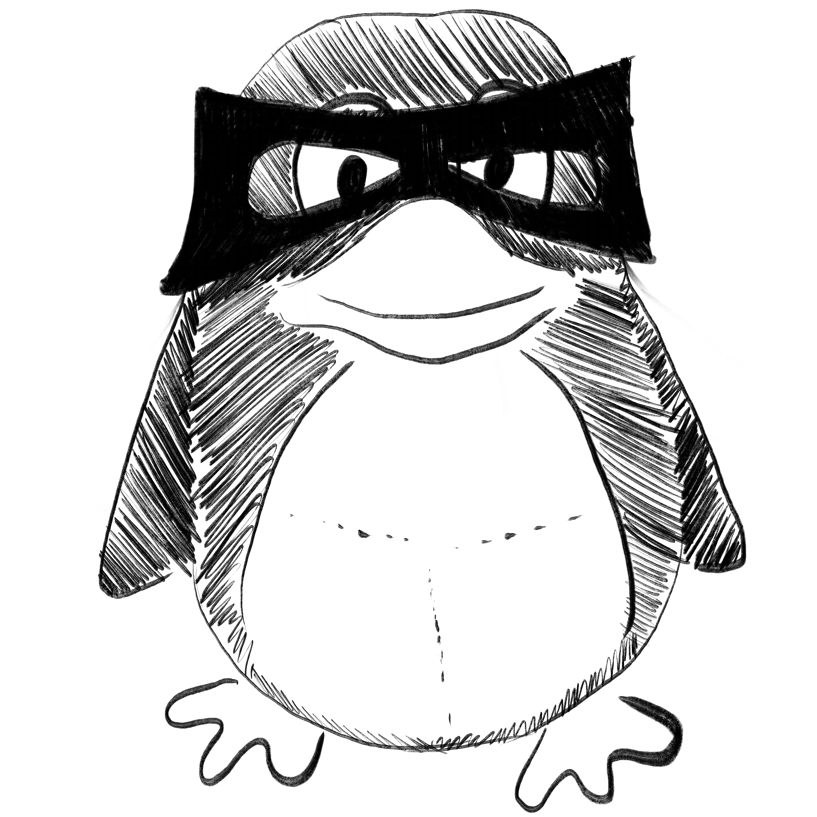
Artificial intelligence based virtual screening study for competitive and allosteric inhibitors of the SARS-CoV-2 main protease.
In Journal of biomolecular structure & dynamics
Charles Ssemuyiga, Edgar Mulumba Pius, Mahapatra Rajani Kanta
2023-Mar-21
Artificial intelligence, COVID-19, SARS-CoV-2 main protease, deep docking, molecular docking, molecular dynamics simulation, neural networks
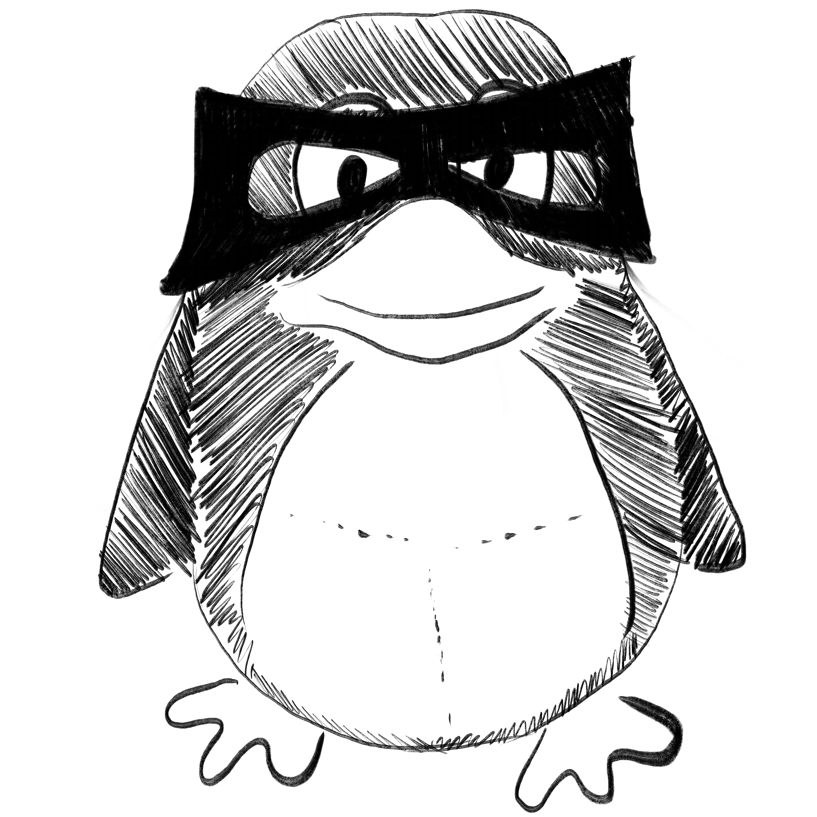
Gender Differences in the Nonspecific and Health-Specific Use of Social Media Before and During the COVID-19 Pandemic: Trend Analysis Using HINTS 2017-2020 Data.
In Journal of health communication ; h5-index 36.0
Ye Linglong, Chen Yang, Cai Yongming, Kao Yi-Wei, Wang Yuanxin, Chen Mingchih, Shia Ben-Chang, Qin Lei
2023-Mar-21
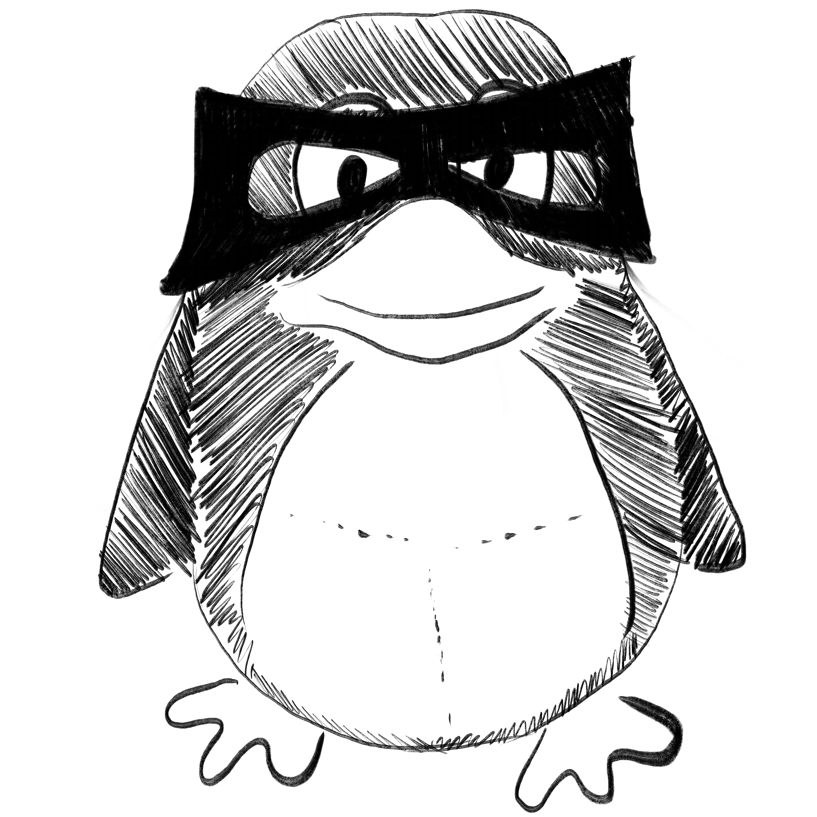
Relationship Between Coronavirus Disease 2019 Vaccination Rates and Rare But Potentially Fatal Adverse Events: A Regression Discontinuity Analysis of Western Countries.
In Journal of Korean medical science
BACKGROUND :
METHODS :
RESULTS :
CONCLUSION :
Chae Seung Hoon, Park Hyung Jun, Radnaabaatar Munkhzul, Park Hojun, Jung Jaehun
2023-Mar-20
COVID-19, Regression Discontinuity Analysis, SARS-CoV-2
Acceptability and Effectiveness of COVID-19 Contact Tracing Applications: A Case Study in Saudi Arabia of the Tawakkalna Application.
In Cureus
Dawood Safia, AlKadi Khulud
2023-Feb
acceptability, contact tracing, covid-19, mhealth, permission, privacy, privilege, security, tawakkalna
Examining thematic and emotional differences across Twitter, Reddit, and YouTube: The case of COVID-19 vaccine side effects.
In Computers in human behavior ; h5-index 125.0
Kwon Soyeon, Park Albert
2023-Jul
Consumer health information, Schema theory, Social media, Social network analysis, Unsupervised machine learning
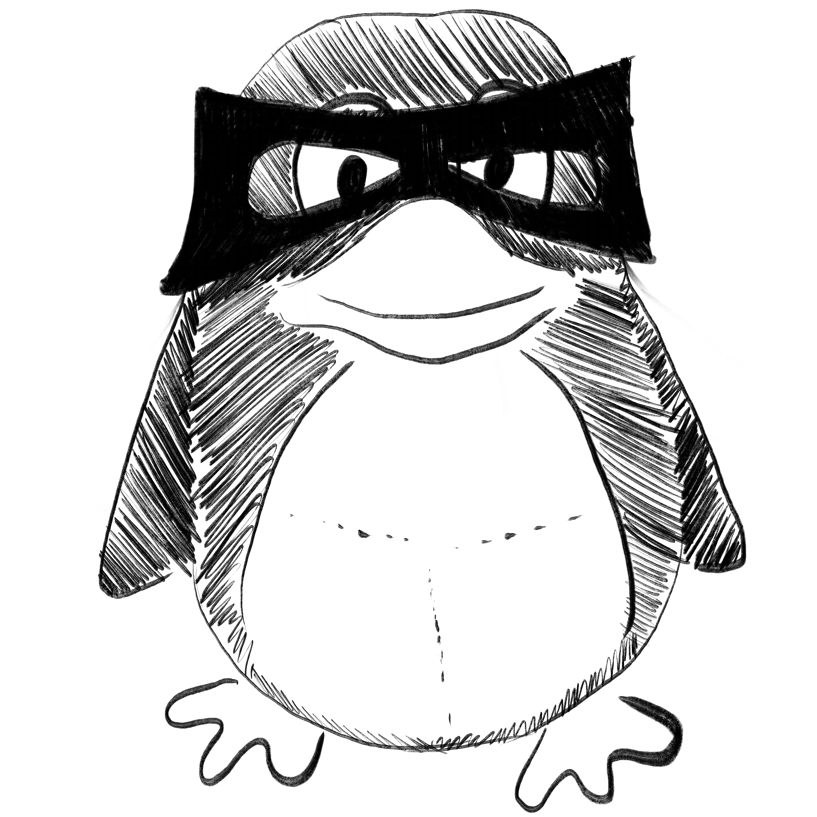
CBCovid19EC: A dataset complete blood count and PCR test for COVID-19 detection in Ecuadorian population.
In Data in brief
Ordoñez-Avila R, Parraga-Alava J, Hormaza J Meza, Vaca-Cárdenas L, Portmann E, Terán L, Dorn M
2023-Apr
Ecuador, Hematological data, Machine learning, SARS-Cov-2
Computerization of the Work of General Practitioners: Mixed Methods Survey of Final-Year Medical Students in Ireland.
In JMIR medical education
BACKGROUND :
OBJECTIVE :
METHODS :
RESULTS :
CONCLUSIONS :
Blease Charlotte, Kharko Anna, Bernstein Michael, Bradley Colin, Houston Muiris, Walsh Ian, D Mandl Kenneth
2023-Mar-20
COVID-19, artificial intelligence, biomedical, design, digital health, general practitioners, machine learning, medical education, medical professional, medical students, survey, technology, tool
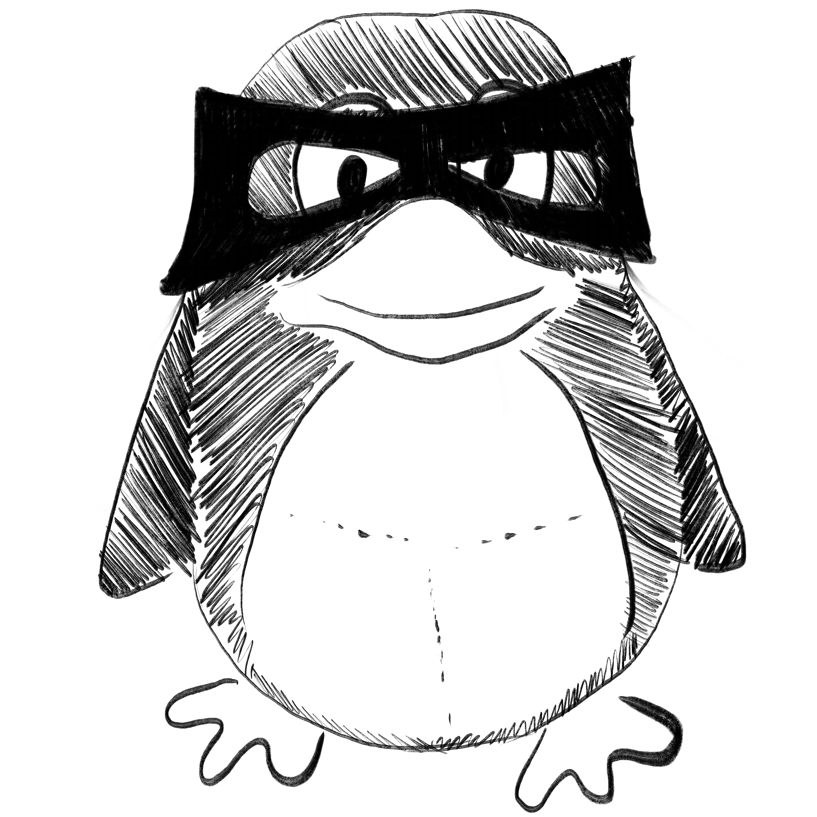
COVID-19 and pneumonia diagnosis from chest X-ray images using convolutional neural networks.
In Network modeling and analysis in health informatics and bioinformatics
Hariri Muhab, Avşar Ercan
2023
COVID-19, Classification, Convolutional neural networks, Deep learning, Lung diseases, Transfer learning
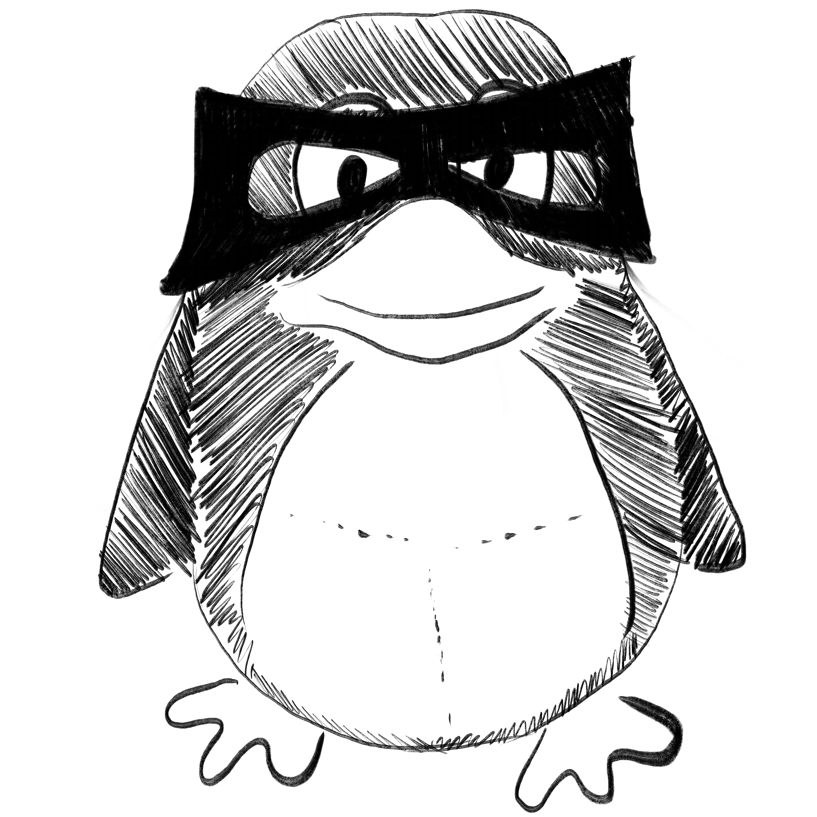
Precision recruitment for high-risk participants in a COVID-19 cohort study.
In Contemporary clinical trials communications
BACKGROUND :
METHODS :
RESULTS :
CONCLUSION :
Mezlini Aziz M, Caddigan Eamon, Shapiro Allison, Ramirez Ernesto, Kondow-McConaghy Helena M, Yang Justin, DeMarco Kerry, Naraghi-Arani Pejman, Foschini Luca
2023-Jun
CDC, Centers for Disease Control and Prevention, COVID-19, Clinical trials, GAMs, generalized additive models, Risk modeling
Computed tomography-based COVID-19 triage through a deep neural network using mask-weighted global average pooling.
In Frontiers in cellular and infection microbiology ; h5-index 53.0
BACKGROUND :
METHODS :
RESULTS :
CONCLUSIONS :
Zhang Hong-Tao, Sun Ze-Yu, Zhou Juan, Gao Shen, Dong Jing-Hui, Liu Yuan, Bai Xu, Ma Jin-Lin, Li Ming, Li Guang, Cai Jian-Ming, Sheng Fu-Geng
2023
artificial intelligence, computed tomography (CT), coronavirus disease 2019 (COVID-19), deep learning, global average pooling (GAP)
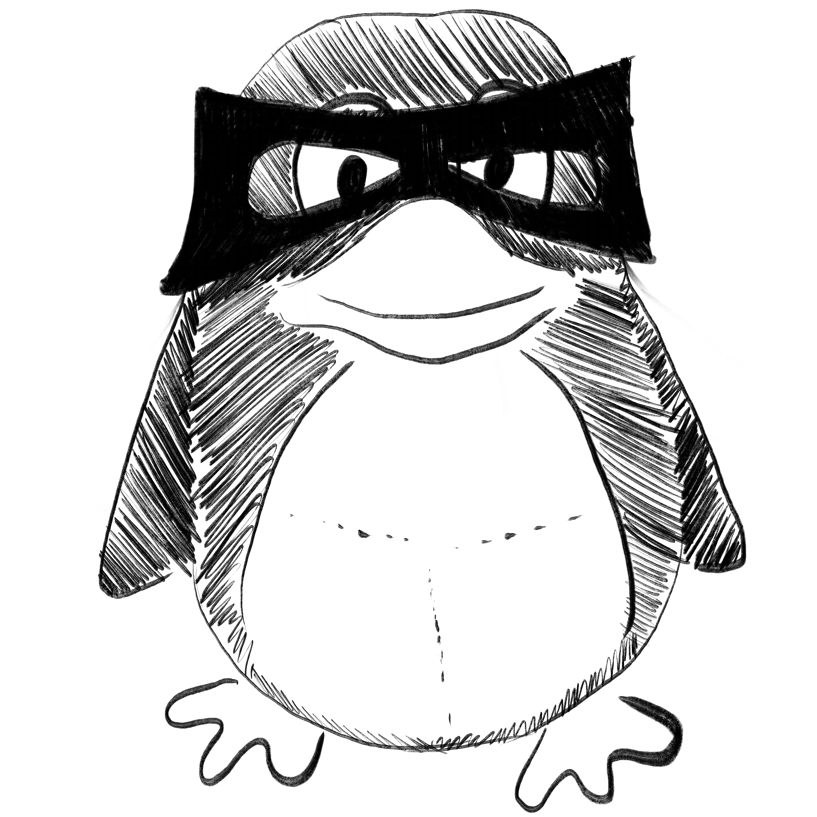
Applying Blockchain Technology in Network Public Opinion Risk Management System in Big Data Environment.
In Computational intelligence and neuroscience
Luo Zhenqing, Zhang Cheng
2023
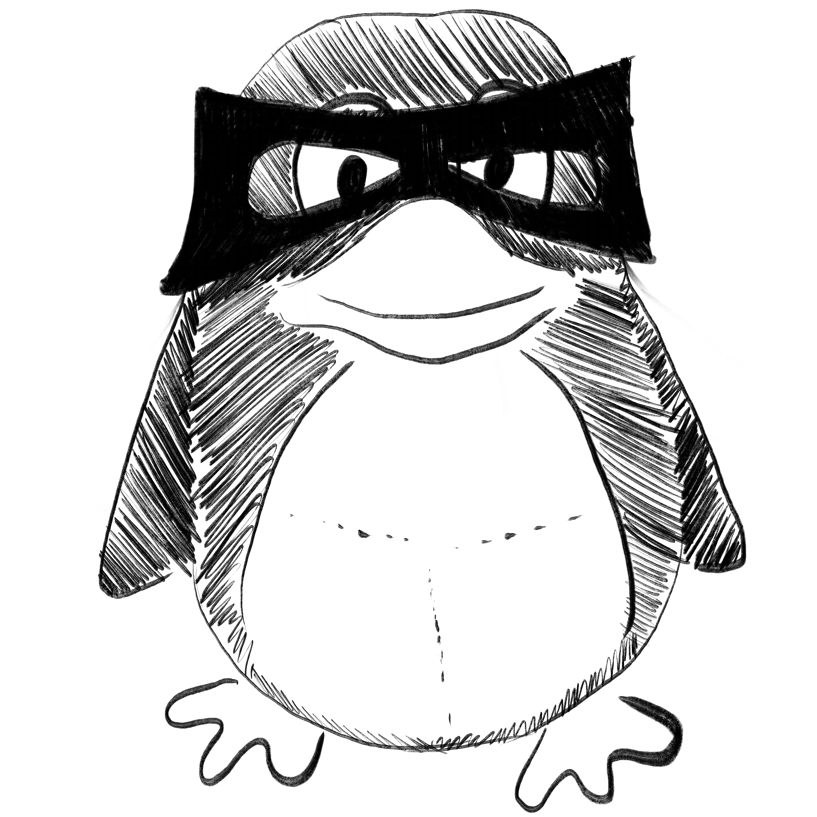
Blood RNA alternative splicing events as diagnostic biomarkers for infectious disease.
In Cell reports methods
Zhang Zijun, Sauerwald Natalie, Cappuccio Antonio, Ramos Irene, Nair Venugopalan D, Nudelman German, Zaslavsky Elena, Ge Yongchao, Gaitas Angelo, Ren Hui, Brockman Joel, Geis Jennifer, Ramalingam Naveen, King David, McClain Micah T, Woods Christopher W, Henao Ricardo, Burke Thomas W, Tsalik Ephraim L, Goforth Carl W, Lizewski Rhonda A, Lizewski Stephen E, Weir Dawn L, Letizia Andrew G, Sealfon Stuart C, Troyanskaya Olga G
2023-Feb-27
RNA splicing, SARS-CoV-2, diagnostic biomarker, host response assays, infectious disease, viral infection
An AI-enabled research support tool for the classification system of COVID-19.
In Frontiers in public health
Tiwari Arti, Bhattacharjee Kamanasish, Pant Millie, Srivastava Shilpa, Snasel Vaclav
2023
Artificial Intelligence, COVID-19, bi-directional LSTM, classification, long short-term memory
Mechanisms influencing the factors of urban built environments and coronavirus disease 2019 at macroscopic and microscopic scales: The role of cities.
In Frontiers in public health
Zhang Longhao, Han Xin, Wu Jun, Wang Lei
2023
COVID-19, computer vision, deep learning, relevance, street view images, urban built environment
PCR-like performance of rapid test with permselective tunable nanotrap.
In Nature communications ; h5-index 260.0
Park Seong Jun, Lee Seungmin, Lee Dongtak, Lee Na Eun, Park Jeong Soo, Hong Ji Hye, Jang Jae Won, Kim Hyunji, Roh Seokbeom, Lee Gyudo, Lee Dongho, Cho Sung-Yeon, Park Chulmin, Lee Dong-Gun, Lee Raeseok, Nho Dukhee, Yoon Dae Sung, Yoo Yong Kyoung, Lee Jeong Hoon
2023-Mar-18
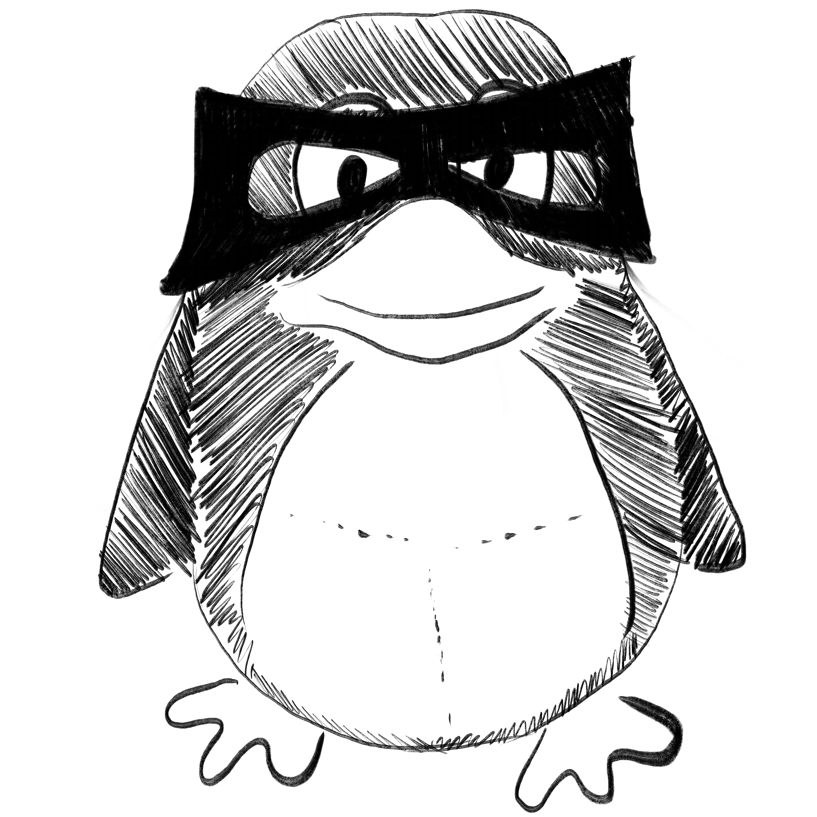
Identification of medicinal plant-based phytochemicals as a potential inhibitor for SARS-CoV-2 main protease (Mpro) using molecular docking and deep learning methods.
In Computers in biology and medicine
Hossain Alomgir, Rahman Md Ekhtiar, Rahman Md Siddiqur, Nasirujjaman Khondokar, Matin Mohammad Nurul, Faruqe Md Omar, Rabbee Muhammad Fazle
2023-Mar-11
Catechin gallate, Deep learning, Main protease, Molecular docking, SARS-CoV-2
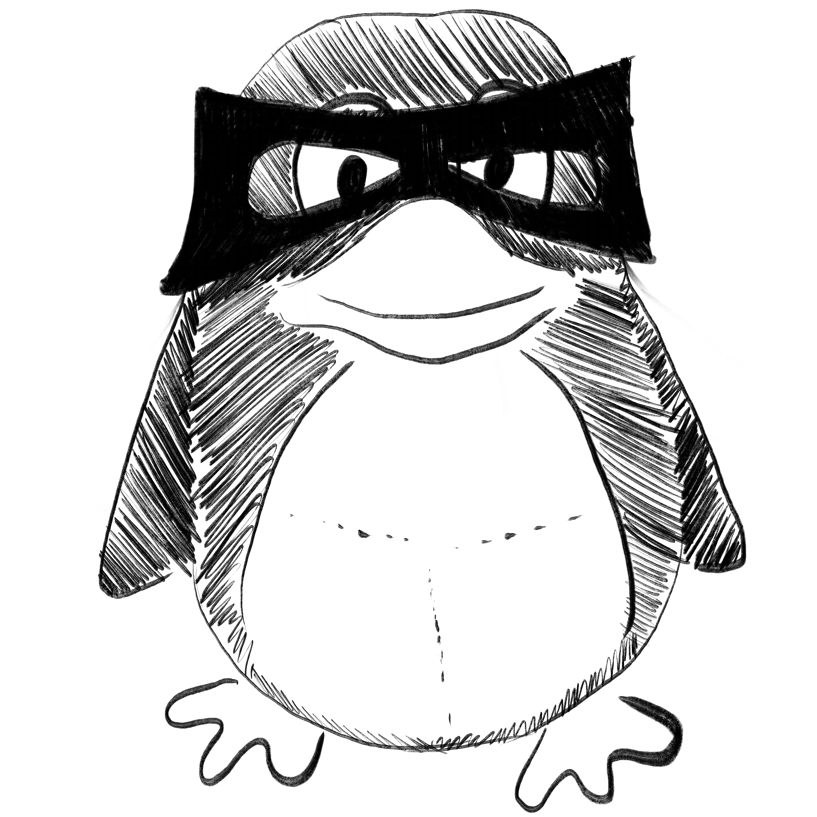
Longitudinal proteomic investigation of COVID-19 vaccination.
In Protein & cell
Wang Yingrui, Zhu Qianru, Sun Rui, Yi Xiao, Huang Lingling, Hu Yifan, Ge Weigang, Gao Huanhuan, Ye Xinfu, Song Yu, Shao Li, Li Yantao, Li Jie, Guo Tiannan, Shi Junping
2023-Feb-06
COVID-19, machine learning, neutralizing antibodies (NAbs), proteomics, vaccination
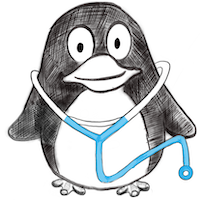
Artificial intelligence-based point-of-care lung ultrasound for screening COVID-19 pneumoniae: Comparison with CT scans.
In PloS one ; h5-index 176.0
BACKGROUND :
METHODS :
RESULTS :
INTERPRETATION :
Kuroda Yumi, Kaneko Tomohiro, Yoshikawa Hitomi, Uchiyama Saori, Nagata Yuichi, Matsushita Yasushi, Hiki Makoto, Minamino Tohru, Takahashi Kazuhisa, Daida Hiroyuki, Kagiyama Nobuyuki
2023
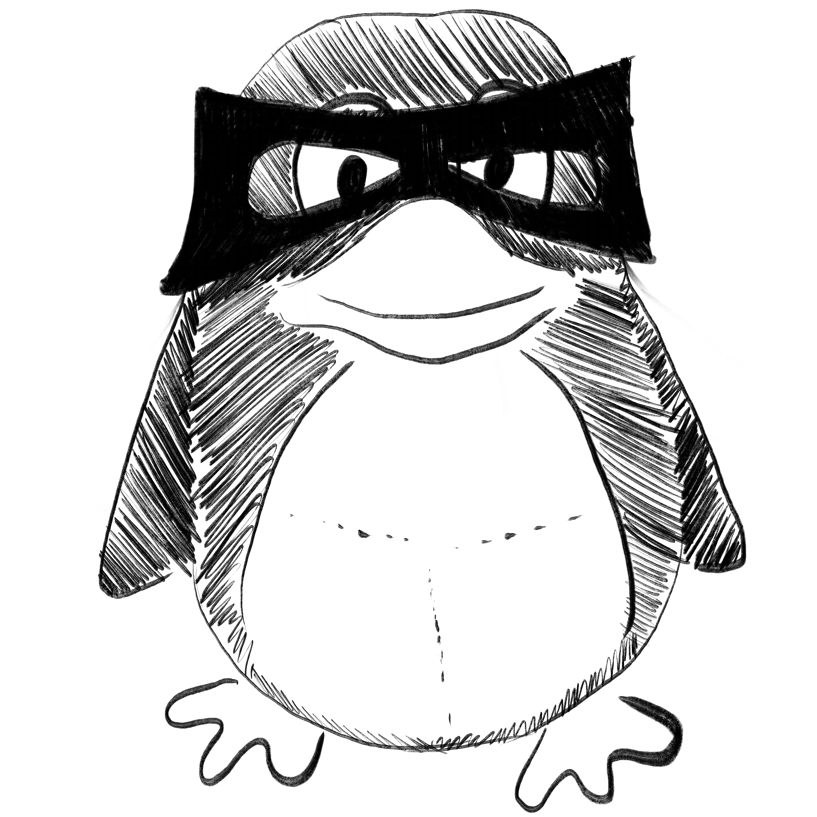
Artificial intelligence-based optimization for chitosan nanoparticles biosynthesis, characterization and in‑vitro assessment of its anti-biofilm potentiality.
In Scientific reports ; h5-index 158.0
El-Naggar Noura El-Ahmady, Dalal Shimaa R, Zweil Amal M, Eltarahony Marwa
2023-Mar-16
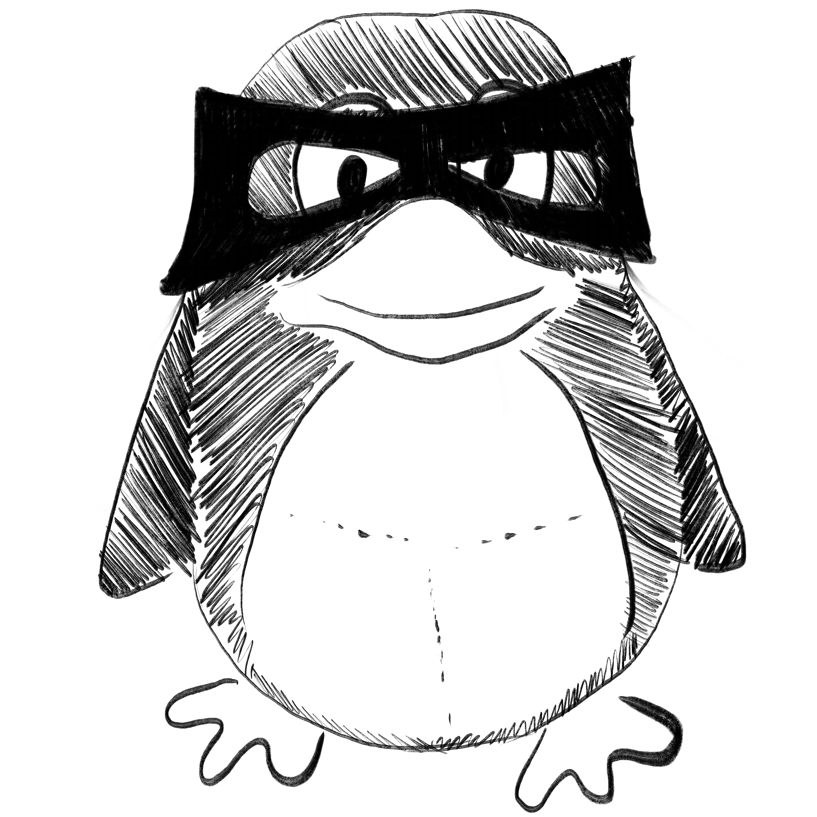
OzNet: A New Deep Learning Approach for Automated Classification of COVID-19 Computed Tomography Scans.
In Big data
Ozaltin Oznur, Yeniay Ozgur, Subasi Abdulhamit
2023-Mar-16
2D-DWT, CNN, COVID-19 CT scans, classification, intensity adjustment
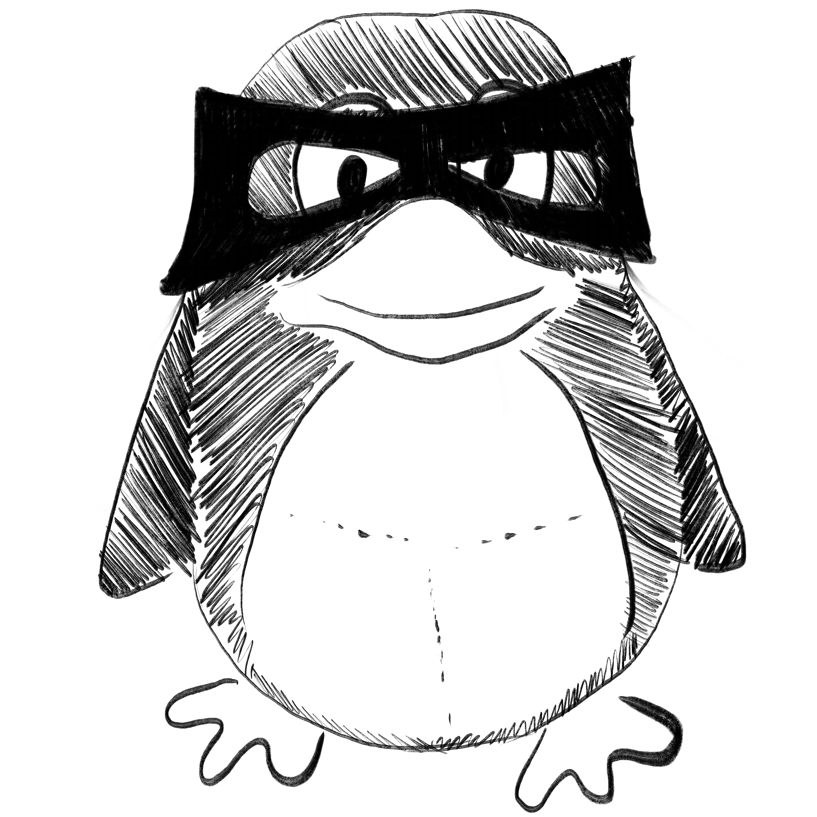
The softening of Chinese digital propaganda: Evidence from the People's Daily Weibo account during the pandemic.
In Frontiers in psychology ; h5-index 92.0
INTRODUCTION :
METHOD :
RESULTS :
DISCUSSION :
Zhang Chang, Zhang Dechun, Shao Hsuan Lei
2023
COVID-19, China, Weibo, propoganda, state-run media
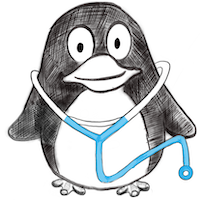
The use of Smart Environments and Robots for Infection Prevention Control: a systematic literature review.
In American journal of infection control ; h5-index 43.0
Piaggio Davide, Zarro Marianna, Pagliara Silvio, Andellini Martina, Almuhini Abdulaziz, Maccaro Alessia, Pecchia Leandro
2023-Mar-14
Infection prevention and control, artificial intelligence, hand hygiene, health 4.0, internet of things, robot
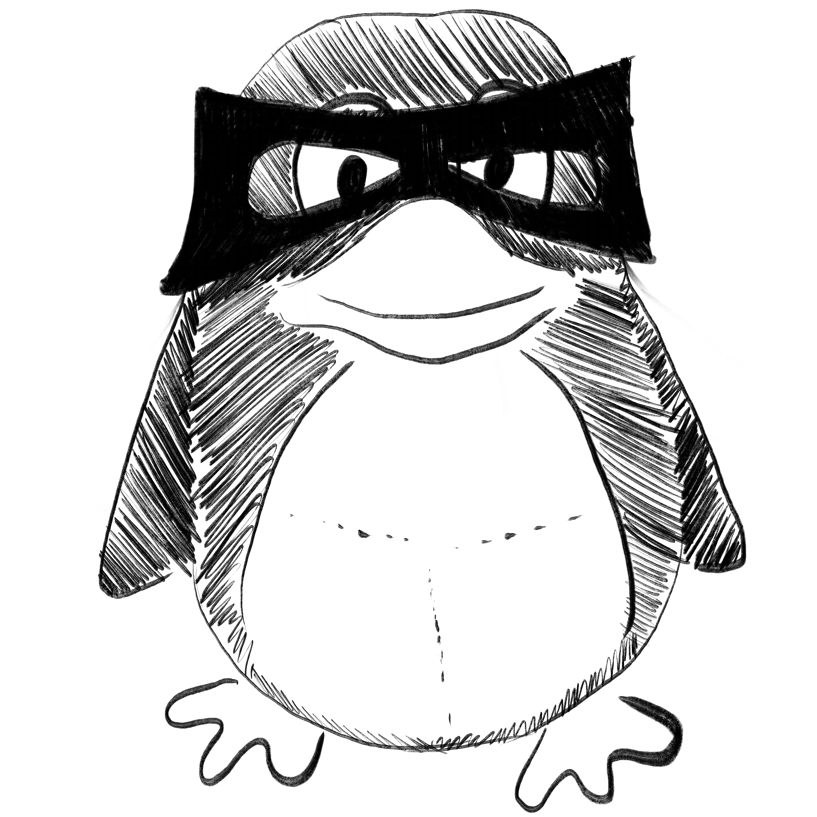
Research on Supply Chain Financial Risk Prevention Based on Machine Learning.
In Computational intelligence and neuroscience
Lei Yang, Qiaoming Hou, Tong Zhao
2023
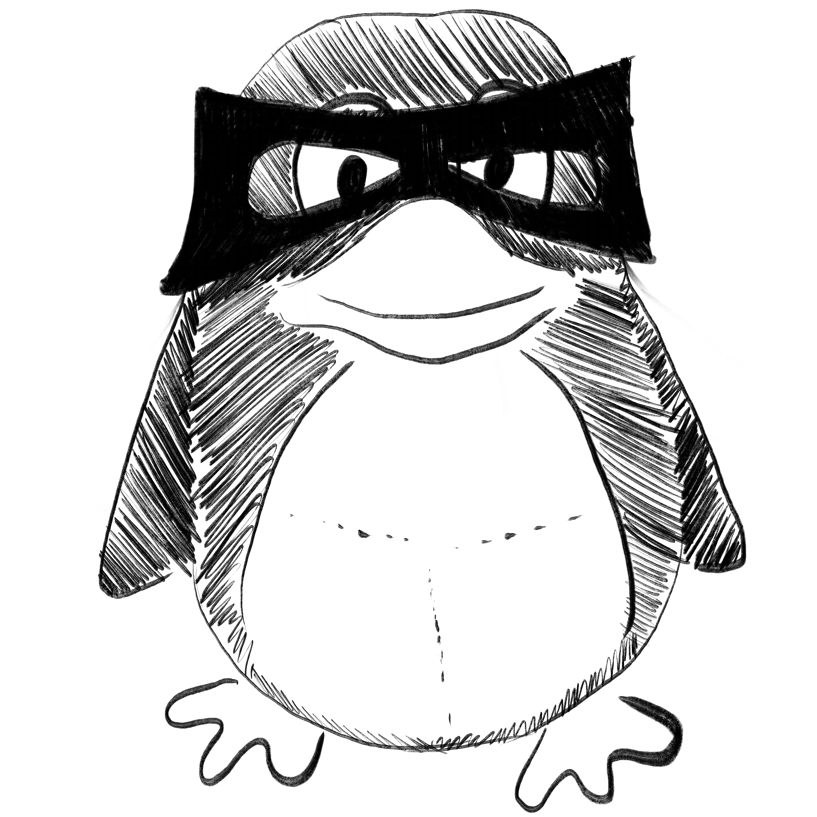
Weibo users and Academia's foci on tourism safety: Implications from institutional differences and digital divide.
In Heliyon
Zeng Liyun, Li Rita Yi Man, Zeng Huiling
2023-Mar
Artificial intelligence, Bibliometrics, Comparative analysis, Digital divide, Information asymmetry, Tourism safety, Web of science, Weibo
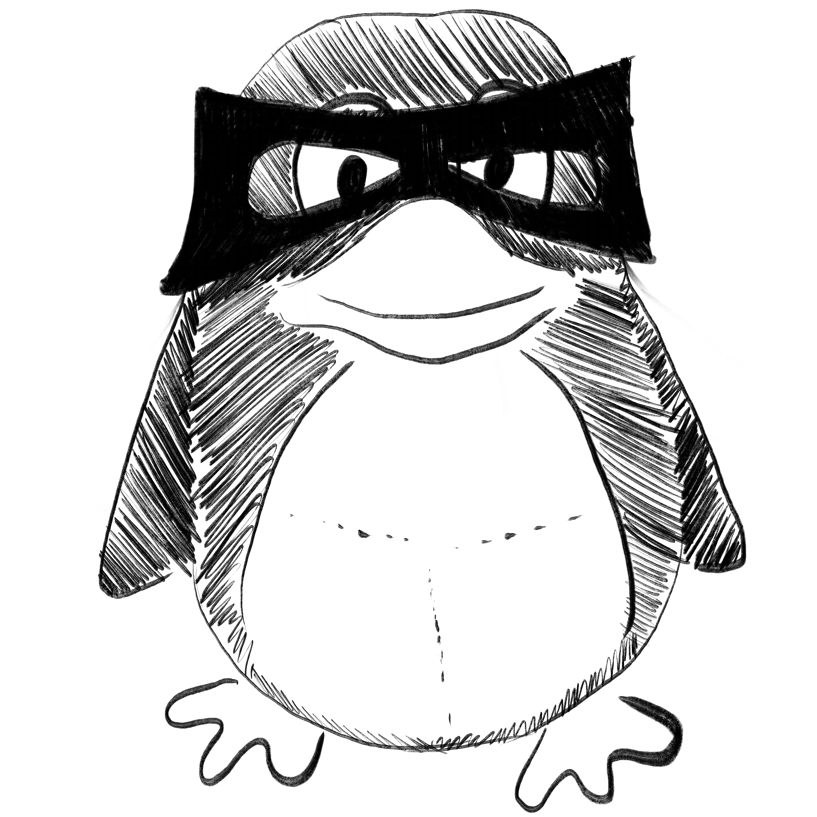
Multi-weight susceptible-infected model for predicting COVID-19 in China.
In Neurocomputing
Zhang Jun, Zheng Nanning, Liu Mingyu, Yao Dingyi, Wang Yusong, Wang Jianji, Xin Jingmin
2023-May-14
COVID-19 prediction, Data processing, Epidemic model, Multi-weight susceptible-infected model
CCTCOVID: COVID-19 detection from chest X-ray images using Compact Convolutional Transformers.
In Frontiers in public health
Marefat Abdolreza, Marefat Mahdieh, Hassannataj Joloudari Javad, Nematollahi Mohammad Ali, Lashgari Reza
2023
COVID-19, Compact Convolutional Transformers, Convolutional Neural Networks, deep learning, vision transformers
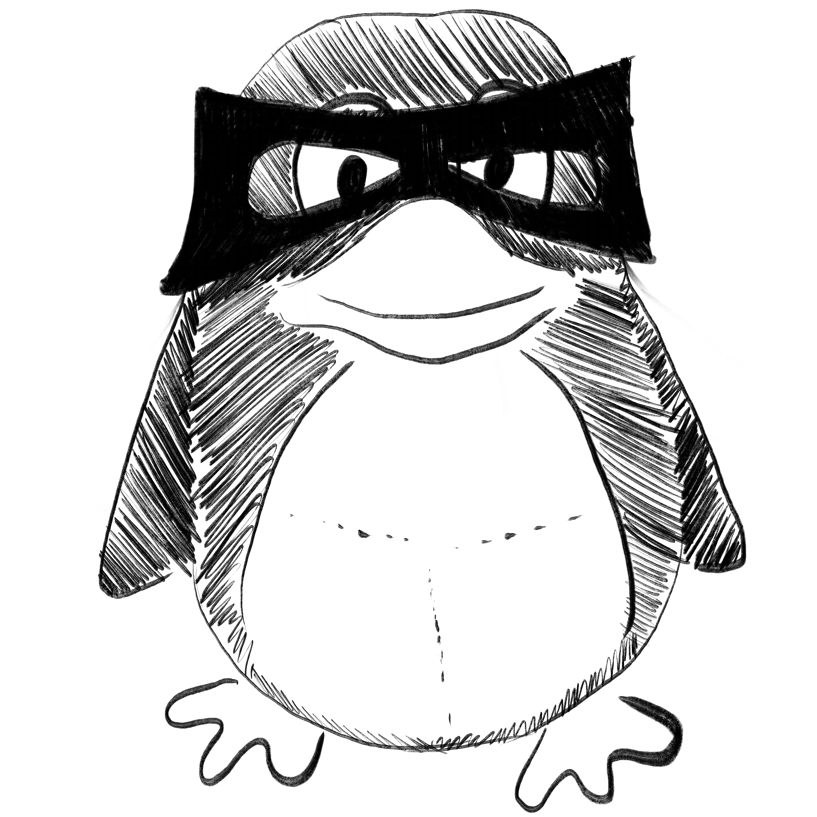
Artificial intelligence evaluation of COVID-19 restrictions and speech therapy effects on the autistic children's behavior.
In Scientific reports ; h5-index 158.0
Sabzevari Fereshteh, Amelirad Omid, Moradi Zohre, Habibi Mostafa
2023-Mar-15
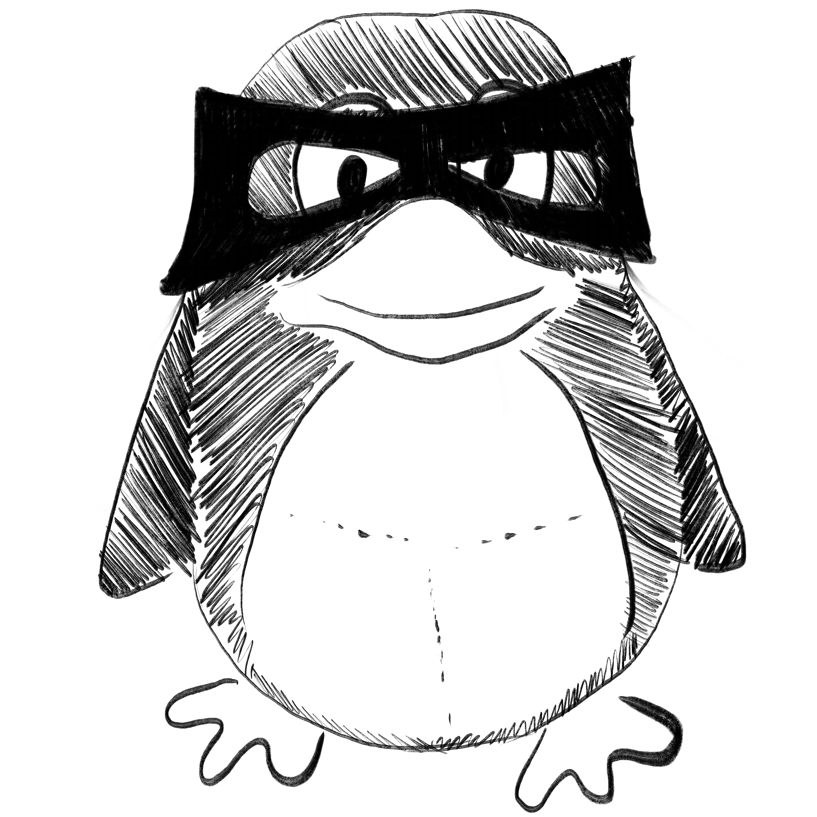
IRCM-Caps: An X-ray image detection method for COVID-19.
In The clinical respiratory journal
OBJECTIVE :
METHODS :
RESULT :
CONCLUSION :
Qiu Shuo, Ma Jinlin, Ma Ziping
2023-Mar-15
CNN, COVID-19, CapseNet, X-ray, cascade network, deep learning
Investigation of liquid biopsy analytes in peripheral blood of individuals after SARS-CoV-2 infection.
In EBioMedicine
BACKGROUND :
METHODS :
FINDINGS :
INTERPRETATION :
FUNDING :
Qi Elizabeth, Courcoubetis George, Liljegren Emmett, Herrera Ergueen, Nguyen Nathalie, Nadri Maimoona, Ghandehari Sara, Kazemian Elham, Reckamp Karen L, Merin Noah M, Merchant Akil, Mason Jeremy, Figueiredo Jane C, Shishido Stephanie N, Kuhn Peter
2023-Mar-13
COVID-19, Liquid biopsy, Long COVID, Post-COVID sequelae, Post-acute COVID-19 syndrome (PACS), SARS-CoV-2
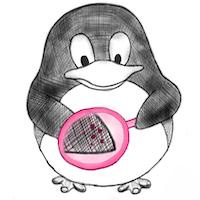
Development and Clinical Evaluation of an Artificial Intelligence Support Tool for Improving Telemedicine Photo Quality.
In JAMA dermatology ; h5-index 54.0
IMPORTANCE :
OBJECTIVE :
DESIGN, SETTING, AND PARTICIPANTS :
INTERVENTIONS :
MAIN OUTCOMES AND MEASURES :
RESULTS :
CONCLUSIONS AND RELEVANCE :
Vodrahalli Kailas, Ko Justin, Chiou Albert S, Novoa Roberto, Abid Abubakar, Phung Michelle, Yekrang Kiana, Petrone Paige, Zou James, Daneshjou Roxana
2023-Mar-15
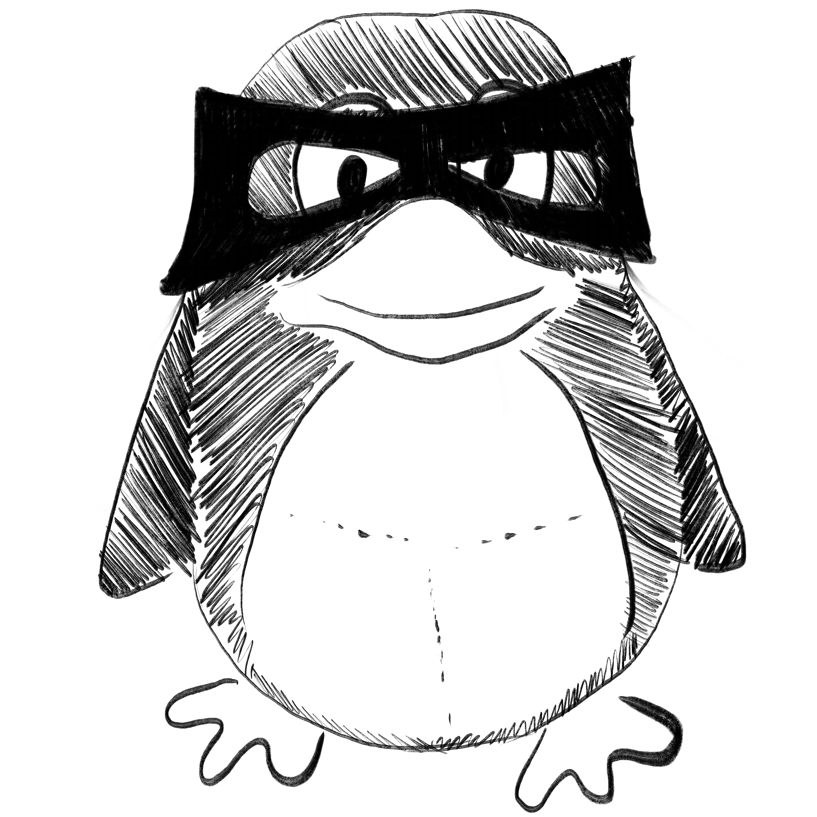
A methylation clock model of mild SARS-CoV-2 infection provides insight into immune dysregulation.
In Molecular systems biology
Mao Weiguang, Miller Clare M, Nair Venugopalan D, Ge Yongchao, Amper Mary Anne S, Cappuccio Antonio, George Mary-Catherine, Goforth Carl W, Guevara Kristy, Marjanovic Nada, Nudelman German, Pincas Hanna, Ramos Irene, Sealfon Rachel S G, Soares-Schanoski Alessandra, Vangeti Sindhu, Vasoya Mital, Weir Dawn L, Zaslavsky Elena, Kim-Schulze Seunghee, Gnjatic Sacha, Merad Miriam, Letizia Andrew G, Troyanskaya Olga G, Sealfon Stuart C, Chikina Maria
2023-Mar-15
DNA methylation, SARS-CoV-2, machine learning model, temporal dynamics, trained immunity
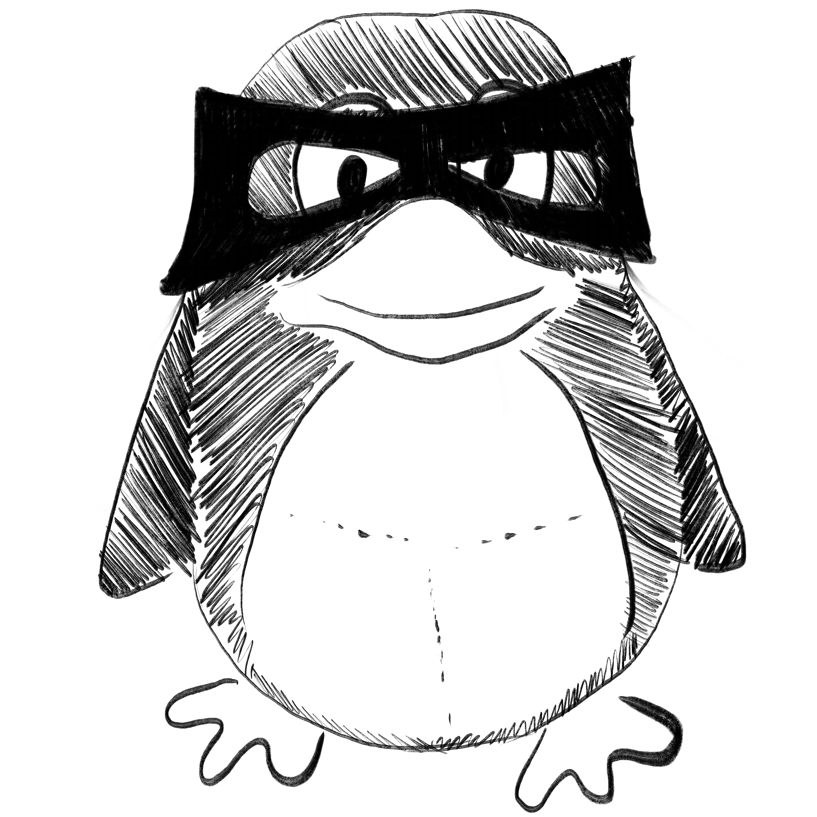
Effect of ferritin, INR, and D-dimer immunological parameters levels as predictors of COVID-19 mortality: A strong prediction with the decision trees.
In Heliyon
BACKGROUND AND OBJECTIVE :
MATERIAL AND METHOD :
RESULTS :
CONCLUSIONS :
Huyut Mehmet Tahir, Huyut Zübeyir
2023-Mar
Artificial intelligence, CHAID decision Trees, COVID-19, Coagulation tests, Ferritin, Immunological tests, Machine learning, Mortality risk biomarkers
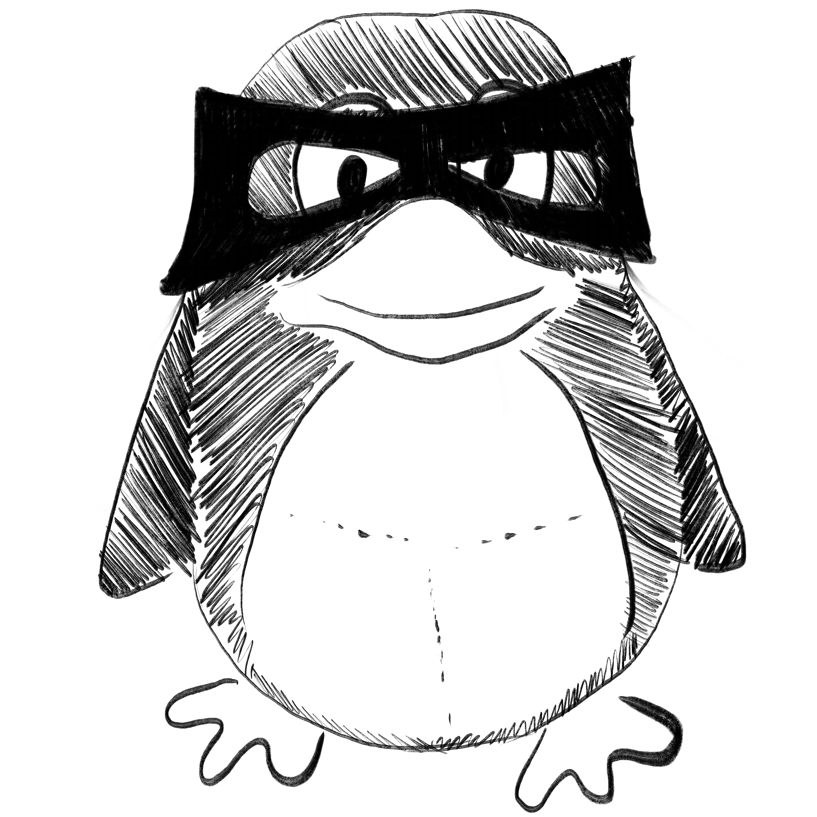
Comprehensive analysis of clinical data for COVID-19 outcome estimation with machine learning models.
In Biomedical signal processing and control
Morís Daniel I, de Moura Joaquim, Marcos Pedro J, Rey Enrique Míguez, Novo Jorge, Ortega Marcos
2023-Jul
COVID-19, Classification, Clinical data, Feature selection, Machine learning
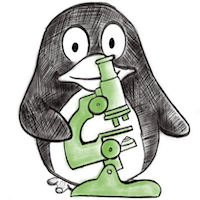
Paving New Roads Using Allium sativum as a Repurposed Drug and Analyzing its Antiviral Action Using Artificial Intelligence Technology.
In Iranian journal of pharmaceutical research : IJPR
CONTEXT :
OBJECTIVES :
METHOD :
RESULTS :
CONCLUSIONS :
Atoum Manar Fayiz, Padma Kanchi Ravi, Don Kanchi Ravi
2022-Dec
Allium sativum, Flavonoid, Immunomodulatory, SARS-CoV-2
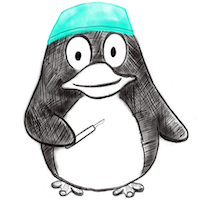
An artificial intelligence approach for predicting death or organ failure after hospitalization for COVID-19: development of a novel risk prediction tool and comparisons with ISARIC-4C, CURB-65, qSOFA, and MEWS scoring systems.
In Respiratory research ; h5-index 45.0
BACKGROUND :
METHODS :
RESULTS :
CONCLUSION :
Kwok Stephen Wai Hang, Wang Guanjin, Sohel Ferdous, Kashani Kianoush B, Zhu Ye, Wang Zhen, Antpack Eduardo, Khandelwal Kanika, Pagali Sandeep R, Nanda Sanjeev, Abdalrhim Ahmed D, Sharma Umesh M, Bhagra Sumit, Dugani Sagar, Takahashi Paul Y, Murad Mohammad H, Yousufuddin Mohammed
2023-Mar-13
COVID-19, Machine learning algorithms, Mortality, Organ failure, Prediction models
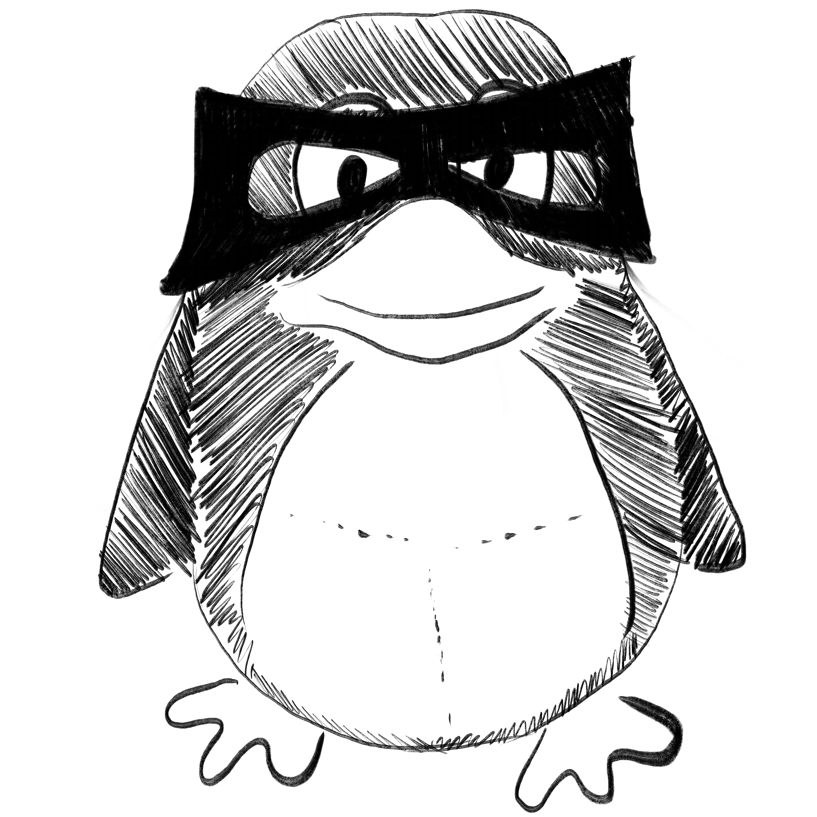
Benchmarking machine learning robustness in Covid-19 genome sequence classification.
In Scientific reports ; h5-index 158.0
Ali Sarwan, Sahoo Bikram, Zelikovsky Alexander, Chen Pin-Yu, Patterson Murray
2023-Mar-13
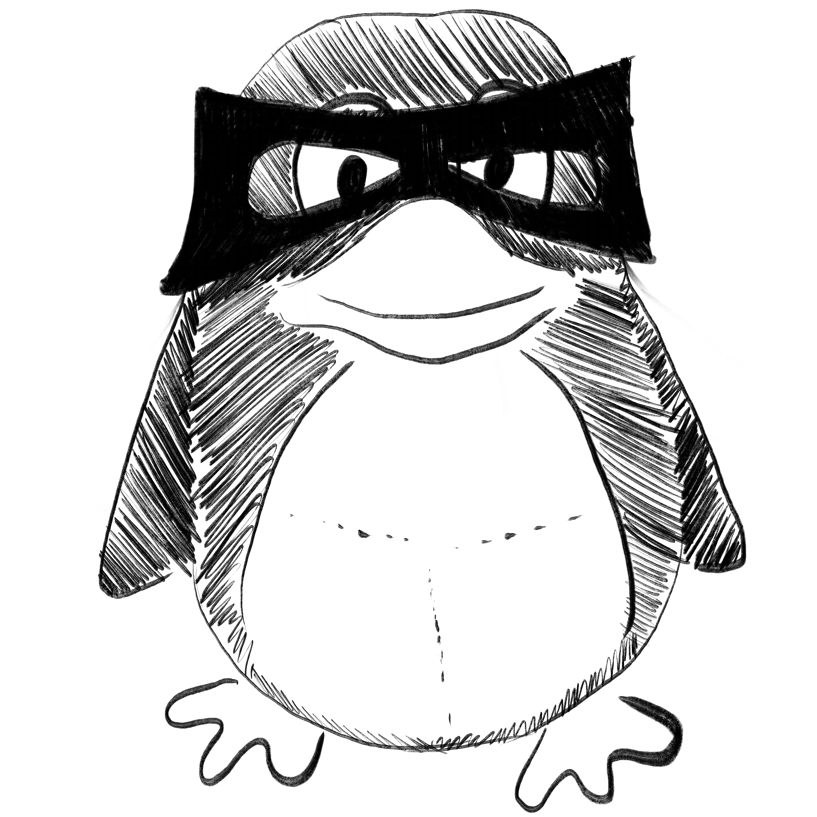
The predictive model for COVID-19 pandemic plastic pollution by using deep learning method.
In Scientific reports ; h5-index 158.0
Nanehkaran Yaser A, Licai Zhu, Azarafza Mohammad, Talaei Sona, Jinxia Xu, Chen Junde, Derakhshani Reza
2023-Mar-13
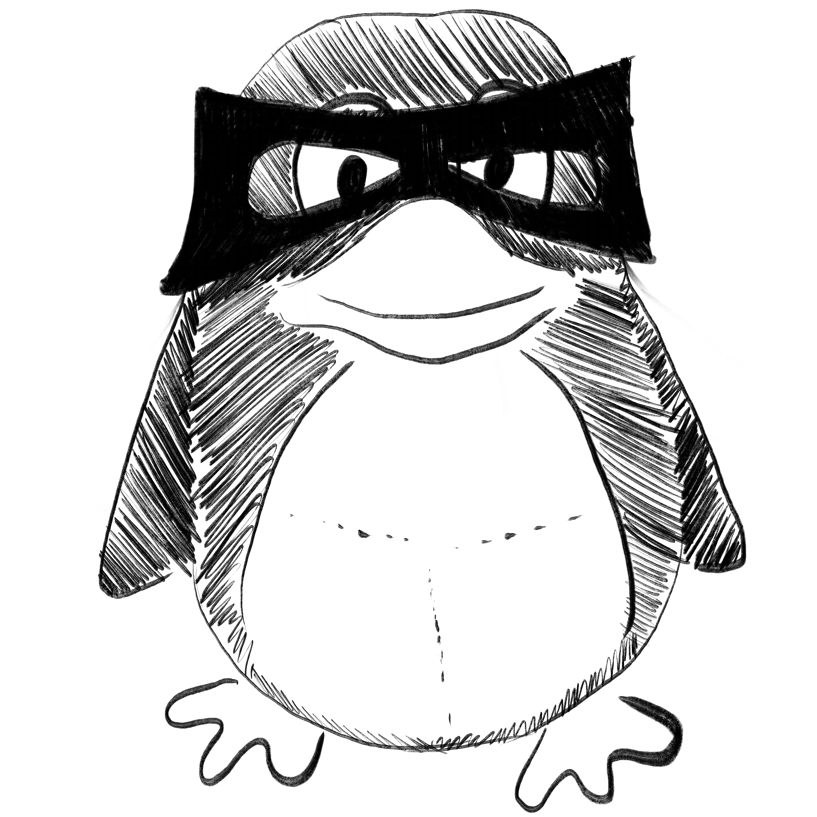
Analysis on factors affecting tourist involvement in coffee tourism after the COVID-19 pandemic in Thailand.
In F1000Research
Madhyamapurush Warach
2022
COVID-19 pandemic, Coffee Tourism, Foreign Tourists, Stochastic Neuro-Fuzzy Decision Tree (SNF-DT)., Tourist Behaviors
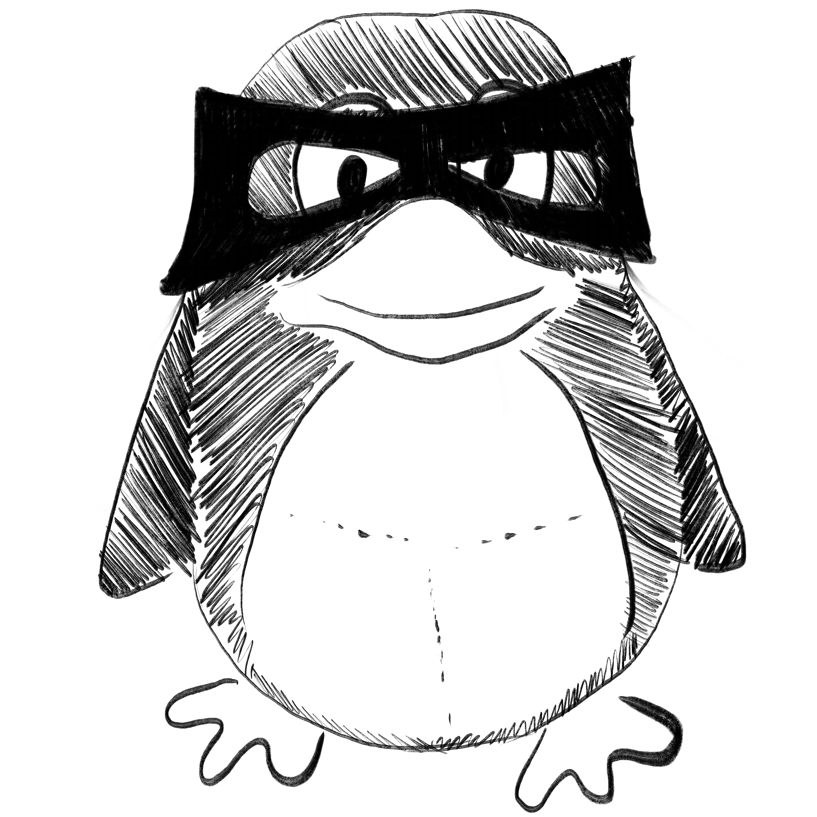
Situation-Aware BDI Reasoning to Detect Early Symptoms of Covid 19 Using Smartwatch.
In IEEE sensors journal
Saleem Kiran, Saleem Misbah, Ahmad Rana Zeeshan, Javed Abdul Rehman, Alazab Mamoun, Gadekallu Thippa Reddy, Suleman Ahmad
2023-Jan
Covid-19, NetLogo, Situation-awareness, ambient intelligence, belief-desire-intention (BDI), healthcare
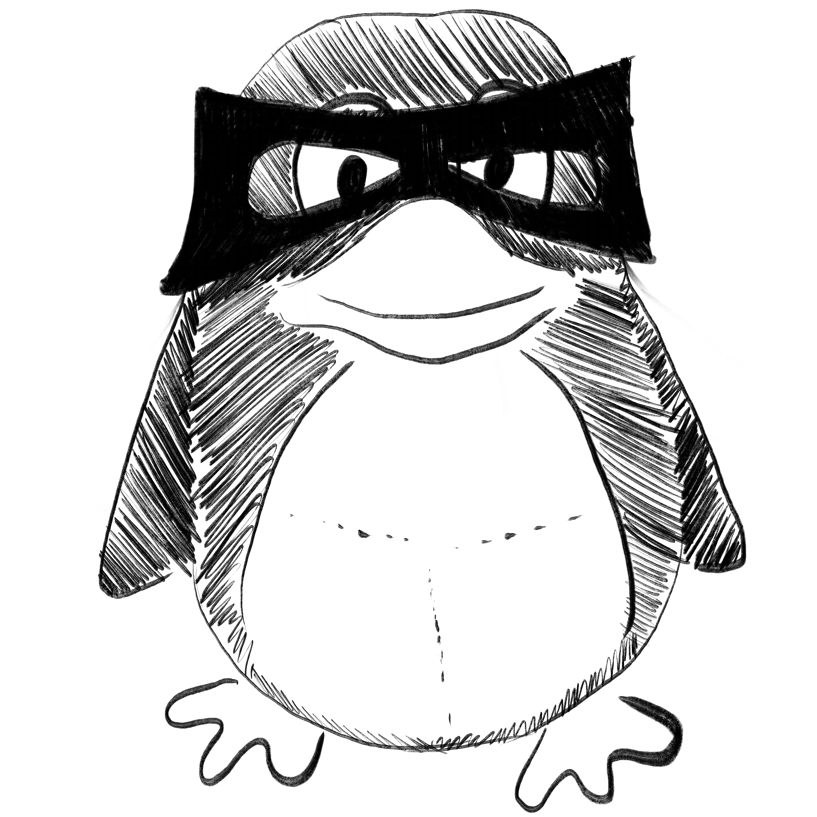
i-Sheet: A Low-Cost Bedsheet Sensor for Remote Diagnosis of Isolated Individuals.
In IEEE sensors journal
Tapwal Riya, Misra Sudip, Deb Pallav Kumar
2023-Jan
Artificial intelligence, COVID-19, remote monitoring, sensors, smart bedsheet
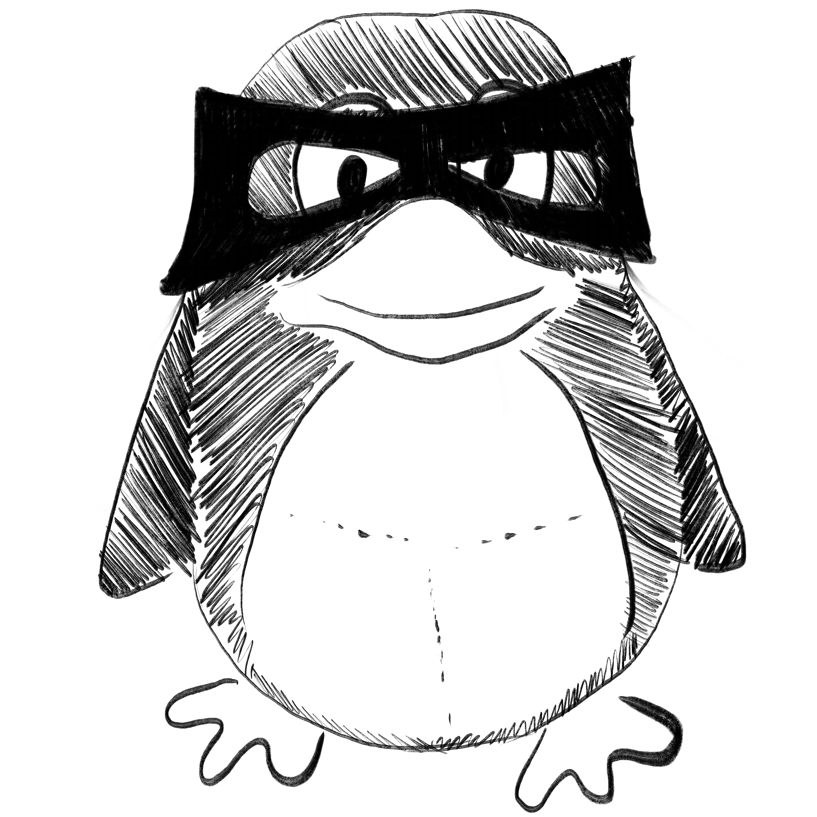
The role of the mass vaccination programme in combating the COVID-19 pandemic: An LSTM-based analysis of COVID-19 confirmed cases.
In Heliyon
Hansun Seng, Charles Vincent, Gherman Tatiana
2023-Mar
COVID-19, Confirmed cases, Deep learning, LSTM, Mass vaccination
Exploring the impact of sentiment on multi-dimensional information dissemination using COVID-19 data in China.
In Computers in human behavior ; h5-index 125.0
Luo Han, Meng Xiao, Zhao Yifei, Cai Meng
2023-Jul
COVID-19, Emotional response, Information authority, Information dissemination, Information richness, Topic influence
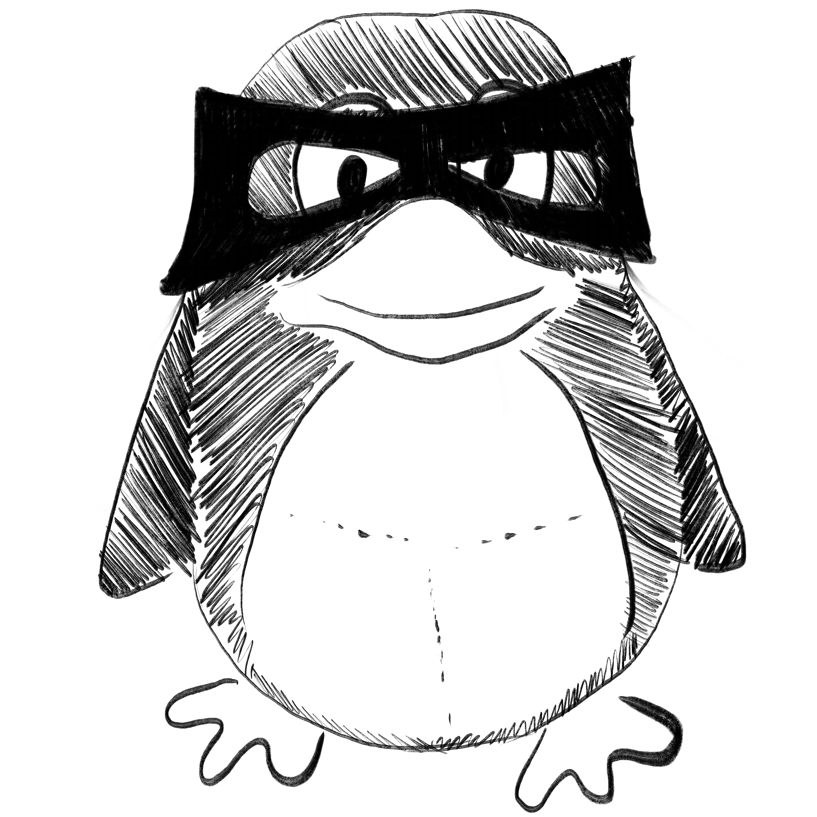
Pathogen-driven cancers from a structural perspective: Targeting host-pathogen protein-protein interactions.
In Frontiers in oncology
Ozdemir Emine Sila, Nussinov Ruth
2023
artificial intelligence, cancer therapeutics, drug discovery, host-pathogen interactions, machine learning, protein-protein interactions
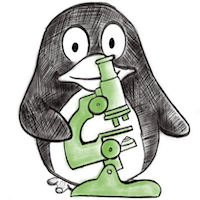
Artificial intelligence-based HDX (AI-HDX) prediction reveals fundamental characteristics to protein dynamics: Mechanisms on SARS-CoV-2 immune escape.
In iScience
Yu Jiali, Uzuner Ugur, Long Bin, Wang Zachary, Yuan Joshua S, Dai Susie Y
2023-Apr-21
Immunology, Virology
An anti-infodemic virtual center for the Americas.
In Revista panamericana de salud publica = Pan American journal of public health
Brooks Ian, D’Agostino Marcelo, Marti Myrna, McDowell Kate, Mejia Felipe, Betancourt-Cravioto Miguel, Gatzke Lisa, Hicks Elaine, Kyser Rebecca, Leicht Kevin, Pereira Dos Santos Eliane, Saw Jessica Jia-Wen, Tomio Ailin, Garcia Saiso Sebastian
2023
Americas, COVID-19, Public Health Informatics, artificial intelligence, communication, social media
IPs-GRUAtt: An attention-based bidirectional gated recurrent unit network for predicting phosphorylation sites of SARS-CoV-2 infection.
In Molecular therapy. Nucleic acids
Zhang Guiyang, Tang Qiang, Feng Pengmian, Chen Wei
2023-Jun-13
MT: Bioinformatics, SARS-CoV-2, attention mechanism, bidirectional gated recurrent unit, deep learning, interpretation, phosphorylation
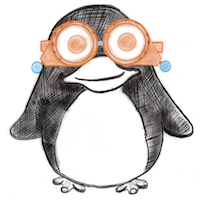
Implementation of deep learning artificial intelligence in vision-threatening disease screenings for an underserved community during COVID-19.
In Journal of telemedicine and telecare ; h5-index 28.0
INTRODUCTION :
METHODS :
RESULTS :
DISCUSSION :
Zhu Aretha, Tailor Priya, Verma Rashika, Zhang Isis, Schott Brian, Ye Catherine, Szirth Bernard, Habiel Miriam, Khouri Albert S
2023-Mar-13
COVID-19, Tele-ophthalmology, deep learning artificial intelligence, vision screenings
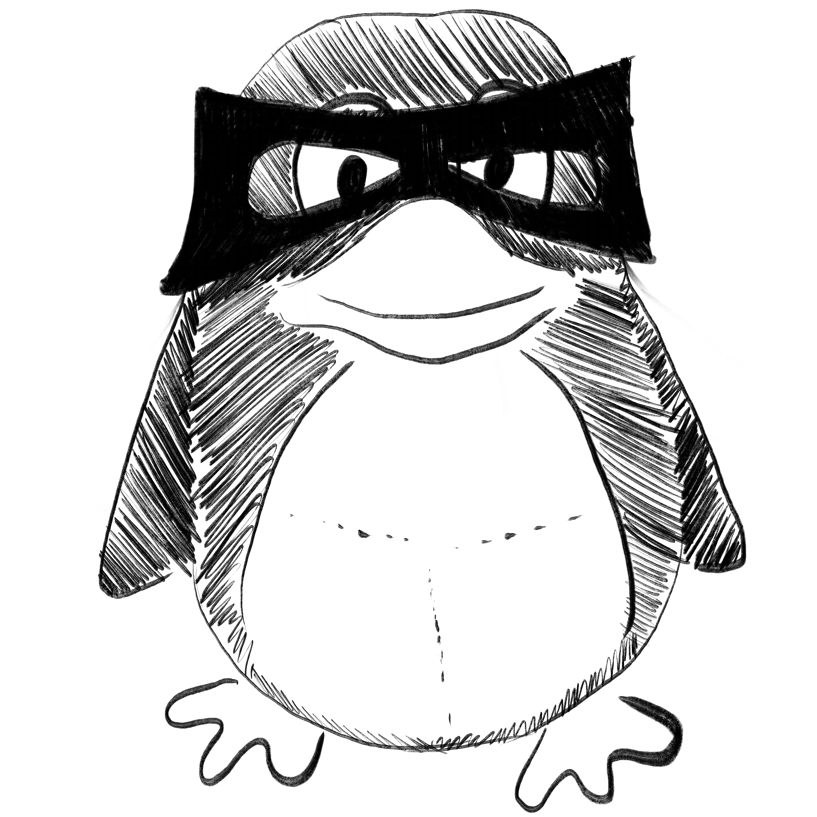
Computational prediction of interactions between Paxlovid and prescription drugs.
In Proceedings of the National Academy of Sciences of the United States of America
Kim Yeji, Ryu Jae Yong, Kim Hyun Uk, Lee Sang Yup
2023-Mar-21
COVID-19, DeepDDI2, Paxlovid, drug interactions
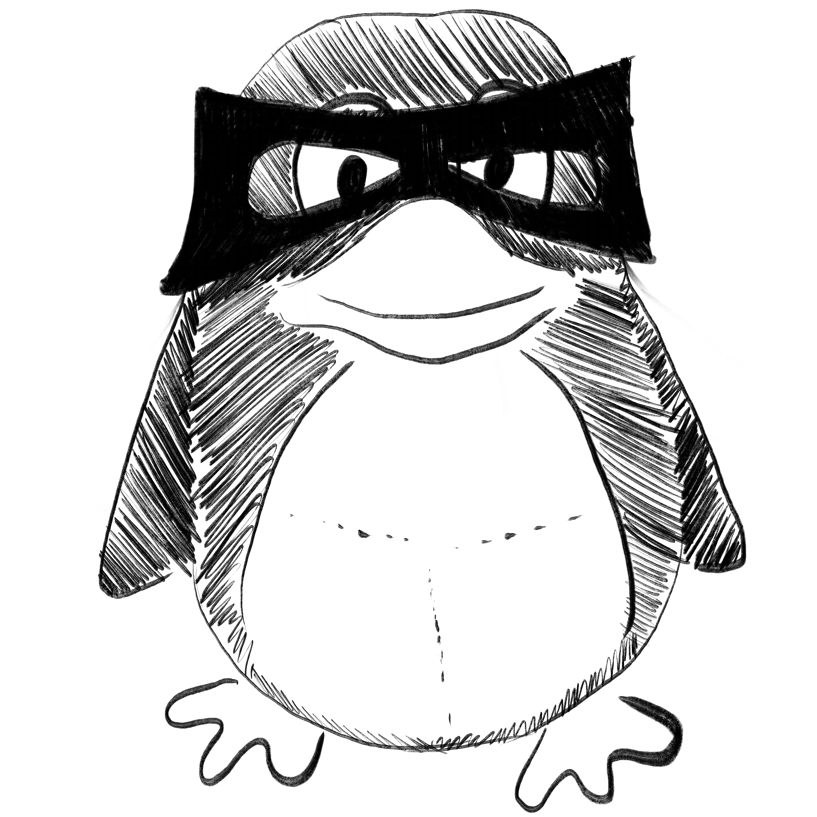
Big data and infectious disease epidemiology: A bibliometric analysis and research agenda.
In Interactive journal of medical research
BACKGROUND :
OBJECTIVE :
METHODS :
RESULTS :
CONCLUSIONS :
Amusa Lateef Babatunde, Twinomurinzi Hossana, Phalane Edith, Phaswana-Mafuya Refilwe Nancy
2022-Nov-29
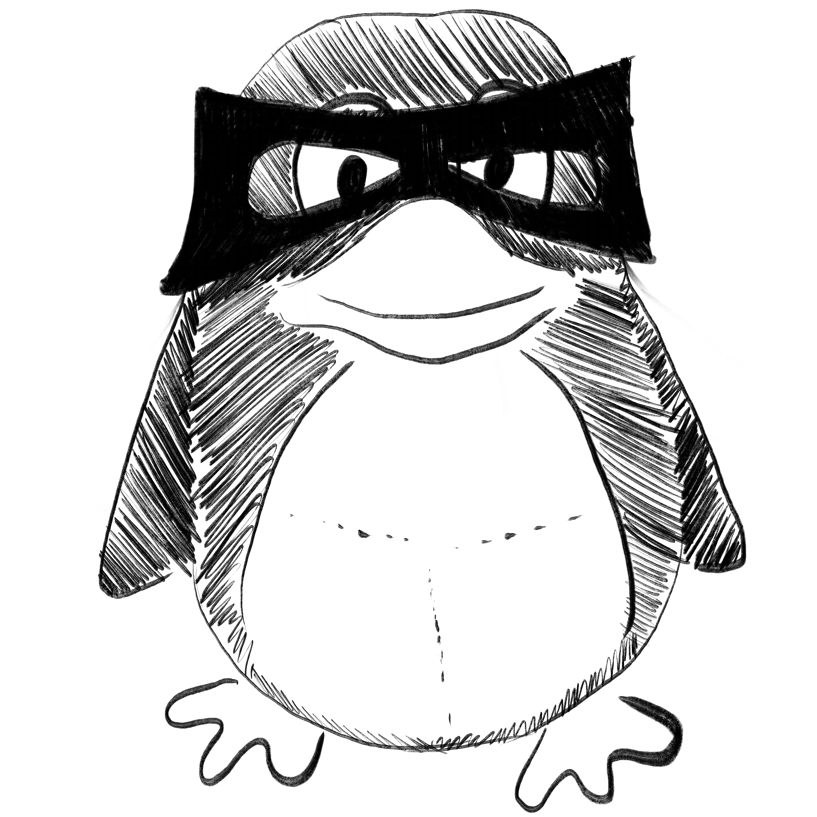
Minimizing Viral Transmission in COVID-19 Like Pandemics: Technologies, Challenges, and Opportunities.
In IEEE sensors journal
Nisar Shibli, Wakeel Abdul, Tahir Wania, Tariq Muhammad
2023-Jan
COVID-19, artificial intelligence, cellular forensics, hidden patterns, pandemic, tracking systems
A machine learning analysis of correlates of mortality among patients hospitalized with COVID-19.
In Scientific reports ; h5-index 158.0
Baker Timothy B, Loh Wei-Yin, Piasecki Thomas M, Bolt Daniel M, Smith Stevens S, Slutske Wendy S, Conner Karen L, Bernstein Steven L, Fiore Michael C
2023-Mar-11
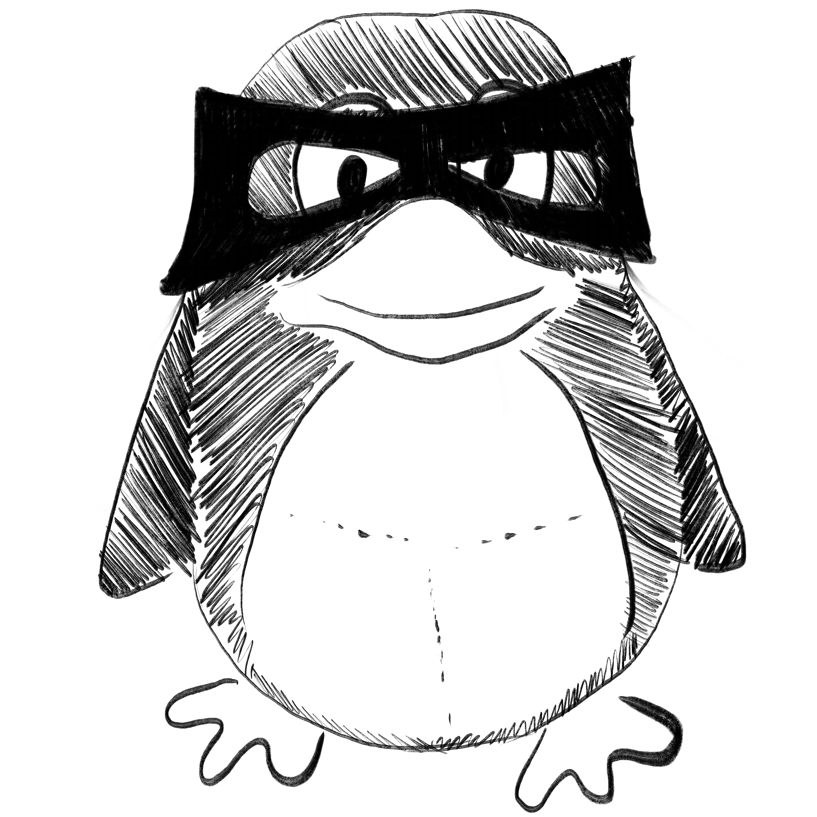
A novel use of an artificially intelligent Chatbot and a live, synchronous virtual question-and answer session for fellowship recruitment.
In BMC medical education
INTRODUCTION :
METHODS :
RESULTS :
CONCLUSION :
Yi Peter K, Ray Neil D, Segall Noa
2023-Mar-11
Artificial intelligence, Graduate medical education, Innovation and technology, Recruitment, Social media
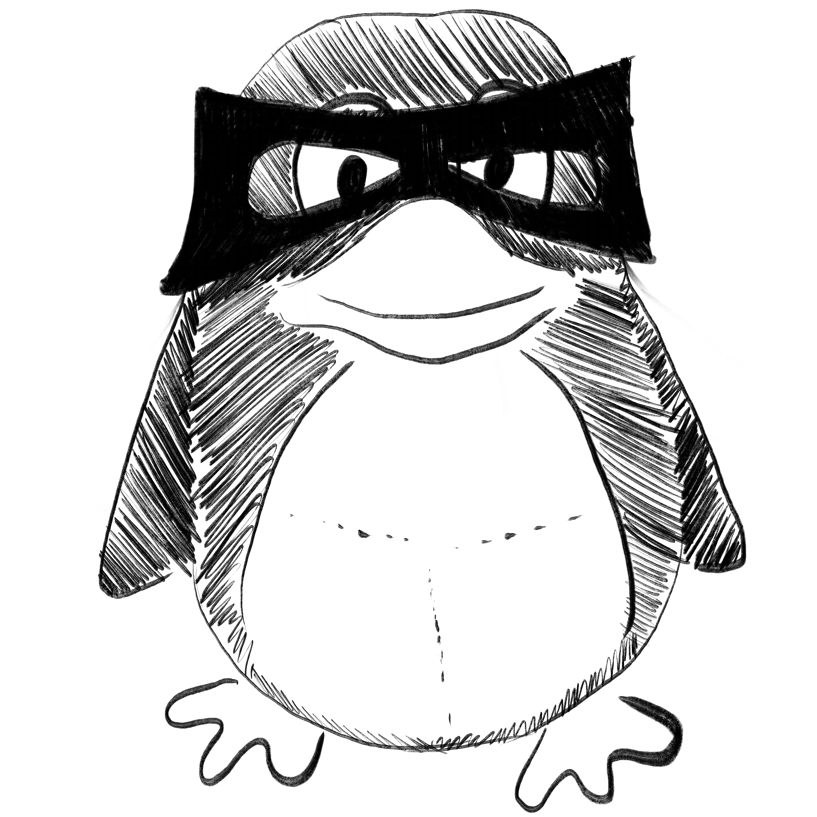
New proposal of viral genome representation applied in the classification of SARS-CoV-2 with deep learning.
In BMC bioinformatics
BACKGROUND :
RESULTS :
CONCLUSIONS :
de Souza Luísa C, Azevedo Karolayne S, de Souza Jackson G, Barbosa Raquel de M, Fernandes Marcelo A C
2023-Mar-11
CGR DFT, COVID-19, Deep learning, GSP, SARS-CoV-2
A hybrid deep learning approach for COVID-19 detection based on genomic image processing techniques.
In Scientific reports ; h5-index 158.0
Hammad Muhammed S, Ghoneim Vidan F, Mabrouk Mai S, Al-Atabany Walid I
2023-Mar-10
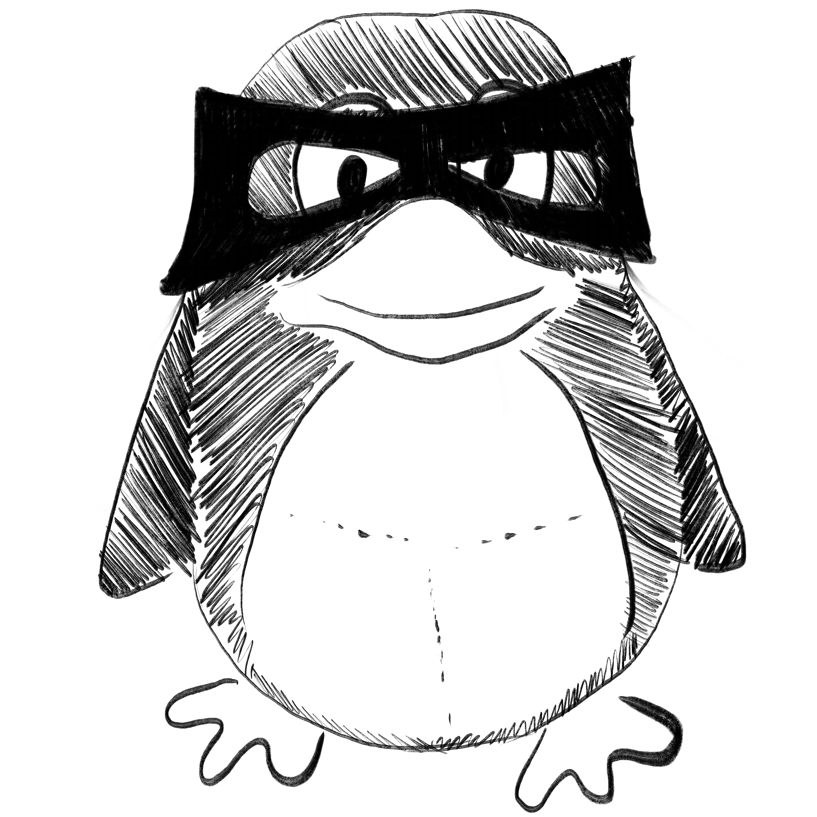
A generalized distributed delay model of COVID-19: An endemic model with immunity waning.
In Mathematical biosciences and engineering : MBE
Iyaniwura Sarafa A, Musa Rabiu, Kong Jude D
2023-Jan-12
** COVID-19 , SARS-CoV-2 , distributed delay equations , endemic model , global stability , immunity waning , linear chain trick , vaccination **
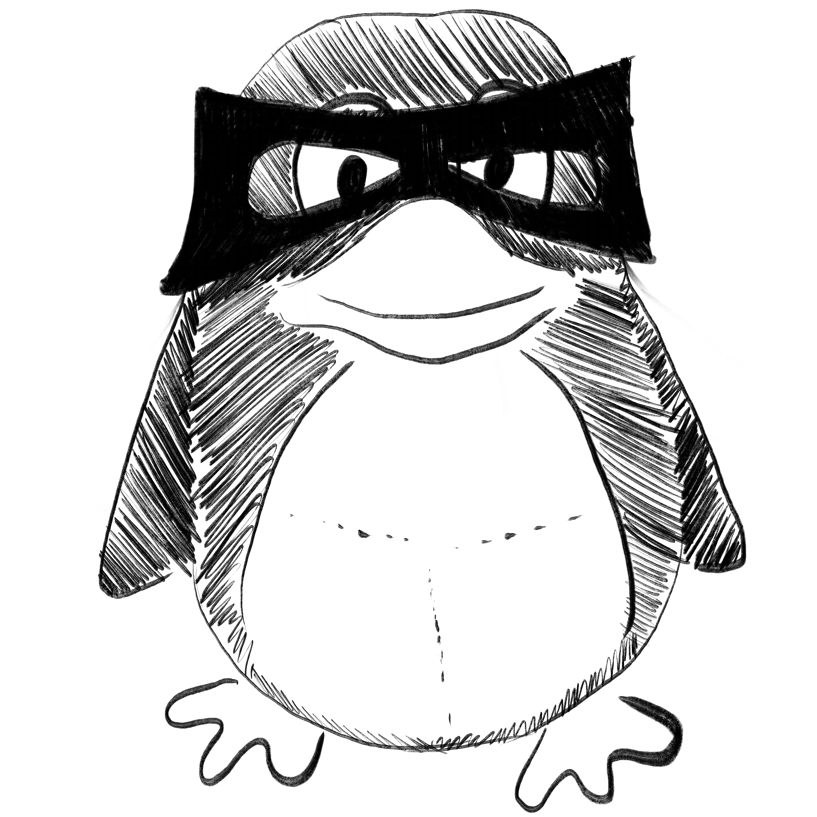
Research of mortality risk prediction based on hospital admission data for COVID-19 patients.
In Mathematical biosciences and engineering : MBE
Shen Qian
2023-Jan-11
** COVID-19 , death risk feature , emergency triage strategy , ensemble learning , machine learning , tree model **
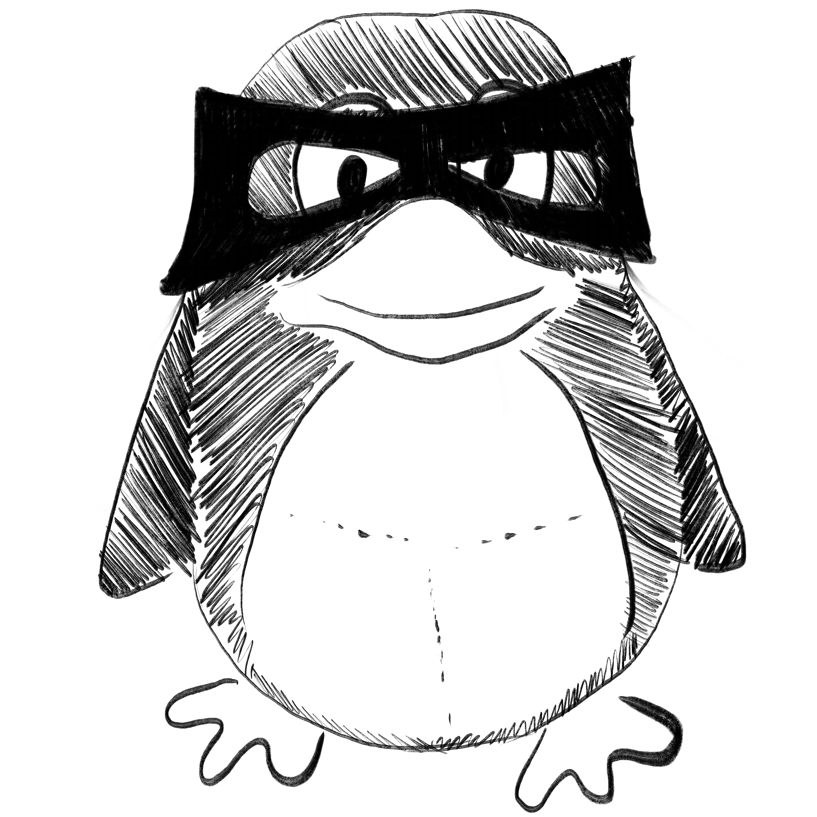
An application of artificial intelligence for investigating the effect of COVID-19 lockdown on three-dimensional temperature variation in equatorial Africa.
In Geoscience frontiers
Okoh Daniel, Onuorah Loretta, Rabiu Babatunde, Obafaye Aderonke, Audu Dauda, Yusuf Najib, Owolabi Oluwafisayo
2022-Mar
COVID-19 lockdown, Equatorial Africa, Neural network, Sunspot number, Temperature, Time-series
CoviExpert: COVID-19 detection from chest X-ray using CNN.
In Measurement. Sensors
Arivoli A, Golwala Devdatt, Reddy Rayirth
2022-Oct
CNN, COVID-19, CT scan, CoviExpert, Deep learning, X-ray
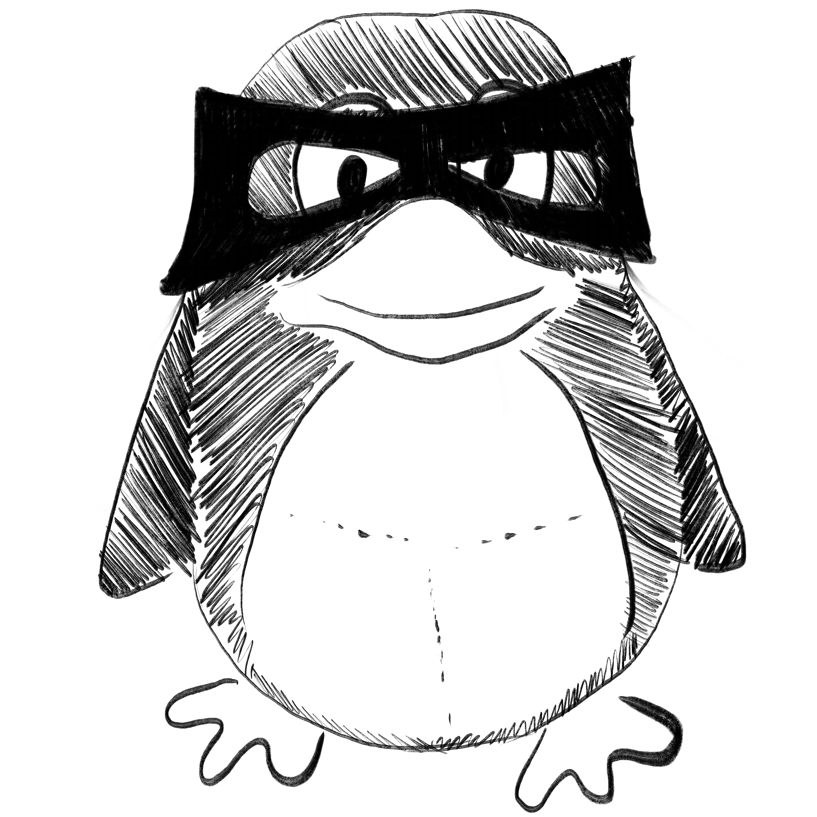
Artificial intelligence and discrete-event simulation for capacity management of intensive care units during the Covid-19 pandemic: A case study.
In Journal of business research
Ortiz-Barrios Miguel, Arias-Fonseca Sebastián, Ishizaka Alessio, Barbati Maria, Avendaño-Collante Betty, Navarro-Jiménez Eduardo
2023-May
Artificial Intelligence (AI), Covid-19, Discrete-Event Simulation (DES), Healthcare, Intensive Care Unit (ICU), Random Forest (RF)
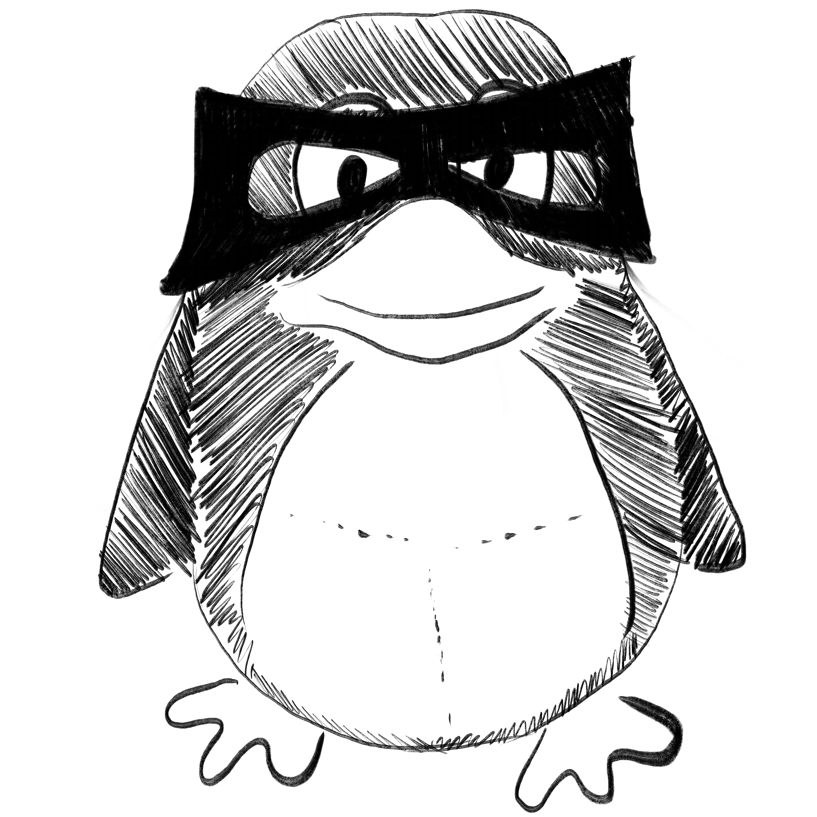
Assessing the effects of data drift on the performance of machine learning models used in clinical sepsis prediction.
In International journal of medical informatics ; h5-index 49.0
BACKGROUND :
METHODS :
RESULTS :
CONCLUSION :
Rahmani Keyvan, Thapa Rahul, Tsou Peiling, Casie Chetty Satish, Barnes Gina, Lam Carson, Foon Tso Chak
2022-Nov-19
Clinical decision support, Data drift, Machine learning, Sepsis
Does social distancing impact pediatric upper airway infections? An observational controlled study and a brief literature review.
In American journal of otolaryngology ; h5-index 23.0
PURPOSE :
MATERIALS AND METHODS :
RESULTS :
CONCLUSIONS :
Franchella Sebastiano, Favaretto Niccolò, Frigo Annachiara, Franz Leonardo, Pilo Simona, Mularoni Francesca, Marciani Silvia, Nicolai Piero, Marioni Gino, Cazzador Diego
2023-Mar-01
COVID-19, Pediatric, SARS-CoV-2, Social distancing, Upper airways infection
Determinants of COVID-19 vaccine hesitancy among students and parents in Sentinel Schools Network of Catalonia, Spain.
In PloS one ; h5-index 176.0
Ganem Fabiana, Folch Cinta, Colom-Cadena Andreu, Bordas Anna, Alonso Lucia, Soriano-Arandes Antoni, Casabona Jordi
2023
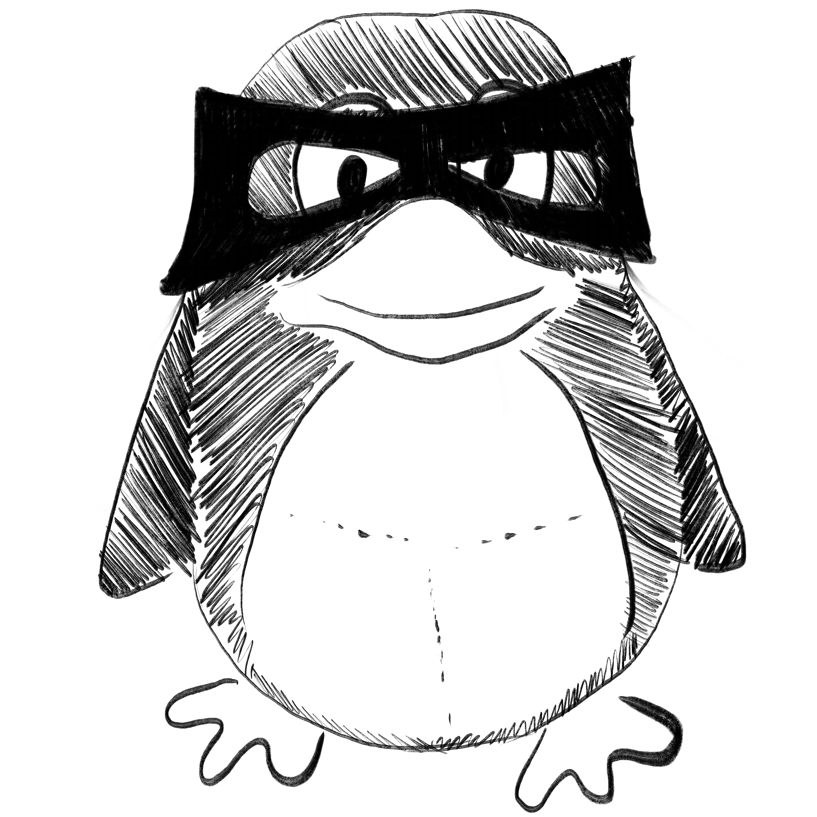
Assessing the effects of therapeutic combinations on SARS-CoV-2 infected patient outcomes: A big data approach.
In PloS one ; h5-index 176.0
BACKGROUND :
METHODS :
RESULTS :
CONCLUSIONS :
Moradi Hamidreza, Bunnell H Timothy, Price Bradley S, Khodaverdi Maryam, Vest Michael T, Porterfield James Z, Anzalone Alfred J, Santangelo Susan L, Kimble Wesley, Harper Jeremy, Hillegass William B, Hodder Sally L
2023
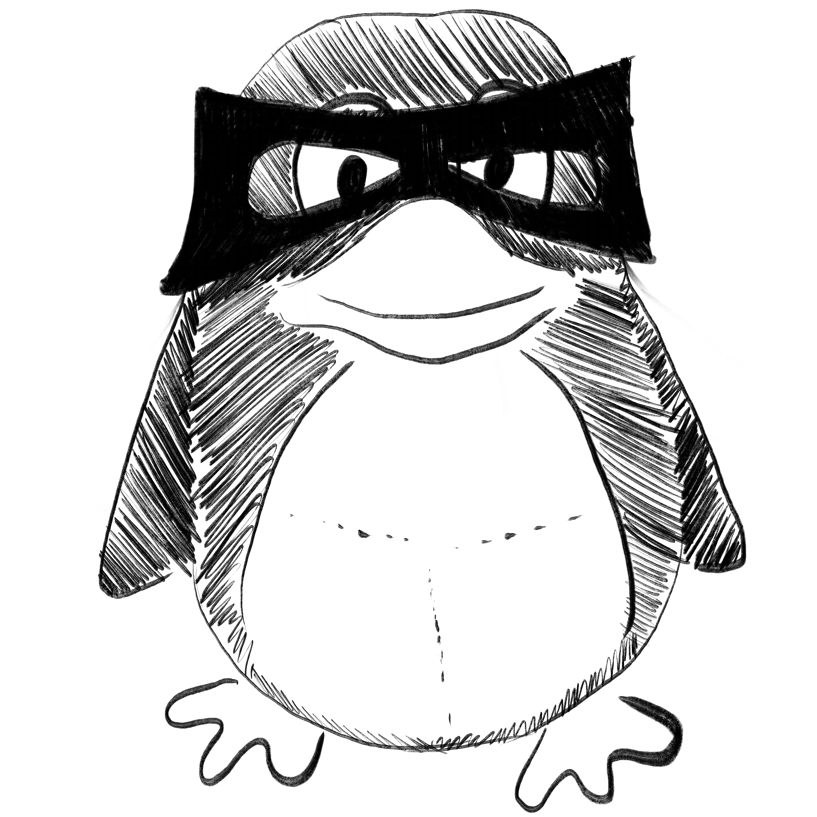
A hybrid CNN and ensemble model for COVID-19 lung infection detection on chest CT scans.
In PloS one ; h5-index 176.0
Akl Ahmed A, Hosny Khalid M, Fouda Mostafa M, Salah Ahmad
2023
COVID-19 imaging, where do we go from here? Bibliometric analysis of medical imaging in COVID-19.
In European radiology ; h5-index 62.0
OBJECTIVES :
METHODS :
RESULTS :
CONCLUSIONS :
Wen Ru, Zhang Mudan, Xu Rui, Gao Yingming, Liu Lin, Chen Hui, Wang Xingang, Zhu Wenyan, Lin Huafang, Liu Chen, Zeng Xianchun
2023-Mar-09
Bibliometrics, COVID-19, CiteSpace, Medical imaging, Treads
Artificial intelligence for assistance of radiology residents in chest CT evaluation for COVID-19 pneumonia: a comparative diagnostic accuracy study.
In Acta radiologica (Stockholm, Sweden : 1987)
BACKGROUND :
PURPOSE :
MATERIAL AND METHODS :
RESULTS :
CONCLUSION :
Mlynska Lucja, Malouhi Amer, Ingwersen Maja, Güttler Felix, Gräger Stephanie, Teichgräber Ulf
2023-Mar-08
Artificial intelligence, COVID-19, SARS-CoV-2, computed tomography, deep learning, neural networks
Machine learning prediction for COVID-19 disease severity at hospital admission.
In BMC medical informatics and decision making ; h5-index 38.0
IMPORTANCE :
OBJECTIVE :
DESIGN, SETTING, AND PARTICIPANTS :
MAIN OUTCOMES AND MEASURES :
RESULTS :
CONCLUSIONS AND RELEVANCE :
Raman Ganesh, Ashraf Bilal, Demir Yusuf Kemal, Kershaw Corey D, Cheruku Sreekanth, Atis Murat, Atis Ahsen, Atar Mustafa, Chen Weina, Ibrahim Ibrahim, Bat Taha, Mete Mutlu
2023-Mar-07
COVID-19, Classification, Laboratory markers, Machine learning, Prediction, SARS-CoV-2, Scoring
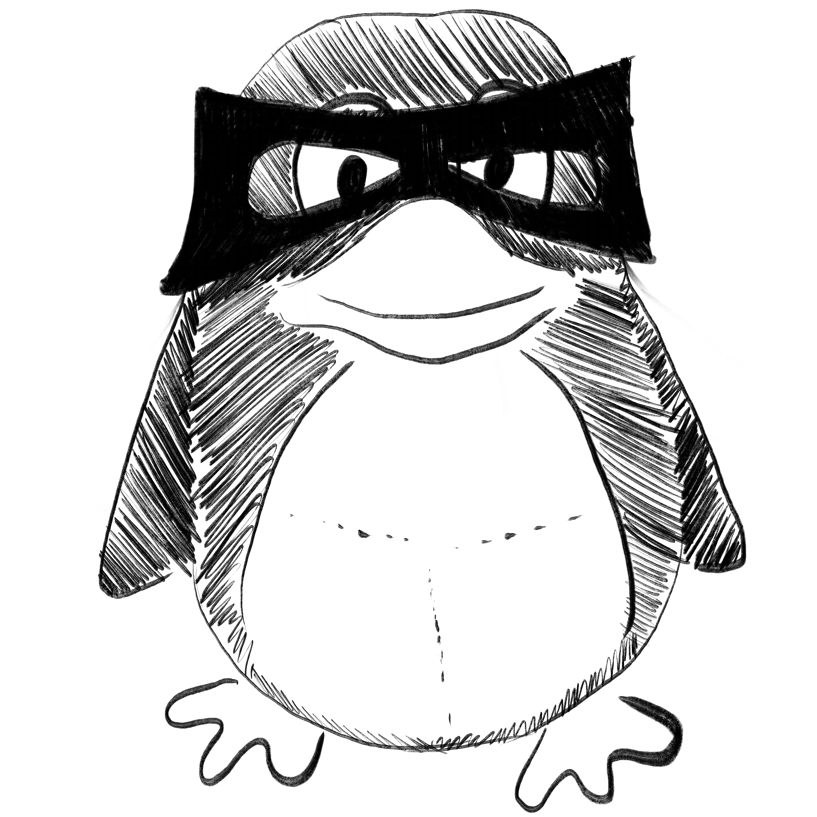
Effects of Antidepressants on COVID Outcome: A Retrospective Study on Large Scale Electronic Health Record Data.
In Interactive journal of medical research
BACKGROUND :
OBJECTIVE :
METHODS :
RESULTS :
CONCLUSIONS :
Rahman Md Mahmudur, Mahi Atqiya Munawara, Melamed Rachel D, Alam Mohammad Arif Ul
2023-Mar-05
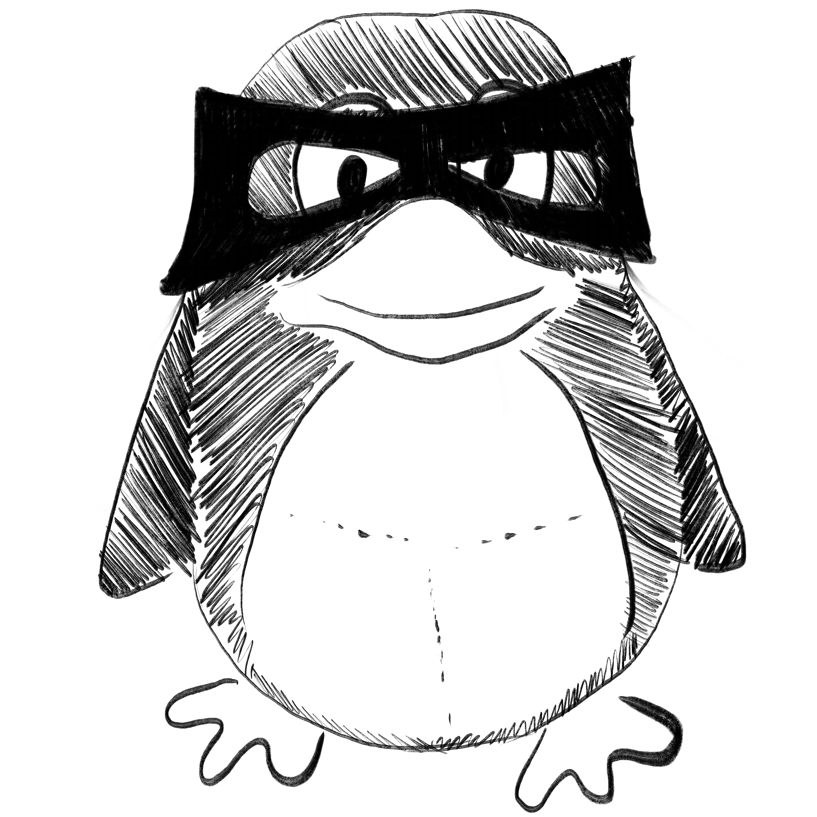
Development and validation of a respiratory-responsive vocal biomarker-based tool for generalizable detection of respiratory impairment: independent case-control studies in multiple respiratory conditions including asthma, chronic obstructive pulmonary disease, and COVID-19.
In Journal of medical Internet research ; h5-index 88.0
BACKGROUND :
OBJECTIVE :
METHODS :
RESULTS :
CONCLUSIONS :
CLINICALTRIAL :
Kaur Savneet, Larsen Erik, Harper James, Purandare Bharat, Uluer Ahmet, Hasdianda Mohammad Adrian, Umale Nikita, Killeen James, Castillo Edward, Jariwala Sunit
2023-Feb-28
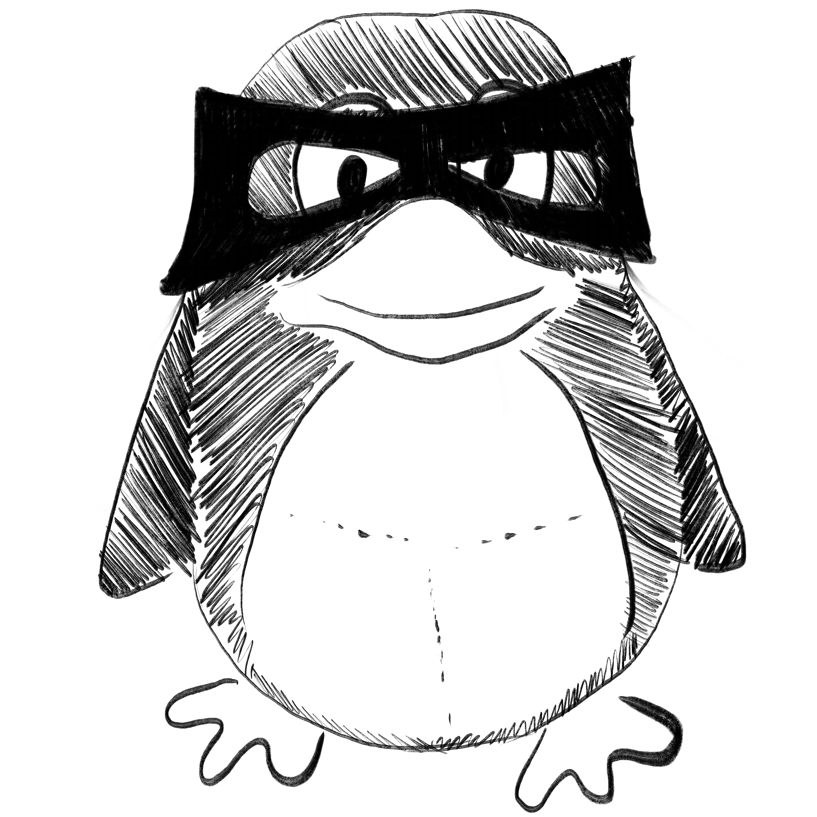
Sentiment analysis and emotion detection of post-COVID educational Tweets: Jordan case.
In Social network analysis and mining
Qaqish Evon, Aranki Aseel, Etaiwi Wael
2023
Deep learning, Education, Emotion detection, Jordan, Natural language processing
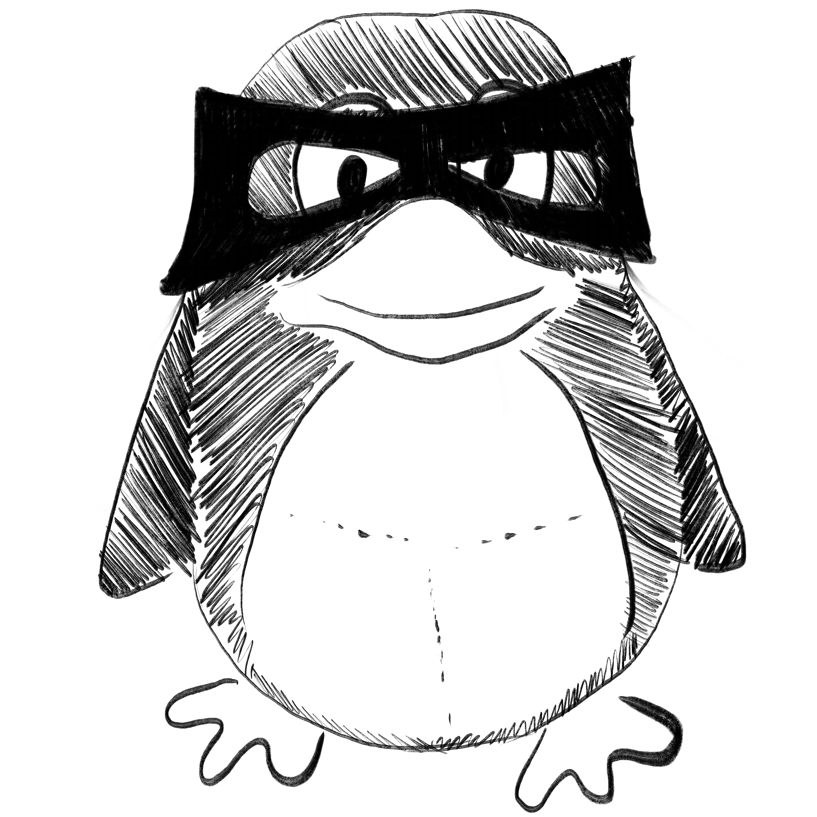
Interpretation of lung disease classification with light attention connected module.
In Biomedical signal processing and control
Choi Youngjin, Lee Hongchul
2023-Jul
Attention, ECA-Net, Grad-CAM, Lung disease, Respiratory sound, eXplainable AI
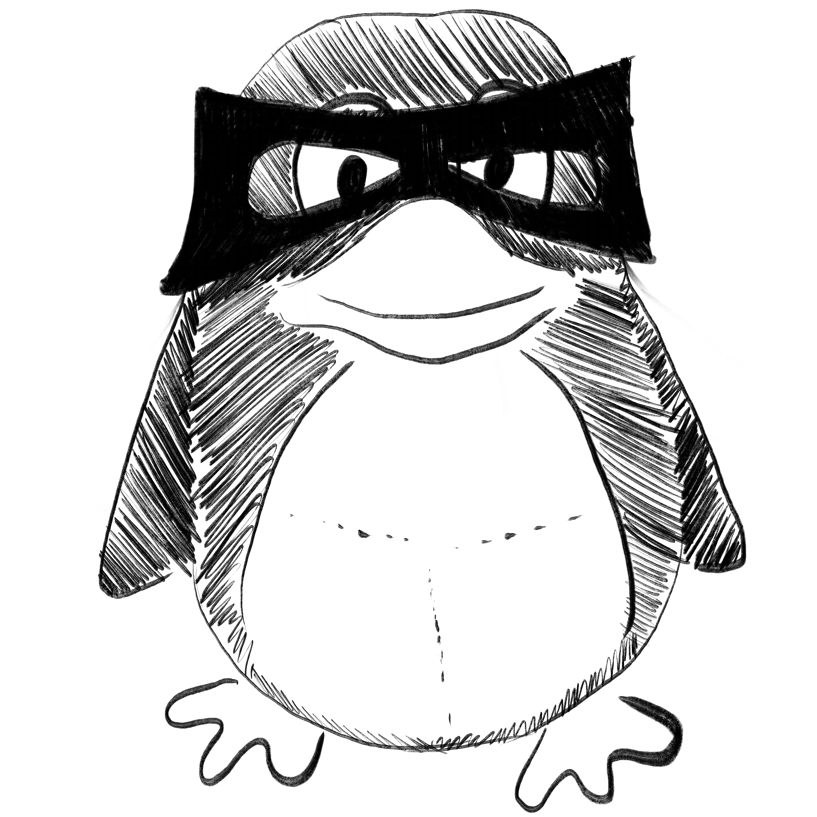
Remote scoring models of rigidity and postural stability of Parkinson's disease based on indirect motions and a low-cost RGB algorithm.
In Frontiers in aging neuroscience ; h5-index 64.0
BACKGROUND AND OBJECTIVES :
METHODS :
RESULTS :
CONCLUSION :
Ma Ling-Yan, Shi Wei-Kun, Chen Cheng, Wang Zhan, Wang Xue-Mei, Jin Jia-Ning, Chen Lu, Ren Kang, Chen Zhong-Lue, Ling Yun, Feng Tao
2023
Parkinson’s disease, computer vision, machine learning, postural stability, remote, rigidity
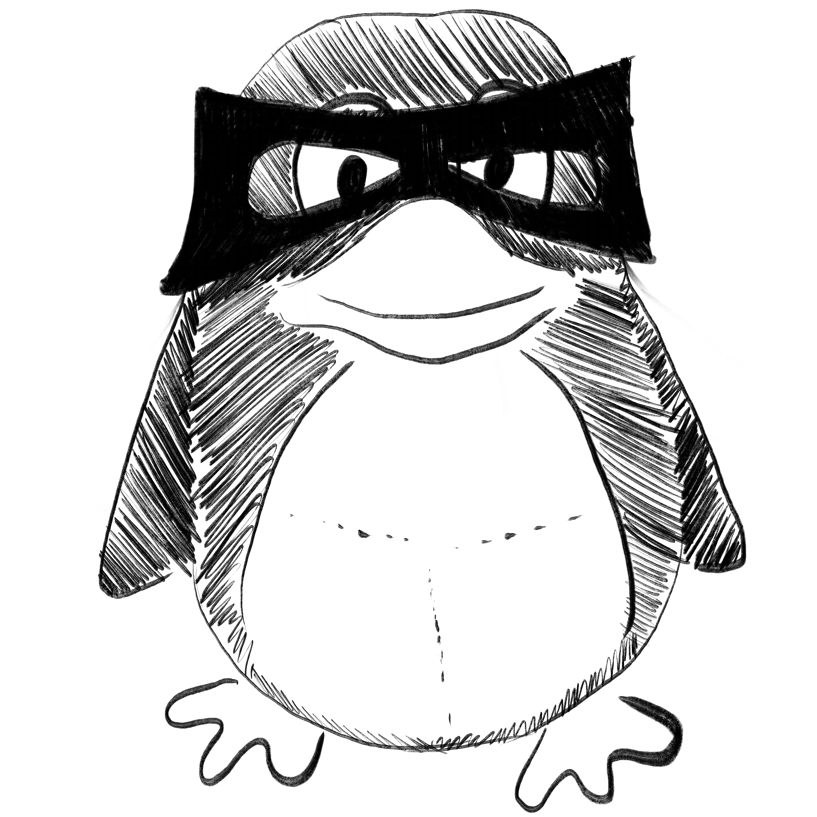
STG-Net: A COVID-19 prediction network based on multivariate spatio-temporal information.
In Biomedical signal processing and control
Song Yucheng, Chen Huaiyi, Song Xiaomeng, Liao Zhifang, Zhang Yan
2023-Jul
COVID-19, Confirmed cases forecasting, Deep learning, STG-Net, Spatial information, Time series
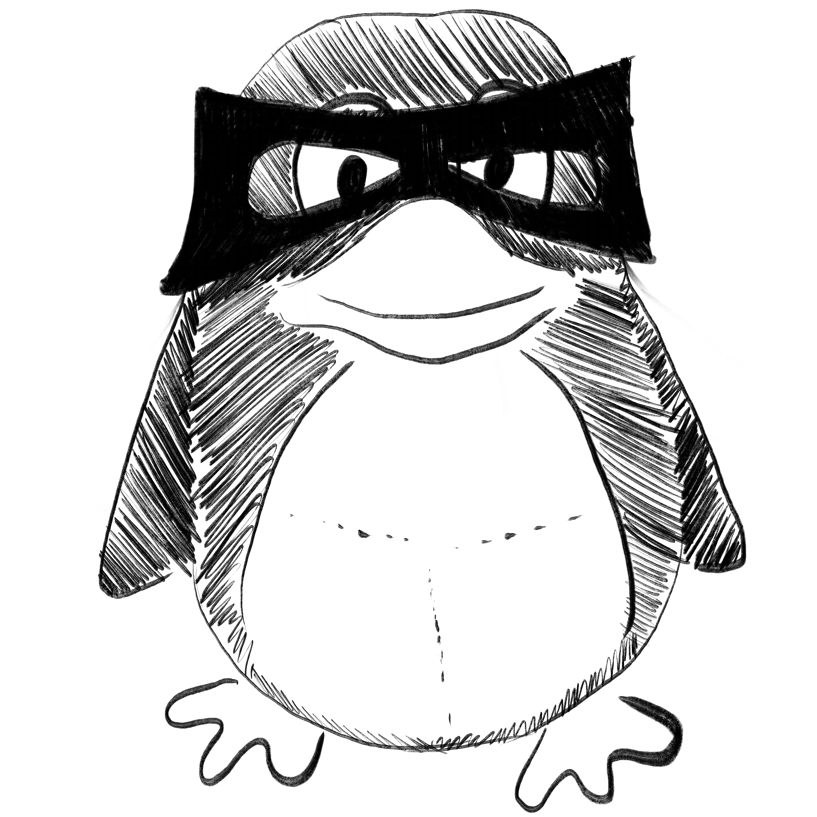
Continuous diagnosis and prognosis by controlling the update process of deep neural networks.
In Patterns (New York, N.Y.)
Sun Chenxi, Li Hongyan, Song Moxian, Cai Derun, Zhang Baofeng, Hong Shenda
2023-Feb-10
COVID-19, biomarker, continuous classification, deep learning, diagnosis, disease staging, prognosis, sepsis, time series
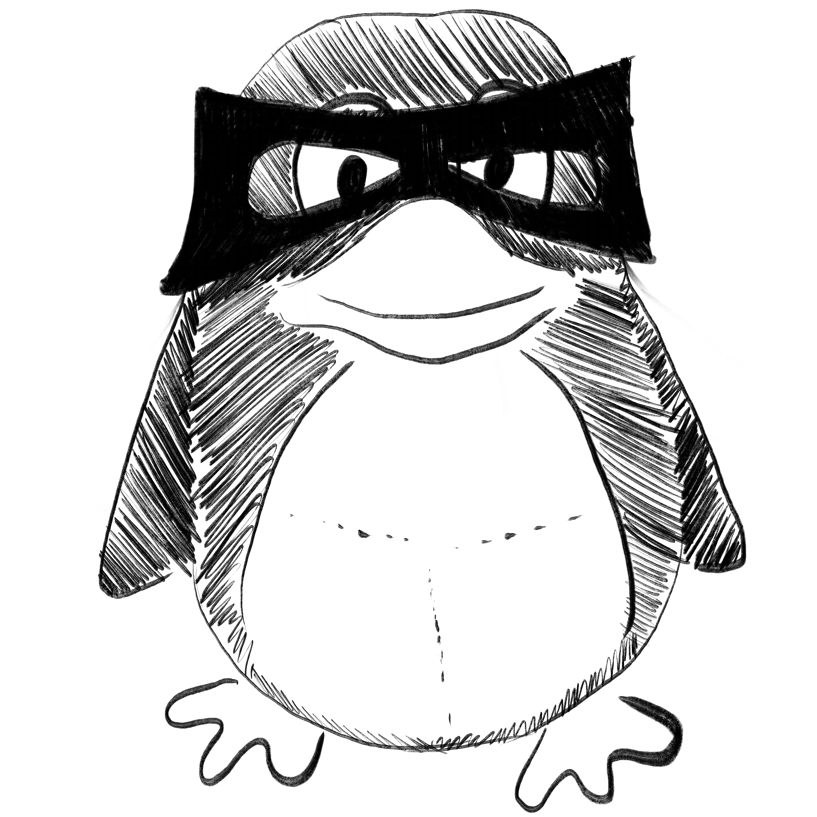
Present and future perspectives in early diagnosis and monitoring for progressive fibrosing interstitial lung diseases.
In Frontiers in medicine
Stanel Stefan Cristian, Rivera-Ortega Pilar
2023
PF-ILD, PPF, idiopathic pulmonary fibrosis, interstitial lung disease, progressive fibrosing interstitial lung disease, progressive pulmonary fibrosis
A multi-use deep learning method for CITE-seq and single-cell RNA-seq data integration with cell surface protein prediction and imputation.
In Nature machine intelligence
Lakkis Justin, Schroeder Amelia, Su Kenong, Lee Michelle Y Y, Bashore Alexander C, Reilly Muredach P, Li Mingyao
2022-Nov
CITE-seq, deep learning, protein prediction, single-cell RNA-seq, single-cell multi-omics
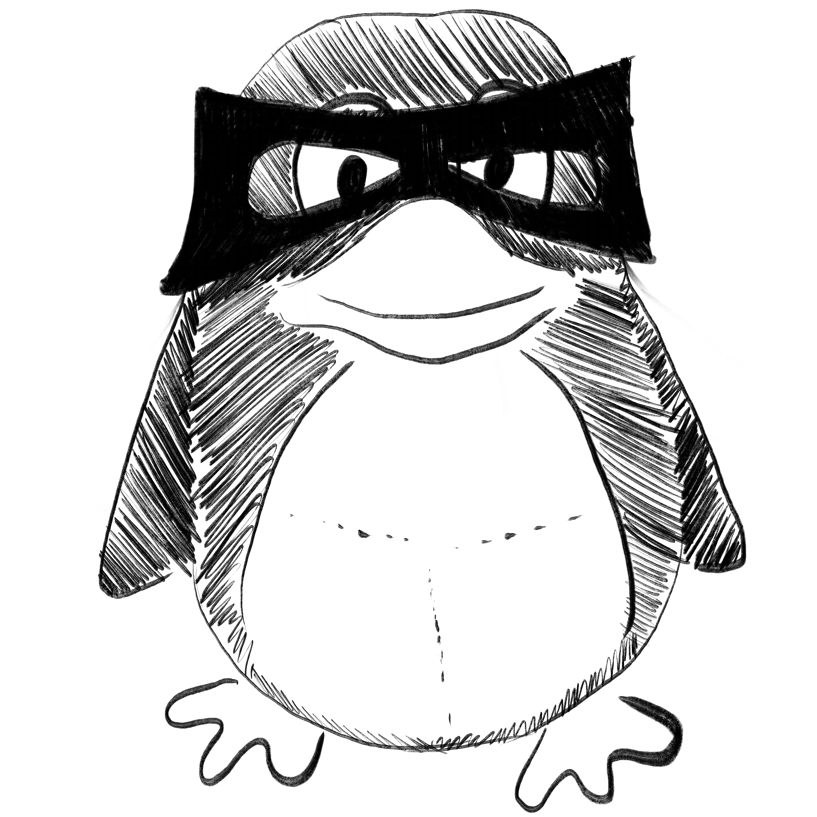
Identifying resilience strategies for disruption management in the healthcare supply chain during COVID-19 by digital innovations: A systematic literature review.
In Informatics in medicine unlocked
Arji Goli, Ahmadi Hossein, Avazpoor Pejman, Hemmat Morteza
2023
COVID-19, Healthcare supply chain, Literature review, Pandemics, Supply chain management
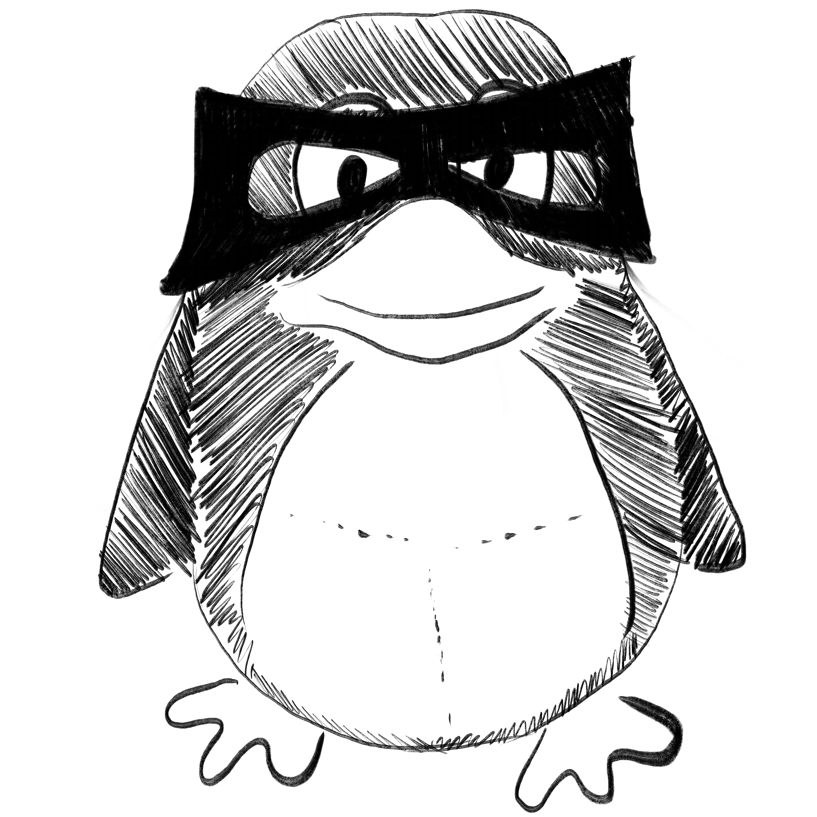
Rough-set based learning: Assessing patterns and predictability of anxiety, depression, and sleep scores associated with the use of cannabinoid-based medicine during COVID-19.
In Frontiers in artificial intelligence
Ramanna Sheela, Ashrafi Negin, Loster Evan, Debroni Karen, Turner Shelley
2023
cannabinoid medicine, electronic health records, machine learning, mental health, rough sets, rough-fuzzy sets
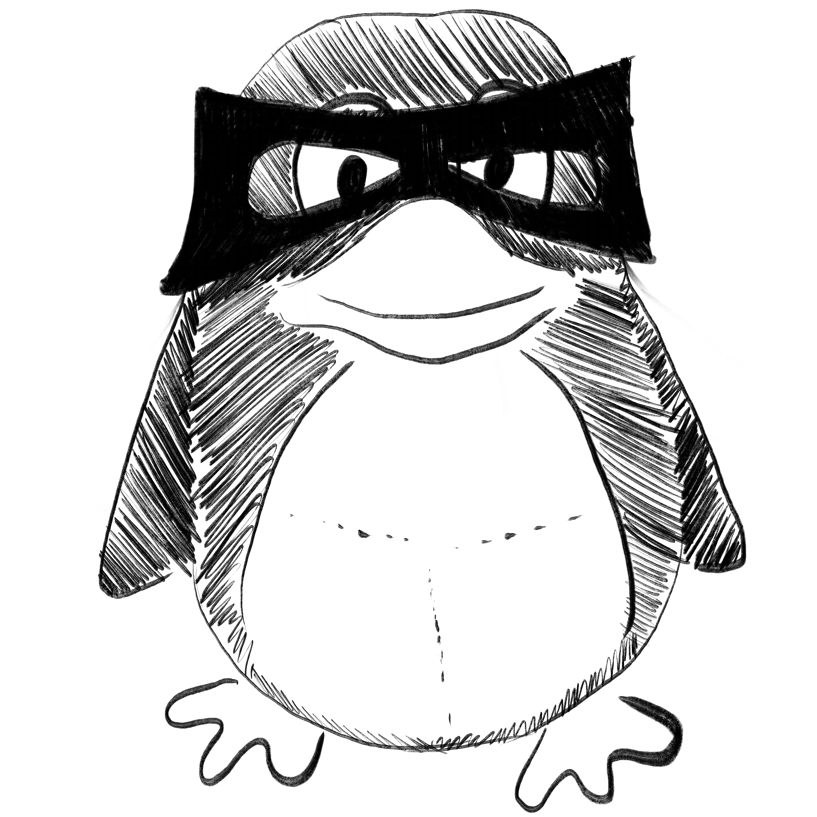
Coronavirus diagnosis using cough sounds: Artificial intelligence approaches.
In Frontiers in artificial intelligence
INTRODUCTION :
METHOD :
RESULT :
CONCLUSION :
Askari Nasab Kazem, Mirzaei Jamal, Zali Alireza, Gholizadeh Sarfenaz, Akhlaghdoust Meisam
2023
artificial intelligence, coronavirus, cough, deep learning, machine learning, respiratory sounds
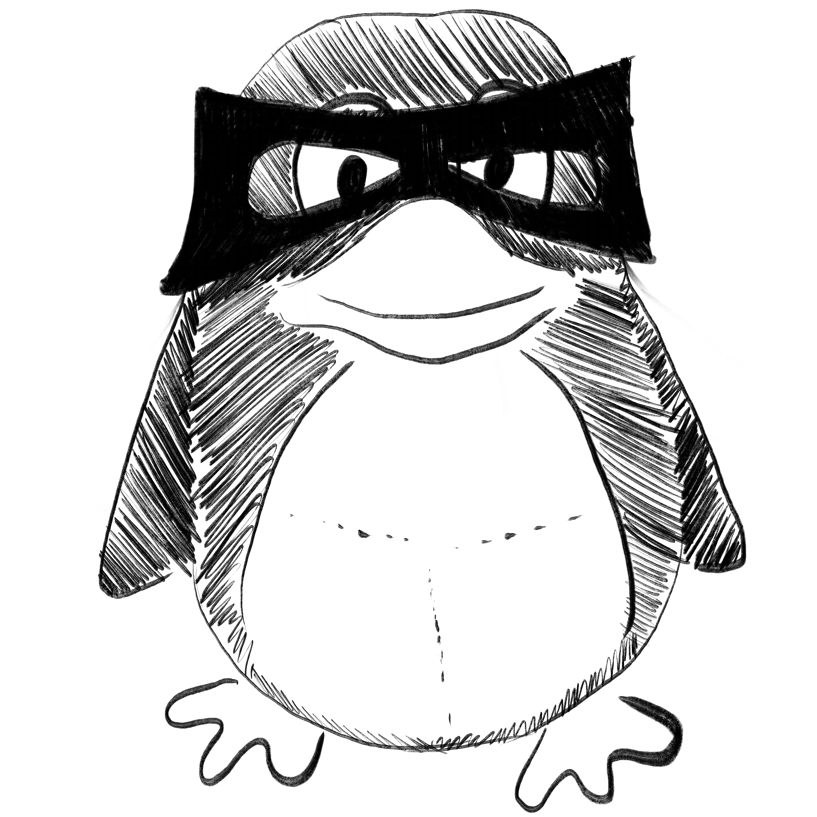
MCSC-Net: COVID-19 detection using deep-Q-neural network classification with RFNN-based hybrid whale optimization.
In Journal of X-ray science and technology
BACKGROUND :
OBJECTIVE :
METHODS :
RESULTS :
CONCLUSION :
Deepak Gerard, Madiajagan M, Kulkarni Sanjeev, Ahmed Ahmed Najat, Gopatoti Anandbabu, Ammisetty Veeraswamy
2023-Feb-28
COVID-19, chest X-Ray, deep-Q-neural networks, hybrid median bilateral filter, robust feature neural network
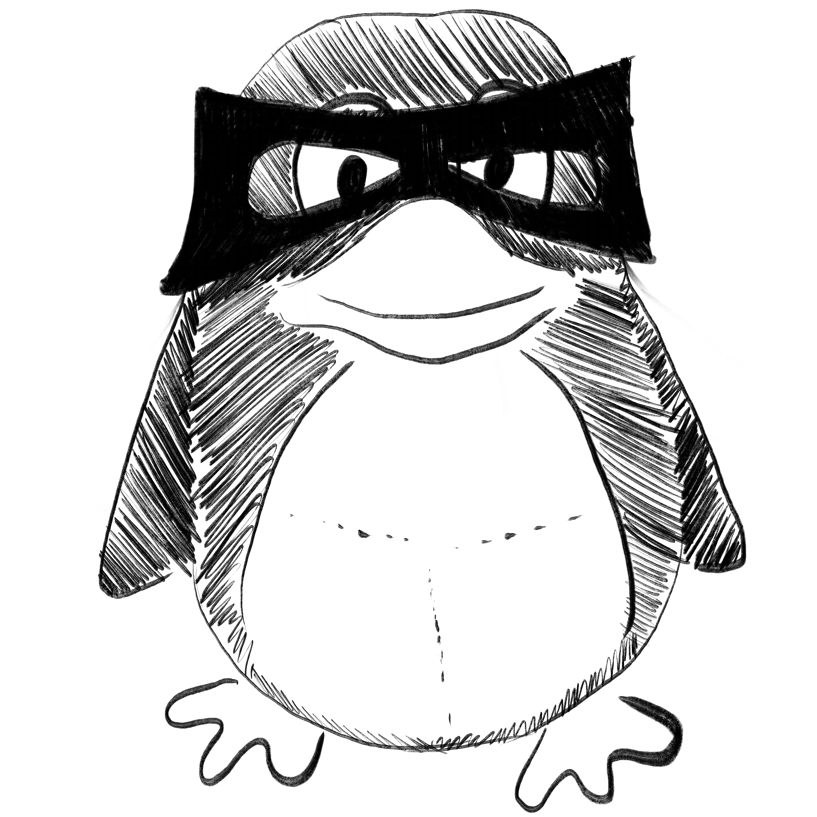
Data driven contagion risk management in low-income countries using machine learning applications with COVID-19 in South Asia.
In Scientific reports ; h5-index 158.0
Shonchoy Abu S, Mahzab Moogdho M, Mahmood Towhid I, Ali Manhal
2023-Mar-06
Application of machine learning approach in emergency department to support clinical decision making for SARS-CoV-2 infected patients.
In Journal of integrative bioinformatics
Casano Nicolò, Santini Silvano Junior, Vittorini Pierpaolo, Sinatti Gaia, Carducci Paolo, Mastroianni Claudio Maria, Ciardi Maria Rosa, Pasculli Patrizia, Petrucci Emiliano, Marinangeli Franco, Balsano Clara
2023-Mar-07
COVID-19, ROX index, SARS-CoV-2, emergency medicine, machine learning
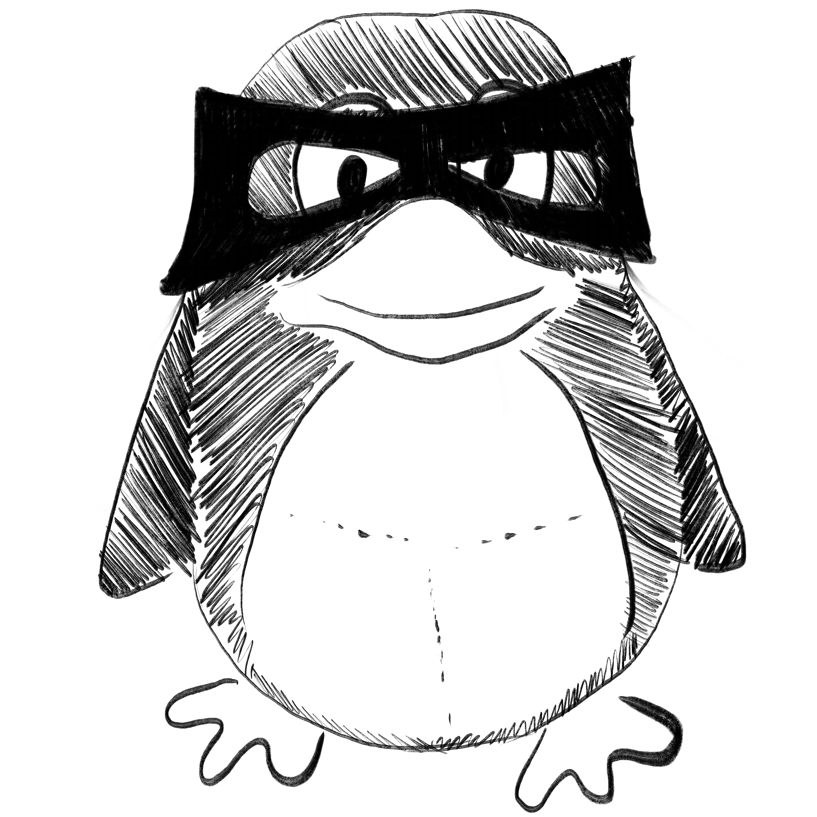
Unveiling mutation effects on the structural dynamics of the main protease from SARS-CoV-2 with hybrid simulation methods.
In Journal of molecular graphics & modelling
Gasparini P, Philot E A, Pantaleão S Q, Torres-Bonfim N E S M, Kliousoff A, Quiroz R C N, Perahia D, Simões R P, Magro A J, Scott A L
2023-Feb-22
Main protease, Molecular dynamics, Mutation, Normal modes, Quantum mechanics, Residue F140, SARS-CoV-2, Structural dynamics
ATR-FTIR spectrum analysis of plasma samples for rapid identification of recovered COVID-19 individuals.
In Journal of biophotonics
Karas Boris Yu, Sitnikova Vera E, Nosenko Tatiana, Dedkov Vladimir G, Arsentieva Natalia A, Gavrilenko Natalia V, Moiseev Ivan S, Totolian Areg A, Kajava Andrey V, Uspenskaya Mayya V
2023-Mar-03
ANN, ATR-FTIR spectroscopy, COVID-19, PCA-LDA, chemometric, plasma, sepsis
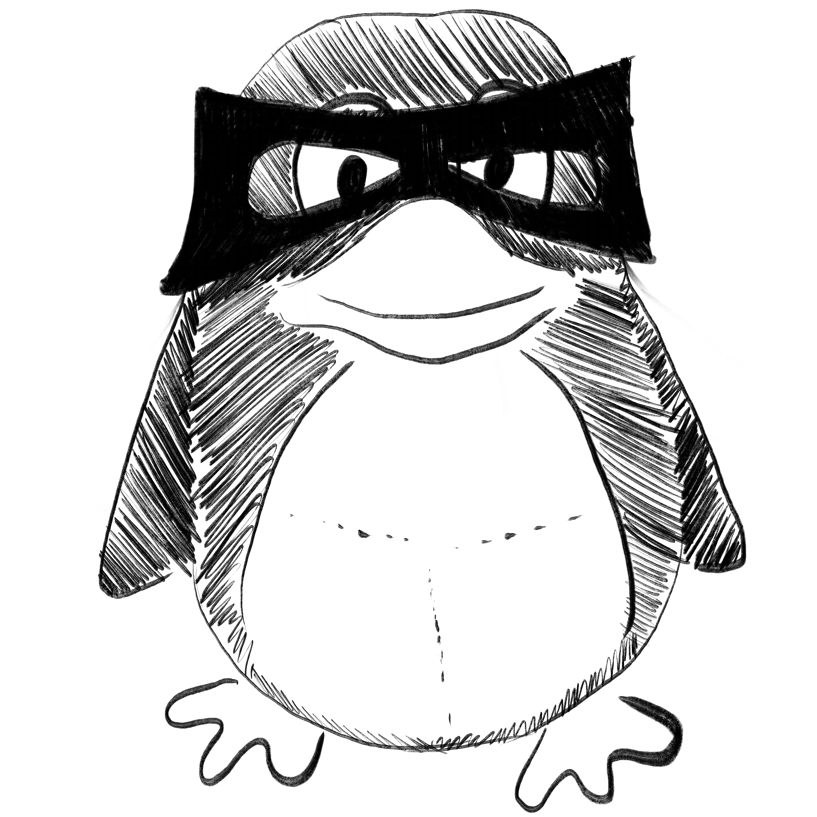
Structural causal model with expert augmented knowledge to estimate the effect of oxygen therapy on mortality in the ICU.
In Artificial intelligence in medicine ; h5-index 34.0
Gani Md Osman, Kethireddy Shravan, Adib Riddhiman, Hasan Uzma, Griffin Paul, Adibuzzaman Mohammad
2023-Mar
Causal inference, Critical care, Expert augmented knowledge, Oxygen therapy, Structural causal model
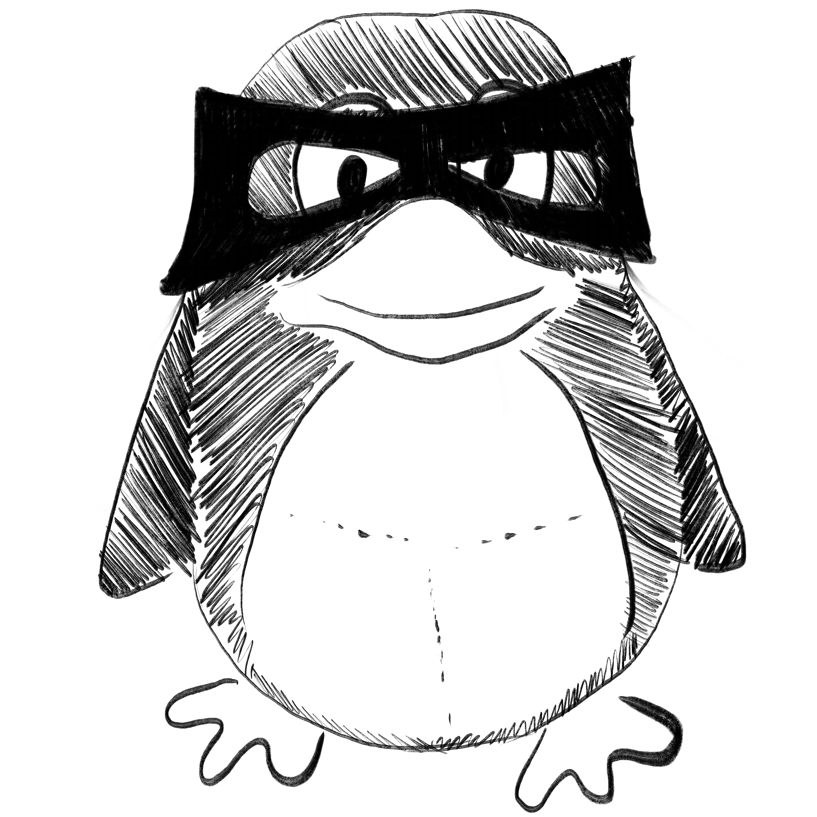
Benchmarking of Machine Learning classifiers on plasma proteomic for COVID-19 severity prediction through interpretable artificial intelligence.
In Artificial intelligence in medicine ; h5-index 34.0
Dimitsaki Stella, Gavriilidis George I, Dimitriadis Vlasios K, Natsiavas Pantelis
2023-Mar
Artificial intelligence, COVID-19, Forecasting, Machine Learning, Severity prediction
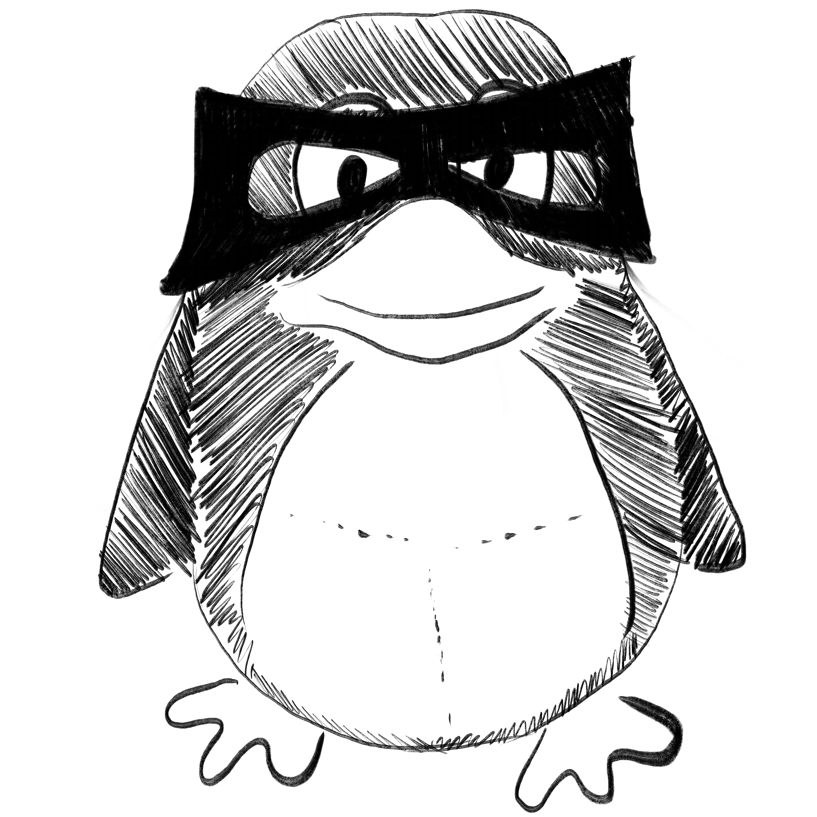
The voice of COVID-19: Breath and cough recording classification with temporal decision trees and random forests.
In Artificial intelligence in medicine ; h5-index 34.0
Manzella F, Pagliarini G, Sciavicco G, Stan I E
2023-Mar
COVID-19, Interval temporal decision trees and forests, Symbolic models
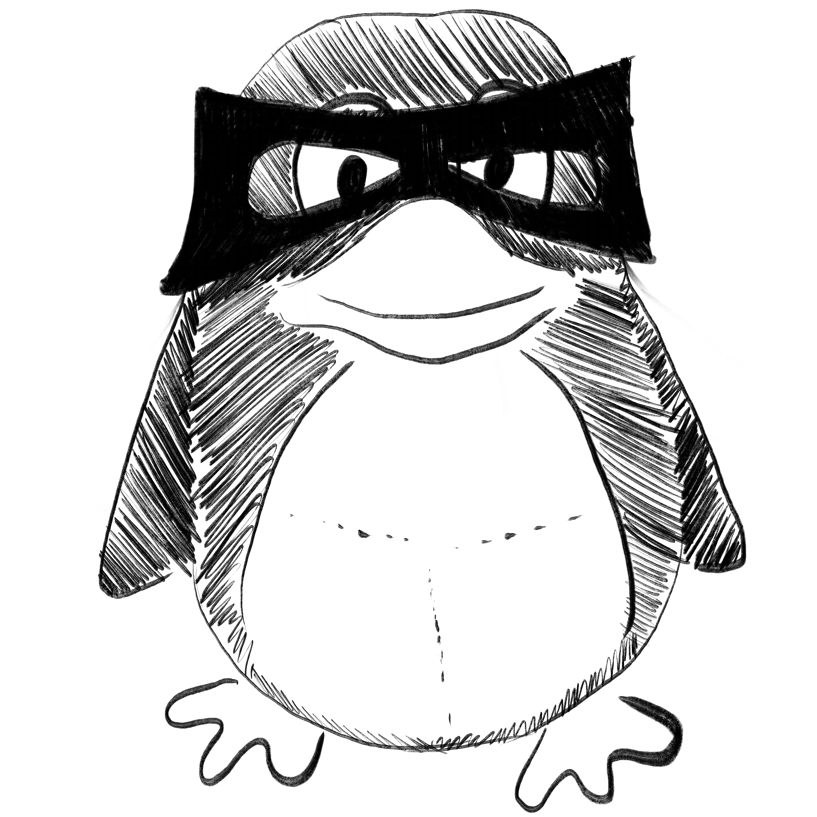
The effect of COVID-19 on self-reported safety incidents in aviation: An examination of the heterogeneous effects using causal machine learning.
In Journal of safety research
INTRODUCTION :
METHOD :
RESULTS :
PRACTICAL APPLICATIONS :
Choi Youngran, Gibson James R
2023-Feb
Aviation incursions/excursions, COVID-19, Heterogeneous treatment effects, Machine learning
Results of the COVID-19 mental health international for the health professionals (COMET-HP) study: depression, suicidal tendencies and conspiracism.
In Social psychiatry and psychiatric epidemiology
INTRODUCTION :
MATERIALS AND METHODS :
STATISTICAL ANALYSIS :
RESULTS :
CONCLUSIONS :
N Fountoulakis Konstantinos, N Karakatsoulis Grigorios, Abraham Seri, Adorjan Kristina, Ahmed Helal Uddin, Alarcón Renato D, Arai Kiyomi, Auwal Sani Salihu, Bobes Julio, Bobes-Bascaran Teresa, Bourgin-Duchesnay Julie, Bredicean Cristina Ana, Bukelskis Laurynas, Burkadze Akaki, Cabrera Abud Indira Indiana, Castilla-Puentes Ruby, Cetkovich Marcelo, Colon-Rivera Hector, Corral Ricardo, Cortez-Vergara Carla, Crepin Piirika, de Berardis Domenico, Zamora Delgado Sergio, de Lucena David, de Sousa Avinash, di Stefano Ramona, Dodd Seetal, Elek Livia Priyanka, Elissa Anna, Erdelyi-Hamza Berta, Erzin Gamze, Etchevers Martin J, Falkai Peter, Farcas Adriana, Fedotov Ilya, Filatova Viktoriia, Fountoulakis Nikolaos K, Frankova Iryna, Franza Francesco, Frias Pedro, Galako Tatiana, Garay Cristian J, Garcia-Álvarez Leticia, García-Portilla Paz, Gonda Xenia, Gondek Tomasz M, Morera González Daniela, Gould Hilary, Grandinetti Paolo, Grau Arturo, Groudeva Violeta, Hagin Michal, Harada Takayuki, Hasan Tasdik M, Azreen Hashim Nurul, Hilbig Jan, Hossain Sahadat, Iakimova Rossitza, Ibrahim Mona, Iftene Felicia, Ignatenko Yulia, Irarrazaval Matias, Ismail Zaliha, Ismayilova Jamila, Jacobs Asaf, Jakovljević Miro, Jakšić Nenad, Javed Afzal, Yilmaz Kafali Helin, Karia Sagar, Kazakova Olga, Khalifa Doaa, Khaustova Olena, Koh Steve, Kopishinskaia Svetlana, Kosenko Korneliia, Koupidis Sotirios A, Kovacs Illes, Kulig Barbara, Lalljee Alisha, Liewig Justine, Majid Abdul, Malashonkova Evgeniia, Malik Khamelia, Iqbal Malik Najma, Mammadzada Gulay, Mandalia Bilvesh, Marazziti Donatella, Marčinko Darko, Martinez Stephanie, Matiekus Eimantas, Mejia Gabriela, Memon Roha Saeed, Meza Martínez Xarah Elenne, Mickevičiūtė Dalia, Milev Roumen, Mohammed Muftau, Molina-López Alejandro, Morozov Petr, Muhammad Nuru Suleiman, Mustač Filip, Naor Mika S, Nassieb Amira, Navickas Alvydas, Okasha Tarek, Pandova Milena, Panfil Anca-Livia, Panteleeva Liliya, Papava Ion, Patsali Mikaella E, Pavlichenko Alexey, Pejuskovic Bojana, Pinto da Costa Mariana, Popkov Mikhail, Popovic Dina, Raduan Nor Jannah Nasution, Vargas Ramírez Francisca, Rancans Elmars, Razali Salmi, Rebok Federico, Rewekant Anna, Reyes Flores Elena Ninoska, Rivera-Encinas María Teresa, Saiz Pilar A, Sánchez de Carmona Manuel, Saucedo Martínez David, Saw Jo Anne, Saygili Görkem, Schneidereit Patricia, Shah Bhumika, Shirasaka Tomohiro, Silagadze Ketevan, Sitanggang Satti, Skugarevsky Oleg, Spikina Anna, Mahalingappa Sridevi Sira, Stoyanova Maria, Szczegielniak Anna, Tamasan Simona Claudia, Tavormina Giuseppe, Tavormina Maurilio Giuseppe Maria, Theodorakis Pavlos N, Tohen Mauricio, Tsapakis Eva-Maria, Tukhvatullina Dina, Ullah Irfan, Vaidya Ratnaraj, Vega-Dienstmaier Johann M, Vrublevska Jelena, Vukovic Olivera, Vysotska Olga, Widiasih Natalia, Yashikhina Anna, Prezerakos Panagiotis E, Berk Michael, Levaj Sarah, Smirnova Daria
2023-Mar-03
Anxiety, COVID-19, Conspiracy theories, Depression, Health professionals, Mental disorders, Mental health, Psychiatry, Suicidality
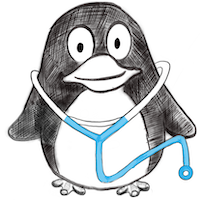
A Computational Approach in the Diagnostic Process of COVID-19: The Missing Link between the Laboratory and Emergency Department.
In Frontiers in bioscience (Landmark edition)
BACKGROUND :
METHODS :
RESULTS :
CONCLUSIONS :
Lanzilao Luisa, Mariniello Antonella, Polenzani Bianca, Aldinucci Alessandra, Nazerian Peiman, Prota Alessio, Grifoni Stefano, Tonietti Barbara, Neri Chiara, Turco Livia, Fanelli Alessandra, Amedei Amedeo, Stanghellini Elena
2023-Feb-22
COVID-19, automated classifiers, diagnosis, laboratory medicine, machine learning, “physicians gestalt”
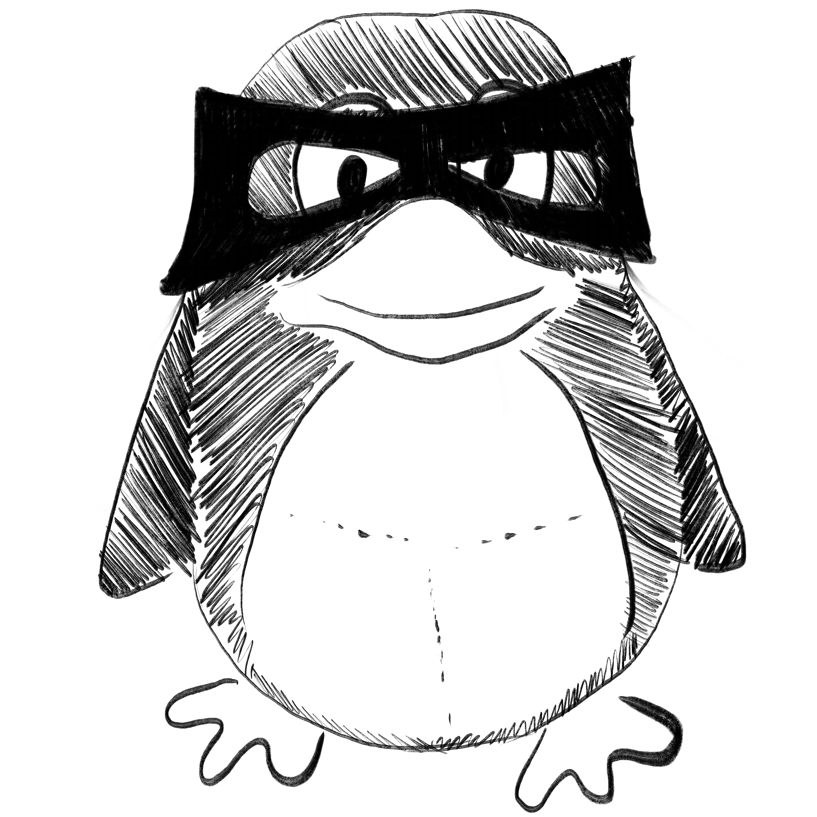
The future of automated infection detection: Innovation to transform practice (Part III/III).
In Antimicrobial stewardship & healthcare epidemiology : ASHE
Branch-Elliman Westyn, Sundermann Alexander J, Wiens Jenna, Shenoy Erica S
2023
Robust framework for COVID-19 identication from a multicenter dataset of chest CT scans.
In PloS one ; h5-index 176.0
Khademi Sadaf, Heidarian Shahin, Afshar Parnian, Enshaei Nastaran, Naderkhani Farnoosh, Rafiee Moezedin Javad, Oikonomou Anastasia, Shafiee Akbar, Babaki Fard Faranak, Plataniotis Konstantinos N, Mohammadi Arash
2023
Optimizing non-pharmaceutical intervention strategies against COVID-19 using artificial intelligence.
In Frontiers in public health
Janko Vito, Reščič Nina, Vodopija Aljoša, Susič David, De Masi Carlo, Tušar Tea, Gradišek Anton, Vandepitte Sophie, De Smedt Delphine, Javornik Jana, Gams Matjaž, Luštrek Mitja
2023
COVID-19, epidemiological modeling, intervention plans, machine learning, multi-objective optimization
Development and testing of a multi-lingual Natural Language Processing-based deep learning system in 10 languages for COVID-19 pandemic crisis: A multi-center study.
In Frontiers in public health
PURPOSE :
METHODS :
RESULTS :
CONCLUSION :
Yang Lily Wei Yun, Ng Wei Yan, Lei Xiaofeng, Tan Shaun Chern Yuan, Wang Zhaoran, Yan Ming, Pargi Mohan Kashyap, Zhang Xiaoman, Lim Jane Sujuan, Gunasekeran Dinesh Visva, Tan Franklin Chee Ping, Lee Chen Ee, Yeo Khung Keong, Tan Hiang Khoon, Ho Henry Sun Sien, Tan Benedict Wee Bor, Wong Tien Yin, Kwek Kenneth Yung Chiang, Goh Rick Siow Mong, Liu Yong, Ting Daniel Shu Wei
2023
Artificial Intelligence, COVID-19, Natural Language Processing, conversational chatbot, health education, pandemic education
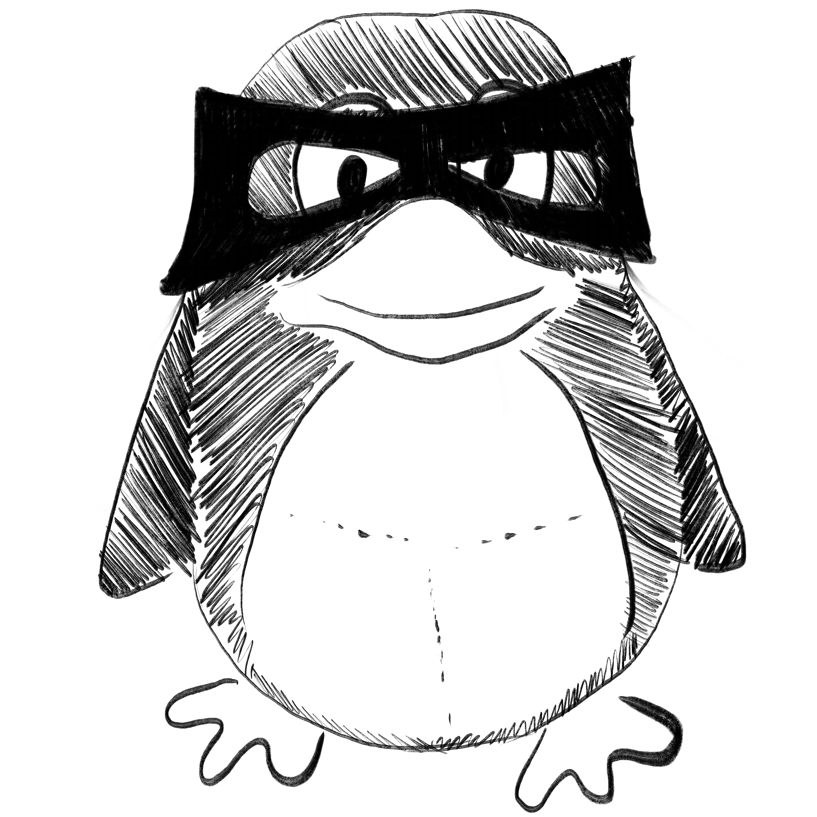
Potential and limitations of machine meta-learning (ensemble) methods for predicting COVID-19 mortality in a large inhospital Brazilian dataset.
In Scientific reports ; h5-index 158.0
de Paiva Bruno Barbosa Miranda, Pereira Polianna Delfino, de Andrade Claudio Moisés Valiense, Gomes Virginia Mara Reis, Souza-Silva Maira Viana Rego, Martins Karina Paula Medeiros Prado, Sales Thaís Lorenna Souza, de Carvalho Rafael Lima Rodrigues, Pires Magda Carvalho, Ramos Lucas Emanuel Ferreira, Silva Rafael Tavares, de Freitas Martins Vieira Alessandra, Nunes Aline Gabrielle Sousa, de Oliveira Jorge Alzira, de Oliveira Maurílio Amanda, Scotton Ana Luiza Bahia Alves, da Silva Carla Thais Candida Alves, Cimini Christiane Corrêa Rodrigues, Ponce Daniela, Pereira Elayne Crestani, Manenti Euler Roberto Fernandes, Rodrigues Fernanda d’Athayde, Anschau Fernando, Botoni Fernando Antônio, Bartolazzi Frederico, Grizende Genna Maira Santos, Noal Helena Carolina, Duani Helena, Gomes Isabela Moraes, Costa Jamille Hemétrio Salles Martins, di Sabatino Santos Guimarães Júlia, Tupinambás Julia Teixeira, Rugolo Juliana Machado, Batista Joanna d’Arc Lyra, de Alvarenga Joice Coutinho, Chatkin José Miguel, Ruschel Karen Brasil, Zandoná Liege Barella, Pinheiro Lílian Santos, Menezes Luanna Silva Monteiro, de Oliveira Lucas Moyses Carvalho, Kopittke Luciane, Assis Luisa Argolo, Marques Luiza Margoto, Raposo Magda Cesar, Floriani Maiara Anschau, Bicalho Maria Aparecida Camargos, Nogueira Matheus Carvalho Alves, de Oliveira Neimy Ramos, Ziegelmann Patricia Klarmann, Paraiso Pedro Gibson, de Lima Martelli Petrônio José, Senger Roberta, Menezes Rochele Mosmann, Francisco Saionara Cristina, Araújo Silvia Ferreira, Kurtz Tatiana, Fereguetti Tatiani Oliveira, de Oliveira Thainara Conceição, Ribeiro Yara Cristina Neves Marques Barbosa, Ramires Yuri Carlotto, Lima Maria Clara Pontello Barbosa, Carneiro Marcelo, Bezerra Adriana Falangola Benjamin, Schwarzbold Alexandre Vargas, de Moura Costa André Soares, Farace Barbara Lopes, Silveira Daniel Vitorio, de Almeida Cenci Evelin Paola, Lucas Fernanda Barbosa, Aranha Fernando Graça, Bastos Gisele Alsina Nader, Vietta Giovanna Grunewald, Nascimento Guilherme Fagundes, Vianna Heloisa Reniers, Guimarães Henrique Cerqueira, de Morais Julia Drumond Parreiras, Moreira Leila Beltrami, de Oliveira Leonardo Seixas, de Deus Sousa Lucas, de Souza Viana Luciano, de Souza Cabral Máderson Alvares, Ferreira Maria Angélica Pires, de Godoy Mariana Frizzo, de Figueiredo Meire Pereira, Guimarães-Junior Milton Henriques, de Paula de Sordi Mônica Aparecida, da Cunha Severino Sampaio Natália, Assaf Pedro Ledic, Lutkmeier Raquel, Valacio Reginaldo Aparecido, Finger Renan Goulart, de Freitas Rufino, Guimarães Silvana Mangeon Meirelles, Oliveira Talita Fischer, Diniz Thulio Henrique Oliveira, Gonçalves Marcos André, Marcolino Milena Soriano
2023-Mar-01
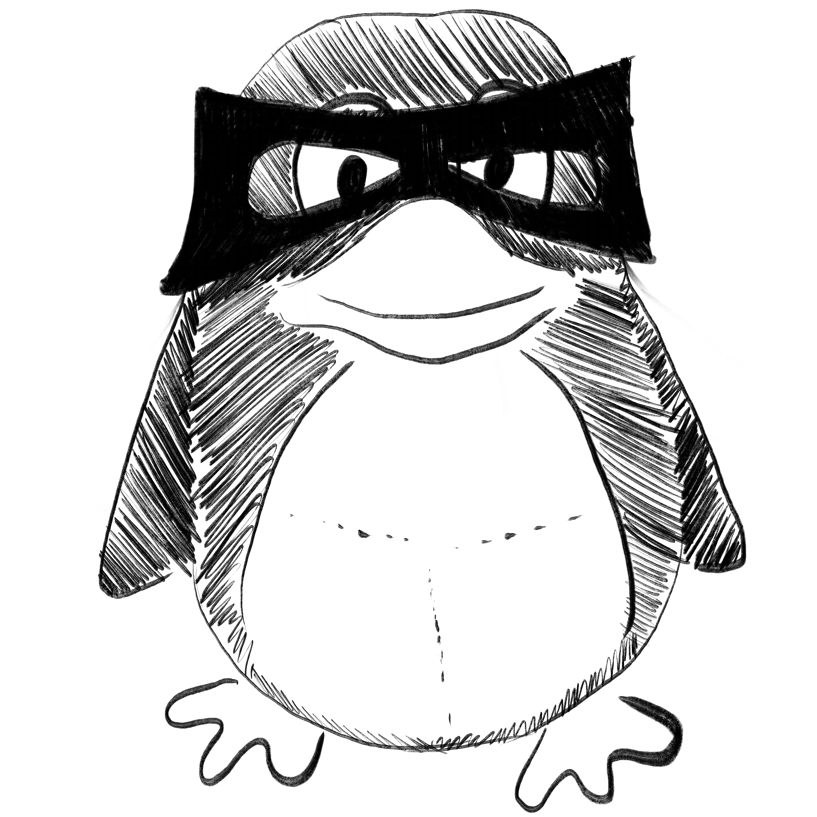
Biases associated with database structure for COVID-19 detection in X-ray images.
In Scientific reports ; h5-index 158.0
Arias-Garzón Daniel, Tabares-Soto Reinel, Bernal-Salcedo Joshua, Ruz Gonzalo A
2023-Mar-01
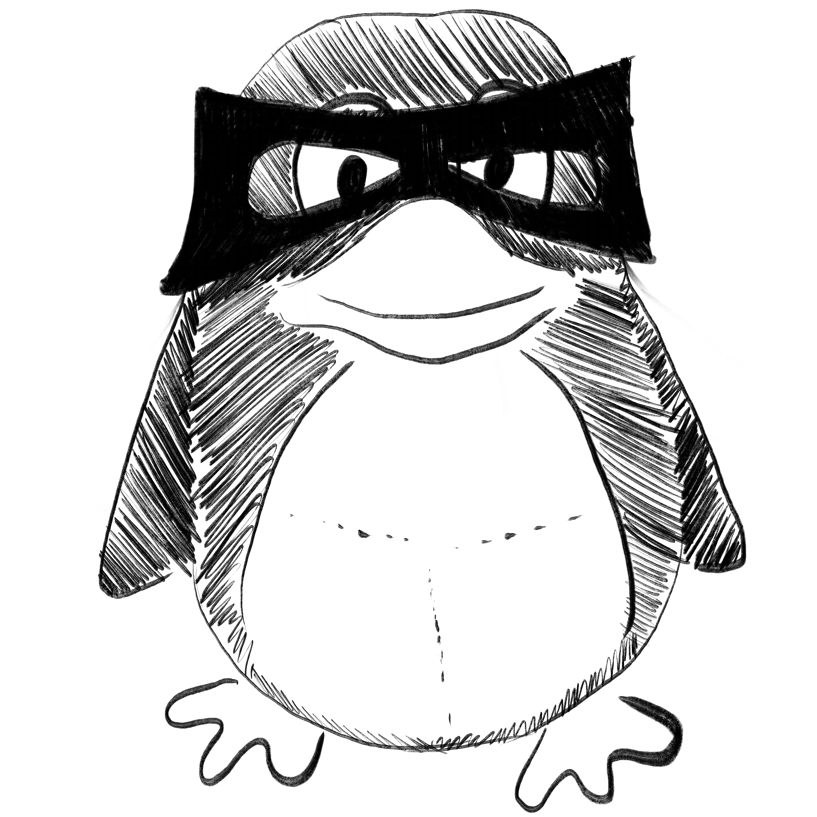
Speaking with mask in the COVID-19 era: Multiclass machine learning classification of acoustic and perceptual parameters.
In The Journal of the Acoustical Society of America
Calà F, Manfredi C, Battilocchi L, Frassineti L, Cantarella G
2023-Feb
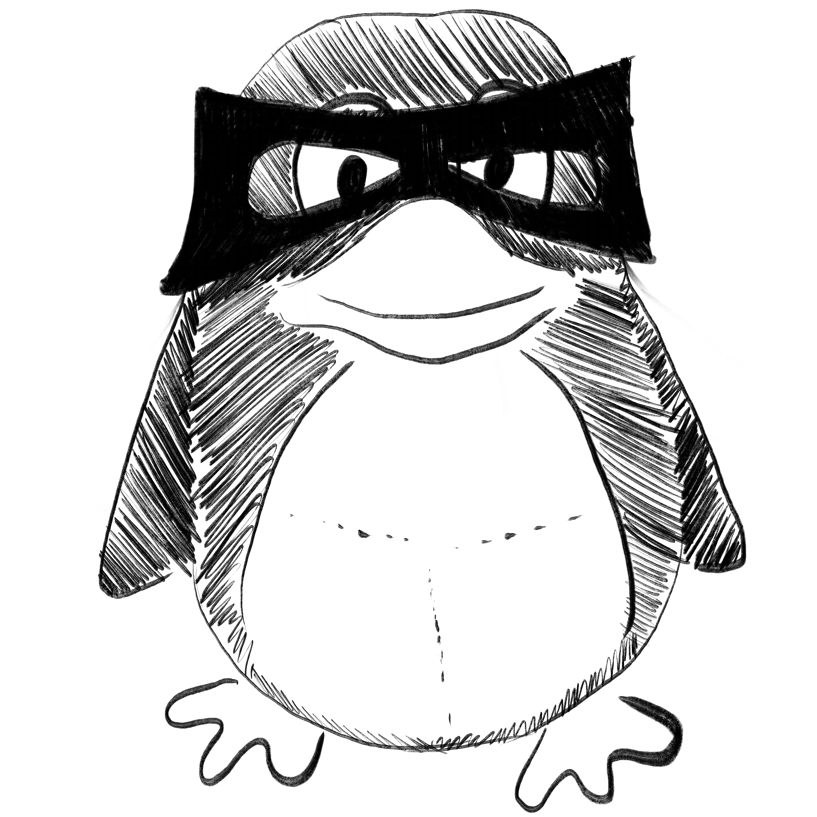
Using machine learning models to predict the willingness to carry lightweight goods by bike and kick-scooter.
In Transportation research interdisciplinary perspectives
Silveira-Santos Tulio, Manuel Vassallo Jose, Torres Ewerton
2022-Mar
Behavioral change, COVID-19, Classifier model, Machine learning, Shopping trips, Urban cycling and scooting
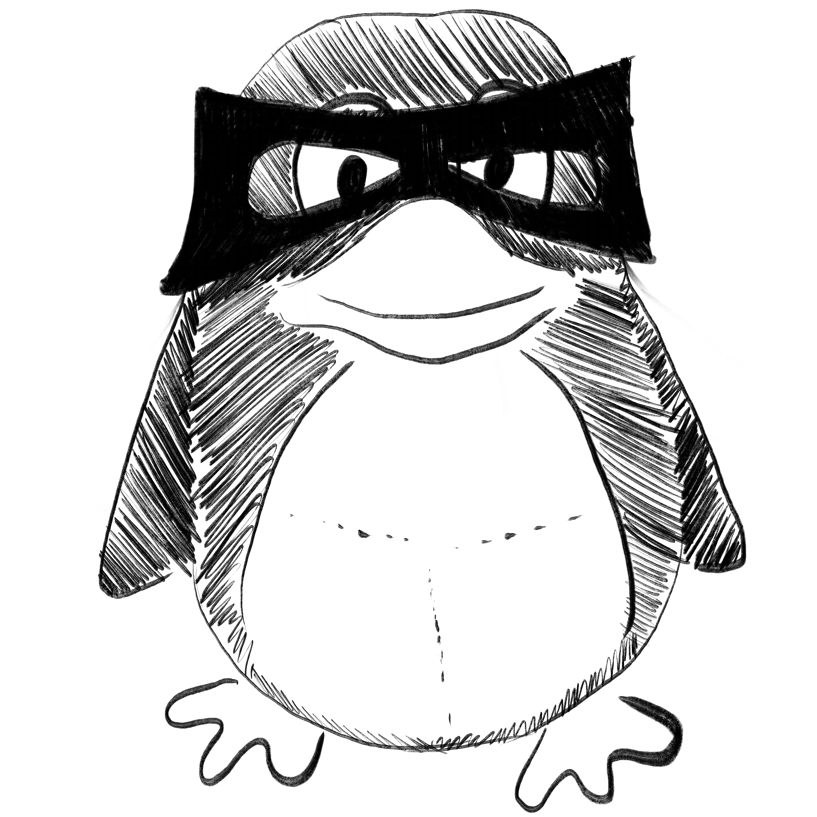
The role of digital social innovations to address SDGs: A systematic review.
In Environment, development and sustainability
Dionisio Marcelo, de Souza Junior Sylvio Jorge, Paula Fábio, Pellanda Paulo César
2023-Feb-23
Digital transformation, Industry 4.0, Social innovation, Systematic literature review, UN sustainable goals
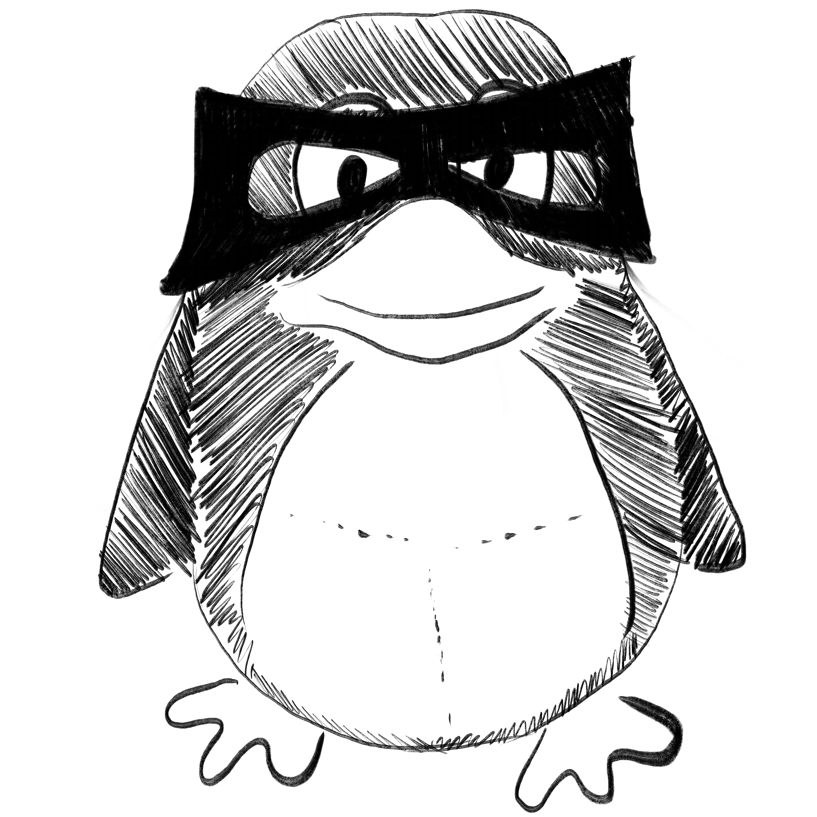
TransCode: Uncovering COVID-19 transmission patterns via deep learning.
In Infectious diseases of poverty ; h5-index 31.0
BACKGROUND :
METHODS :
RESULTS :
CONCLUSIONS :
Ren Jinfu, Liu Mutong, Liu Yang, Liu Jiming
2023-Feb-28
COVID-19, Deep transfer learning, Densely populated regions, Human mobility and contact behaviors, Meta-population, Spatiotemporal transmission dynamics and heterogeneity, TransCode
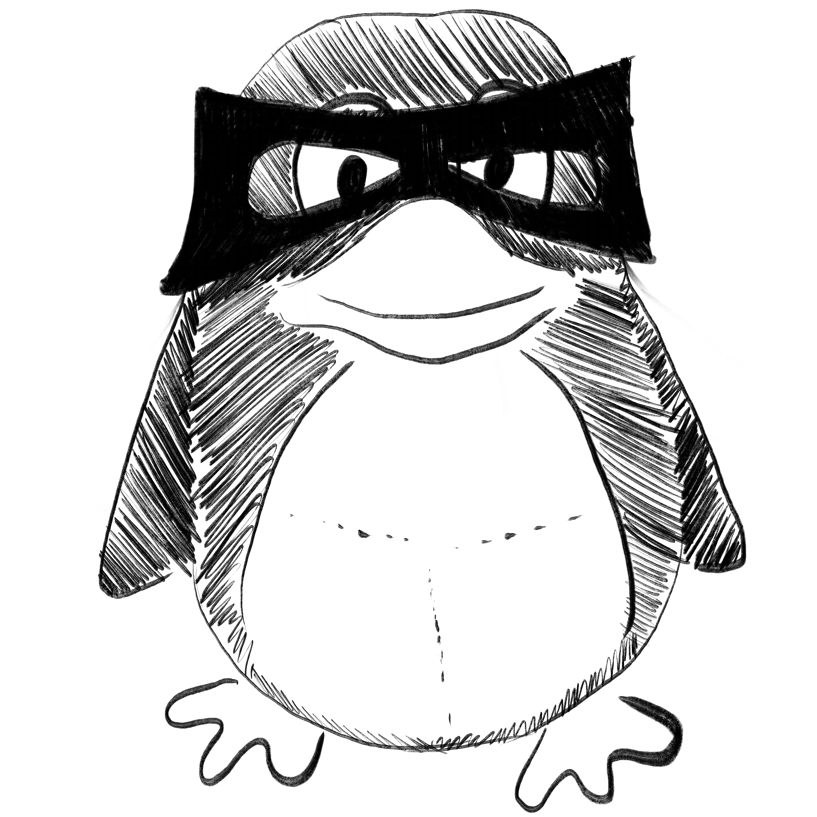
New, fast, and precise method of COVID-19 detection in nasopharyngeal and tracheal aspirate samples combining optical spectroscopy and machine learning.
In Brazilian journal of microbiology : [publication of the Brazilian Society for Microbiology]
Ceccon Denny M, Amaral Paulo Henrique R, Andrade Lídia M, da Silva Maria I N, Andrade Luis A F, Moraes Thais F S, Bagno Flavia F, Rocha Raissa P, de Almeida Marques Daisymara Priscila, Ferreira Geovane Marques, Lourenço Alice Aparecida, Ribeiro Ágata Lopes, Coelho-Dos-Reis Jordana G A, da Fonseca Flavio G, Gonzalez J C
2023-Feb-28
Artificial intelligence, COVID-19, Label-free diagnosis, Machine learning, Optical spectroscopy
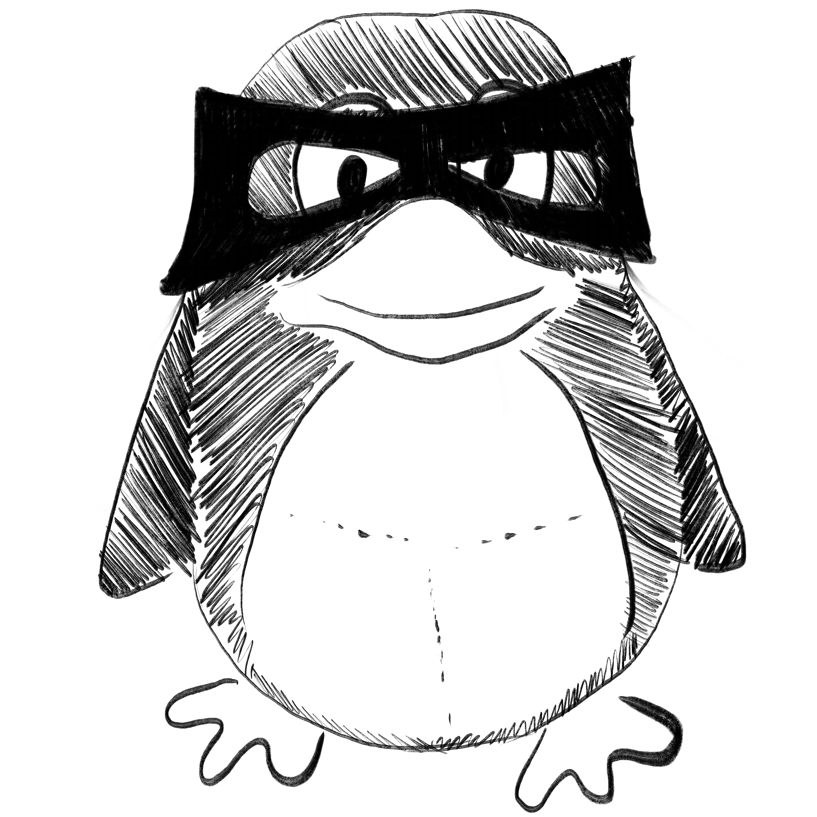
Open Science Discovery of Potent Non-Covalent SARS-CoV-2 Main Protease Inhibitors
bioRxiv Preprint
The COVID Moonshot Consortium, ; Achdout, H.; Aimon, A.; Alonzi, D. S.; Arbon, R.; Bar-David, E.; Barr, H.; Ben-Shmuel, A.; Bennett, J.; Bilenko, V. A.; Bilenko, V. A.; Boby, M. L.; Borden, B.; Boulet, P.; Bowman, G. R.; Brun, J.; Brwewitz, L.; BVNBS, S.; Calmiano, M.; Carbery, A.; Carney, D.; Cattermole, E.; Chang, E.; Chernyshenko, E.; Chodera, J. D.; Clyde, A.; Coffland, J. E.; Cohen, G.; Cole, J.; Contini, A.; Cox, L.; Croll, T. I.; Cvitkovic, M.; Dias, A.; Donckers, K.; Dotson, D. L.; Douangamath, A.; Duberstein, S.; Dudgeon, T.; Dunnett, L.; Eastman, P. K.; Erez, N.; Eyermann, C. J.; Fa
2023-03-02
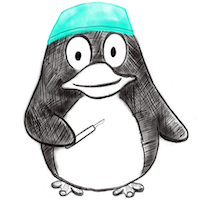
Multi-omics and immune cells' profiling of COVID-19 patients for ICU admission prediction: in silico analysis and an integrated machine learning-based approach in the framework of Predictive, Preventive, and Personalized Medicine.
In The EPMA journal
BACKGROUND :
METHODS :
RESULTS :
CONCLUSION :
SUPPLEMENTARY INFORMATION :
Zhu Kun, Chen Zhonghua, Xiao Yi, Lai Dengming, Wang Xiaofeng, Fang Xiangming, Shu Qiang
2023-Feb-21
COVID-19, CSF1R, Immune cells, Machine learning, Monocytes, Nomogram, PI16, Predictive Preventive Personalized medicine (PPPM / 3PM), Predictive model, Triage
Understanding Covid-19 Mobility Through Human Capital: A Unified Causal Framework.
In Computational economics
Bilgel Fırat, Karahasan Burhan Can
2023-Feb-21
Causal structure discovery, Do-calculus, Instrumental variables, Machine learning, Sample selection, Workplace mobility
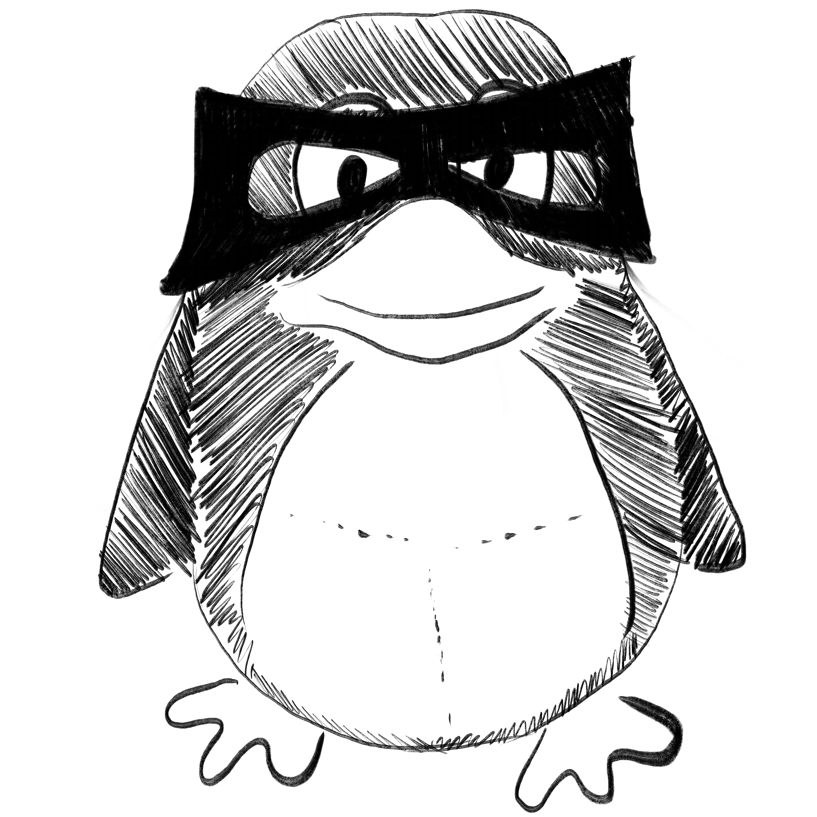
Detection of COVID-19 Case from Chest CT Images Using Deformable Deep Convolutional Neural Network.
In Journal of healthcare engineering
Foysal Md, Hossain A B M Aowlad, Yassine Abdulsalam, Hossain M Shamim
2023
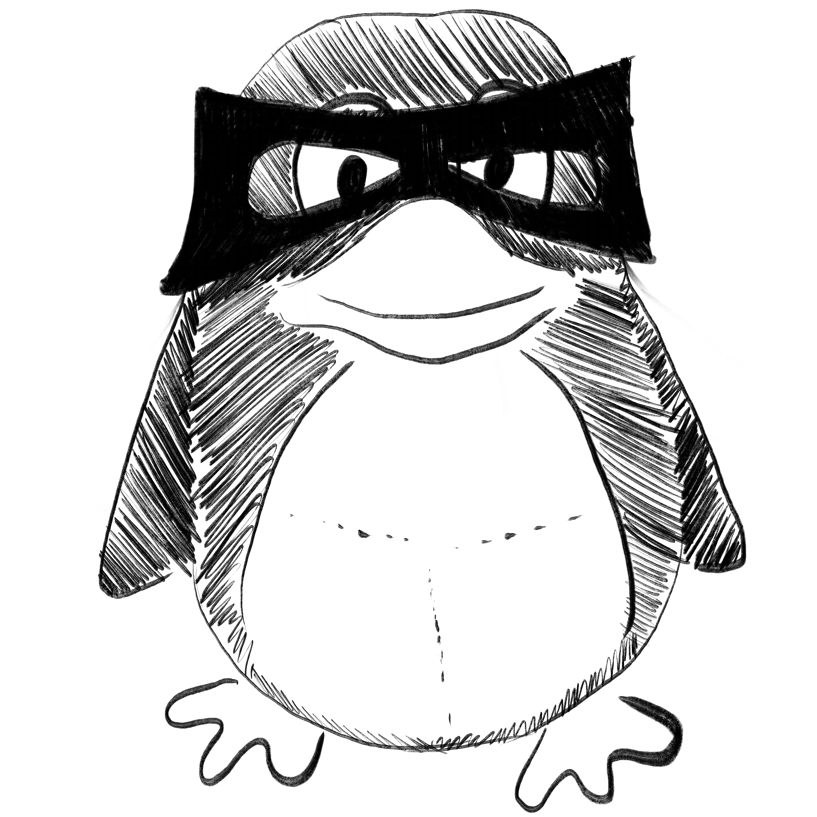
Classification modeling of intention to donate for victims of Typhoon Odette using deep learning neural network.
In Environmental development
German Josephine D, Ong Ardvin Kester S, Redi Anak Agung Ngurah Perwira, Prasetyo Yogi Tri, Robas Kirstien Paola E, Nadlifatin Reny, Chuenyindee Thanatorn
2023-Mar
Deep learning neural network, Donation, Natural disaster, Typhoon odette, Typhoon victims
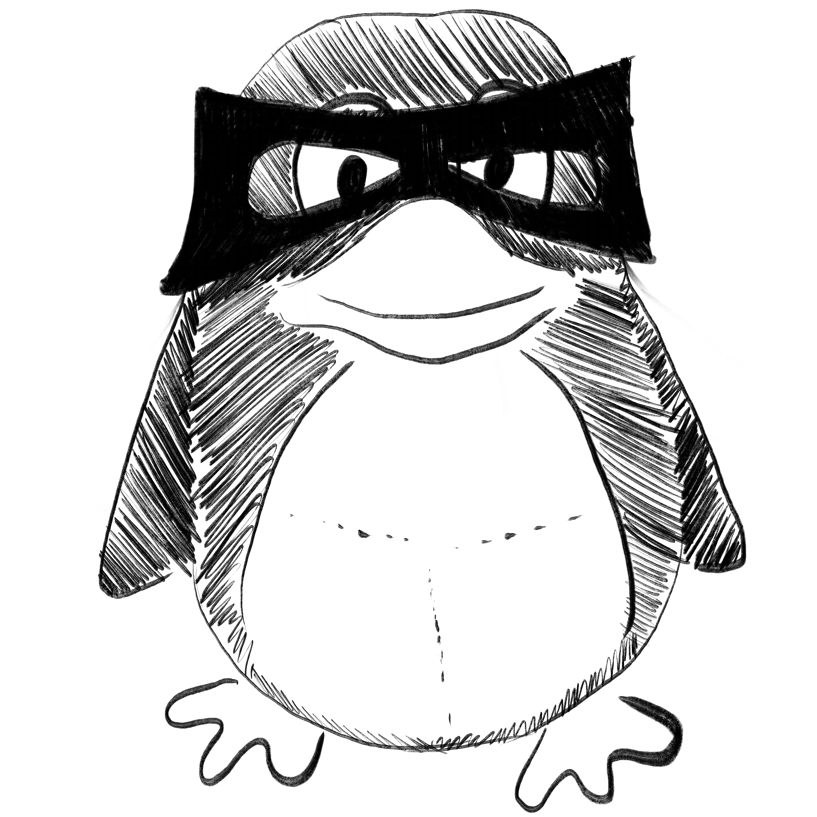
Emerging trends in point-of-care biosensing strategies for molecular architectures and antibodies of SARS-CoV-2.
In Biosensors & bioelectronics: X
Karuppaiah Gopi, Vashist Arti, Nair Madhavan, Veerapandian Murugan, Manickam Pandiaraj
2023-May
Biomarkers, COVID-19, Electrochemical and optical biosensors, Infectious diseases, Nanobiosensors
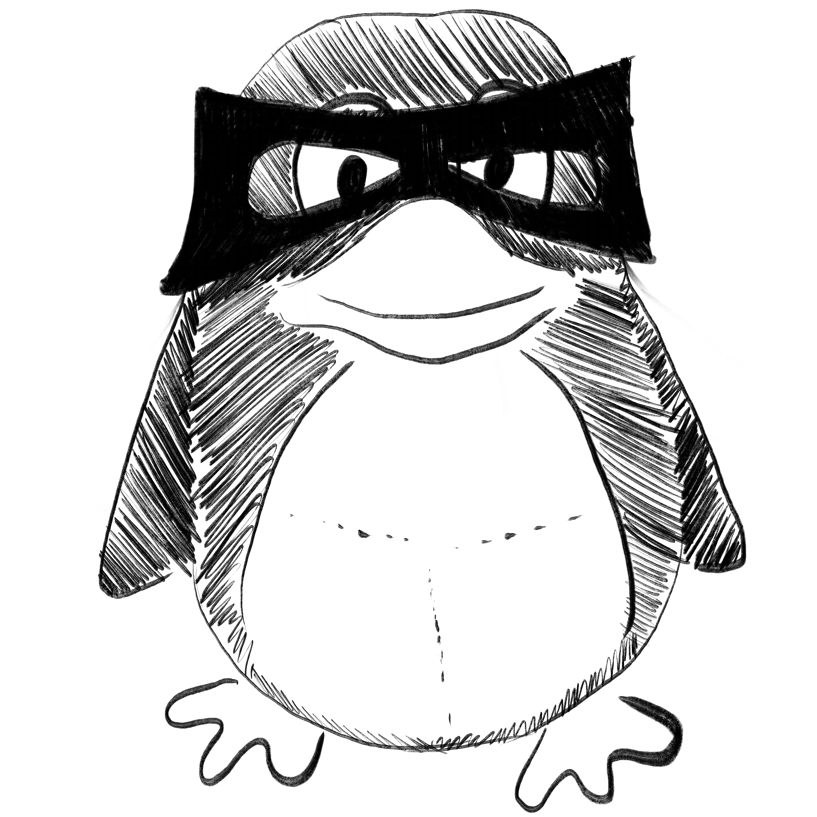
Exploration of SARS-CoV-2 Mpro Noncovalent Natural Inhibitors Using Structure-Based Approaches.
In ACS omega
Duong Cuong Quoc, Nguyen Phuong Thuy Viet
2023-Feb-21
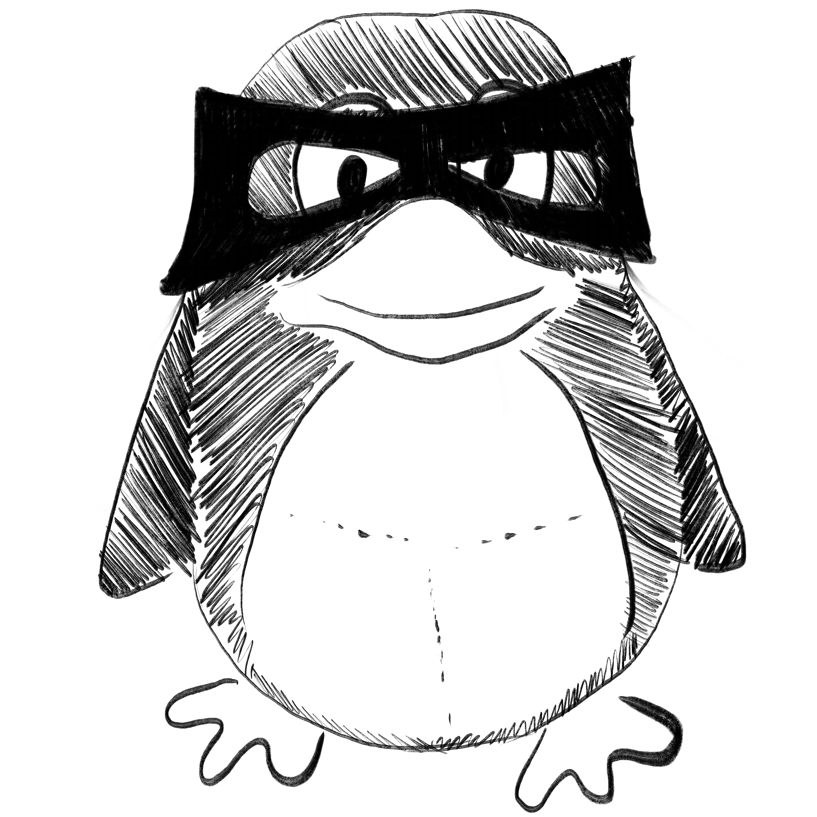
SARS-CoV-2 Diagnosis Using Transcriptome Data: A Machine Learning Approach.
In SN computer science
UNLABELLED :
SUPPLEMENTARY INFORMATION :
Jeyananthan Pratheeba
2023
COVID-19 diagnosis, Differently expressed genes, Feature selection, GO analysis, Machine learning models, Transcriptome data
Detecting Tweets Containing Cannabidiol-Related COVID-19 Misinformation Using Transformer Language Models and Warning Letters From Food and Drug Administration: Content Analysis and Identification.
In JMIR infodemiology
BACKGROUND :
OBJECTIVE :
METHODS :
RESULTS :
CONCLUSIONS :
Turner Jason, Kantardzic Mehmed, Vickers-Smith Rachel, Brown Andrew G
2023
COVID-19, Twitter, cannabidiol, content analysis, deep learning, health information, infodemic, infodemiology, language model, misinformation, pandemic, sentence vector, social media, transformer
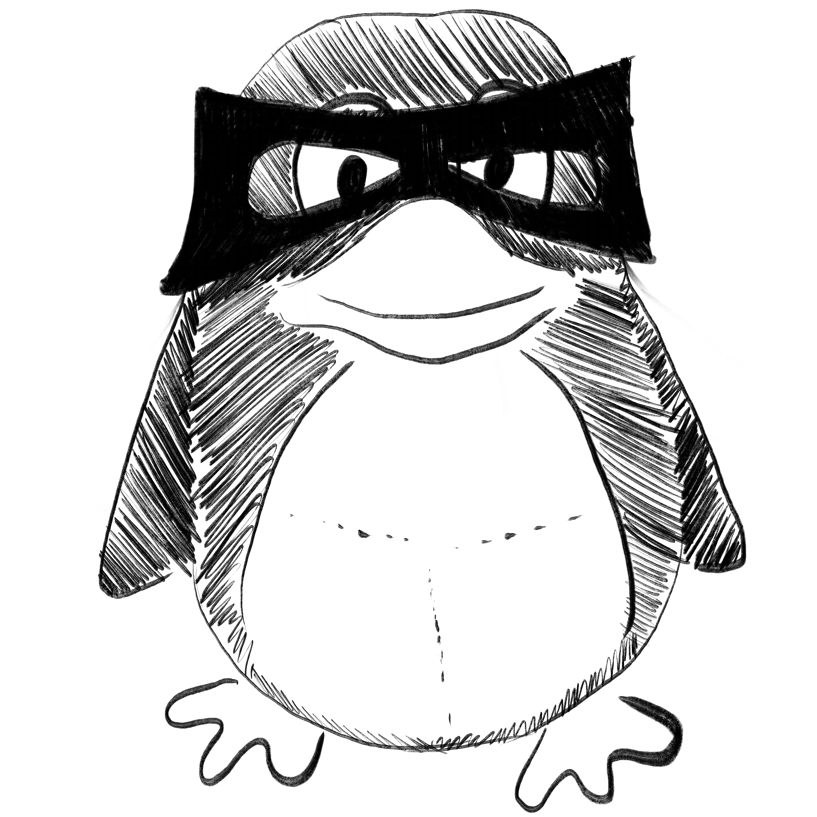
MonkeyNet: A robust deep convolutional neural network for monkeypox disease detection and classification.
In Neural networks : the official journal of the International Neural Network Society
Bala Diponkor, Hossain Md Shamim, Hossain Mohammad Alamgir, Abdullah Md Ibrahim, Rahman Md Mizanur, Manavalan Balachandran, Gu Naijie, Islam Mohammad S, Huang Zhangjin
2023-Feb-22
Classification, Convolutional neural network, Dataset, Deep learning, Machine learning, Monkeypox disease
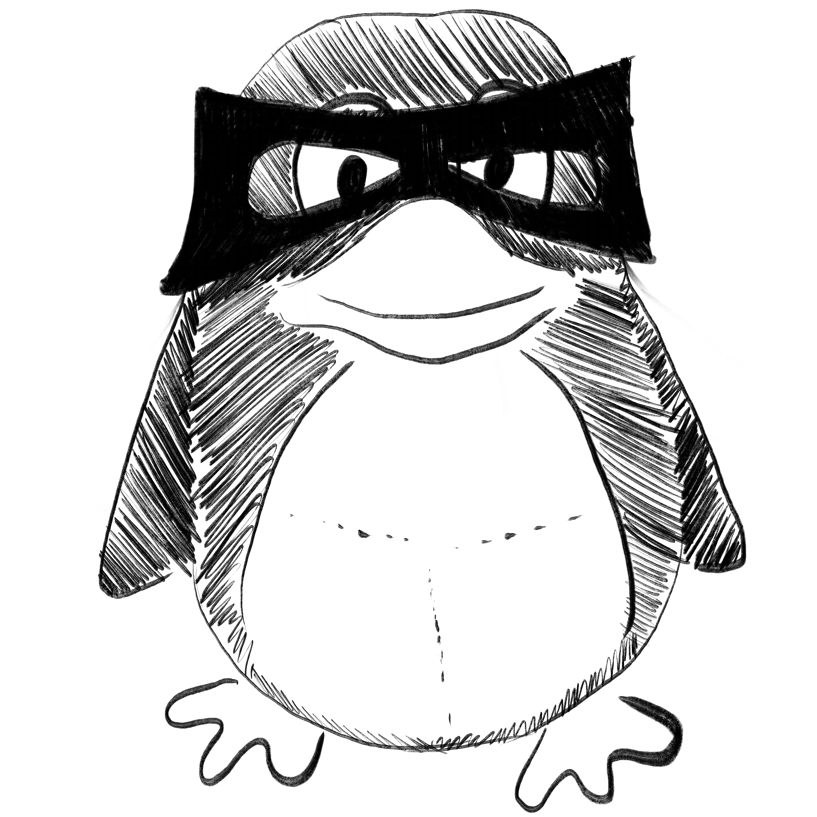
How to avoid a local epidemic becoming a global pandemic.
In Proceedings of the National Academy of Sciences of the United States of America
Stenseth Nils Chr, Schlatte Rudolf, Liu Xiaoli, Pielke Roger, Li Ruiyun, Chen Bin, Bjørnstad Ottar N, Kusnezov Dimitri, Gao George F, Fraser Christophe, Whittington Jason D, Bai Yuqi, Deng Ke, Gong Peng, Guan Dabo, Xiao Yixiong, Xu Bing, Johnsen Einar Broch
2023-Mar-07
Disease X, coupled simulation model, data science, digital twin model, epidemiology
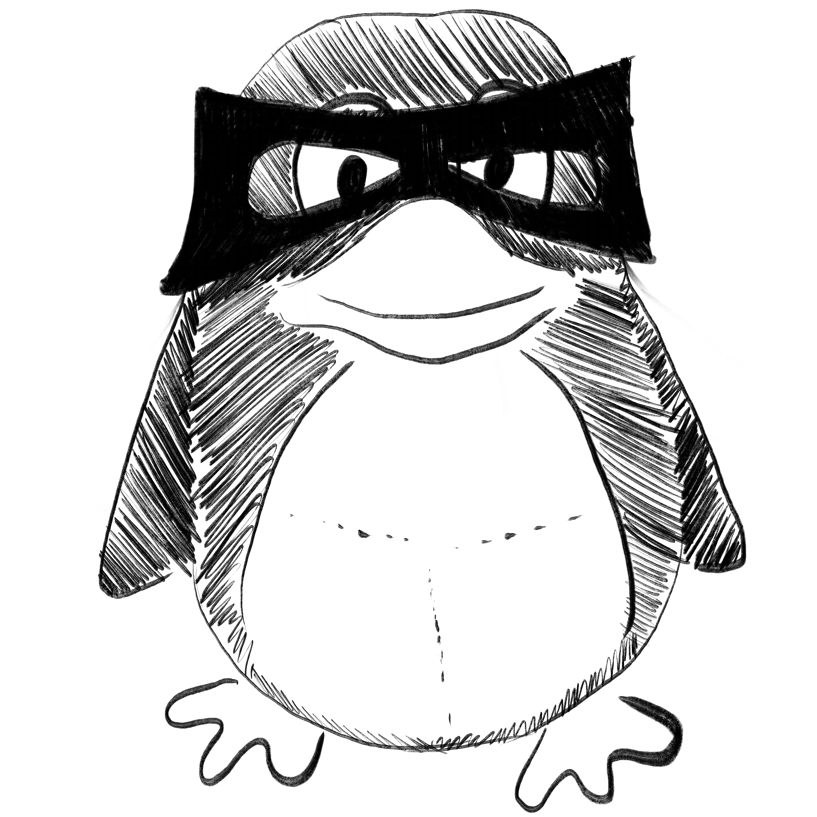
Temporal analysis of academic performance in higher education before, during and after COVID-19 confinement using artificial intelligence.
In PloS one ; h5-index 176.0
Subirats Laia, Palacios Corral Aina, Pérez-Ruiz Sof Ia, Fort Santi, Sacha Go Mez-Mon Ivas
2023
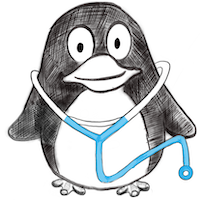
Evaluation of EfficientNet models for COVID-19 detection using lung parenchyma.
In Neural computing & applications
Kurt Zuhal, Işık Şahin, Kaya Zeynep, Anagün Yıldıray, Koca Nizameddin, Çiçek Sümeyye
2023-Feb-20
COVID-19 detection, CT scan, Deep learning, EfficientNet, K-means, Lung parenchyma
Impact of human mobility on COVID-19 transmission according to mobility distance, locations and demographic factors in the Greater Bay area of China:a population-based study.
In JMIR public health and surveillance
BACKGROUND :
OBJECTIVE :
METHODS :
RESULTS :
CONCLUSIONS :
Xia Jizhe, Yin Kun, Yue Yang, Li Qingquan, Wang Xiling, Hu Dongsheng, Wang Xiong, Du Zhanwei, Cowling Ben J, Chen Erzhen, Zhou Ying
2023-Feb-23
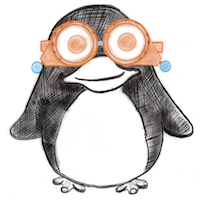
Glaucoma and Telemedicine.
In Journal of glaucoma
Brandão-de-Resende Camilo, de Alcântara Liliane de Abreu Rosa, Vasconcelos-Santos Daniel Vítor, Diniz-Filho Alberto
2023-Feb-28
Artificial Intelligence Functionalities During the COVID-19 Pandemic.
In Disaster medicine and public health preparedness
BACKGROUND :
METHODS :
RESULTS :
CONCLUSIONS :
Ahmadi Marzaleh Milad, Peyravi Mahmoudreza, Mousavi Shahrokh, Sarpourian Fatemeh, Seyedi Milad, Shalyari Naseh
2023-Feb-27
COVID-19, artificial intelligence, deep learning, machine learning, neural networks
Can we predict critical care mortality with non-conventional inflammatory markers in SARS-CoV-2 infected patients?
In Clinical hemorheology and microcirculation
BACKGROUND :
OBJECTIVE :
METHODS :
RESULTS :
CONCLUSIONS :
Rozanovic Martin, Domokos Kamilla, Márovics Gergő, Rohonczi Mirtill, Csontos Csaba, Bogár Lajos, Rendeki Szilárd, Kiss Tamás, Rozanovic Melánia Nacira, Loibl Csaba
2023-Feb-18
C-reactive protein, COVID-19, infection, inflammatory response, leukocyte antisedimentation rate, neutrophil-lymphocyte ratio, procalcitonin
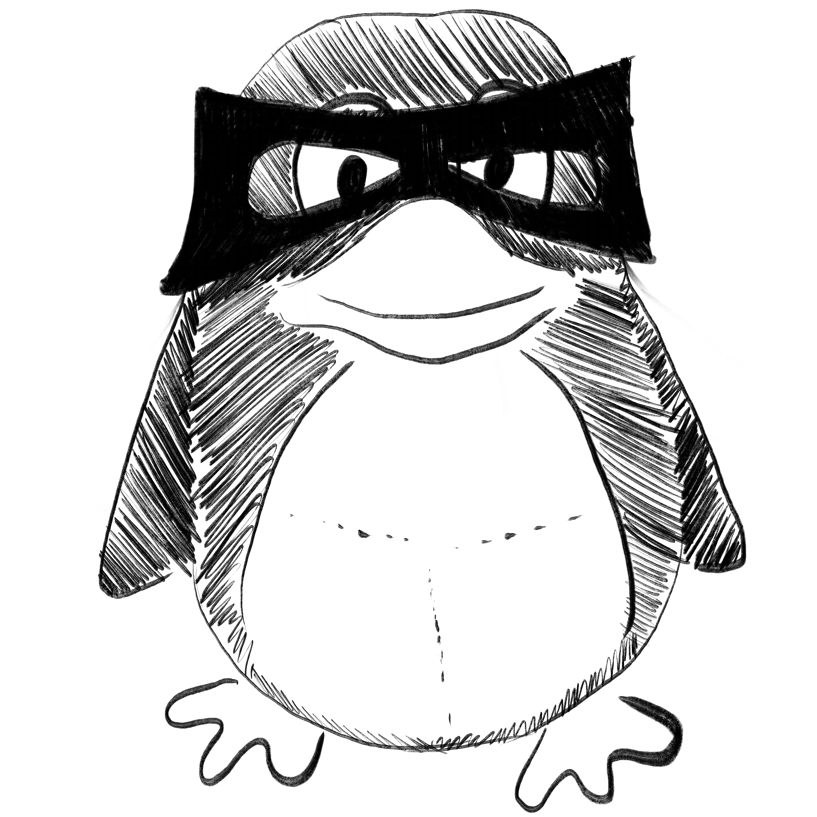
A new sentiment analysis method to detect and Analyse sentiments of Covid-19 moroccan tweets using a recommender approach.
In Multimedia tools and applications
Madani Youness, Erritali Mohammed, Bouikhalene Belaid
2023-Feb-22
Classification, Collaborative filtering, Covid-19, Recommendation system, Sentiment analysis
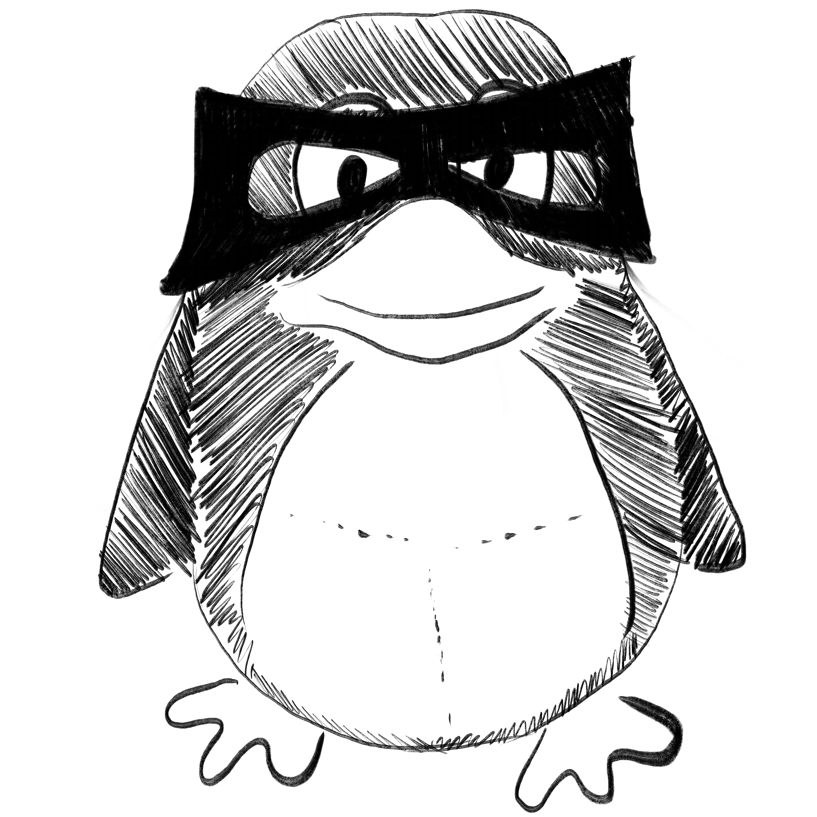
Conv-CapsNet: capsule based network for COVID-19 detection through X-Ray scans.
In Multimedia tools and applications
Sharma Pulkit, Arya Rhythm, Verma Richa, Verma Bindu
2023-Feb-21
COVID-19 detection, Capsule networks, Chest X-ray classification, Medical imaging
Visual Positioning of Nasal Swab Robot Based on Hierarchical Decision.
In Journal of Shanghai Jiaotong University (science)
Li Guozhi, Zou Shuizhong, Ding Shuxue
2023-Feb-21
hierarchical decision, nasal swab sampling, surgical robot, vision servo
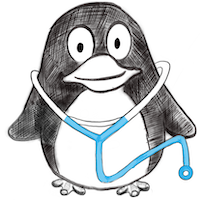
A dataset of COVID-19 x-ray chest images.
In Data in brief
Fraiwan Mohammad, Khasawneh Natheer, Khassawneh Basheer, Ibnian Ali
2023-Apr
Artificial intelligence, COVID-19, Chest X-ray, Deep learning, Detection, Diagnosis
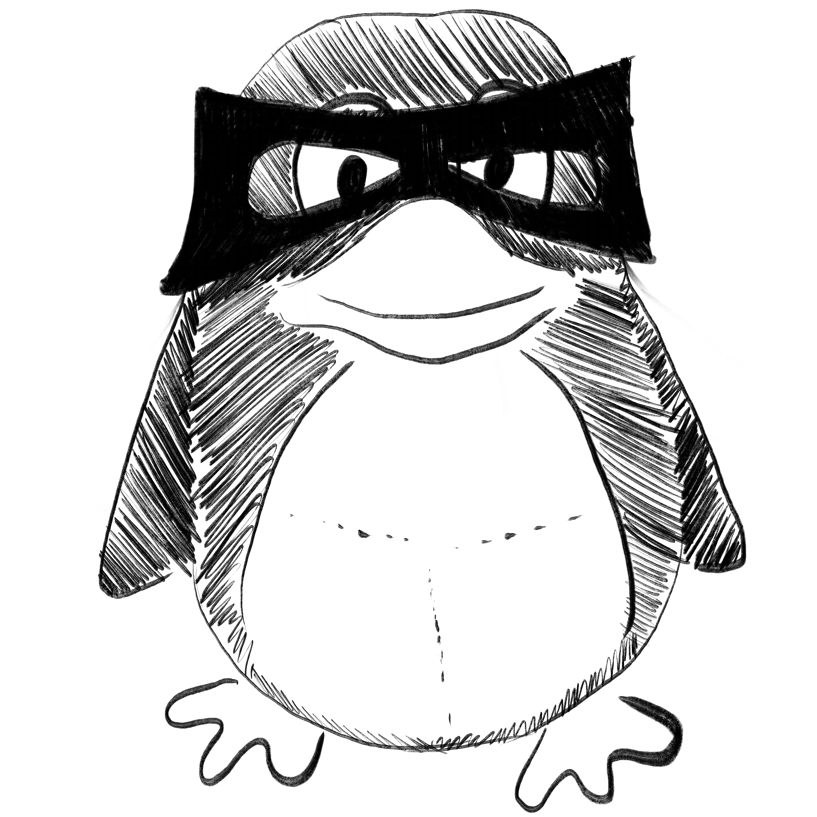
Investigating brain cortical activity in patients with post-COVID-19 brain fog.
In Frontiers in neuroscience ; h5-index 72.0
Wojcik Grzegorz M, Shriki Oren, Kwasniewicz Lukasz, Kawiak Andrzej, Ben-Horin Yarden, Furman Sagi, Wróbel Krzysztof, Bartosik Bernadetta, Panas Ewelina
2023
COVID-19, EEG, ERP, LDA, brain fog, cortical activity
Barriers to and solutions for representative inclusion across the lifespan and in life course research: The need for structural competency highlighted by the COVID-19 pandemic.
In Journal of clinical and translational science
LeCroy Madison N, Potter Lindsey N, Bandeen-Roche Karen, Bianco Monica E, Cappola Anne R, Carter Ebony B, Dayan Peter S, Eckstrom Elizabeth, Edwards Dorothy F, Farabi Sarah S, Fisher Sheehan D, Giordano Judy, Hanson Heidi A, Jenkins Emerald, Juhn Young, Kaskel Frederick, Stake Christine E, Reeds Dominic N, Schleiss Mark R, Wafford Q Eileen, McColley Susanna A
2023
Life course research, research participation, social determinants of health, special populations, structural competency
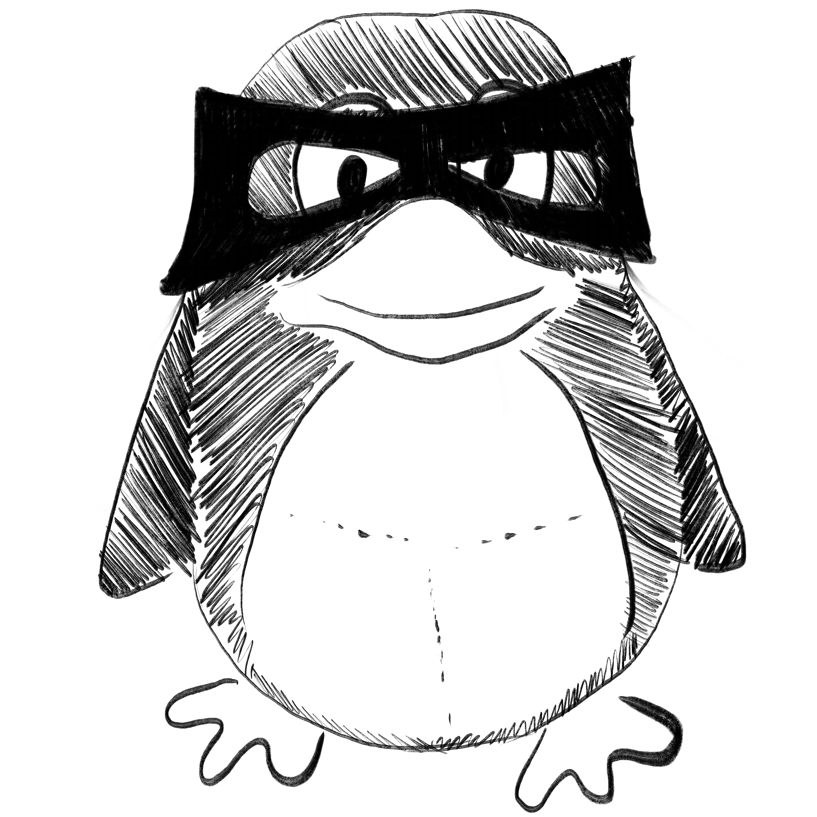
Multi-scale Triplet Hashing for Medical Image Retrieval.
In Computers in biology and medicine
Chen Yaxiong, Tang Yibo, Huang Jinghao, Xiong Shengwu
2023-Feb-08
Convolutional self-attention, Deep hashing, Hierarchical similarity, Medical image retrieval
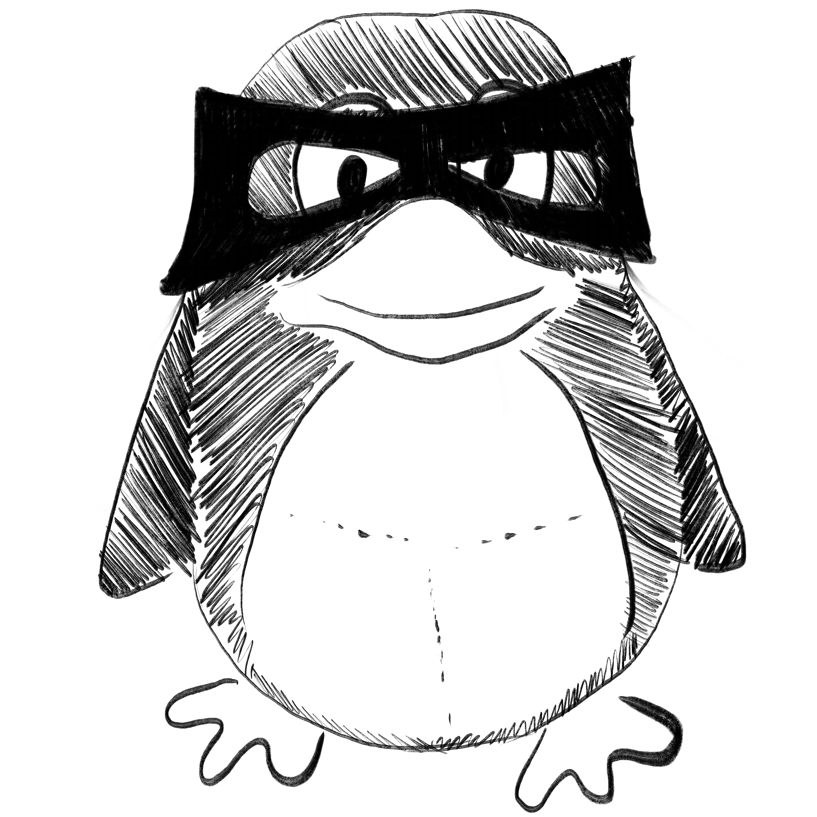
Machine Learning Guided Design of High-Affinity ACE2 Decoys for SARS-CoV-2 Neutralization.
In The journal of physical chemistry. B
Chan Matthew C, Chan Kui K, Procko Erik, Shukla Diwakar
2023-Feb-24
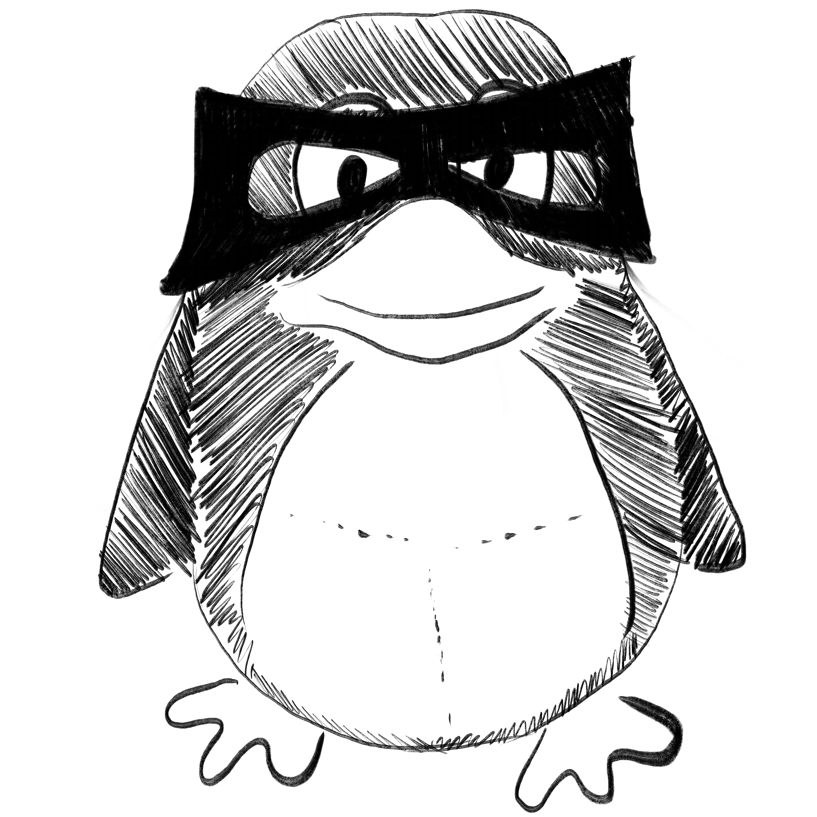
Novel Light Convolutional Neural Network for COVID Detection with Watershed Based Region Growing Segmentation.
In Journal of imaging
Khan Hassan Ali, Gong Xueqing, Bi Fenglin, Ali Rashid
2023-Feb-13
CNN, COVID-19, CT scans, X-rays, classification, convolutional neural network, segmentation, watershed segmentation
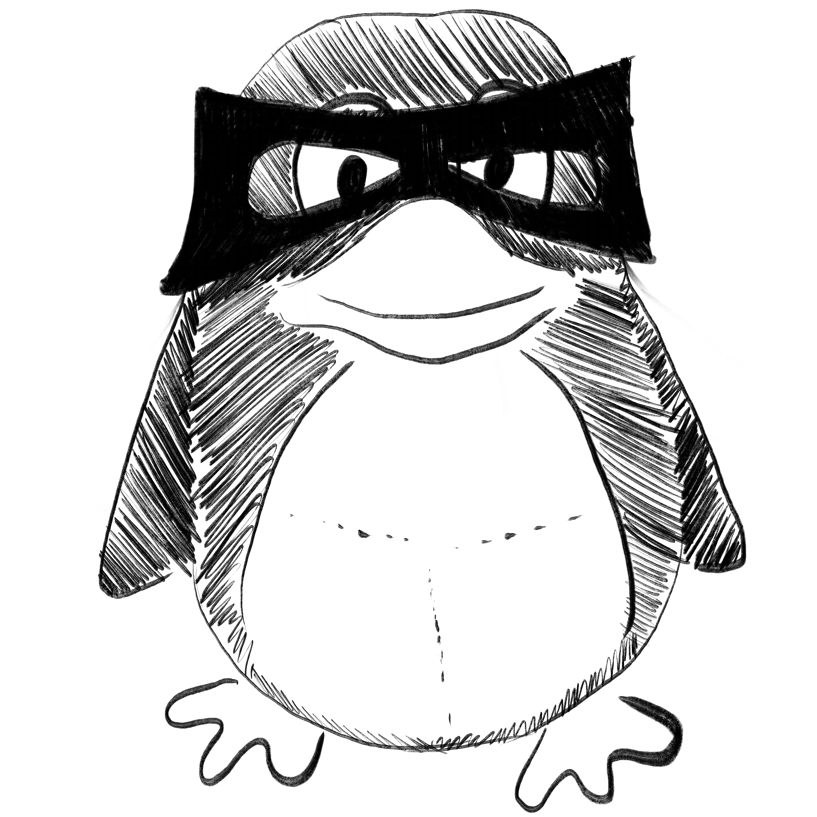
Masked Face Recognition Using Histogram-Based Recurrent Neural Network.
In Journal of imaging
Chong Wei-Jie Lucas, Chong Siew-Chin, Ong Thian-Song
2023-Feb-08
deep learning, histogram of gradients, masked face recognition, neural network, recurrent
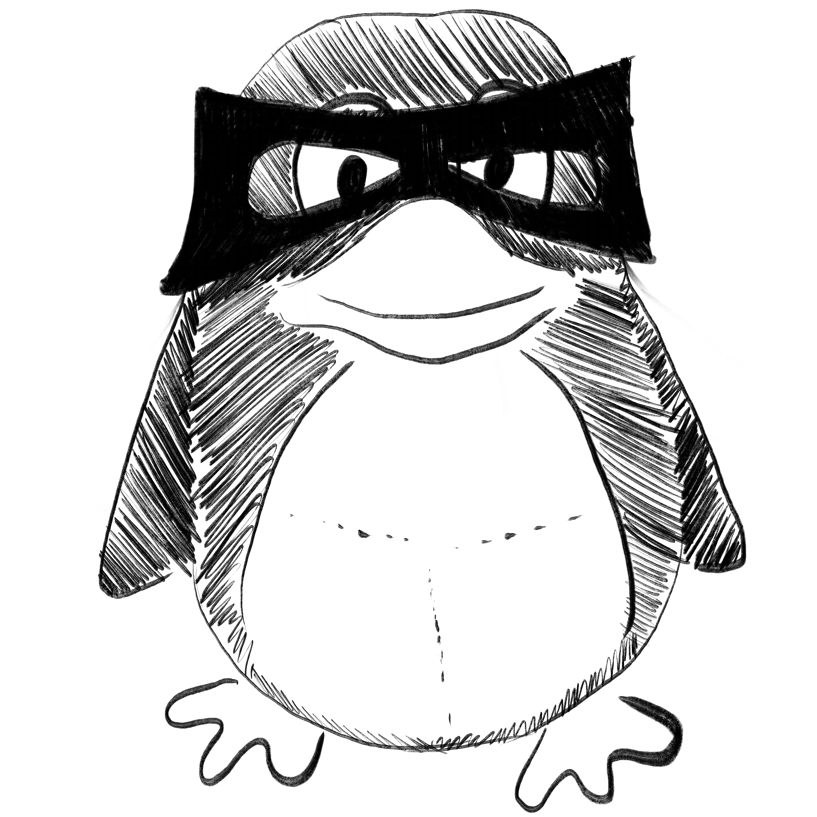
Impact of Wavelet Kernels on Predictive Capability of Radiomic Features: A Case Study on COVID-19 Chest X-ray Images.
In Journal of imaging
Prinzi Francesco, Militello Carmelo, Conti Vincenzo, Vitabile Salvatore
2023-Jan-30
COVID-19 prognosis, chest X-ray images, machine learning models, predictive capability, radiomic features, wavelet kernels, wavelet-derived features
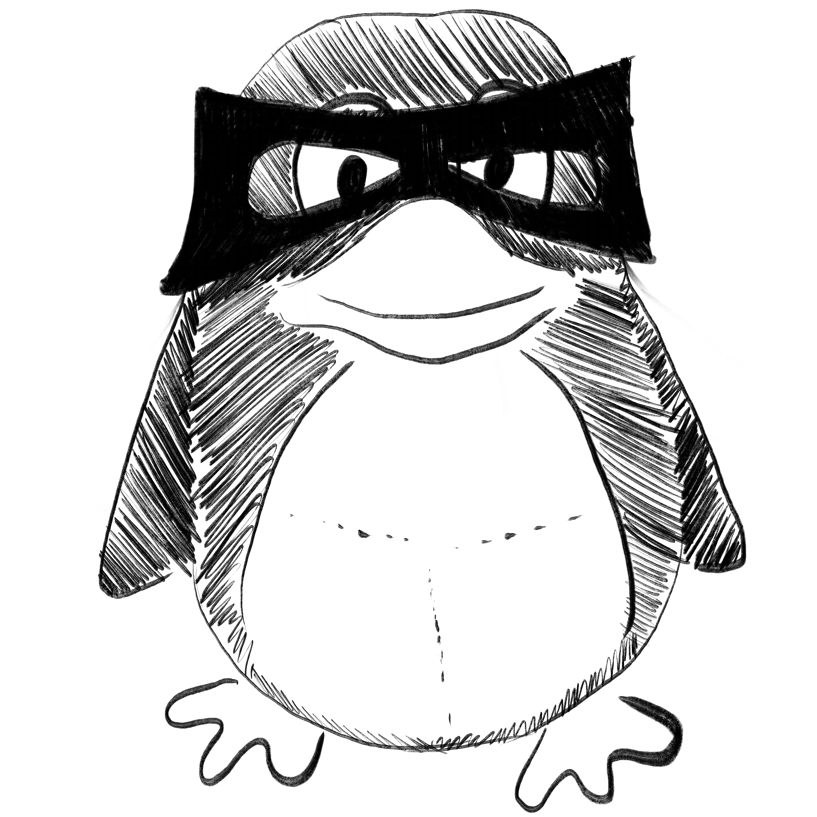
Cardiovascular and Renal Comorbidities Included into Neural Networks Predict the Outcome in COVID-19 Patients Admitted to an Intensive Care Unit: Three-Center, Cross-Validation, Age- and Sex-Matched Study.
In Journal of cardiovascular development and disease
Ovcharenko Evgeny, Kutikhin Anton, Gruzdeva Olga, Kuzmina Anastasia, Slesareva Tamara, Brusina Elena, Kudasheva Svetlana, Bondarenko Tatiana, Kuzmenko Svetlana, Osyaev Nikolay, Ivannikova Natalia, Vavin Grigory, Moses Vadim, Danilov Viacheslav, Komossky Egor, Klyshnikov Kirill
2023-Jan-23
C-reactive protein, COVID-19, blood urea nitrogen, chronic kidney disease, coronary artery disease, lymphocyte count, machine learning, neural networks, neutrophil-to-lymphocyte ratio, prognostication
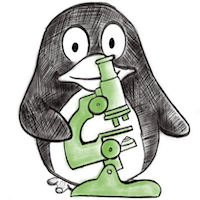
Variant-specific deleterious mutations in the SARS-CoV-2 genome reveal immune responses and potentials for prophylactic vaccine development.
In Frontiers in pharmacology
Islam Md Aminul, Shahi Shatila, Marzan Abdullah Al, Amin Mohammad Ruhul, Hasan Mohammad Nayeem, Hoque M Nazmul, Ghosh Ajit, Barua Abanti, Khan Abbas, Dhama Kuldeep, Chakraborty Chiranjib, Bhattacharya Prosun, Wei Dong-Qing
2023
COVID-19, SARS-CoV-2, deleterious mutation, delta variant, immune response, omicron variant, unique mutation, vaccine designing
Anti-vaccination attitude trends during the COVID-19 pandemic: A machine learning-based analysis of tweets.
In Digital health
OBJECTIVE :
METHODS :
RESULTS :
CONCLUSIONS :
To Quyen G, To Kien G, Huynh Van-Anh N, Nguyen Nhung Tq, Ngo Diep Tn, Alley Stephanie, Tran Anh Nq, Tran Anh Np, Pham Ngan Tt, Bui Thanh X, Vandelanotte Corneel
2023
deep learning, neural network, social media, stance analysis, twitter, vaccine hesitancy
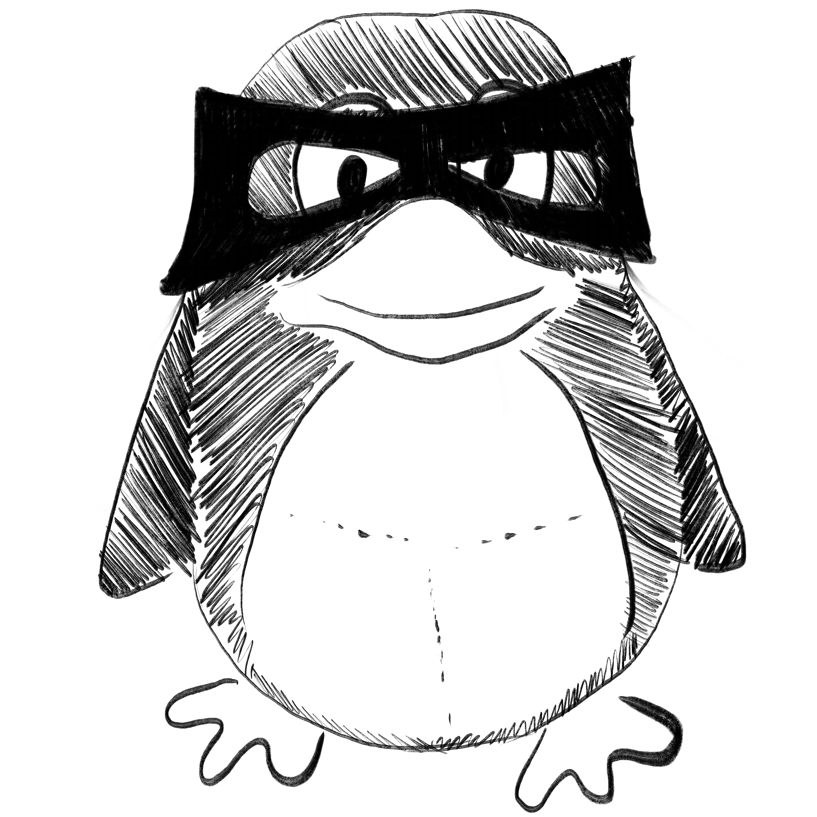
A wearable device for at-home obstructive sleep apnea assessment: State-of-the-art and research challenges.
In Frontiers in neurology
Tran Ngoc Thai, Tran Huu Nam, Mai Anh Tuan
2023
COVID-19, OSA, SCOPER, machine learning, wearable device
Targeted plasma proteomics reveals signatures discriminating COVID-19 from sepsis with pneumonia.
In Respiratory research ; h5-index 45.0
BACKGROUND :
METHODS :
RESULTS :
CONCLUSIONS :
Palma Medina Laura M, Babačić Haris, Dzidic Majda, Parke Åsa, Garcia Marina, Maleki Kimia T, Unge Christian, Lourda Magda, Kvedaraite Egle, Chen Puran, Muvva Jagadeeswara Rao, Cornillet Martin, Emgård Johanna, Moll Kirsten, Michaëlsson Jakob, Flodström-Tullberg Malin, Brighenti Susanna, Buggert Marcus, Mjösberg Jenny, Malmberg Karl-Johan, Sandberg Johan K, Gredmark-Russ Sara, Rooyackers Olav, Svensson Mattias, Chambers Benedict J, Eriksson Lars I, Pernemalm Maria, Björkström Niklas K, Aleman Soo, Ljunggren Hans-Gustaf, Klingström Jonas, Strålin Kristoffer, Norrby-Teglund Anna
2023-Feb-24
COVID-19, Community acquired pneumonia, Olink proximity extension assays, Sepsis, Septic shock
Mining of EHR for interface terminology concepts for annotating EHRs of COVID patients.
In BMC medical informatics and decision making ; h5-index 38.0
BACKGROUND :
METHODS :
RESULTS :
CONCLUSION :
Keloth Vipina K, Zhou Shuxin, Lindemann Luke, Zheng Ling, Elhanan Gai, Einstein Andrew J, Geller James, Perl Yehoshua
2023-Feb-24
COVID-19 ontologies, Concept mining, EHR annotation, Interface terminology
Association of statin use with outcomes of patients admitted with COVID-19: an analysis of electronic health records using superlearner.
In BMC infectious diseases ; h5-index 58.0
IMPORTANCE :
OBJECTIVE :
DESIGN, SETTING AND PARTICIPANTS :
EXPOSURE :
MAIN OUTCOME :
RESULTS :
CONCLUSIONS AND RELEVANCE :
Rivera Adovich S, Al-Heeti Omar, Petito Lucia C, Feinstein Mathew J, Achenbach Chad J, Williams Janna, Taiwo Babafemi
2023-Feb-24
COVID-19, Critical care, Mortality, Observational studies, Statin, Targeted maximum likelihood estimation
MSCCov19Net: multi-branch deep learning model for COVID-19 detection from cough sounds.
In Medical & biological engineering & computing ; h5-index 32.0
Ulukaya Sezer, Sarıca Ahmet Alp, Erdem Oğuzhan, Karaali Ali
2023-Feb-24
Coronavirus, Coughing, Deep learning, Ensemble learning, Telehealth
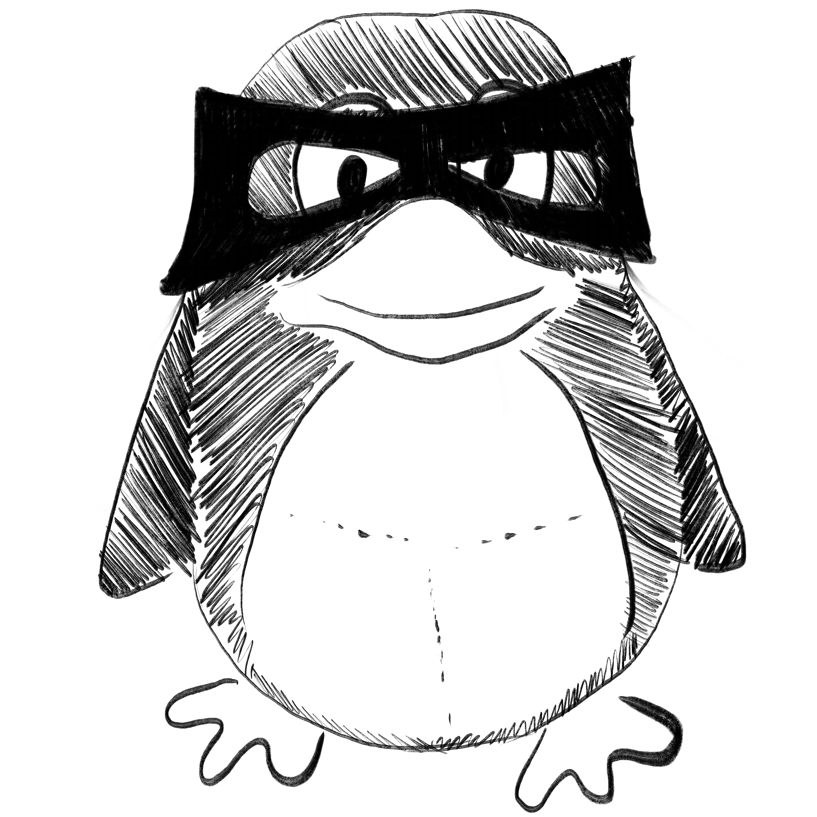
Machine learning on large scale perturbation screens for SARS-CoV-2 host factors identifies β-catenin/CBP inhibitor PRI-724 as a potent antiviral
bioRxiv Preprint
Kelch, M. A.; Vera-Guapi, A. L.; Beder, T.; Oswald, M.; Hiemisch, A.; Beil, N.; Wajda, P.; Ciesek, S.; Erfle, H.; Toptan, T.; König, R.
2023-02-24
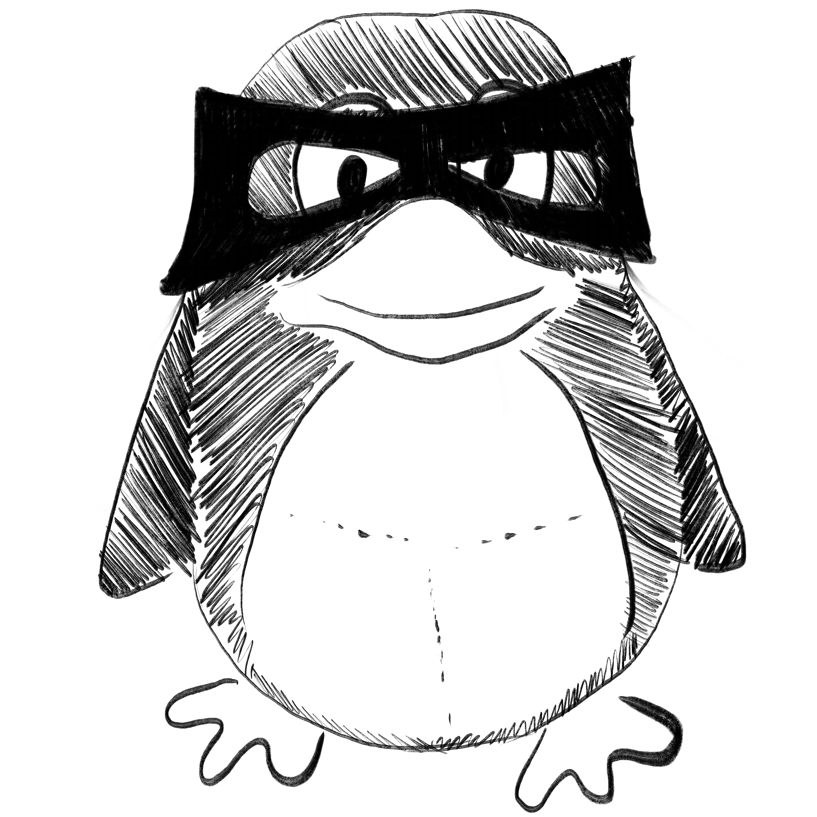
Make it or break it: On-time vaccination intent at the time of Covid-19.
In Vaccine ; h5-index 70.0
Bughin Jacques, Cincera Michele, Peters Kelly, Reykowska Dorota, Żyszkiewicz Marcin, Ohme Rafal
2023-Feb-08
Covid-19, Machine Learning, Random-forest, Social trust, Vaccine strategy
Telehealth utilization in U.S. medicare beneficiaries aged 65 years and older during the COVID-19 pandemic.
In BMC public health ; h5-index 82.0
BACKGROUND :
METHODS :
FINDINGS :
CONCLUSIONS :
Lu Min, Liao Xinyi
2023-Feb-20
COVID-19, Medicare, Older adults, Primary care, Telehealth, Telemedicine
A digital health platform to manage COVID-19: decentralizing technology to empower rural and remote jurisdictions.
In Rural and remote health
INTRODUCTION :
METHODS :
RESULTS :
DISCUSSION :
Katapally Tarun
2023-Jan
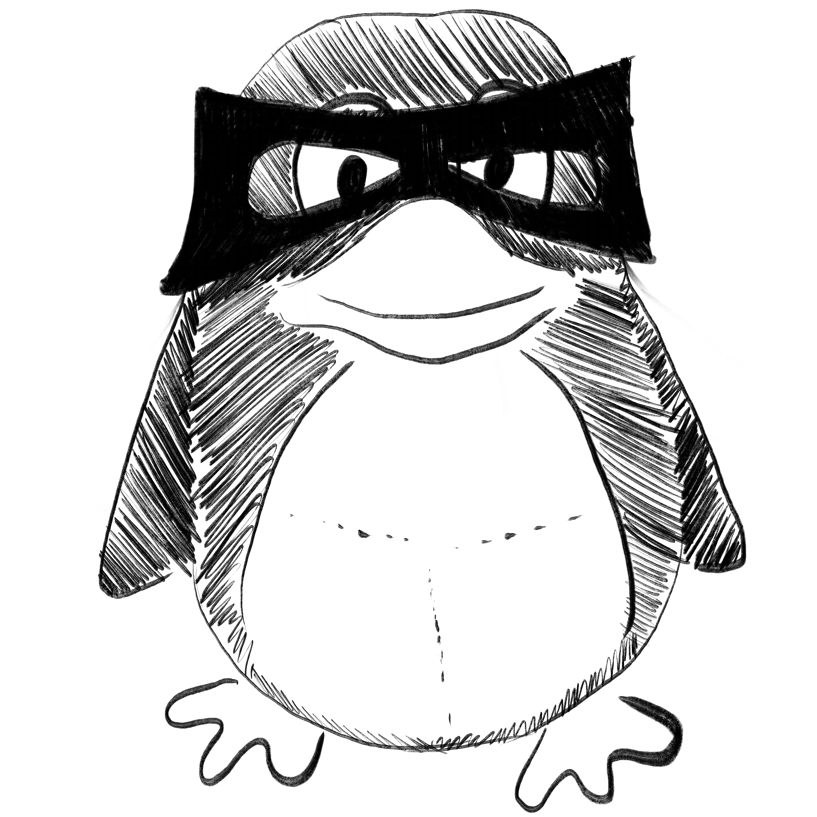
Analysis of COVID-19 vaccine adverse event using language model and unsupervised machine learning.
In PloS one ; h5-index 176.0
BACKGROUND :
METHODS AND FINDINGS :
CONCLUSIONS :
Cheon Saeyeon, Methiyothin Thanin, Ahn Insung
2023
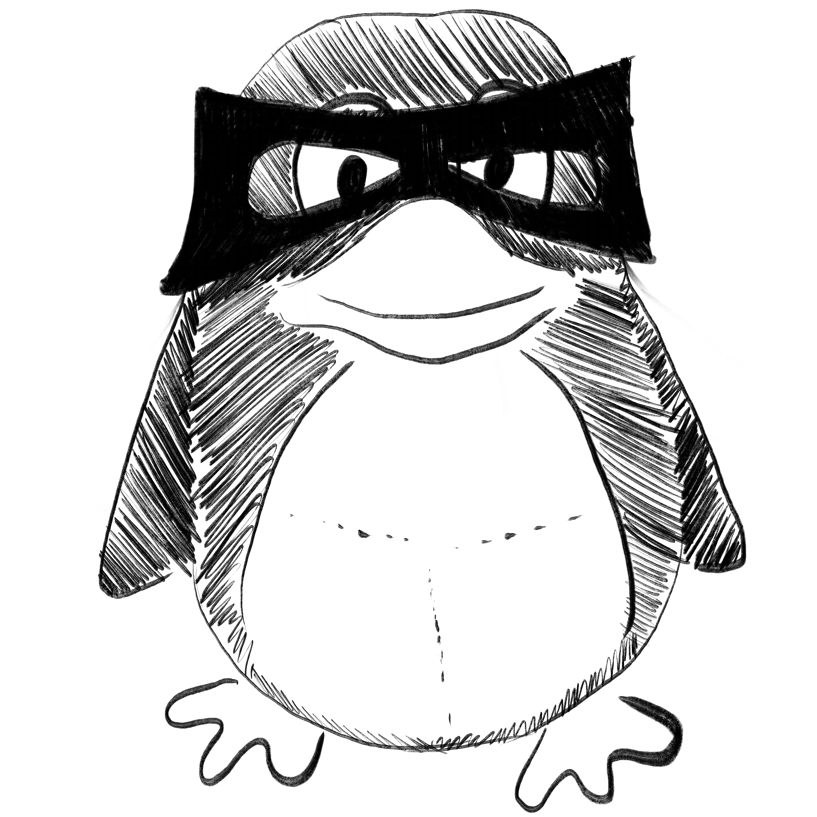
Comprehensive classification of proteins based on structures that engage lipids by COMPOSEL.
In Biophysical chemistry
Overduin Michael, Kervin Troy A, Klarenbach Zachary, Adra Trixie Rae C, Bhat Rakesh K
2023-Feb-08
Bilayer insertion, Intrinsically disordered region, Ligand complex, Lipid interaction, Monotopic, Myristoylation, Palmitoylation, Peripheral membrane domain, Polytopic, Protein classification, Transmembrane
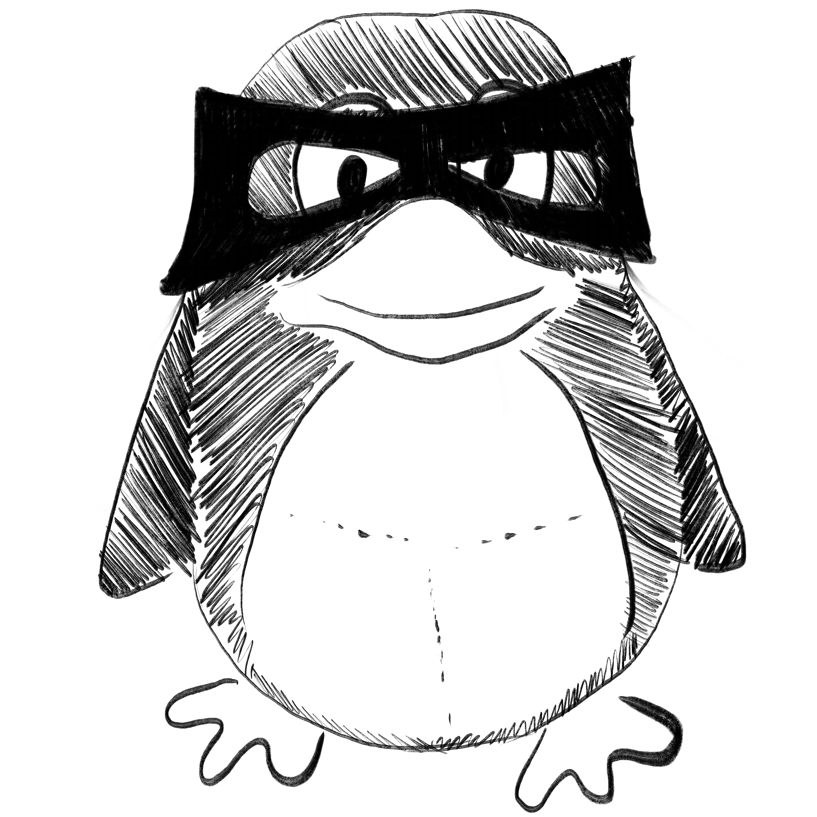
Interpreting biologically informed neural networks for enhanced biomarker discovery and pathway analysis
bioRxiv Preprint
Hartman, E.; Scott, A. M.; Malmström, L.; Malmström, J.
2023-02-21
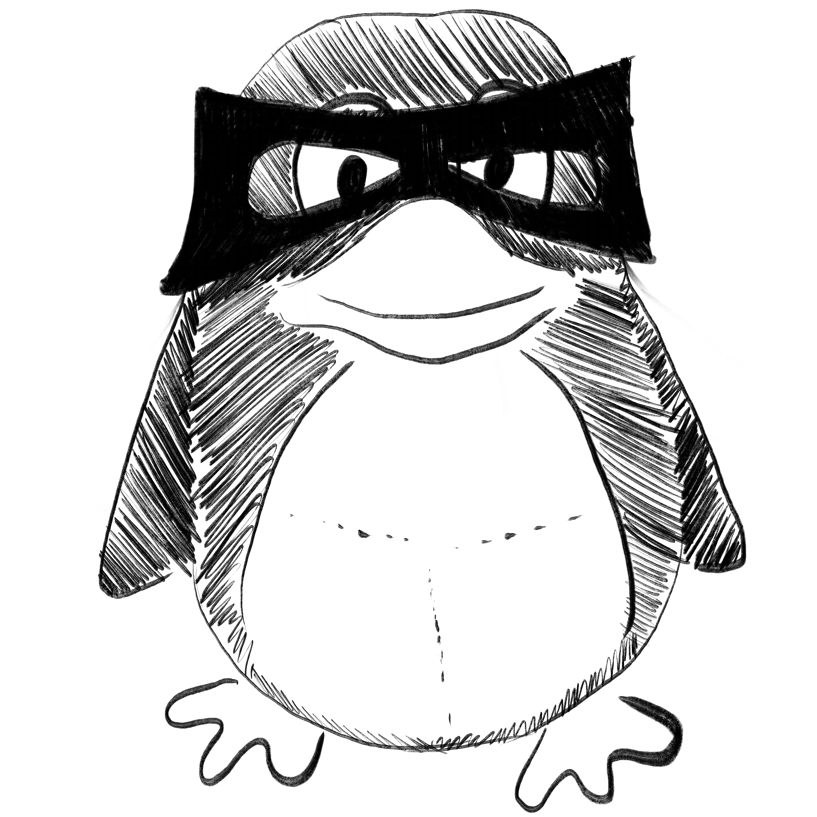
Multilingual Content Moderation: A Case Study on Reddit
ArXiv Preprint
Meng Ye, Karan Sikka, Katherine Atwell, Sabit Hassan, Ajay Divakaran, Malihe Alikhani
2023-02-19
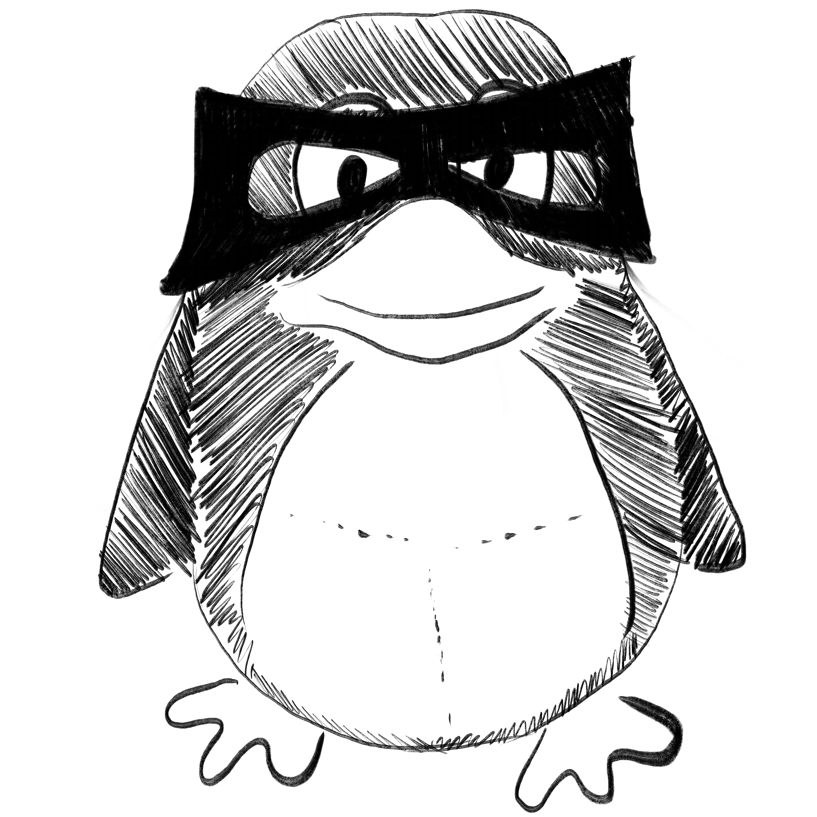
Understanding how the use of AI decision support tools affect critical thinking and over-reliance on technology by drug dispensers in Tanzania
ArXiv Preprint
Ally Jr Salim, Megan Allen, Kelvin Mariki, Kevin James Masoy, Jafary Liana
2023-02-19
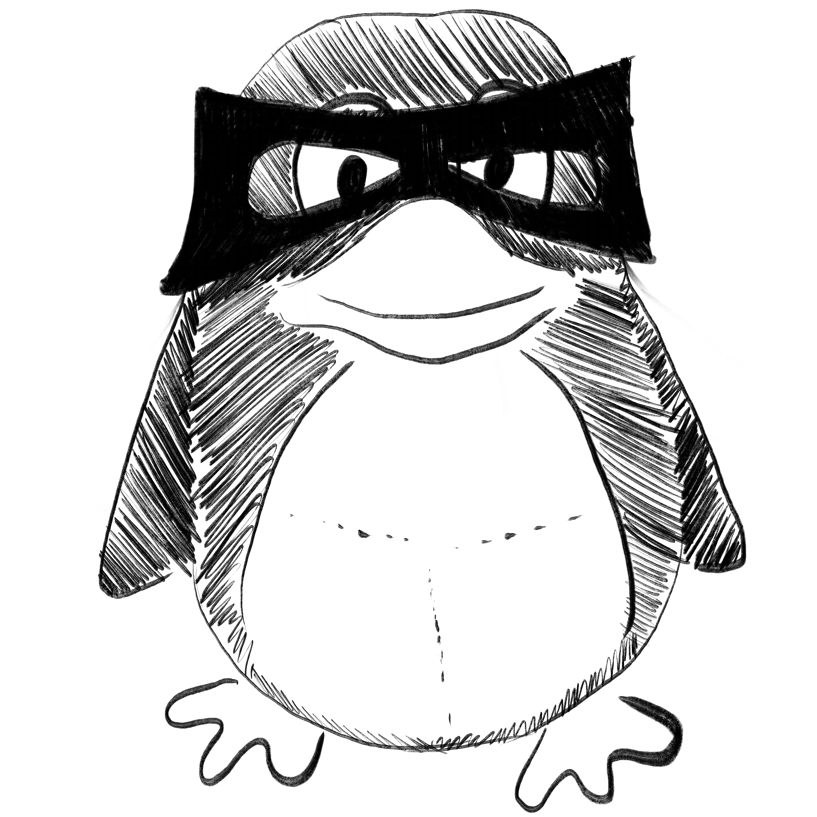
Turning any bed into an intensive care unit with the Internet of things and artificial intelligence technology. Presenting the enhanced mechanical ventilator.
In F1000Research
Pulido Morales Leidy Lorena, Buitrago Romero Juan Sebastian, Ardila Sanchez Ismael A, Yepes-Calderon Fernando
2022
AWS implementations, Artificial Neuronal Networks in medicine, Artificial intelligence in medicine, Covid 19 mitigation, Evalu@ implementations, Intense care units everywhere, Technology in healthcare, mechanical ventilators
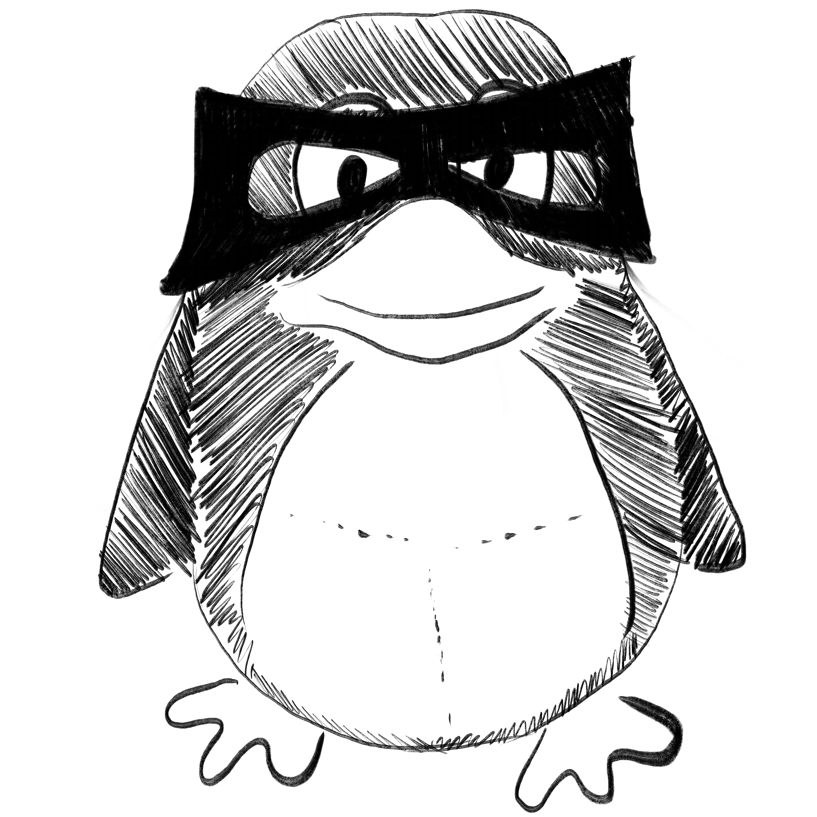
Infrared image method for possible COVID-19 detection through febrile and subfebrile people screening.
In Journal of thermal biology
Brioschi Marcos Leal, Dalmaso Neto Carlos, Toledo Marcos de, Neves Eduardo Borba, Vargas José Viriato Coelho, Teixeira Manoel Jacobsen
2023-Feb
Artificial intelligence, Convolutional neural network, Infrared imaging
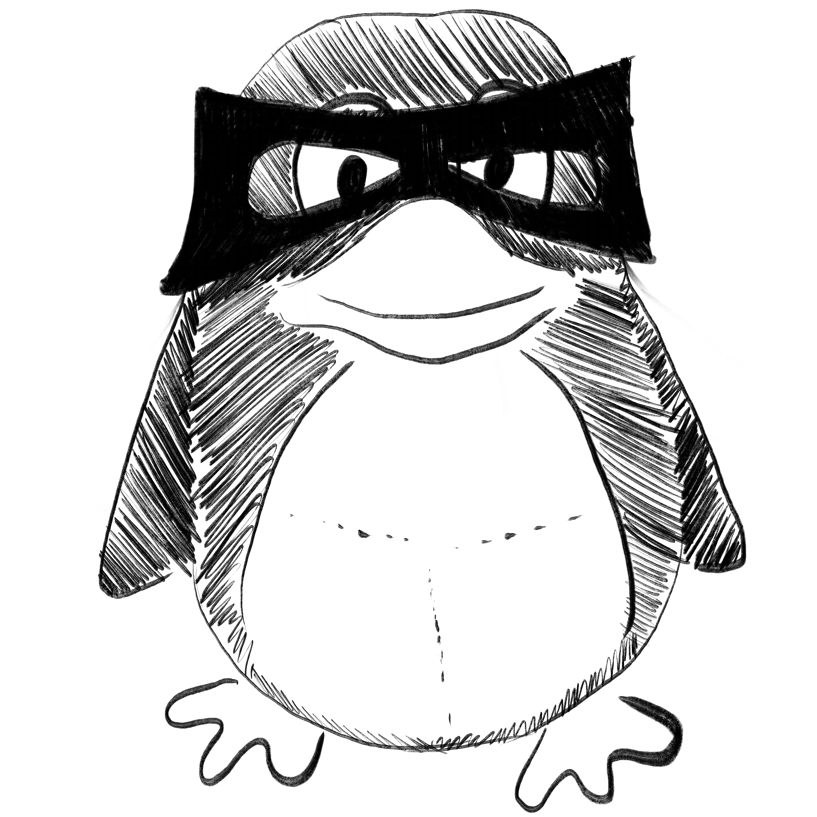
An efficient method for qualitation and quantitation of multi-components of the herbal medicine Qingjin Yiqi Granules.
In Journal of pharmaceutical and biomedical analysis
Yang Xiaohua, Wang Shangqi, Qi Lina, Chen Shujing, Du Kunze, Shang Ye, Guo Jiading, Fang Shiming, Li Jin, Zhang Han, Chang Yanxu
2023-Feb-11
COVID-19, Deep-learning MDF, Qingjin Yiqi Granules, UHPLC-Q-TOF/MS, UHPLC-sMRM
Deep Learning With Chest Radiographs for Making Prognoses in Patients With COVID-19: Retrospective Cohort Study.
In Journal of medical Internet research ; h5-index 88.0
BACKGROUND :
OBJECTIVE :
METHODS :
RESULTS :
CONCLUSIONS :
Lee Hyun Woo, Yang Hyun Jun, Kim Hyungjin, Kim Ue-Hwan, Kim Dong Hyun, Yoon Soon Ho, Ham Soo-Youn, Nam Bo Da, Chae Kum Ju, Lee Dabee, Yoo Jin Young, Bak So Hyeon, Kim Jin Young, Kim Jin Hwan, Kim Ki Beom, Jung Jung Im, Lim Jae-Kwang, Lee Jong Eun, Chung Myung Jin, Lee Young Kyung, Kim Young Seon, Lee Sang Min, Kwon Woocheol, Park Chang Min, Kim Yun-Hyeon, Jeong Yeon Joo, Jin Kwang Nam, Goo Jin Mo
2023-Feb-16
AI model, COVID-19, artificial intelligence, clinical outcome, deep learning, machine learning, medical imaging, prediction model, prognosis, radiography, thoracic
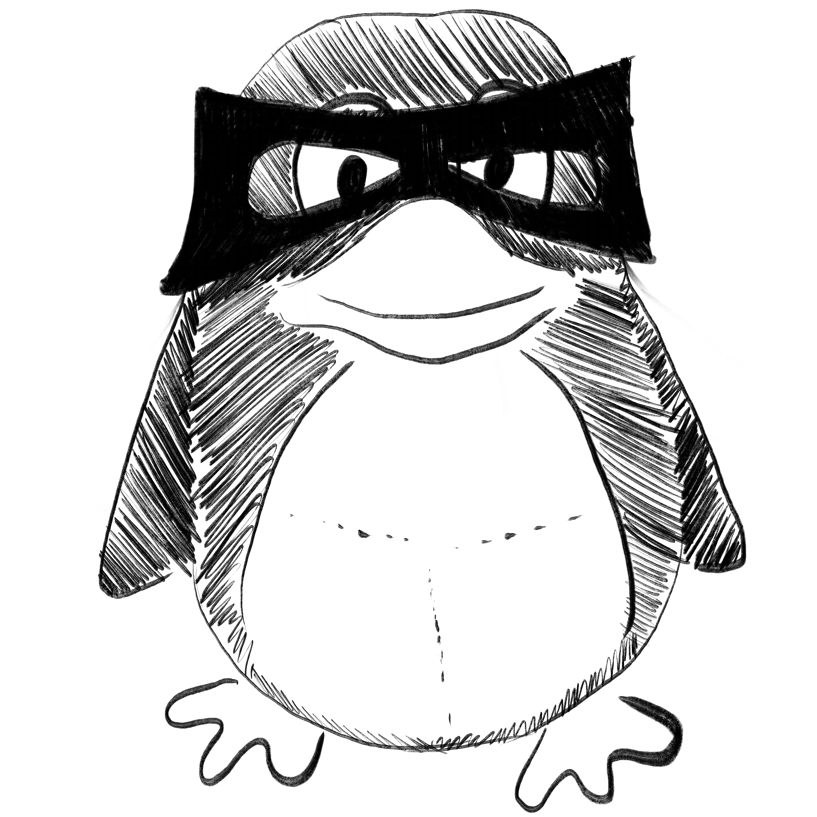
Forecasting the concentration of NO2 using statistical and machine learning methods: A case study in the UAE.
In Heliyon
Al Yammahi Aishah, Aung Zeyar
2023-Feb
ARIMA, Classical statistics, LSTM, Machine learning, NAR, NO2, SARIMA
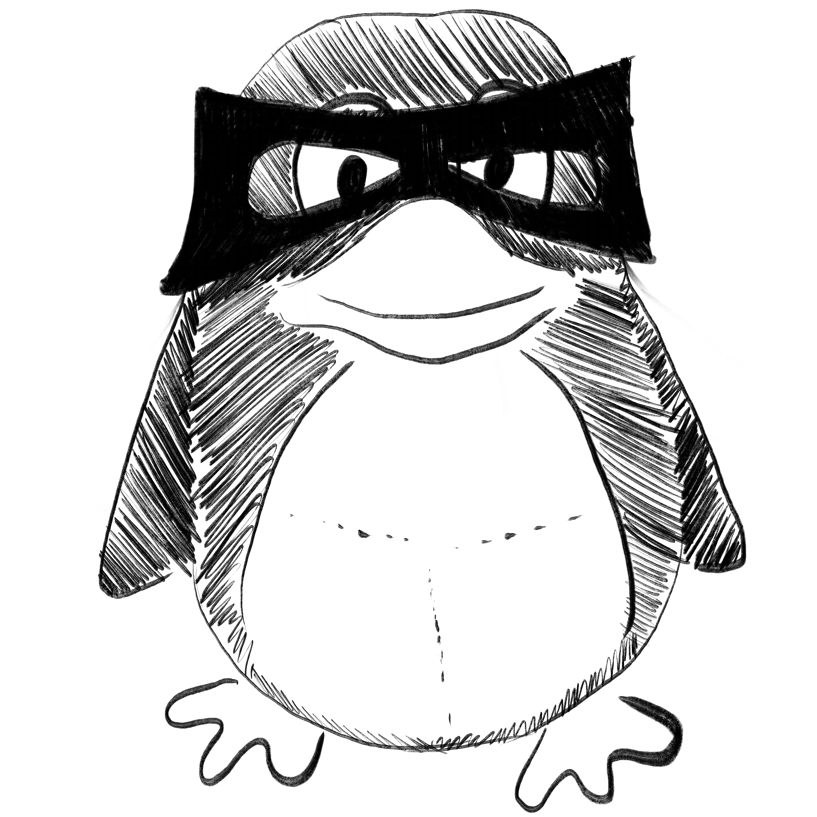
DRaW: prediction of COVID-19 antivirals by deep learning-an objection on using matrix factorization.
In BMC bioinformatics
BACKGROUND :
METHODS :
RESULTS :
CONCLUSIONS :
Hashemi S Morteza, Zabihian Arash, Hooshmand Mohsen, Gharaghani Sajjad
2023-Feb-15
COVID-19, Deep learning, Drug repurposing, Matrix factorization
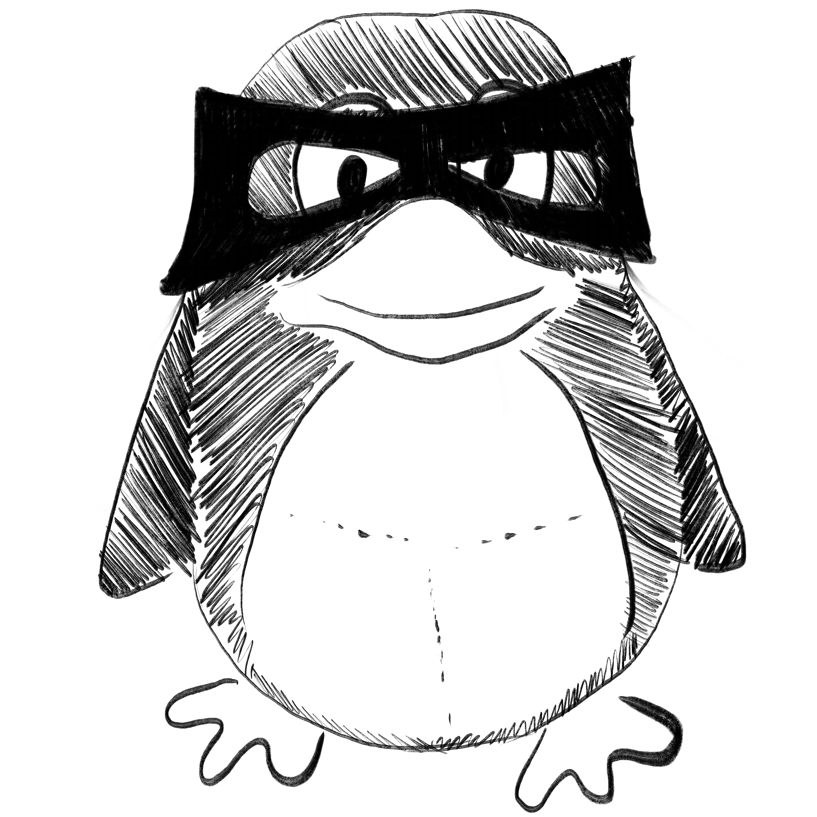
Efficient Classification of SARS-CoV-2 Spike Sequences Using Federated Learning
ArXiv Preprint
Prakash Chourasia, Taslim Murad, Zahra Tayebi, Sarwan Ali, Imdad Ullah Khan, Murray Patterson
2023-02-17
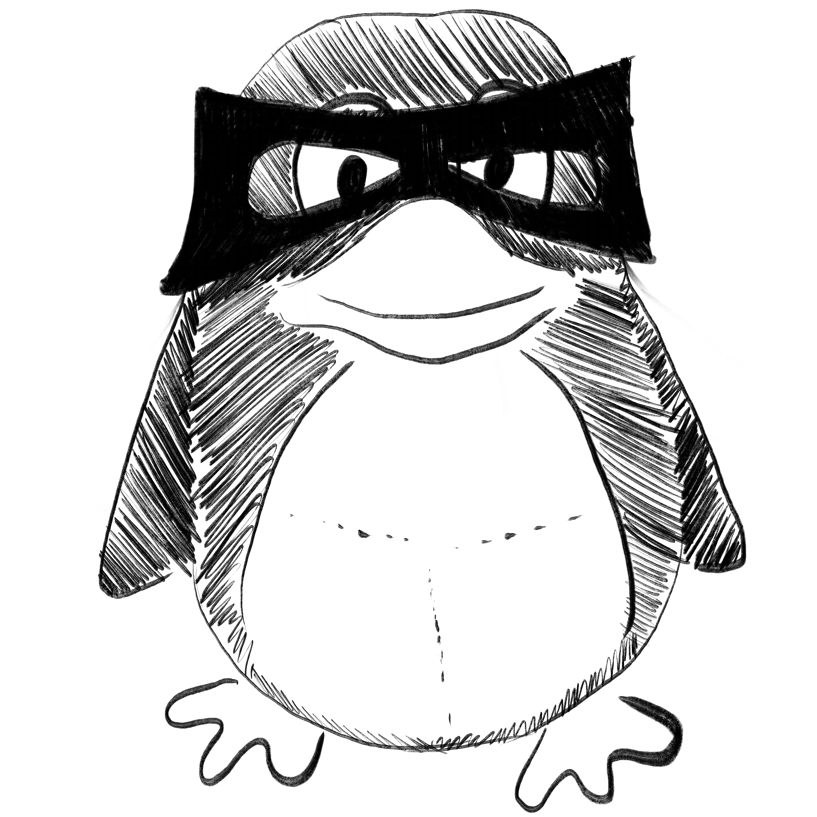
LDANet: Automatic lung parenchyma segmentation from CT images.
In Computers in biology and medicine
Chen Ying, Feng Longfeng, Zheng Cheng, Zhou Taohui, Liu Lan, Liu Pengfei, Chen Yi
2023-Feb-10
CT images, DAGM, LDB, Lung parenchyma segmentation
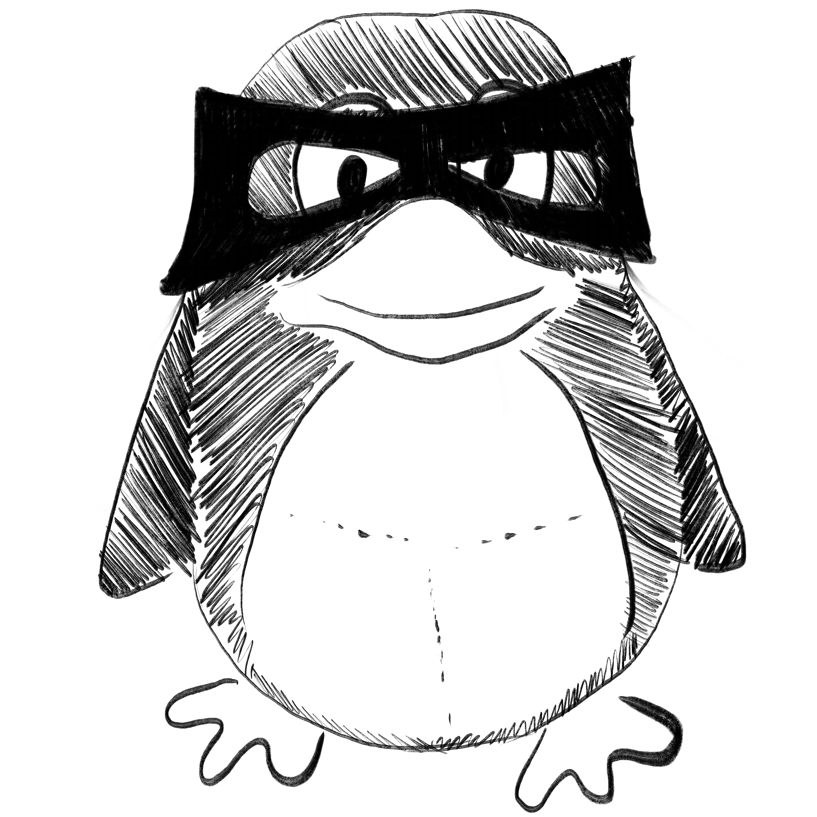
Artificial intelligence assessment of the potential of tocilizumab along with corticosteroids therapy for the management of COVID-19 evoked acute respiratory distress syndrome.
In PloS one ; h5-index 176.0
Segú-Vergés Cristina, Artigas Laura, Coma Mireia, Peck Richard W
2023
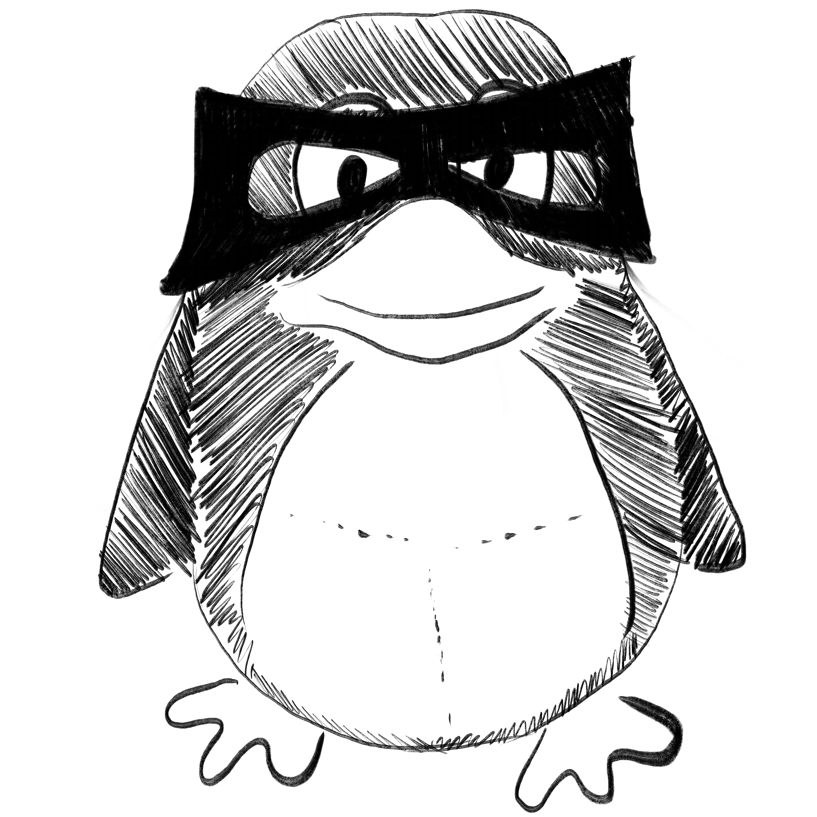
Using machine learning to improve our understanding of COVID-19 infection in children.
In PloS one ; h5-index 176.0
PURPOSE :
METHODS :
RESULTS :
CONCLUSIONS :
Piparia Shraddha, Defante Andrew, Tantisira Kelan, Ryu Julie
2023
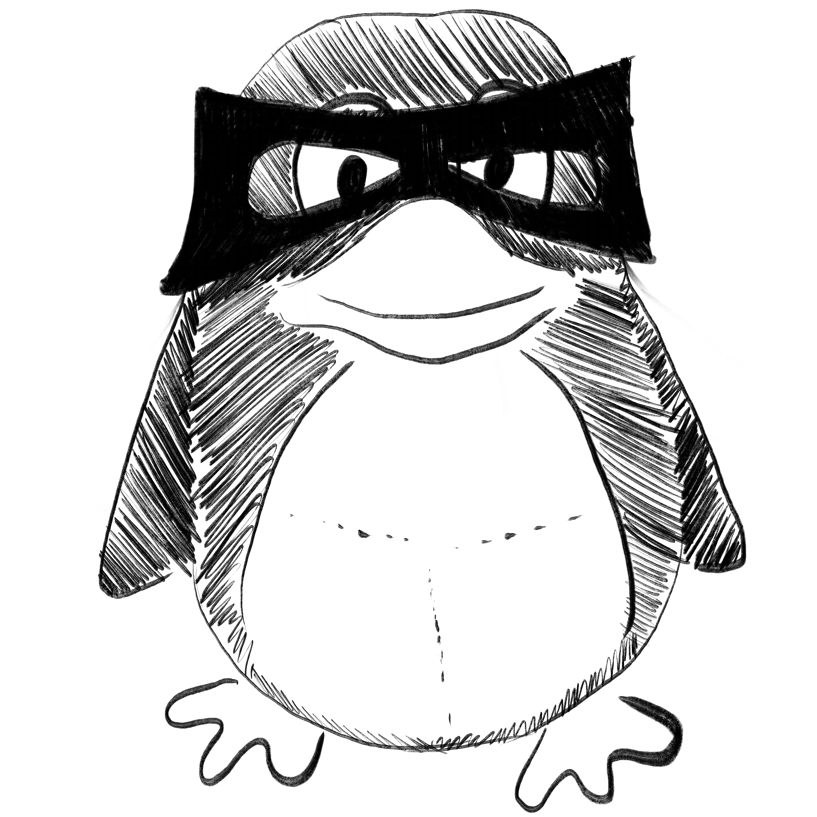
Examining Rural and Urban Sentiment Difference in COVID-19-Related Topics on Twitter: Word Embedding-Based Retrospective Study.
In Journal of medical Internet research ; h5-index 88.0
BACKGROUND :
OBJECTIVE :
METHODS :
RESULTS :
CONCLUSIONS :
Liu Yongtai, Yin Zhijun, Ni Congning, Yan Chao, Wan Zhiyu, Malin Bradley
2023-Feb-15
COVID-19, Twitter, data, epidemic, machine learning, management, model, prevention, rural, sentiment analysis, social media, topic analysis, training, urban, vaccination, word embedding
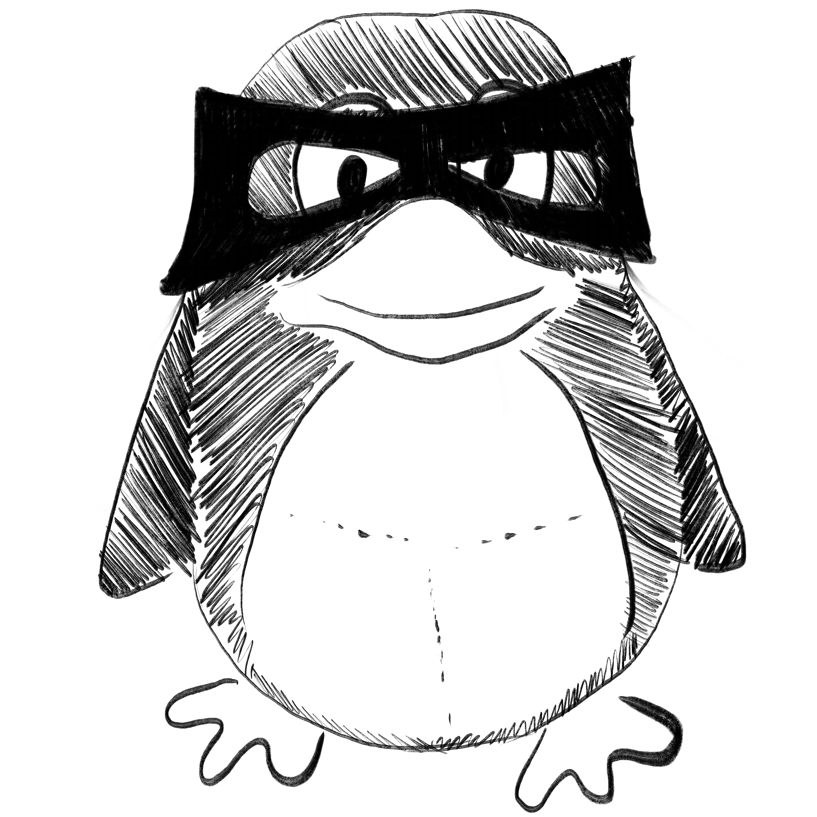
Grayscale Image Statistical Attributes Effectively Distinguish the Severity of Lung Abnormalities in CT Scan Slices of COVID-19 Patients.
In SN computer science
Ghashghaei Sara, Wood David A, Sadatshojaei Erfan, Jalilpoor Mansooreh
2023
COVID-19 lung abnormalities, Computed tomography (CT) scan analysis, Confusion matrices, Grayscale image attributes, Machine and deep learning predictions, Visual and algorithmic classifications
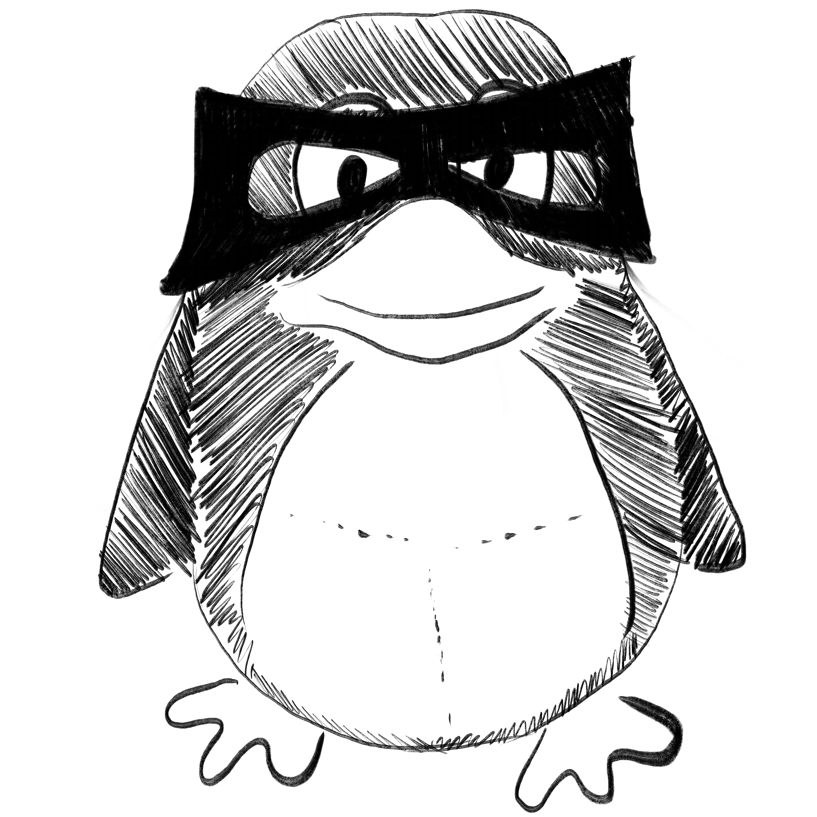
A multimodal facial cues based engagement detection system in e-learning context using deep learning approach.
In Multimedia tools and applications
Gupta Swadha, Kumar Parteek, Tekchandani Rajkumar
2023-Feb-10
Deep learning, Emotion detection, Engagement detection, Eye-blinking, Facial expressions, Head-movement, Online learning, Real-time
A Potential Role of the Spike Protein in Neurodegenerative Diseases: A Narrative Review.
In Cureus
Seneff Stephanie, Kyriakopoulos Anthony M, Nigh Greg, McCullough Peter A
2023-Feb
amyloidosis, cd16+ monocytes, diabetes, exosomes, g quadruplexes, mrna vaccines, neurodegeneration, prion disease, sars-cov-2, spike protein
Scientific novelty beyond the experiment.
In Microbial biotechnology
Hallsworth John E, Udaondo Zulema, Pedrós-Alió Carlos, Höfer Juan, Benison Kathleen C, Lloyd Karen G, Cordero Radamés J B, de Campos Claudia B L, Yakimov Michail M, Amils Ricardo
2023-Feb-14
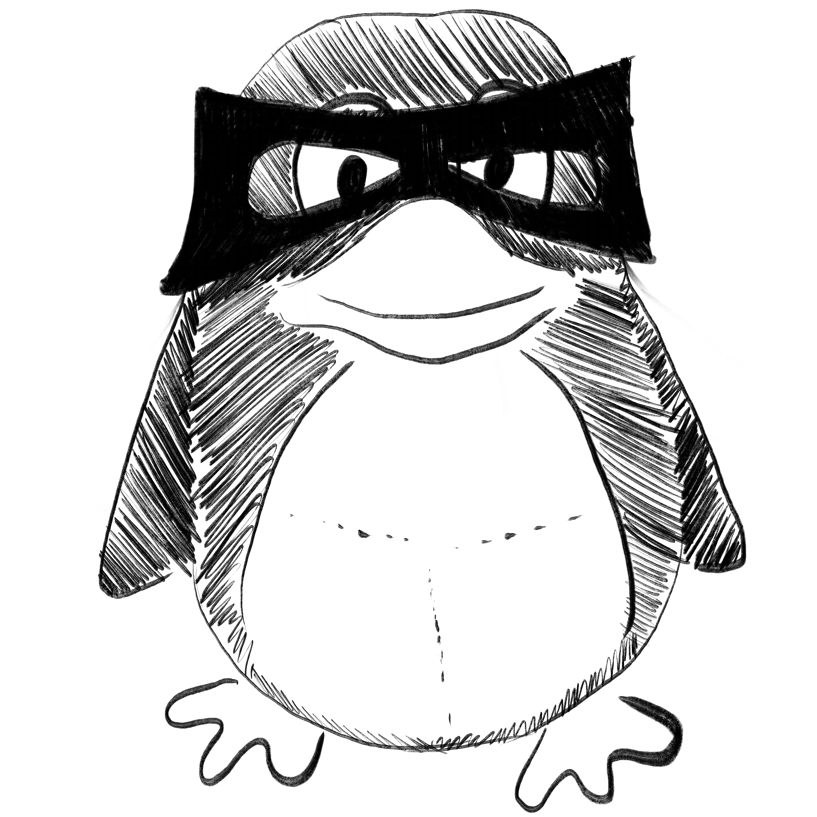
A novel firefly algorithm approach for efficient feature selection with COVID-19 dataset.
In Microprocessors and microsystems
Bacanin Nebojsa, Venkatachalam K, Bezdan Timea, Zivkovic Miodrag, Abouhawwash Mohamed
2023-Apr
COVID-19 dataset, Feature selection, Firefly algorithm, Genetic operators, Quasi-reflection-based learning, Swarm intelligence
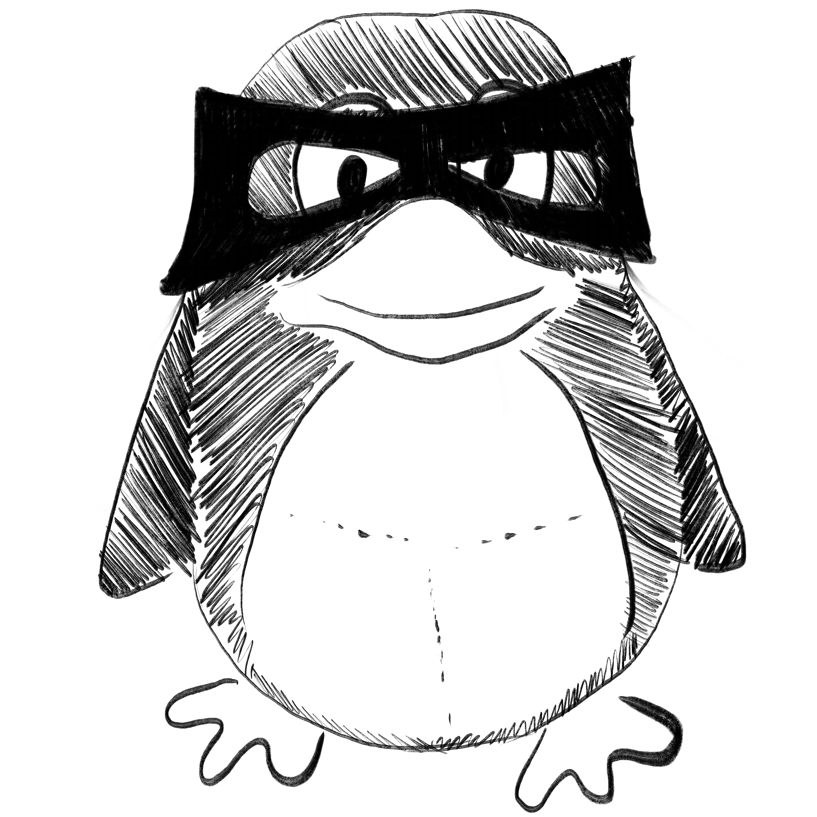
Transcriptomics secondary analysis of severe human infection with SARS-CoV-2 identifies gene expression changes and predicts three transcriptional biomarkers in leukocytes.
In Computational and structural biotechnology journal
Clancy Jeffrey, Hoffmann Curtis S, Pickett Brett E
2023
AUC, Area under the curve, Bioinformatics, Biomarkers, COVID-19, COVID-19, Coronavirus Disease of 2019, DEG, Differentially expressed gene, Data mining, GEO, Gene Expression Omnibus, GO, Gene Ontology, RNA, RNA-sequencing, ROC, Receiver-operator characteristic, SARS-CoV-2, SARS-CoV-2, Severe Acute Respiratory Syndrome Coronavirus 2, Virus
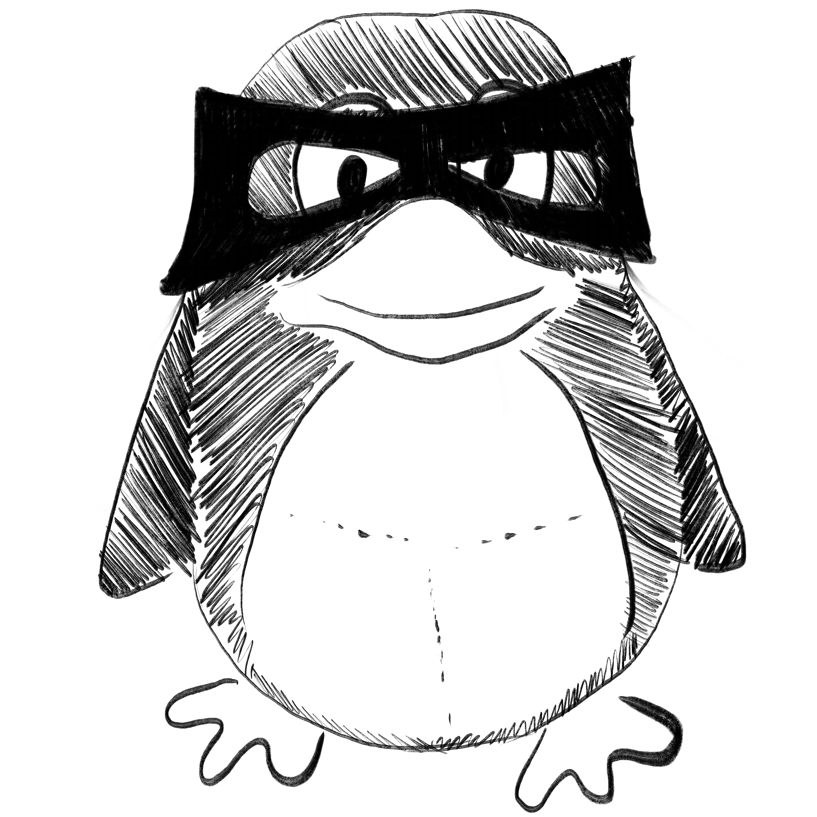
Systematic review of MCDM approach applied to the medical case studies of COVID-19: trends, bibliographic analysis, challenges, motivations, recommendations, and future directions.
In Complex & intelligent systems
Alamoodi A H, Zaidan B B, Albahri O S, Garfan Salem, Ahmaro Ibraheem Y Y, Mohammed R T, Zaidan A A, Ismail Amelia Ritahani, Albahri A S, Momani Fayiz, Al-Samarraay Mohammed S, Jasim Ali Najm
2023-Feb-03
COVID-19, Data privacy, Federated learning, Monoclonal antibodies, Multi-criterion decision making, Treatment
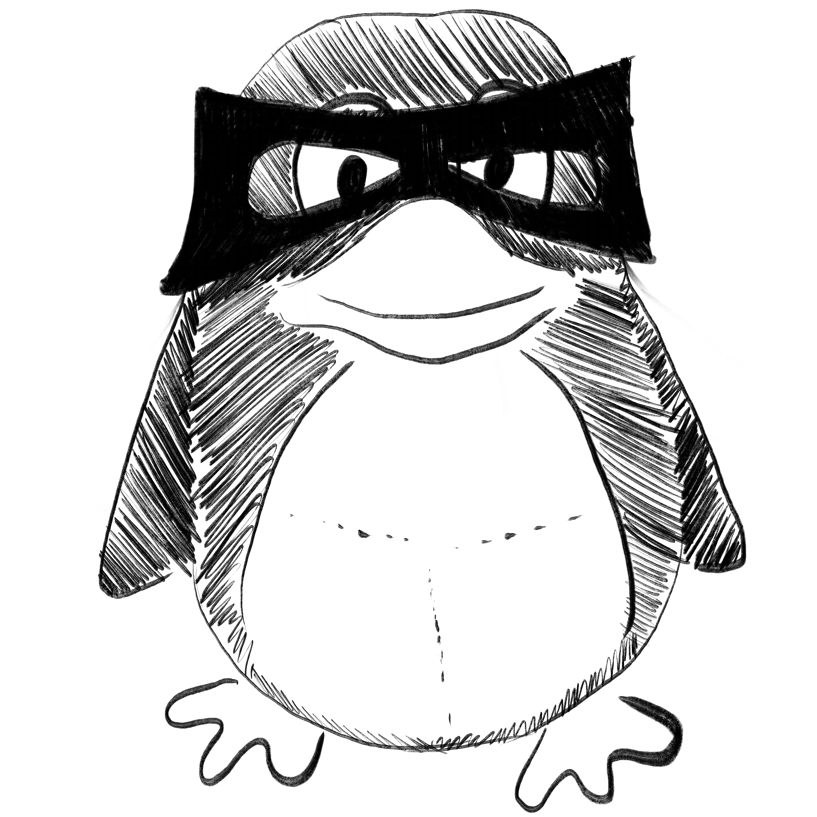
The Effect of Machine Learning Algorithms on the Prediction of Layer-by-Layer Coating Properties.
In ACS omega
Šušteršič Tijana, Gribova Varvara, Nikolic Milica, Lavalle Philippe, Filipovic Nenad, Vrana Nihal Engin
2023-Feb-07
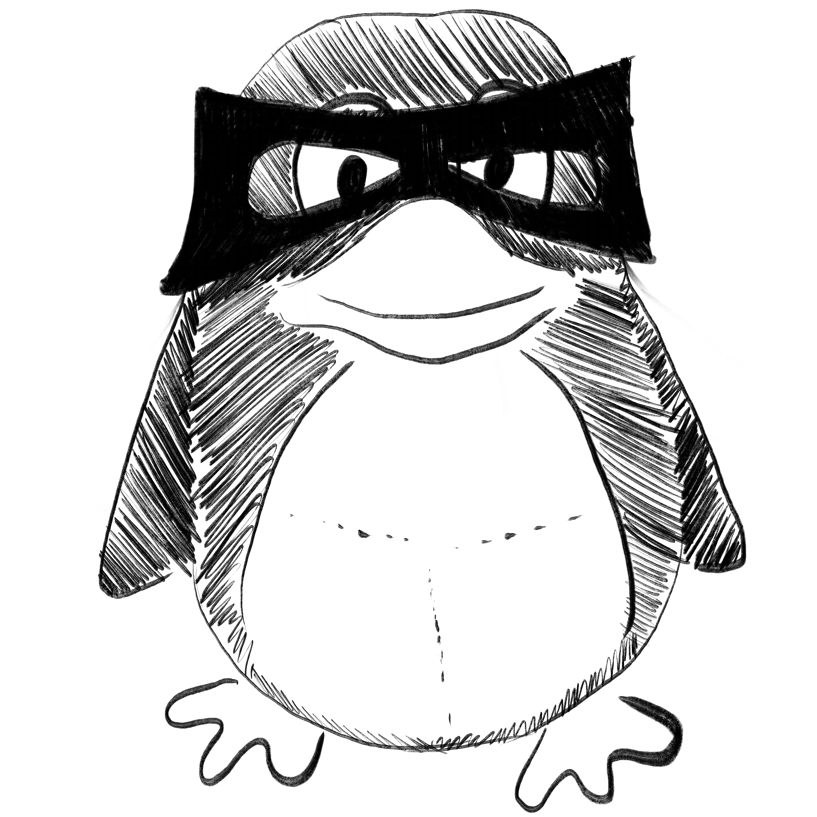
Improved Reptile Search Algorithm by Salp Swarm Algorithm for Medical Image Segmentation.
In Journal of bionic engineering
Abualigah Laith, Habash Mahmoud, Hanandeh Essam Said, Hussein Ahmad MohdAziz, Shinwan Mohammad Al, Zitar Raed Abu, Jia Heming
2023-Feb-07
Bioinspired, Image segmentation, Meta-heuristic algorithm, Multi-level thresholding, Reptile Search Algorithm, Salp Swarm Algorithm
Governing AI during a pandemic crisis: Initiatives at the EU level.
In Technology in society
Fontes Catarina, Corrigan Caitlin, Lütge Christoph
2023-Feb-03
AI Ethics, AI Governance, Artificial intelligence, Covid-19, Robotics
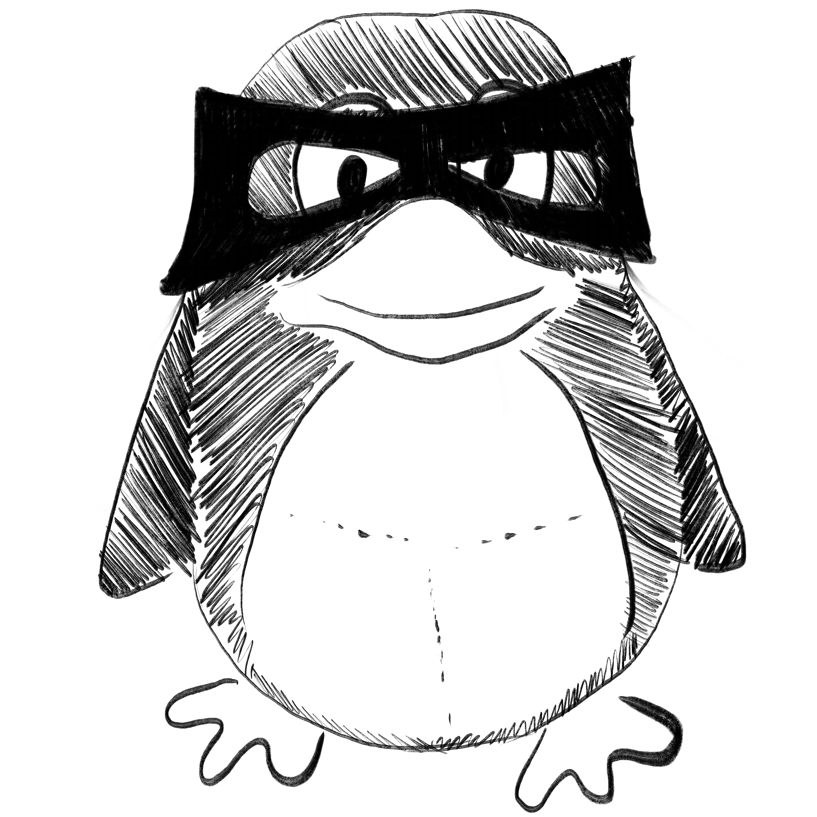
An optimized EBRSA-Bi LSTM model for highly undersampled rapid CT image reconstruction.
In Biomedical signal processing and control
Sarvari A V P, Sridevi K
2023-May
Computed tomography (CT), Deep learning, Image reconstruction, K-space data, Under-sampling
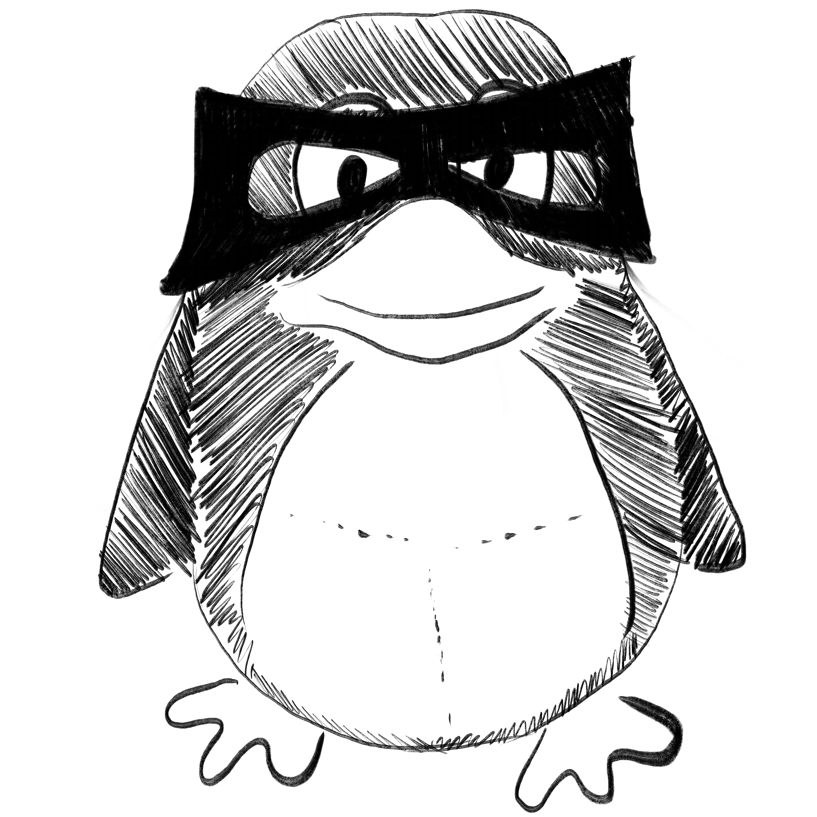
Improved autoregressive integrated moving average model for COVID-19 prediction by using statistical significance and clustering techniques.
In Heliyon
PURPOSE :
METHODS :
RESULTS :
CONCLUSIONS :
Ilu Saratu Yusuf, Prasad Rajesh
2023-Feb
ARIMA, And clustering, Coronavirus, Feature selection, Machine learning, Prediction
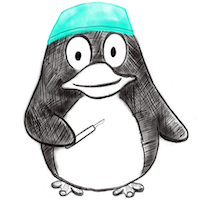
Elevated IFNA1 and suppressed IL12p40 associated with persistent hyperinflammation in COVID-19 pneumonia.
In Frontiers in immunology ; h5-index 100.0
INTRODUCTION :
METHODS :
RESULTS :
DISCUSSION :
Jeon Kyeongseok, Kim Yuri, Kang Shin Kwang, Park Uni, Kim Jayoun, Park Nanhee, Koh Jaemoon, Shim Man-Shik, Kim Minsoo, Rhee Youn Ju, Jeong Hyeongseok, Lee Siyoung, Park Donghyun, Lim Jinyoung, Kim Hyunsu, Ha Na-Young, Jo Hye-Yeong, Kim Sang Cheol, Lee Ju-Hee, Shon Jiwon, Kim Hoon, Jeon Yoon Kyung, Choi Youn-Soo, Kim Hye Young, Lee Won-Woo, Choi Murim, Park Hyun-Young, Park Woong-Yang, Kim Yeon-Sook, Cho Nam-Hyuk
2023
COVID-19, IFNa, IL-12p40, SARS-CoV-2, inflammation, pneumonia
Machine-learning prediction of BMI change among doctors and nurses in North China during the COVID-19 pandemic.
In Frontiers in nutrition
OBJECTIVE :
METHODS :
RESULTS :
CONCLUSION :
Wang Qihe, Chu Haiyun, Qu Pengfeng, Fang Haiqin, Liang Dong, Liu Sana, Li Jinliang, Liu Aidong
2023
BMI change, COVID-19 pandemic, China, doctors and nurses, machine learning
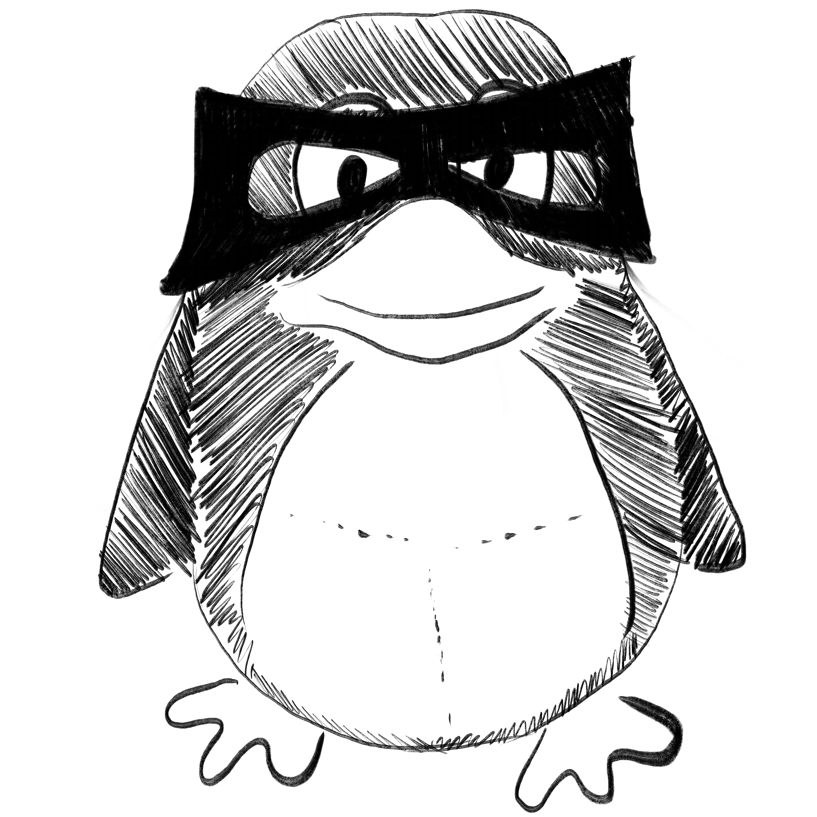
A Survey on harnessing the Applications of Mobile Computing in Healthcare during the COVID-19 Pandemic: Challenges and Solutions.
In Computer networks
Ali Yasir, Khan Habib Ullah
2023-Apr
COVID-19, Coronavirus, Healthcare, Mobile computing, Pervasive computing, SARS-COV-2
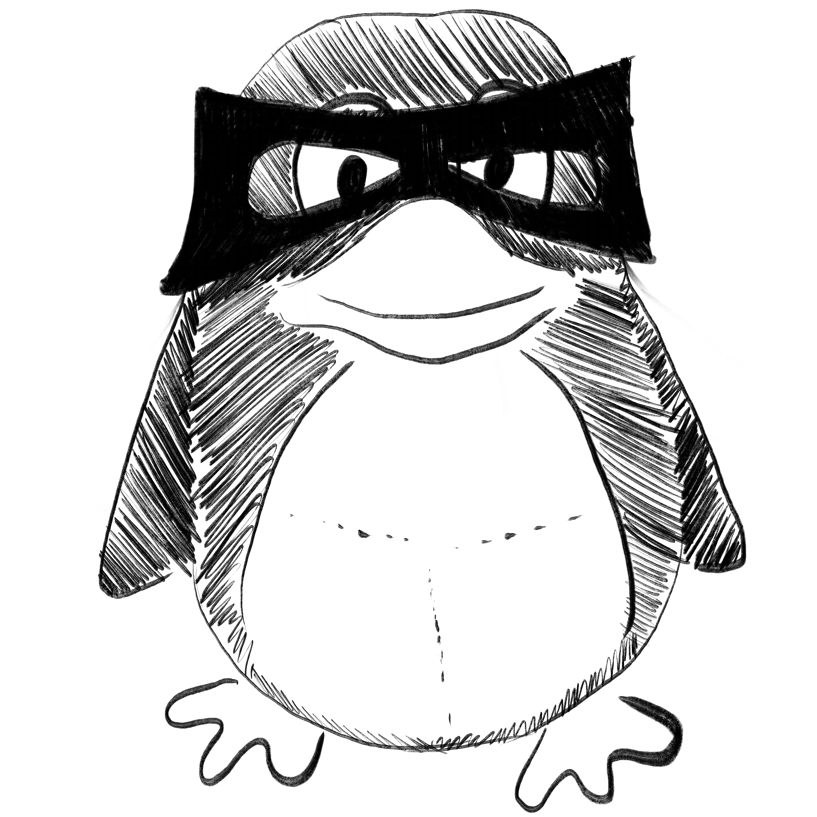
Predicting the antigenic evolution of SARS-COV-2 with deep learning
bioRxiv Preprint
Han, W.; Chen, N.; Xu, X.; Sahil, A.; Zhou, J.; Li, Z.; Zhong, H.; Gao, E.; Zhang, R.; Wang, Y.; Sun, S.; Gao, X.; Cheung, P. P.-H.
2023-02-14
Home alone: A population neuroscience investigation of brain morphology substrates.
In NeuroImage ; h5-index 117.0
Noonan MaryAnn, Zajner Chris, Bzdok Danilo
2023-Feb-11
Bayesian hierarchical modelling, Population neuroscience, amygdala nuclei groups, hippocampus subfields, social brain
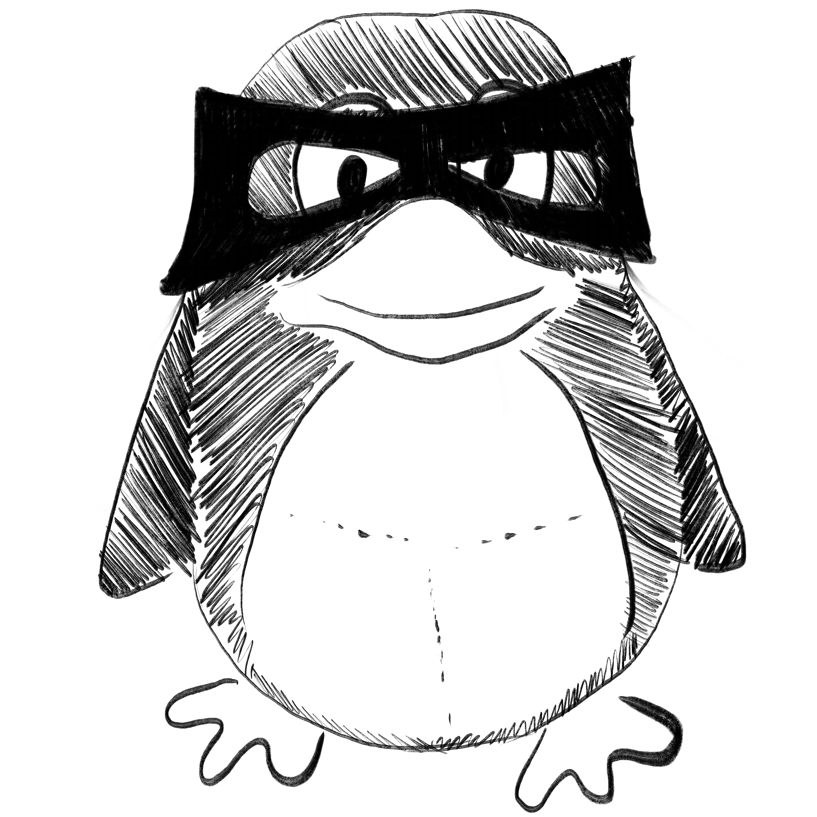
Classifying COVID-19 patients from Chest X-ray Images Using Hybrid Machine Learning Techniques: Development and Evaluation.
In JMIR formative research
BACKGROUND :
OBJECTIVE :
METHODS :
RESULTS :
CONCLUSIONS :
Phumkuea Thanakorn, Wongsirichot Thakerng, Damkliang Kasikrit, Navasakulpong Asma
2023-Feb-13
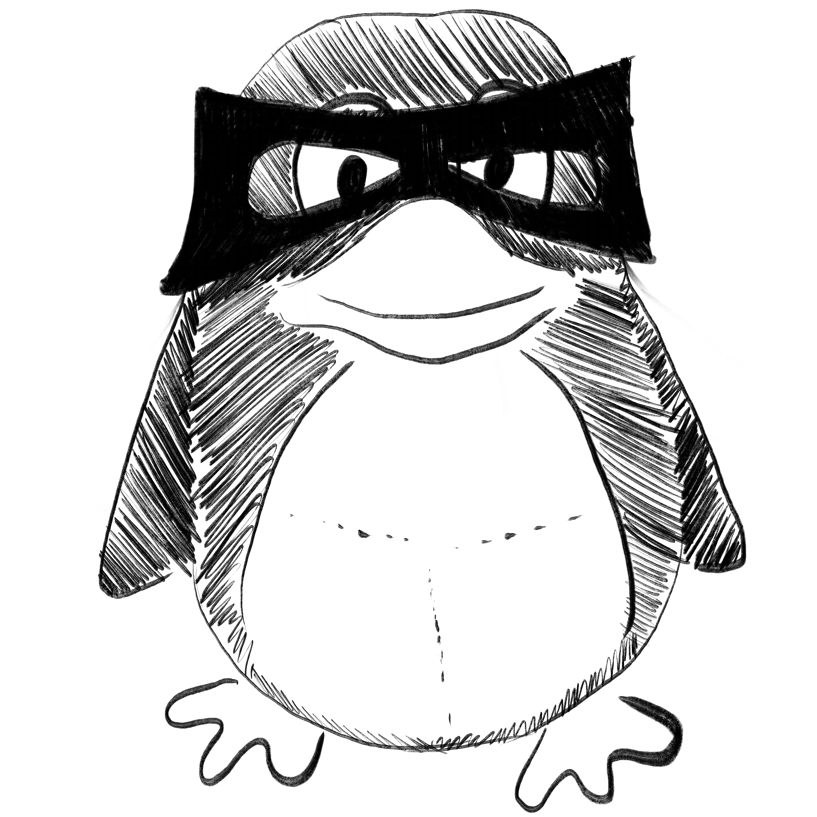
Hybrid model for early identification post-Covid-19 sequelae.
In Journal of ambient intelligence and humanized computing
de Andrade Evandro Carvalho, Pinheiro Luana Ibiapina C C, Pinheiro Plácido Rogério, Nunes Luciano Comin, Pinheiro Mirian Calíope Dantas, Pereira Maria Lúcia Duarte, de Abreu Wilson Correia, Filho Raimir Holanda, Simão Filho Marum, Pinheiro Pedro Gabriel C D, Nunes Rafael Espíndola Comin
2023-Feb-06
Covid-19, Decision support systems, Hybrid model, Machine learning, Medical diagnostic optimization, Verbal decision analysis
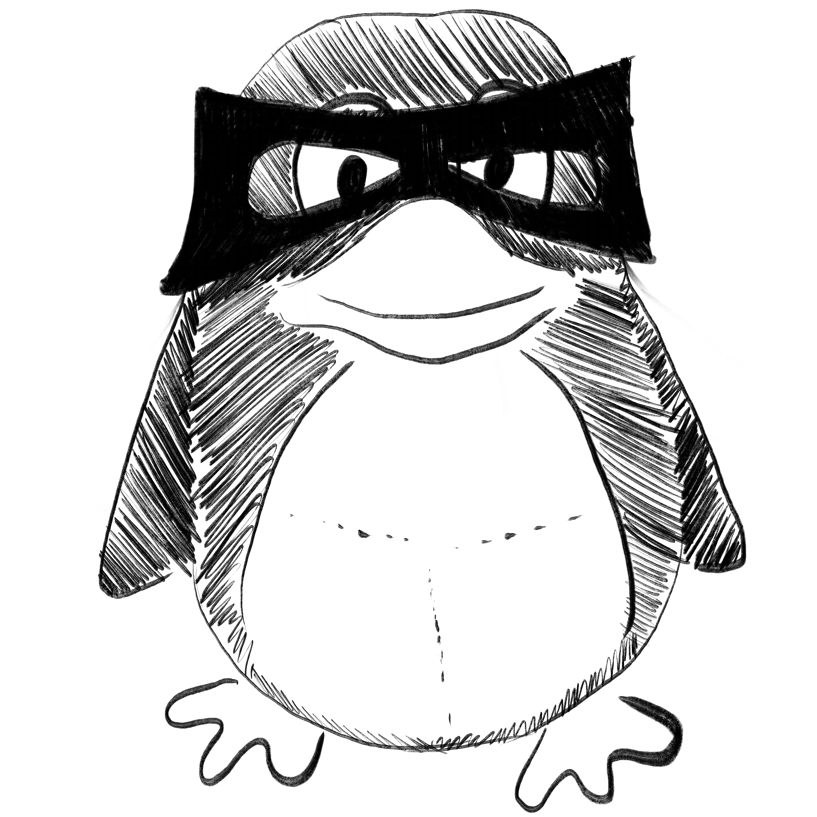
Adoption of AI in response to COVID-19-a configurational perspective.
In Personal and ubiquitous computing
Mi Lili, Liu Wei, Yuan Yu-Hsi, Shao Xuefeng, Zhong Yifan
2023-Feb-07
Artificial intelligence, Fuzzy-set qualitative comparative analysis, Strategic responses to COVID-19, Technology–organization–environment framework
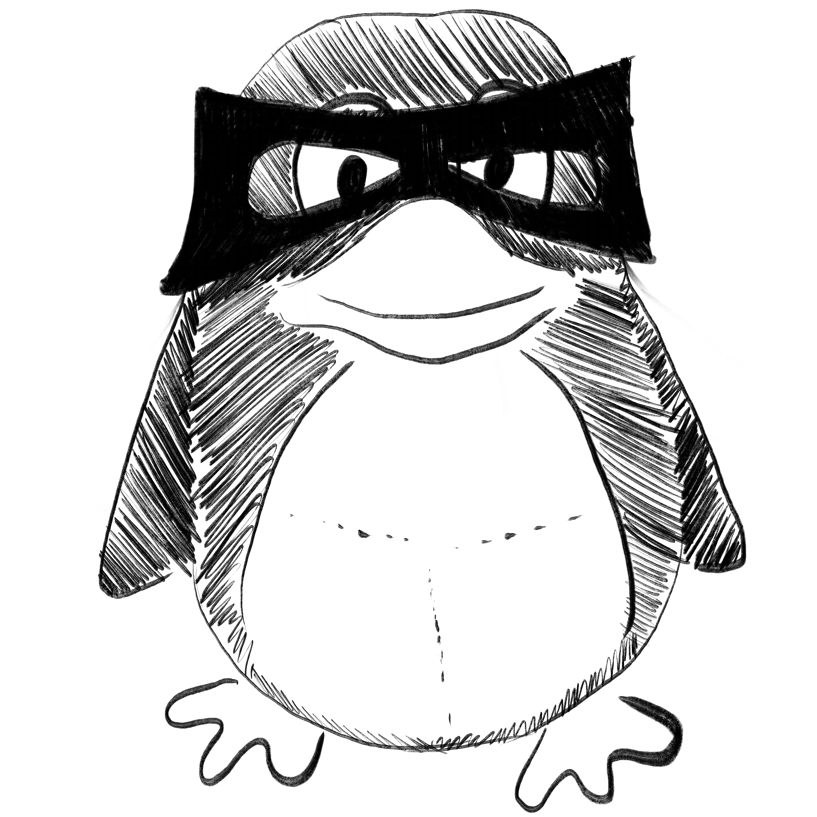
Single-cell multi-omic topic embedding reveals cell-type-specific and COVID-19 severity-related immune signatures.
In bioRxiv : the preprint server for biology
Zhou Manqi, Zhang Hao, Bai Zilong, Mann-Krzisnik Dylan, Wang Fei, Li Yue
2023-Jan-31
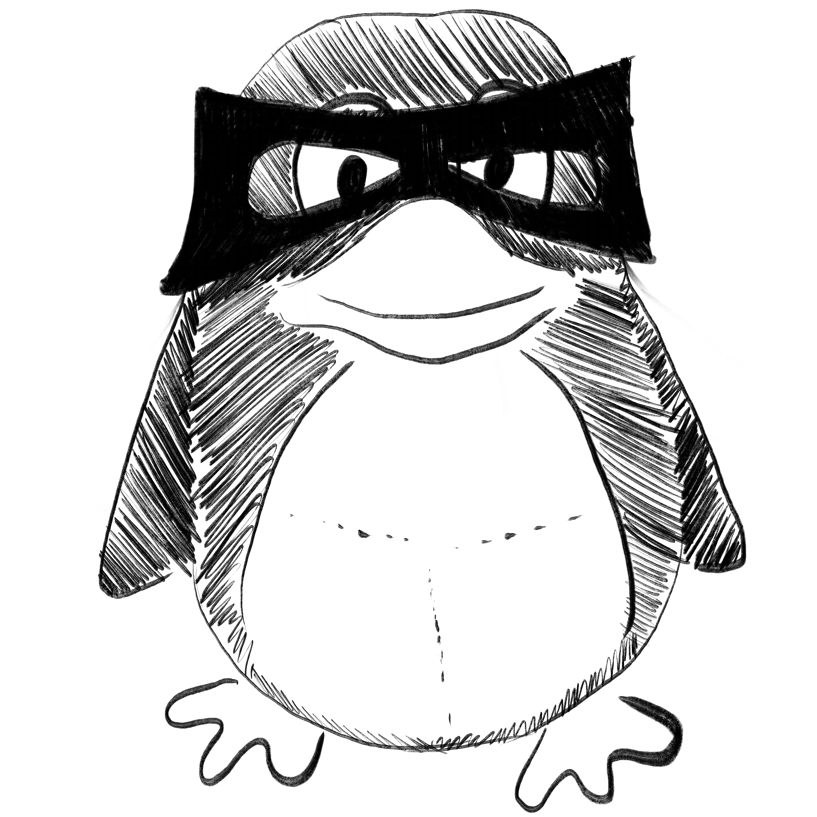
Evaluating deep learning predictions for COVID-19 from X-ray images using leave-one-out predictive densities.
In Neural computing & applications
Hernández Sergio, López-Córtes Xaviera
2023-Feb-06
Bayesian learning, COVID X-ray, Markov chain Monte Carlo
Characterizing Human Collective Behaviors During COVID-19 - Hong Kong SAR, China, 2020.
In China CDC weekly
WHAT IS ALREADY KNOWN ABOUT THIS TOPIC? :
WHAT IS ADDED BY THIS REPORT? :
WHAT ARE THE IMPLICATIONS FOR PUBLIC HEALTH PRACTICE? :
Du Zhanwei, Zhang Xiao, Wang Lin, Yao Sidan, Bai Yuan, Tan Qi, Xu Xiaoke, Pei Sen, Xiao Jingyi, Tsang Tim K, Liao Qiuyan, Lau Eric H Y, Wu Peng, Gao Chao, Cowling Benjamin J
2023-Jan-27
COVID-19, Collective Behavior, Hong Kong
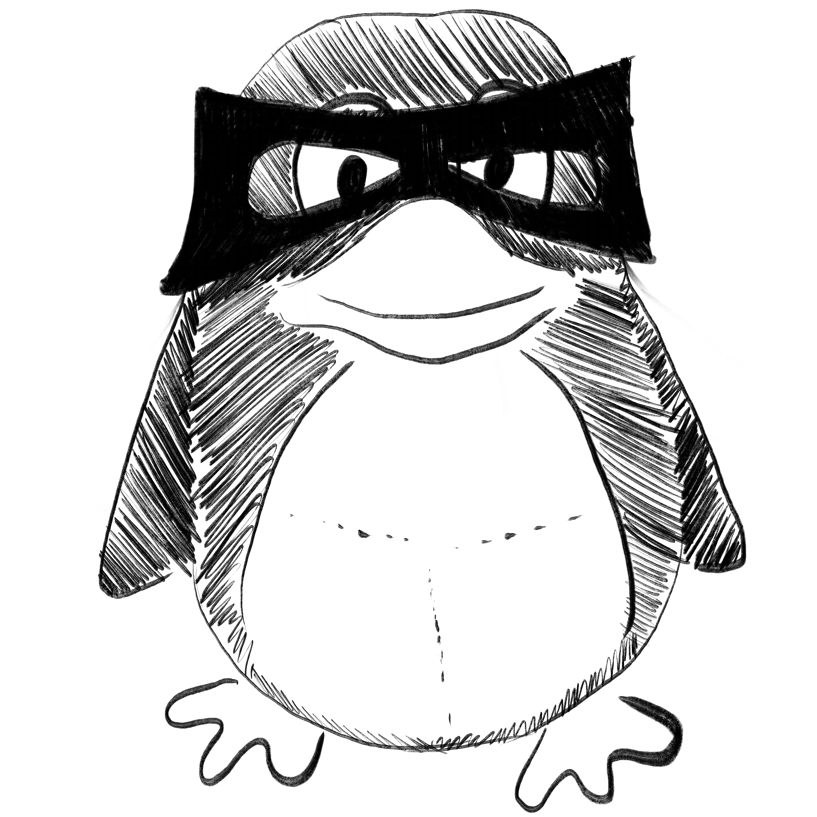
Feature selection of pre-trained shallow CNN using the QLESCA optimizer: COVID-19 detection as a case study.
In Applied intelligence (Dordrecht, Netherlands)
Hamad Qusay Shihab, Samma Hussein, Suandi Shahrel Azmin
2023-Feb-06
Features extraction, Features selection, Q-learning embedded sine cosine algorithm (QLESCA), SVM, Shallow convolutional neural networks, Swarm intelligence
Generalizable machine learning approach for COVID-19 mortality risk prediction using on-admission clinical and laboratory features.
In Scientific reports ; h5-index 158.0
Barough Siavash Shirzadeh, Safavi-Naini Seyed Amir Ahmad, Siavoshi Fatemeh, Tamimi Atena, Ilkhani Saba, Akbari Setareh, Ezzati Sadaf, Hatamabadi Hamidreza, Pourhoseingholi Mohamad Amin
2023-Feb-10
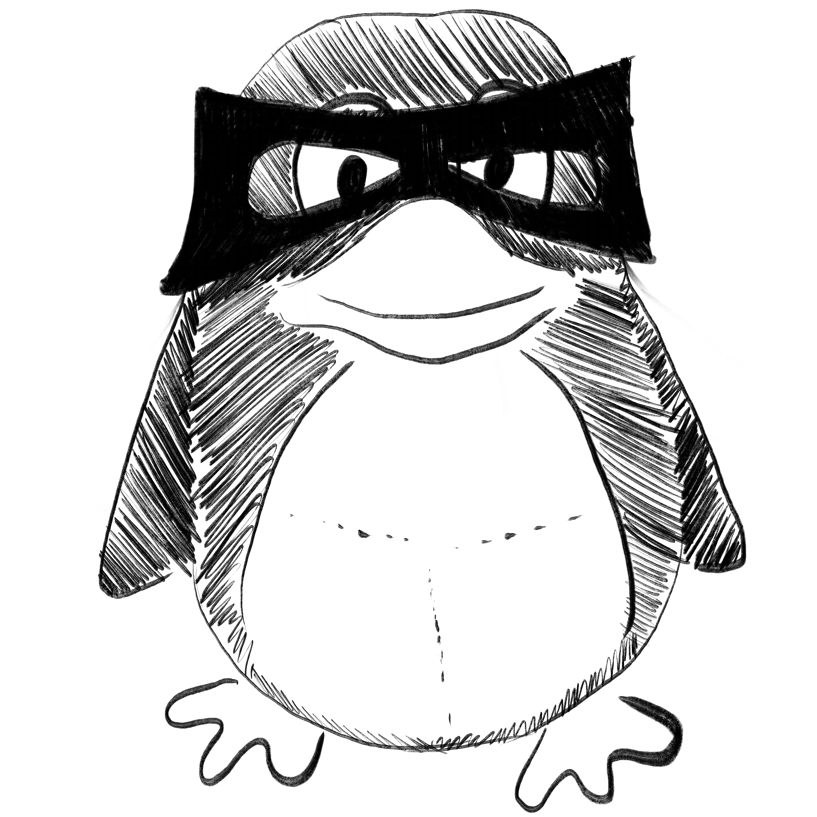
A year of pandemic: Levels, changes and validity of well-being data from Twitter. Evidence from ten countries.
In PloS one ; h5-index 176.0
Sarracino Francesco, Greyling Talita, O’Connor Kelsey, Peroni Chiara, Rossouw Stephanié
2023
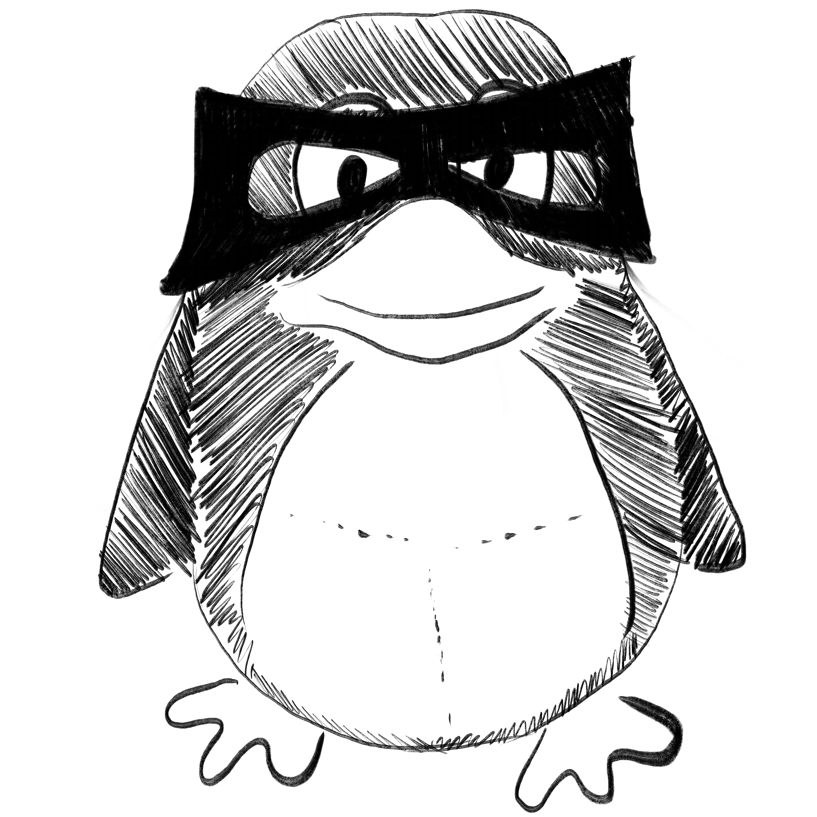
Trajectory tracking of changes digital divide prediction factors in the elderly through machine learning.
In PloS one ; h5-index 176.0
RESEARCH MOTIVATION :
RESEARCH OBJECTIVE :
METHODS AND MATERIALS :
RESULTS :
DISCUSSION :
CONCLUSION :
Park Jung Ryeol, Feng Yituo
2023
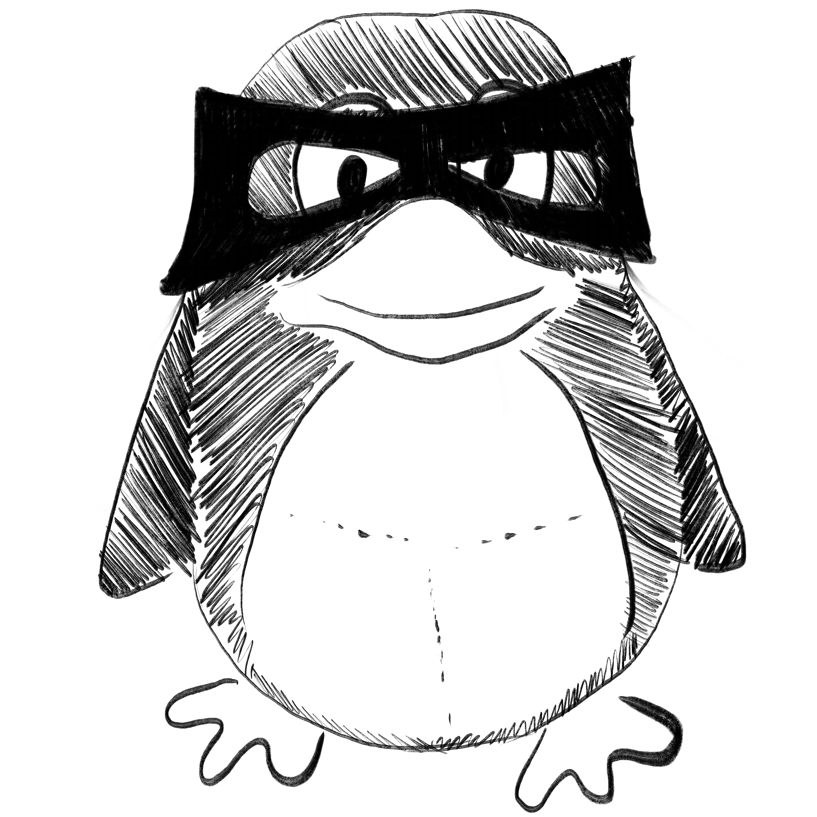
Blood transcriptome and machine learning identified the crosstalk between COVID-19 and fibromyalgia: a preliminary study.
In Clinical and experimental rheumatology ; h5-index 43.0
OBJECTIVES :
METHODS :
RESULTS :
CONCLUSIONS :
Zhang Zhao, Zhu Zhijie, Liu Dong, Mi Zhenz, Tao Huiren, Fan Hongbin
2023-Feb-08
Predicting the distribution of COVID-19 through CGAN-Taking Macau as an example.
In Frontiers in big data
Zheng Liang, Chen Yile, Jiang Shan, Song Junxin, Zheng Jianyi
2023
CGAN, epidemic hotspots, machine learning, risk assessment, urban public health
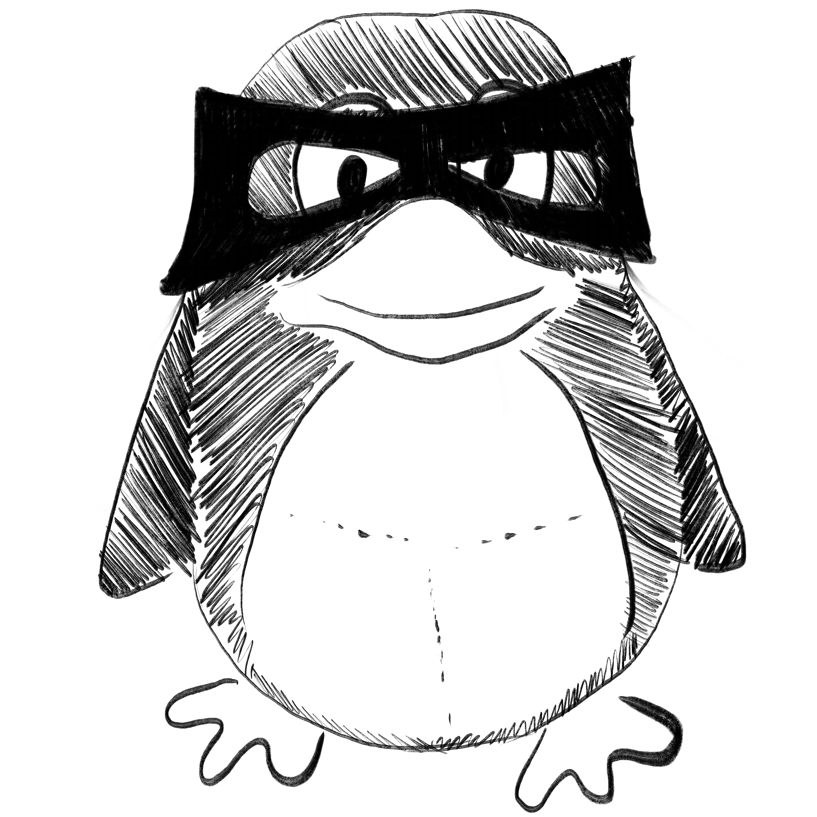
Fireworks explosion boosted Harris Hawks optimization for numerical optimization: Case of classifying the severity of COVID-19.
In Frontiers in neuroinformatics
Wang Mingjing, Chen Long, Heidari Ali Asghar, Chen Huiling
2022
CEC2014 benchmark functions, COVID-19, Harris Hawks optimization, fireworks algorithm, numerical optimization
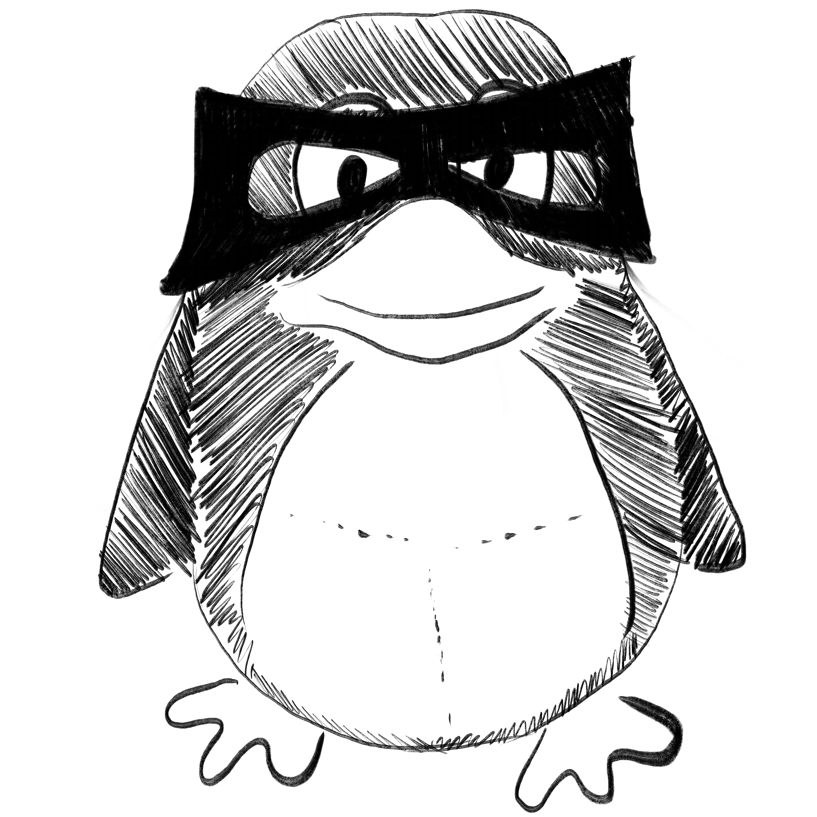
Prediction models for the impact of the COVID-19 pandemic on research activities of Japanese nursing researchers using deep learning.
In Japan journal of nursing science : JJNS
AIM :
METHODS :
RESULTS :
CONCLUSIONS :
Lee Kumsun, Takahashi Fusako, Kawasaki Yuki, Yoshinaga Naoki, Sakai Hiroko
2023-Feb-09
COVID-19, Japan, deep learning, nursing research, university
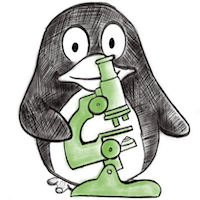
A machine learning approach identifies distinct early-symptom cluster phenotypes which correlate with hospitalization, failure to return to activities, and prolonged COVID-19 symptoms.
In PloS one ; h5-index 176.0
BACKGROUND :
METHODS :
RESULTS :
CONCLUSIONS :
Epsi Nusrat J, Powers John H, Lindholm David A, Mende Katrin, Malloy Allison, Ganesan Anuradha, Huprikar Nikhil, Lalani Tahaniyat, Smith Alfred, Mody Rupal M, Jones Milissa U, Bazan Samantha E, Colombo Rhonda E, Colombo Christopher J, Ewers Evan C, Larson Derek T, Berjohn Catherine M, Maldonado Carlos J, Blair Paul W, Chenoweth Josh, Saunders David L, Livezey Jeffrey, Maves Ryan C, Sanchez Edwards Margaret, Rozman Julia S, Simons Mark P, Tribble David R, Agan Brian K, Burgess Timothy H, Pollett Simon D
2023
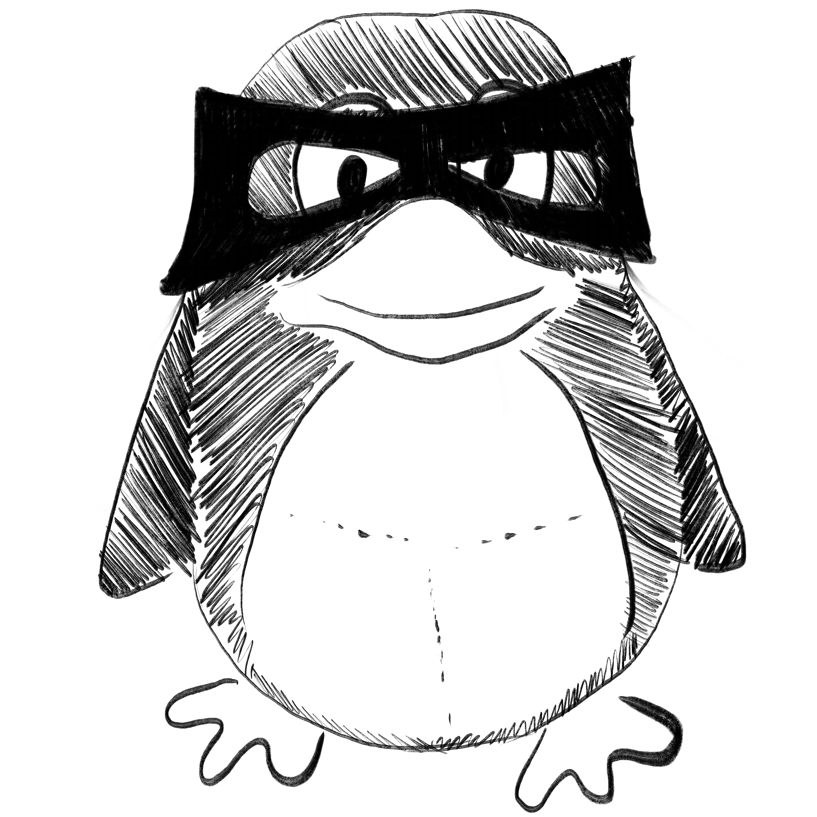
Association of Recent SARS-CoV-2 Infection With New-Onset Alcohol Use Disorder, January 2020 Through January 2022.
In JAMA network open
IMPORTANCE :
OBJECTIVE :
DESIGN, SETTING, AND PARTICIPANTS :
EXPOSURES :
MAIN OUTCOMES AND MEASURES :
RESULTS :
CONCLUSIONS AND RELEVANCE :
Olaker Veronica R, Kendall Ellen K, Wang Christina X, Parran Theodore V, Terebuh Pauline, Kaelber David C, Xu Rong, Davis Pamela B
2023-Feb-01
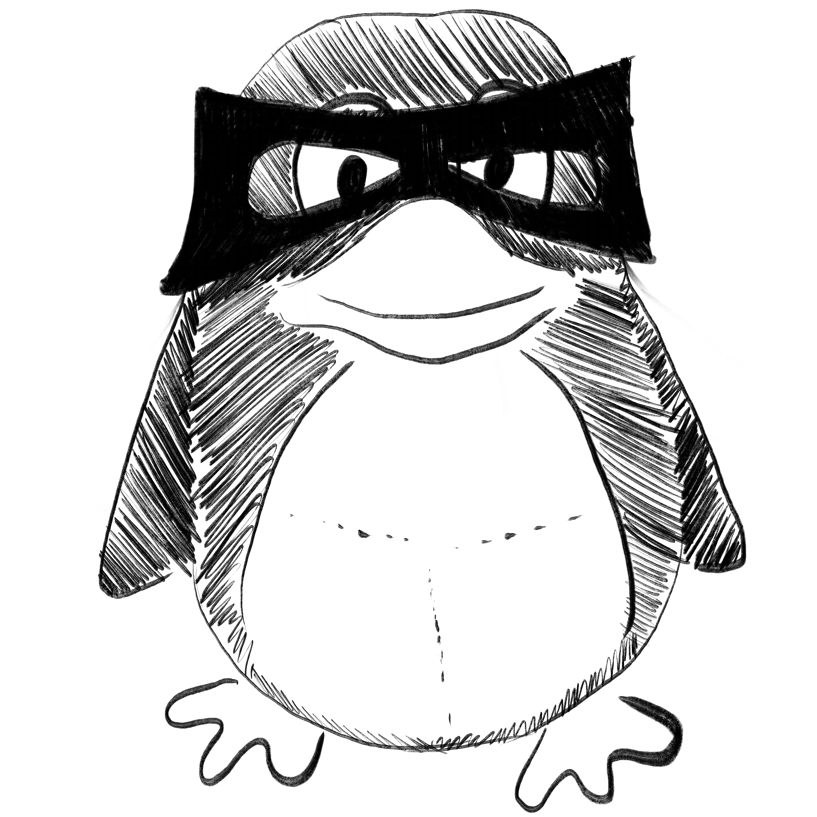
Machine learning-augmented surface-enhanced spectroscopy toward next-generation molecular diagnostics.
In Nanoscale advances
Zhou Hong, Xu Liangge, Ren Zhihao, Zhu Jiaqi, Lee Chengkuo
2023-Jan-31
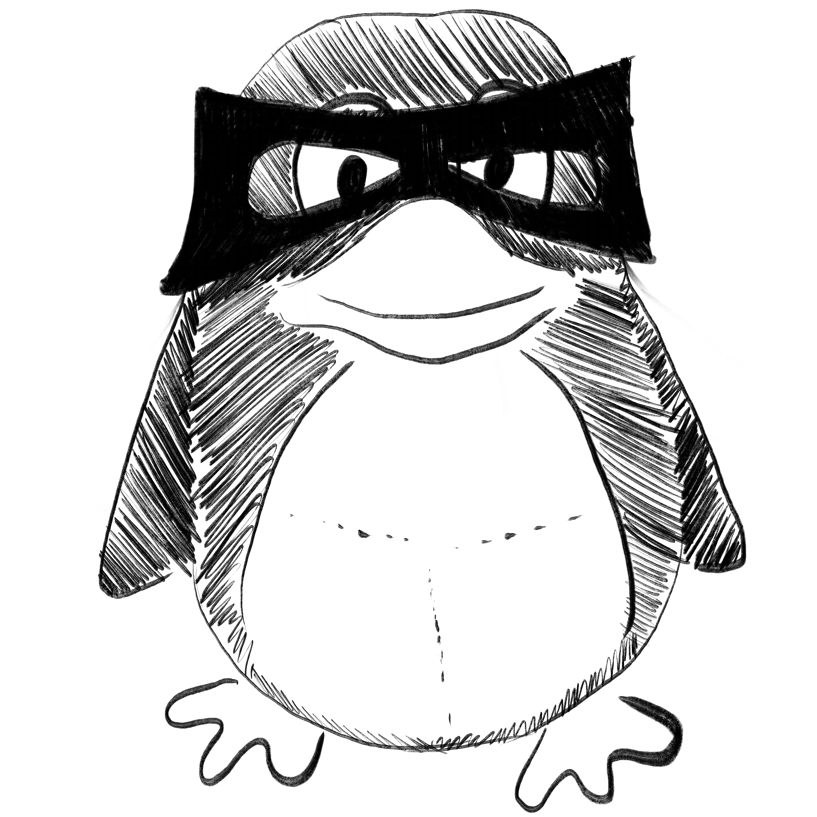
Learning virus genotype-fitness landscape in embedding space
bioRxiv Preprint
Liu, Y.; Luo, Y.; Lu, X.; Gao, H.; He, R.; Zhang, X.; Zhang, X.; Li, Y.
2023-02-10
Early detection of SARS-CoV-2 variants through dynamic co-mutation network surveillance.
In Frontiers in public health
BACKGROUND :
METHODS :
RESULTS :
CONCLUSION :
Huang Qiang, Qiu Huining, Bible Paul W, Huang Yong, Zheng Fangfang, Gu Jing, Sun Jian, Hao Yuantao, Liu Yu
2023
SARS-CoV-2, co-mutation, community detection, network, surveillance
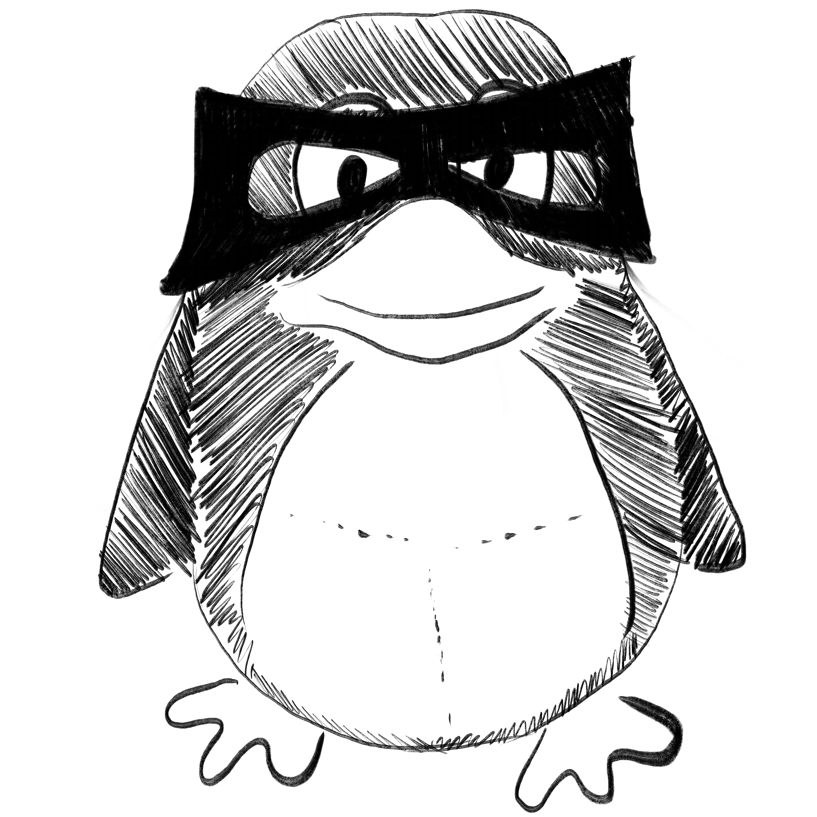
Deep learning approaches to viral phylogeography are fast and as robust as likelihood methods to model misspecification
bioRxiv Preprint
Thompson, A.; Liebeskind, B.; Scully, E. J.; Landis, M.
2023-02-10
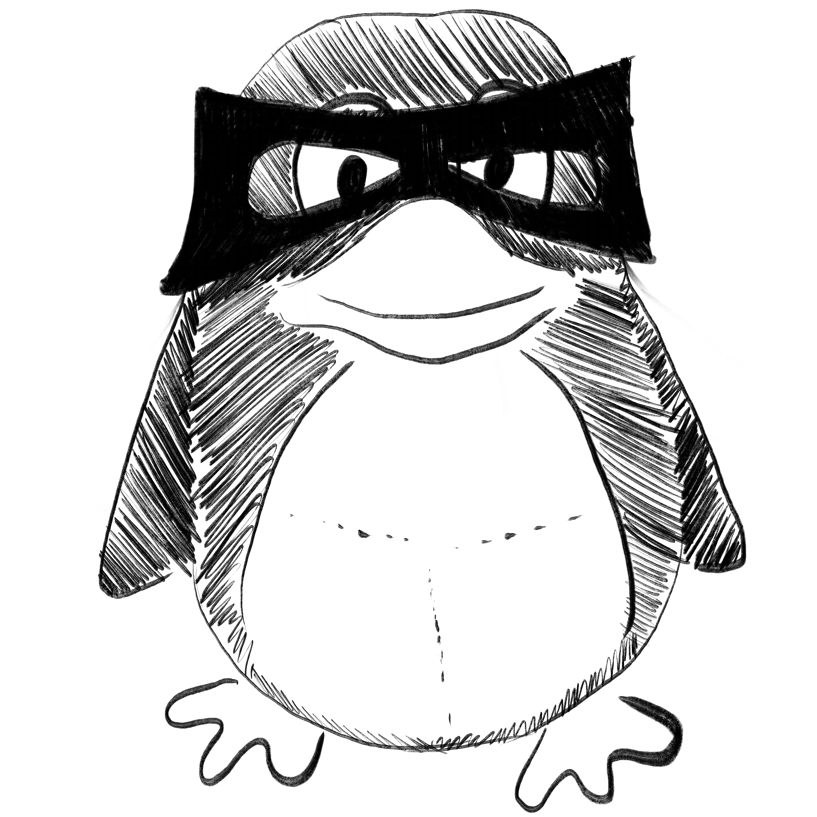
A data-driven approach to the "Everesting" cycling challenge.
In Scientific reports ; h5-index 158.0
Seo Junhyeon, Raeymaekers Bart
2023-Feb-08
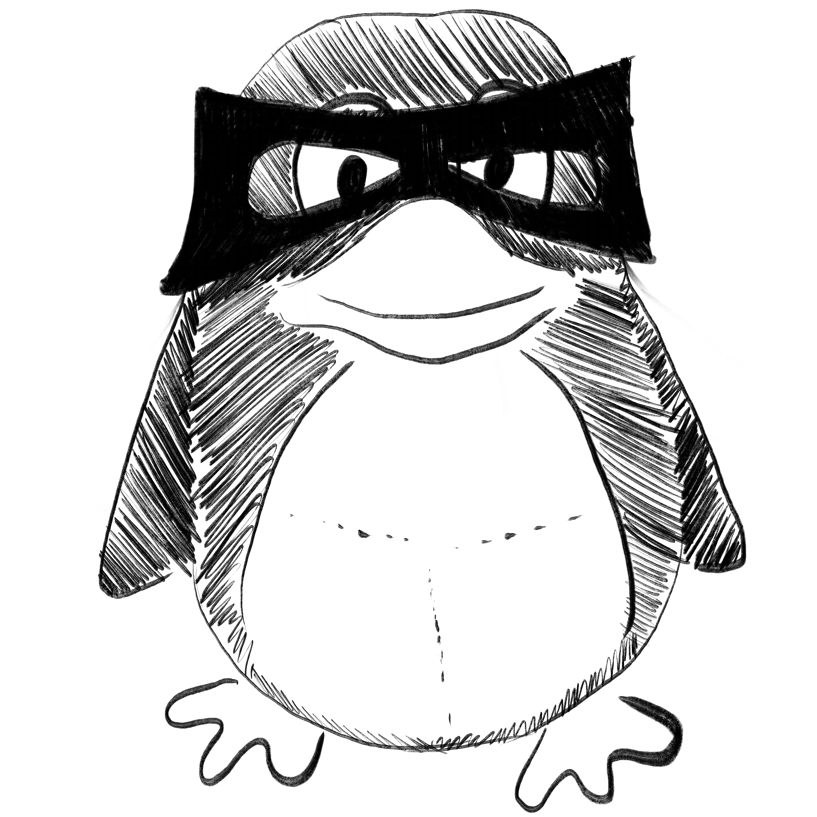
Outcome classification model for Covid-19 patients using artificial intelligence.
In Salud publica de Mexico
Saad-Manzanera María Isabel, Hernández-Galván Jesús Alan, González-Cristóbal Sofía Carolina, Vázquez-Torres Eliden, Ramírez-Alonso Graciela María de Jesús, Valenzuela-Aldaba Yaeli Estefanía, Hinojos-Gallardo Luis Carlos, Enríquez-Sánchez Luis Bernardo
2023-Jan-02
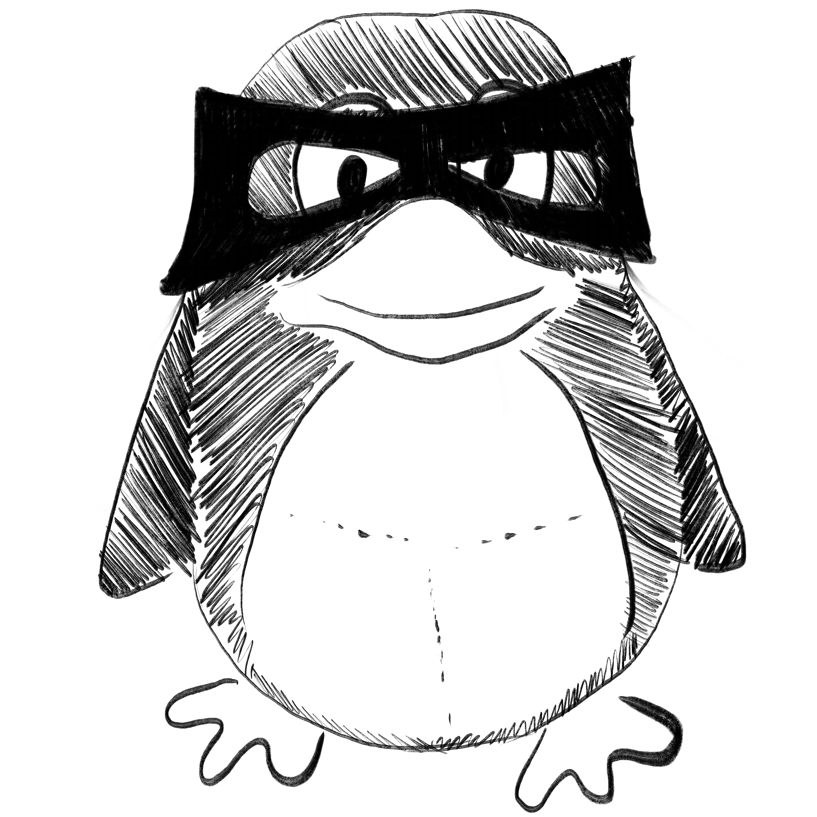
COVID-19 Predictive Models Based on Grammatical Evolution.
In SN computer science
Tsoulos Ioannis G, Stylios Chrysostomos, Charalampous Vlasis
2023
COVID-19, Feature construction, Grammatical evolution, Machine learning, Predictive models
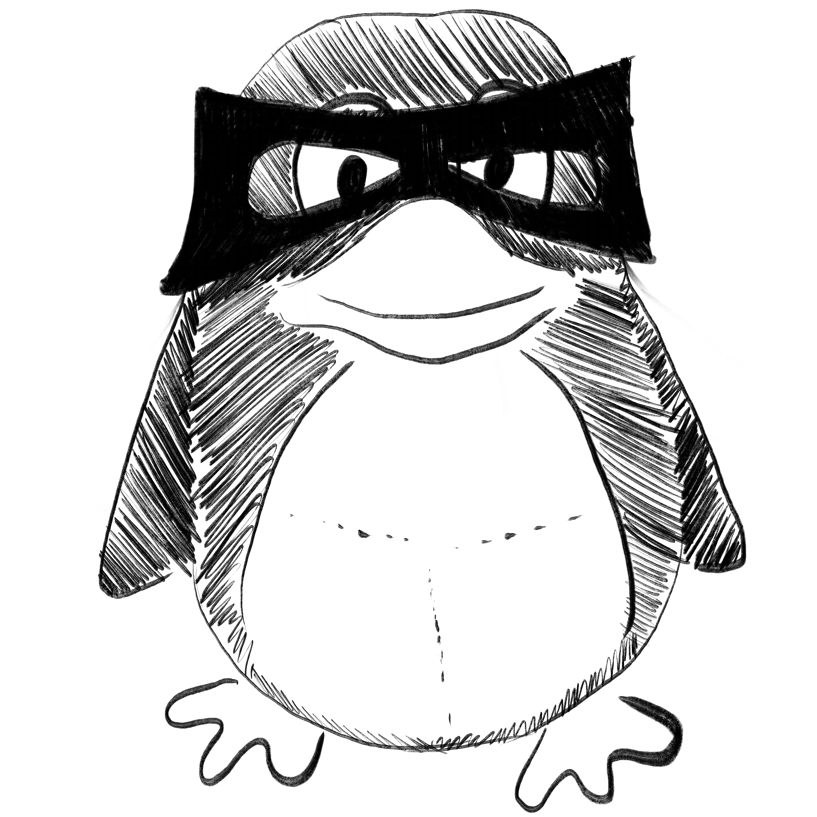
Recent development of machine learning models for the prediction of drug-drug interactions.
In The Korean journal of chemical engineering
Hong Eujin, Jeon Junhyeok, Kim Hyun Uk
2023
Adverse Drug Reaction, Drug-Drug Interaction, Featurization, Machine Learning, Polypharmacy
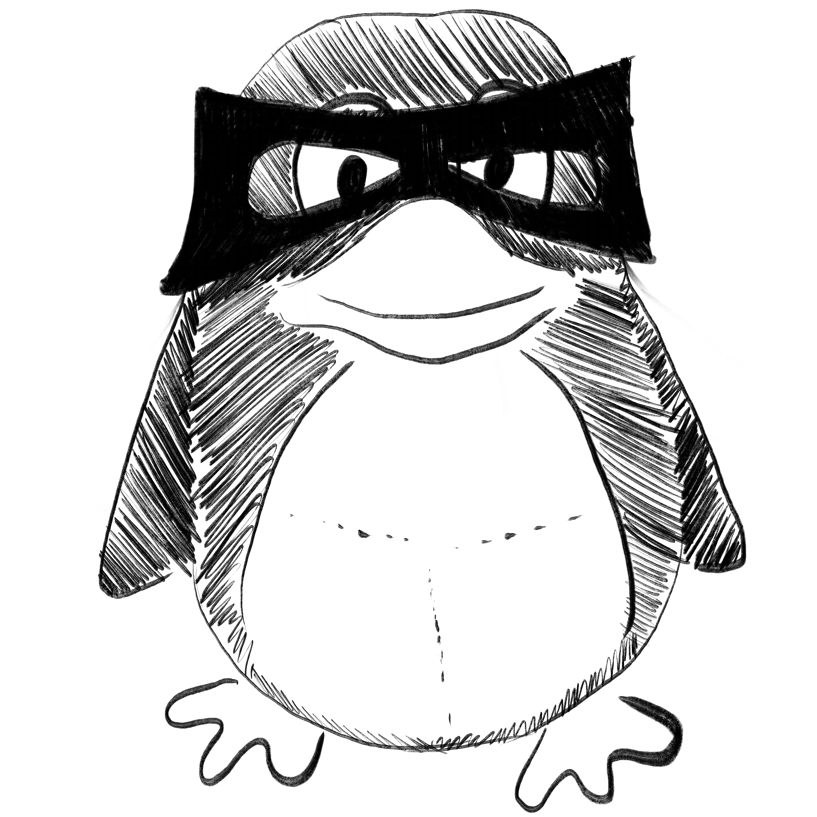
A smartphone-based zero-effort method for mitigating epidemic propagation.
In EURASIP journal on advances in signal processing
Wang Qu, Fu Meixia, Wang Jianquan, Sun Lei, Huang Rong, Li Xianda, Jiang Zhuqing
2023
COVID-19, Contact tracing, Epidemic warning, Human activity recognition, Indoor positioning, Social distance
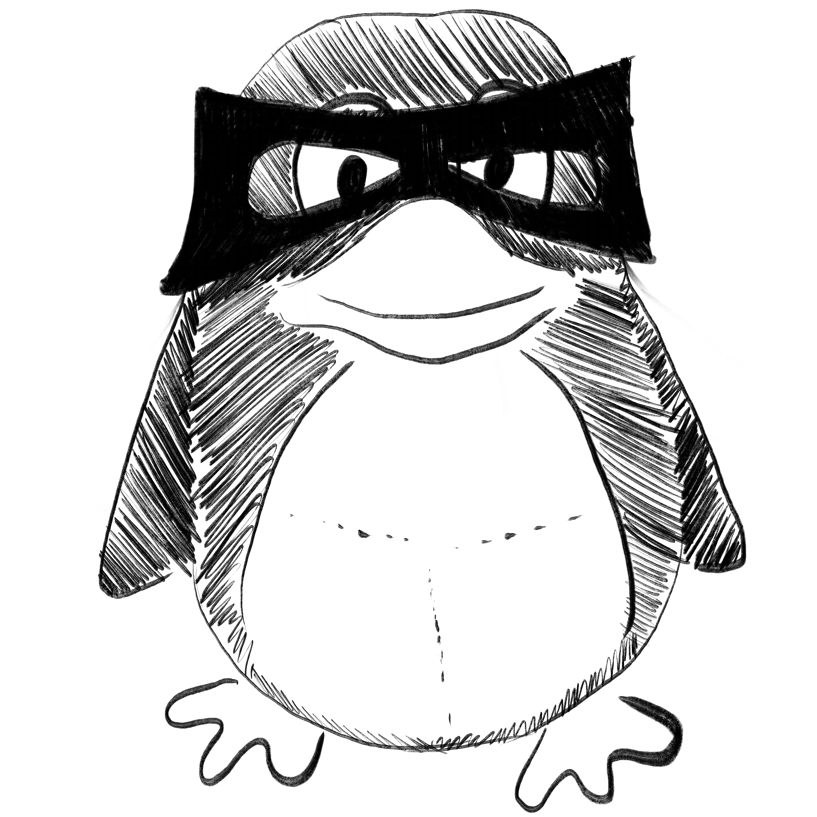
Real-time face mask position recognition system based on MobileNet model.
In Smart health (Amsterdam, Netherlands)
Rahman Md Hafizur, Jannat Mir Kanon Ara, Islam Md Shafiqul, Grossi Giuliano, Bursic Sathya, Aktaruzzaman Md
2023-Jan-31
COVID-19, Dataset, Face-mask position recognition, MobileNet, Real-time, Transfer learning
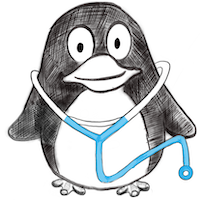
Machine learning models for predicting severe COVID-19 outcomes in hospitals.
In Informatics in medicine unlocked
Wendland Philipp, Schmitt Vanessa, Zimmermann Jörg, Häger Lukas, Göpel Siri, Schenkel-Häger Christof, Kschischo Maik
2023
Clinical decision support, Covid-19, Machine learning, Predictive modelling
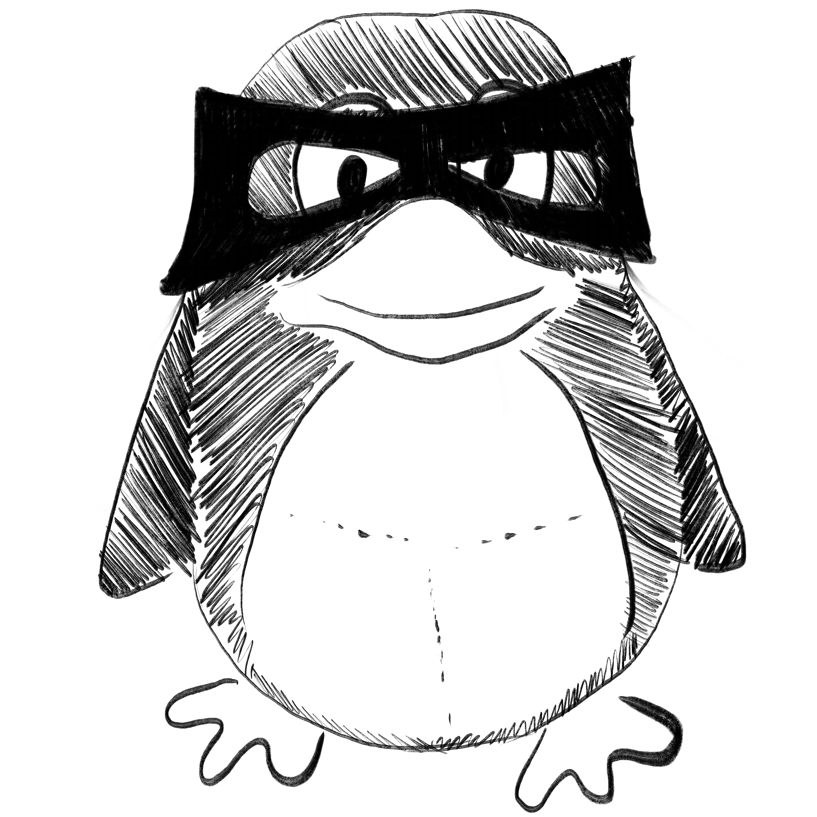
Omnidirectional 2.5D representation for COVID-19 diagnosis using chest CTs.
In Journal of visual communication and image representation
da Silveira Thiago L T, Pinto Paulo G L, Lermen Thiago S, Jung Cláudio R
2023-Mar
2.5D representation, COVID-19 diagnosis, Ground-glass opacity, Omnidirectional imaging
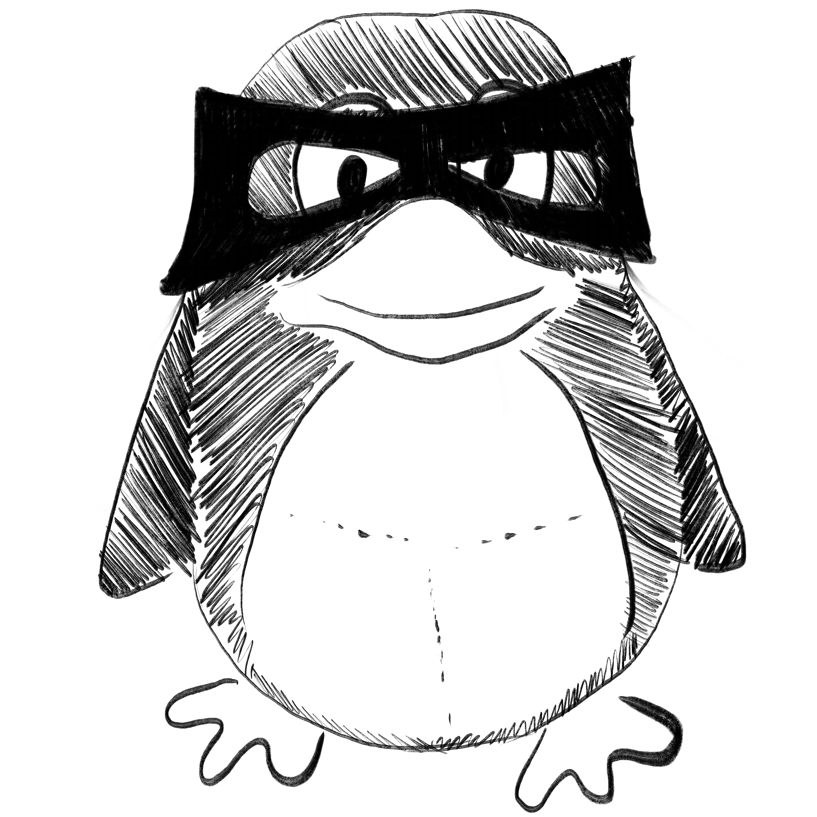
A Comparative Analysis of Multidimensional COVID-19 Poverty Determinants: An Observational Machine Learning Approach.
In New generation computing
Satapathy Sandeep Kumar, Saravanan Shreyaa, Mishra Shruti, Mohanty Sachi Nandan
2023-Feb-01
Feature selection, Machine learning, Multidimensional, Poverty, Prediction, Regression
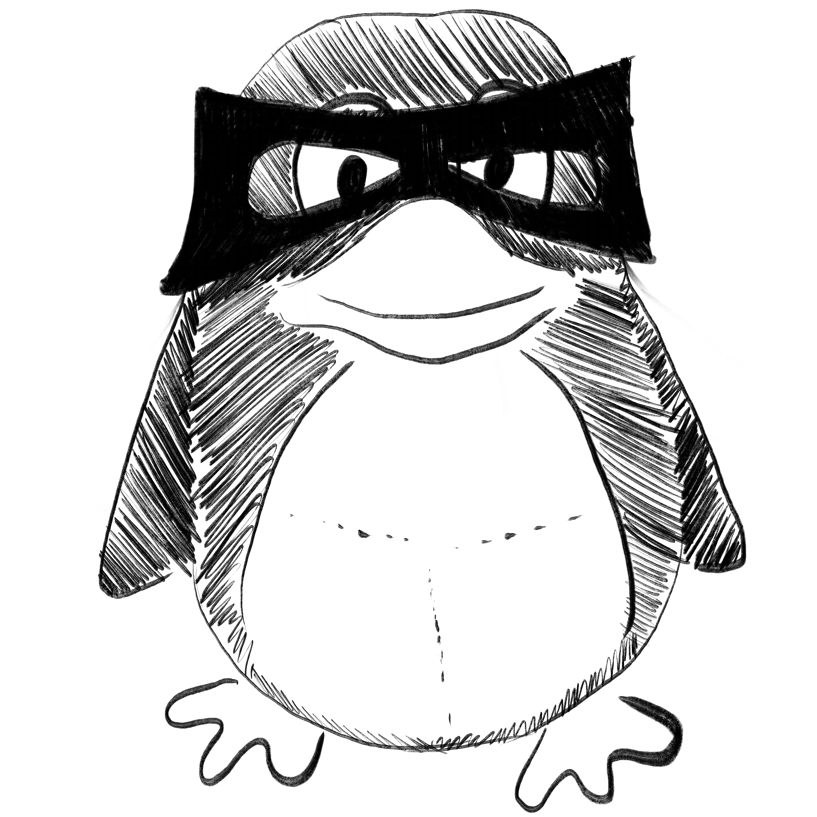
Transfer learning for the efficient detection of COVID-19 from smartphone audio data.
In Pervasive and mobile computing
Campana Mattia Giovanni, Delmastro Franca, Pagani Elena
2023-Feb
COVID-19, Deep audio embeddings, Deep learning, Transfer learning, m-health
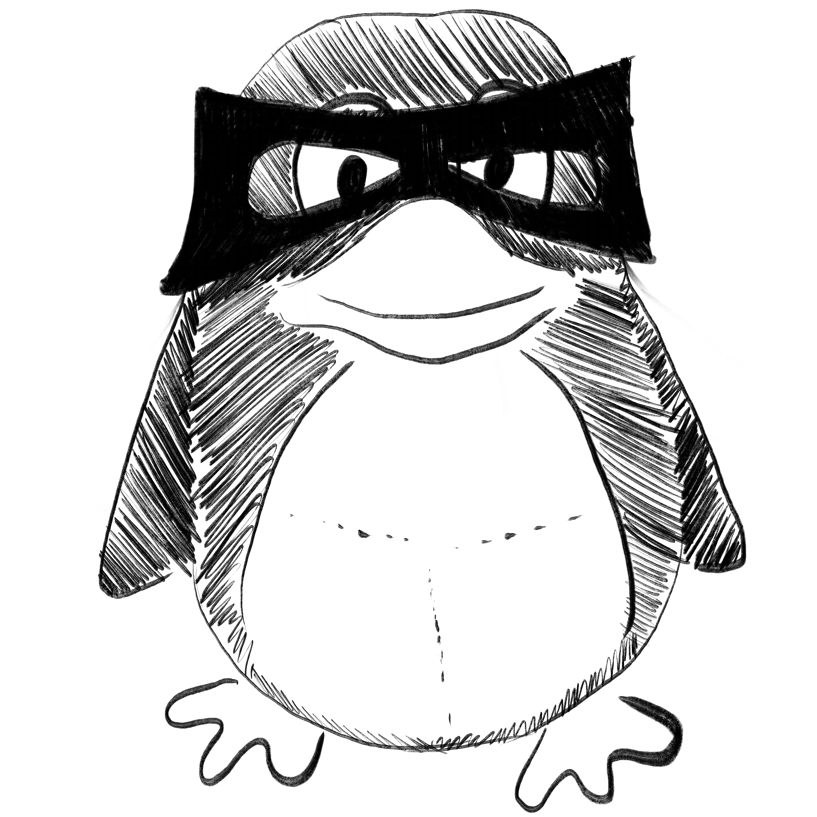
A novel approach for detection of COVID-19 and Pneumonia using only binary classification from chest CT-scans.
In Neuroscience informatics
Hasija Sanskar, Akash Peddaputha, Bhargav Hemanth Maganti, Kumar Ankit, Sharma Sanjeev
2022-Dec
CNN, COVID-19, Chest CT scan, Classification metrics, Multiclass classification, Two binary classifications
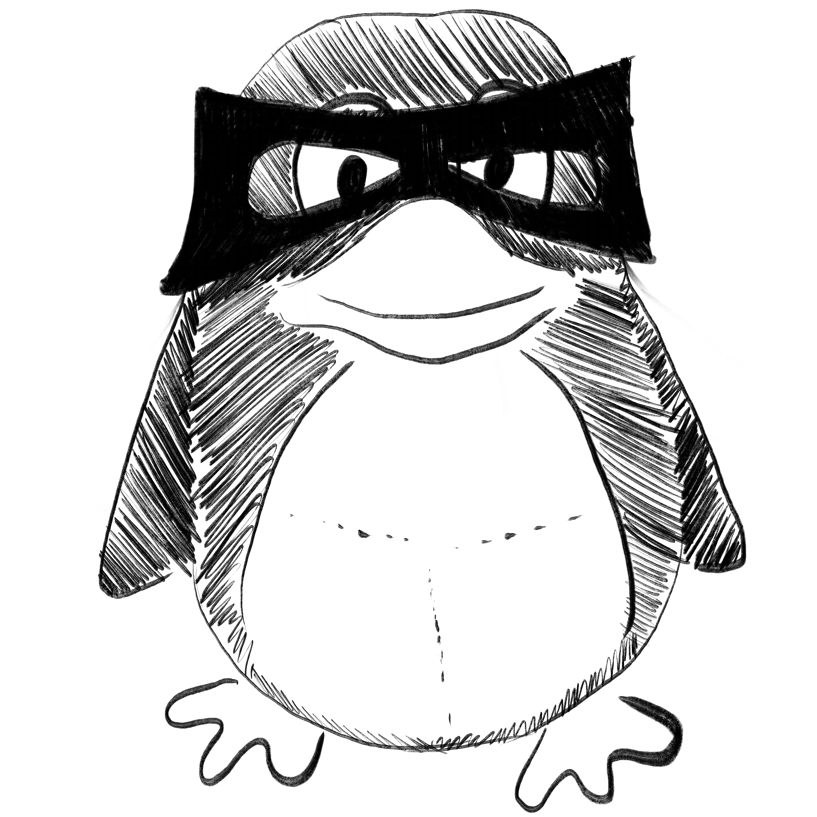
Configurational patterns for COVID-19 related social media rumor refutation effectiveness enhancement based on machine learning and fsQCA.
In Information processing & management
Li Zongmin, Zhao Ye, Duan Tie, Dai Jingqi
2023-May
COVID-19, Fuzzy-set qualitative comparative analysis (FsQCA), Infodemic, LGBM regression model, Rumor refutation effectiveness
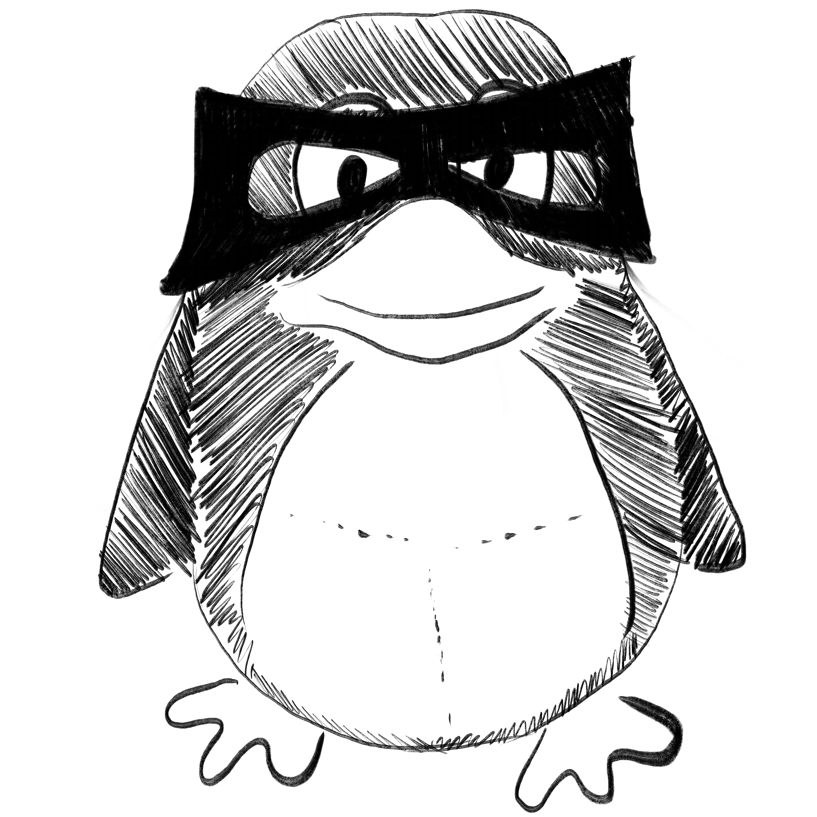
Modeling the artificial intelligence-based imperatives of industry 5.0 towards resilient supply chains: A post-COVID-19 pandemic perspective.
In Computers & industrial engineering
Ahmed Tazim, Lekha Karmaker Chitra, Benta Nasir Sumaiya, Abdul Moktadir Md, Kumar Paul Sanjoy
2023-Jan-31
Bayesian Best-Worst Method, Industry 5.0, Post-COVID-19 pandemic, artificial intelligence, supply chain resilience
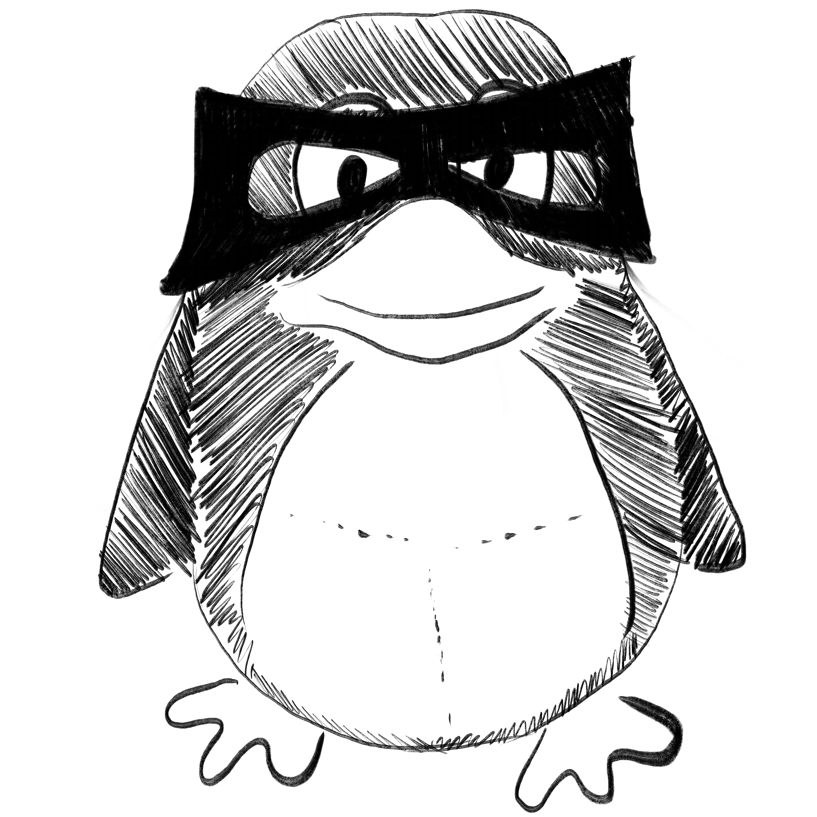
AI-accelerated protein-ligand docking for SARS-CoV-2 is 100-fold faster with no significant change in detection.
In Scientific reports ; h5-index 158.0
Clyde Austin, Liu Xuefeng, Brettin Thomas, Yoo Hyunseung, Partin Alexander, Babuji Yadu, Blaiszik Ben, Mohd-Yusof Jamaludin, Merzky Andre, Turilli Matteo, Jha Shantenu, Ramanathan Arvind, Stevens Rick
2023-Feb-06
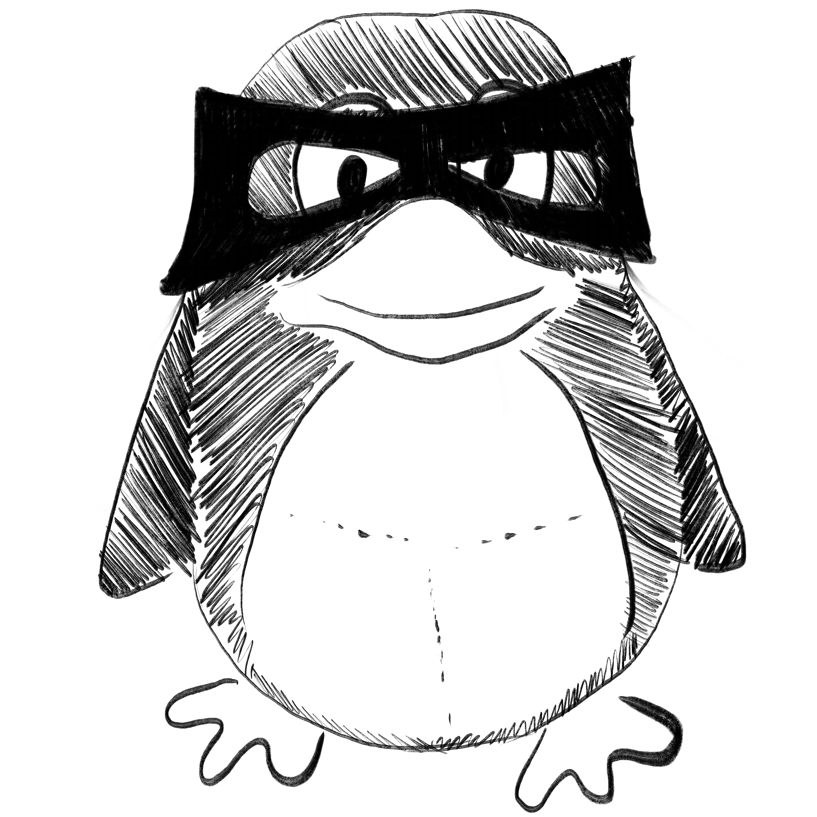
Evolution of Clinical Phenotypes of COVID-19 Patients During Intensive Care Treatment: An Unsupervised Machine Learning Analysis.
In Journal of intensive care medicine ; h5-index 29.0
BACKGROUND :
METHODS :
RESULTS :
CONCLUSIONS :
Siepel Sander, Dam Tariq A, Fleuren Lucas M, Girbes Armand R J, Hoogendoorn Mark, Thoral Patrick J, Elbers Paul W G, Bennis Frank C
2023-Feb-06
clinical phenotype half-life, clinical phenotypes, clustering, coronavirus disease 2019, endotypes, intensive care, subphenotypes
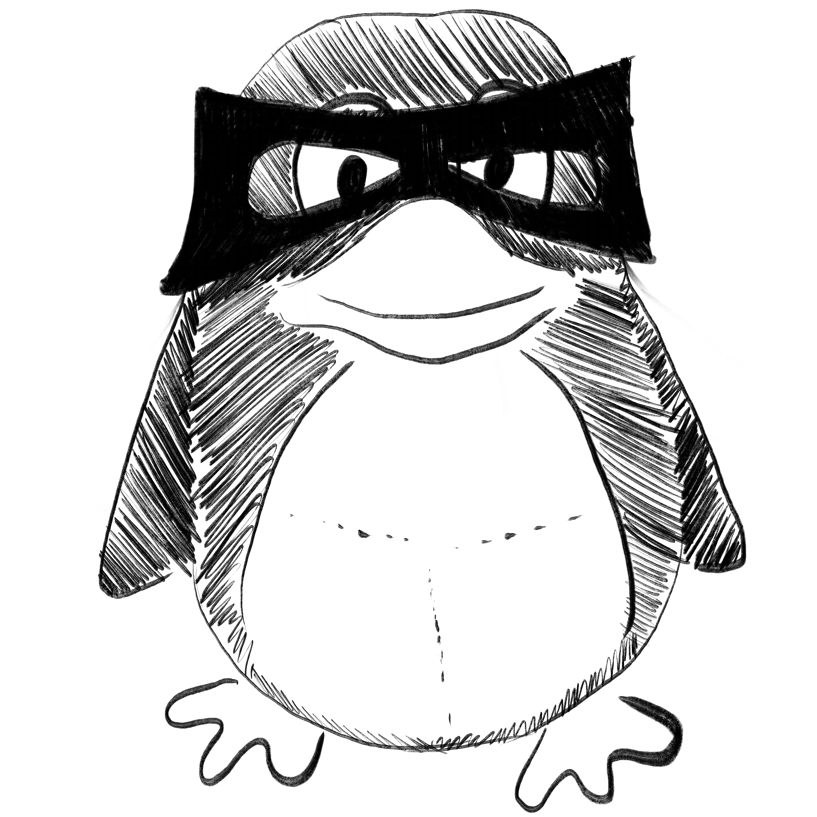
A new YOLO-based method for real-time crowd detection from video and performance analysis of YOLO models.
In Journal of real-time image processing
Gündüz Mehmet Şirin, Işık Gültekin
2023
Area estimation, Deep learning, People counting, Person detection, Real-time video processing, YOLO
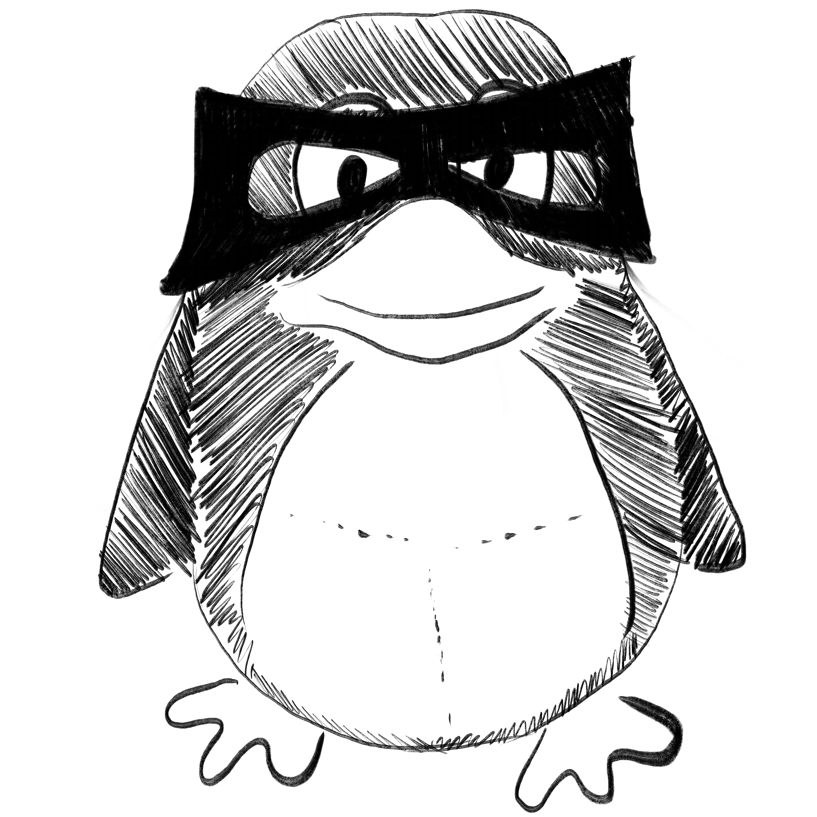
[Performance in prognostic capacity and efficiency of the Thoracic Care Suite GE AI tool applied to chest radiography of patients with COVID-19 pneumonia].
In Radiologia
OBJECTIVE :
METHODS :
RESULTS :
CONCLUSIONS :
Plasencia-Martínez Juana María, Pérez-Costa Rafael, Ballesta-Ruiz Mónica, María García-Santos José
2023-Jan-31
AI (Artificial Intelligence), Biomedical Technology, COVID 19, Coronavirus, Prognoses, Radiography
Effect of daily new cases of COVID-19 on public sentiment and concern: Deep learning-based sentiment classification and semantic network analysis.
In Zeitschrift fur Gesundheitswissenschaften = Journal of public health
AIM :
SUBJECT AND METHODS :
RESULTS :
CONCLUSION :
Che ShaoPeng, Wang Xiaoke, Zhang Shunan, Kim Jang Hyun
2023-Jan-31
COVID-19, Crisis and Emergency Risk Communication, Daily new cases, Deep learning, Public health agency, Semantic network analysis
Whale Optimization with Random Contraction and Rosenbrock Method for COVID-19 disease prediction.
In Biomedical signal processing and control
Zhang Meilin, Wu Qianxi, Chen Huiling, Asghar Heidari Ali, Cai Zhennao, Li Jiaren, Md Abdelrahim Elsaid, Mansour Romany F
2023-Feb-01
COVID-19, Feature selection, Random contraction strategy, Rosenbrock method, Swarm intelligence, Whale optimization algorithm
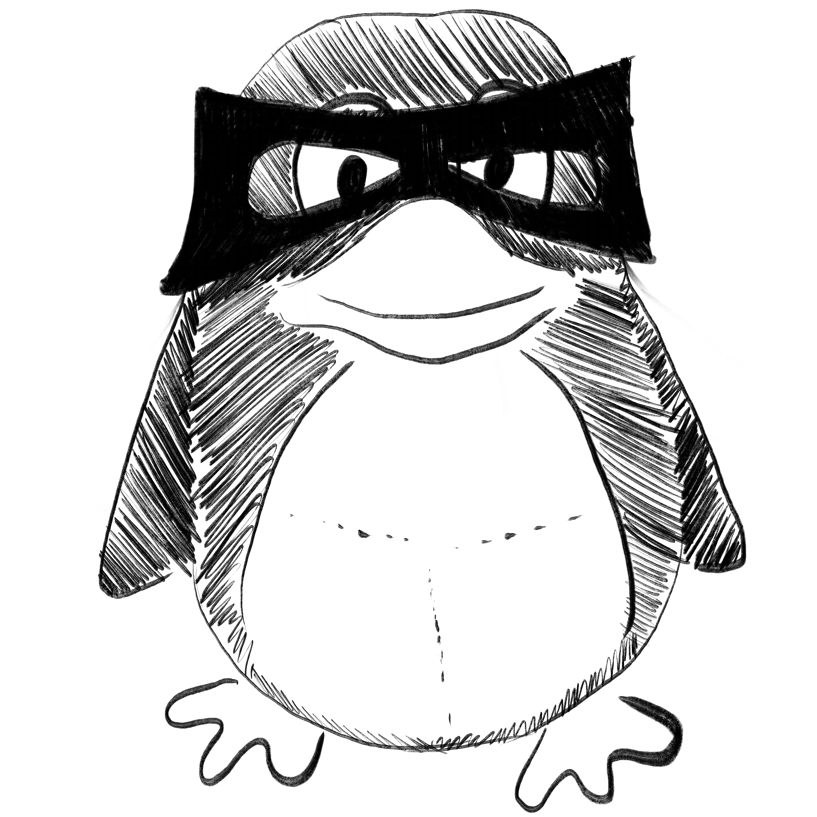
Prediction and comparison of psychological health during COVID-19 among Indian population and Rajyoga meditators using machine learning algorithms.
In Procedia computer science
Shobhika Kumar, Prashant Chandra
2023
COVID-19, DASS, Machine learning algorithms, mental health
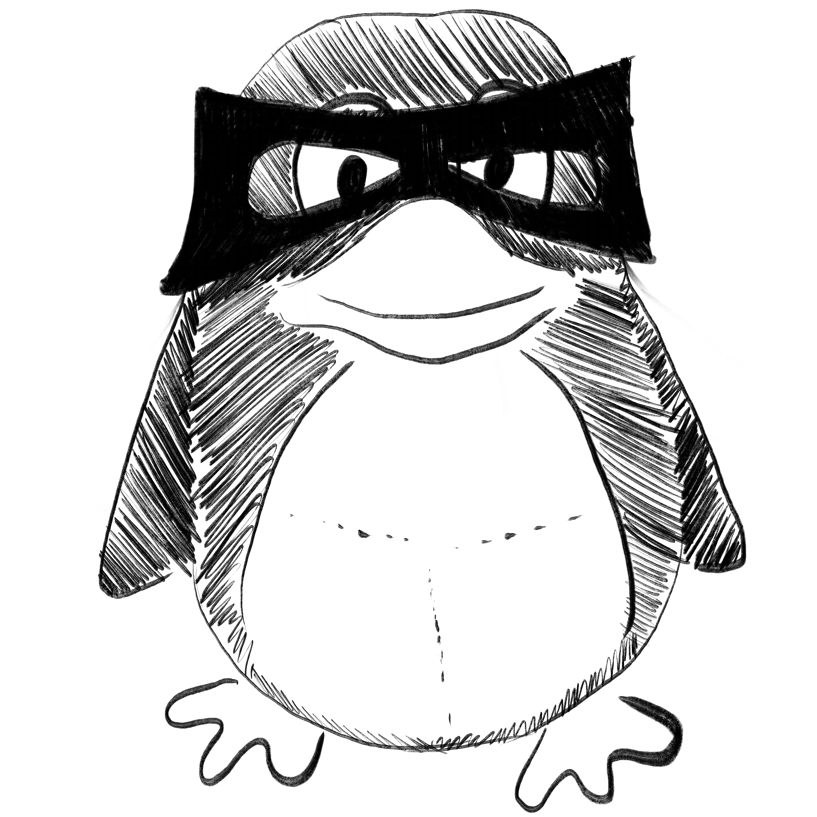
A Unified Framework for Monitoring Social Distancing and Face Mask Wearing Using Deep Learning: An Approach to Reduce COVID-19 Risk.
In Procedia computer science
Kaviya P, Chitra P, Selvakumar B
2023
COVID-19, Deep Learning, Face Mask Detection, ResNet50v2, Social Distance Prediction, Top View Transform Model (TVTM), YOLOv3
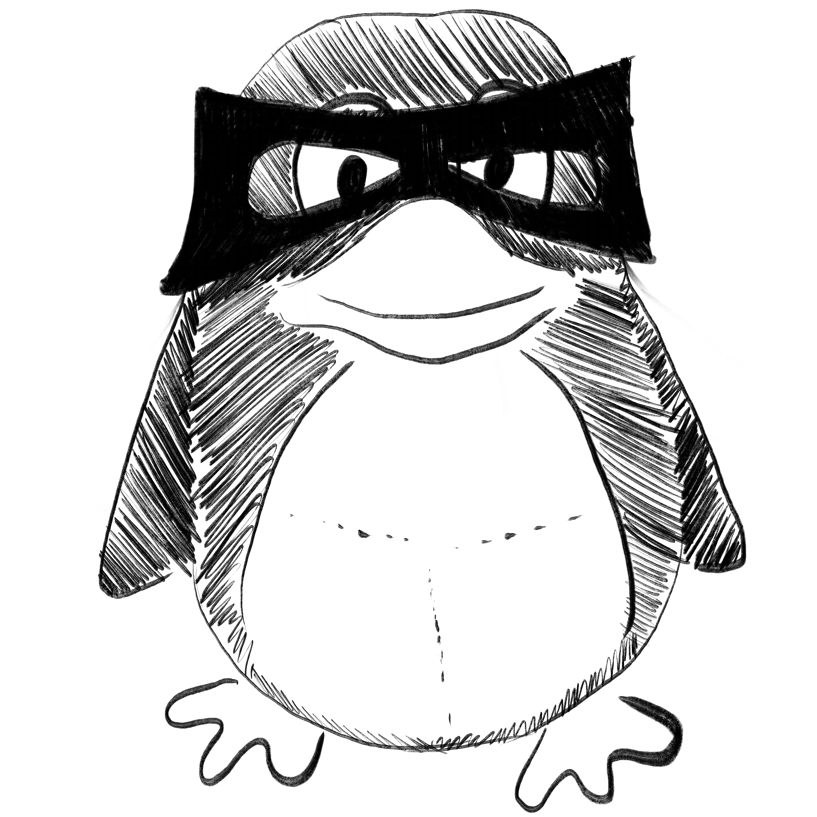
Coronavirus disease identification using Multi-subband feature analysis in DWT domain.
In Procedia computer science
Ali Nikhat, Yadav Jyotsna
2023
Covid-19, Discrete wavelet transform (DWT), X-ray, classification, machine learning, multi-subband
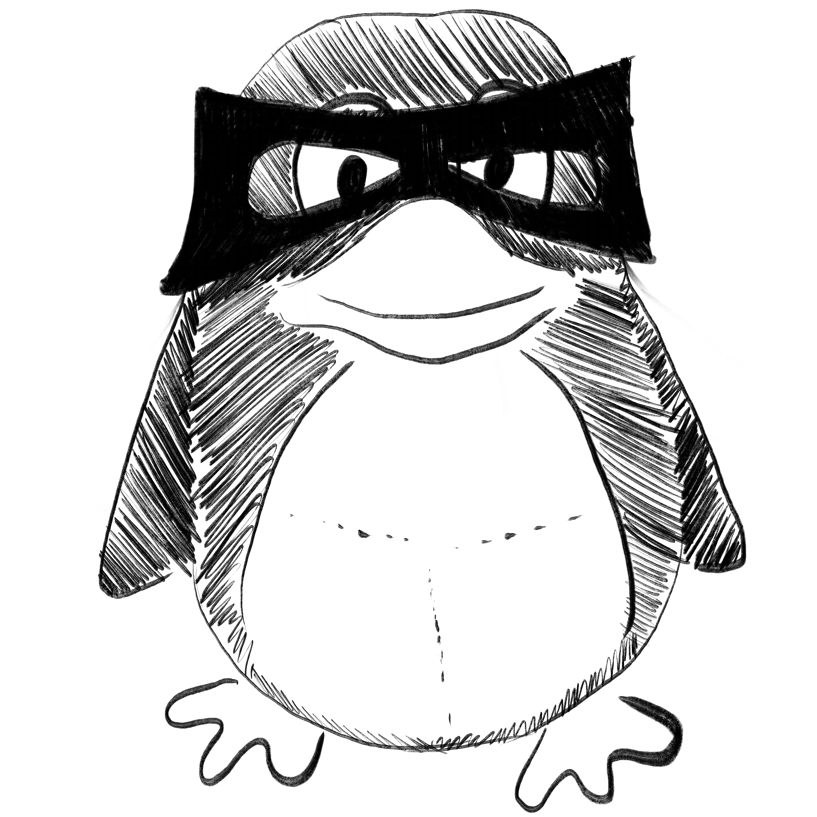
Data Mining Based Techniques for Covid-19 Predictions.
In Procedia computer science
Rane Rahul, Dubey Aditya, Rasool Akhtar, Wadhvani Rajesh
2023
Deep Learning Models, Soft Computing-based Models, Stochastic Forecasting Models, Supervised ML Models
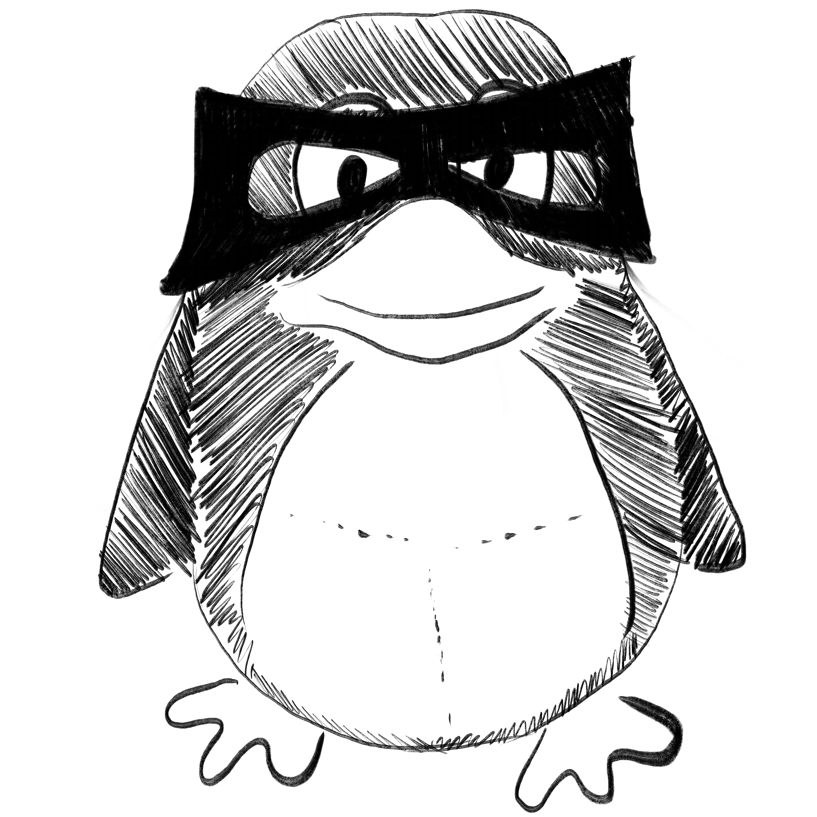
SCS-Net: An efficient and practical approach towards Face Mask Detection.
In Procedia computer science
Masud Umar, Siddiqui Momin, Sadiq Mohd, Masood Sarfaraz
2023
CNNs, cosine similarity, covid-19, deep learning, face mask detection, image classification
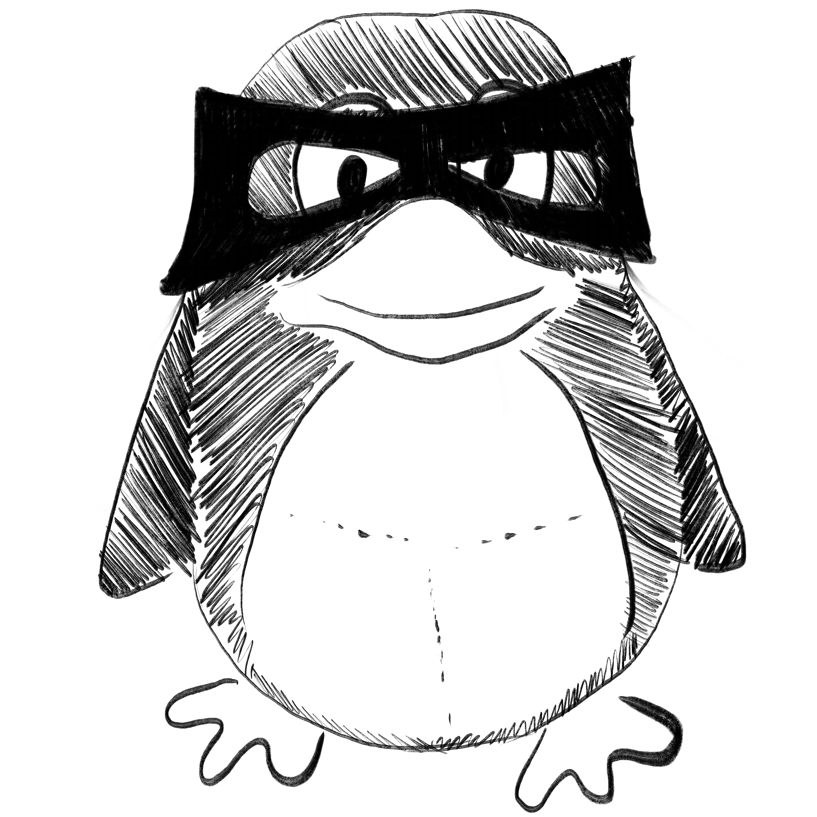
VGG-COVIDNet: A Novel model for COVID detection from X-Ray and CT Scan images.
In Procedia computer science
Goyal Lakshay, Dhull Anuradha, Singh Akansha, Kukreja Sonal, Singh Krishna Kant
2023
Covid-19;Deep Learning, VGG-NET;Medical Iamging
AMSFMap Methodology to improve prediction accuracy of CNN model for Covid19 using X-ray images.
In Procedia computer science
Chauhan Hetal, Modi Kirit
2023
CNN, Channel Attention, Covid-19 Diagnosis, Deep Learning, Multi Scale Features, Severity Prediction
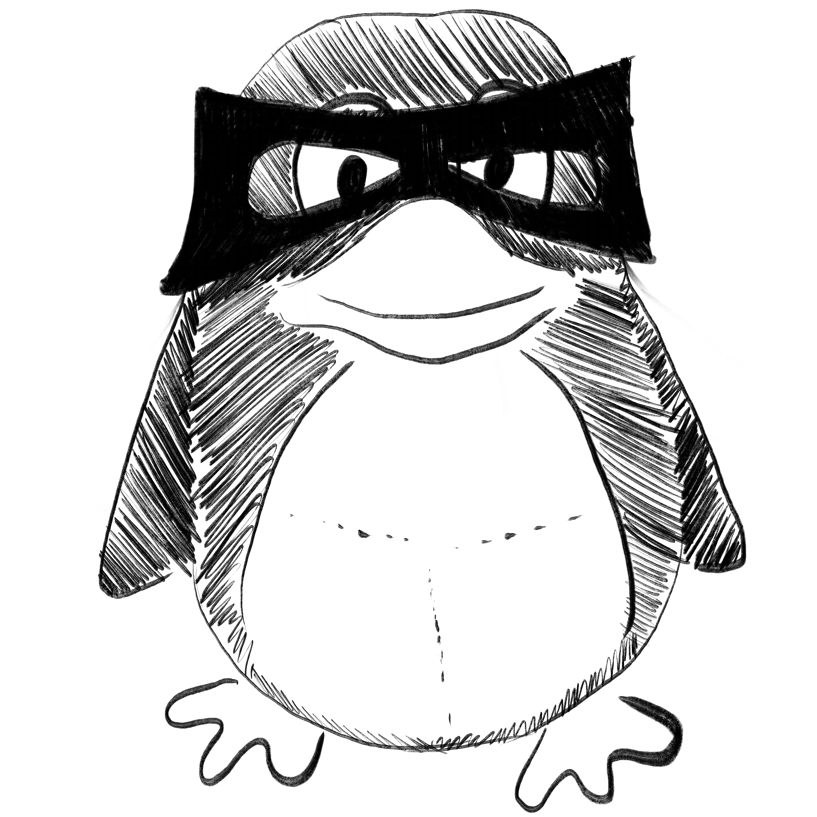
COVID-19 Lung CT image segmentation using localization and enhancement methods with U-Net.
In Procedia computer science
Ilhan Ahmet, Alpan Kezban, Sekeroglu Boran, Abiyev Rahib
2023
COVID-19, Enhancement, Localization, Lung CT, U-Net
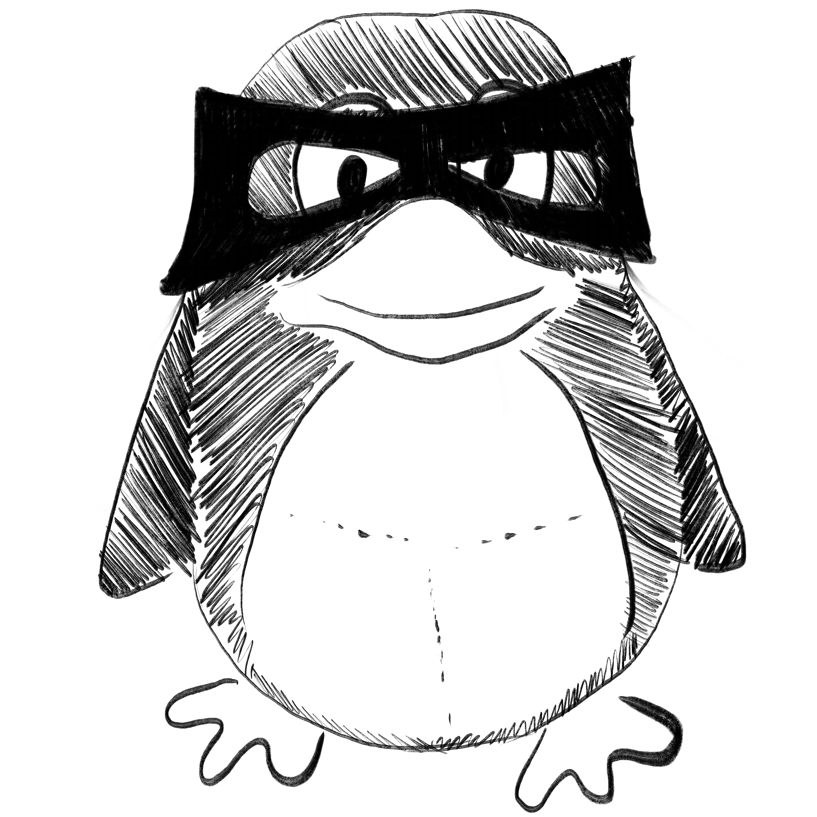
Simulating Federated Transfer Learning for Lung Segmentation using Modified UNet Model.
In Procedia computer science
Ambesange Sateesh, Annappa B, Koolagudi Shashidhar G
2023
Federated Learning, Federated Transfer Learning, Lung image segmentation, MRI image segmentation, Transfer Learning, U-net Architecture, X-ray Image segmentation, data privacy
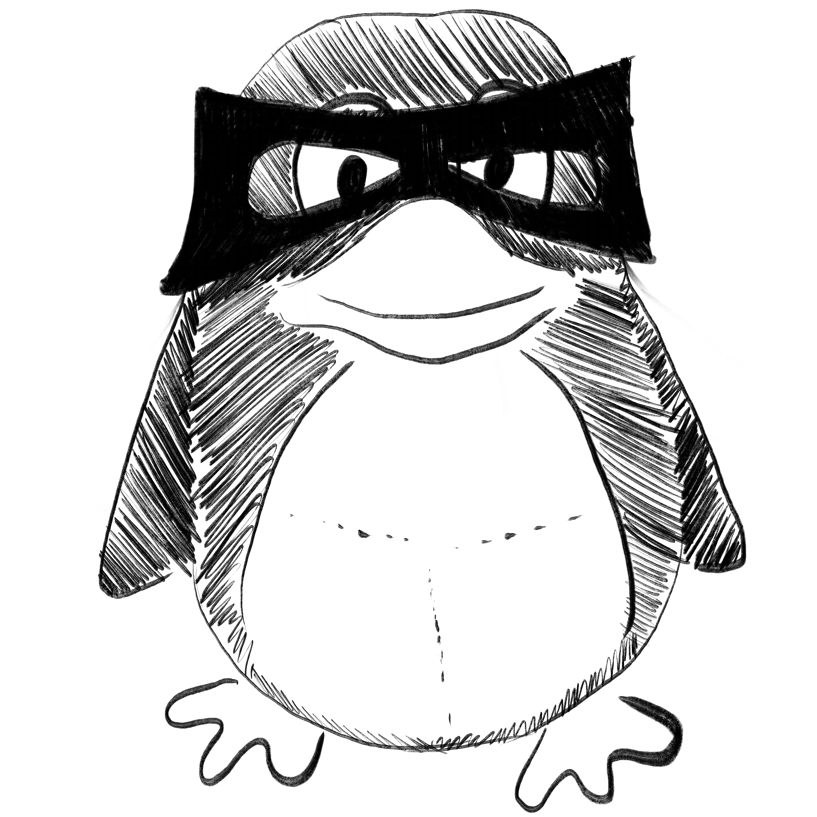
Impact on Air Quality Index of India Due to Lockdown.
In Procedia computer science
Dubey Aditya, Rasool Akhtar
2023
Deep Learning Models, Soft Computing-based Models, Stochastic Forecasting Models, Supervised ML Models
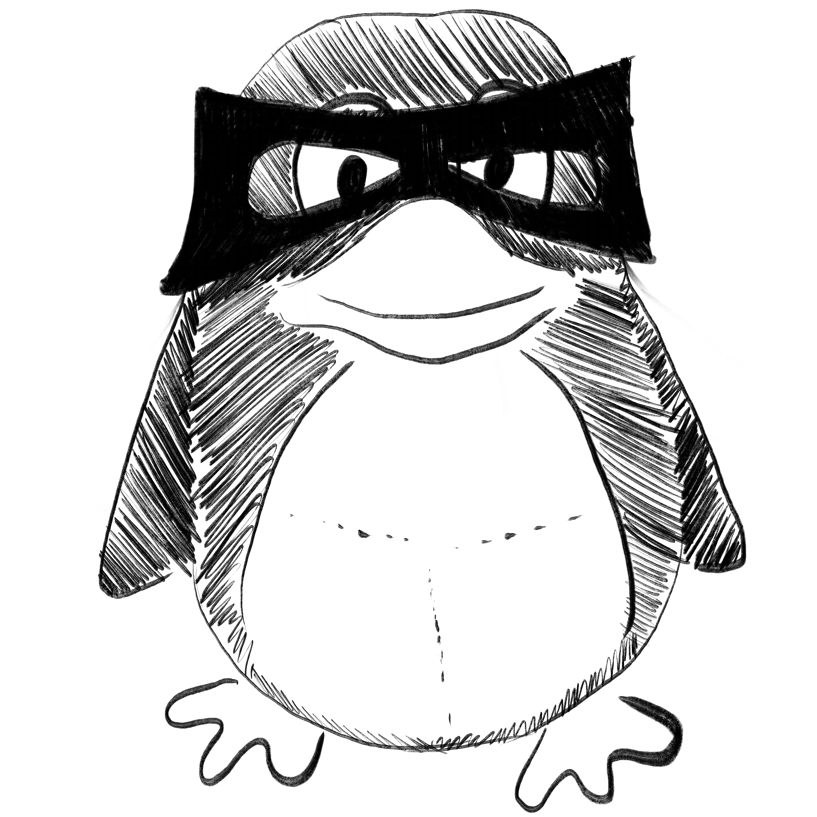
DapNet-HLA: Adaptive dual-attention mechanism network based on deep learning to predict non-classical HLA binding sites.
In Analytical biochemistry
Jing Yuanyuan, Zhang Shengli, Wang Houqiang
2023-Feb-03
Bahdanau attention mechanism, Non-classical HLA binding sites, SENet attention mechanism, Word embedding
Explainable artificial intelligence model for identifying COVID-19 gene biomarkers.
In Computers in biology and medicine
AIM :
METHODS :
RESULTS :
CONCLUSIONS :
Yagin Fatma Hilal, Cicek İpek Balikci, Alkhateeb Abedalrhman, Yagin Burak, Colak Cemil, Azzeh Mohammad, Akbulut Sami
2023-Feb-01
COVID-19, Explainable artificial intelligence, LIME, SHAP, XGBoost
Classification of COVID-19 from community-acquired pneumonia: Boosting the performance with capsule network and maximum intensity projection image of CT scans.
In Computers in biology and medicine
BACKGROUND :
METHODS :
RESULTS :
CONCLUSIONS :
Wu Yanan, Qi Qianqian, Qi Shouliang, Yang Liming, Wang Hanlin, Yu Hui, Li Jianpeng, Wang Gang, Zhang Ping, Liang Zhenyu, Chen Rongchang
2023-Jan-23
COVID-19, Capsule network, Community-acquired pneumonia, Computed tomography, Maximum intensity projection
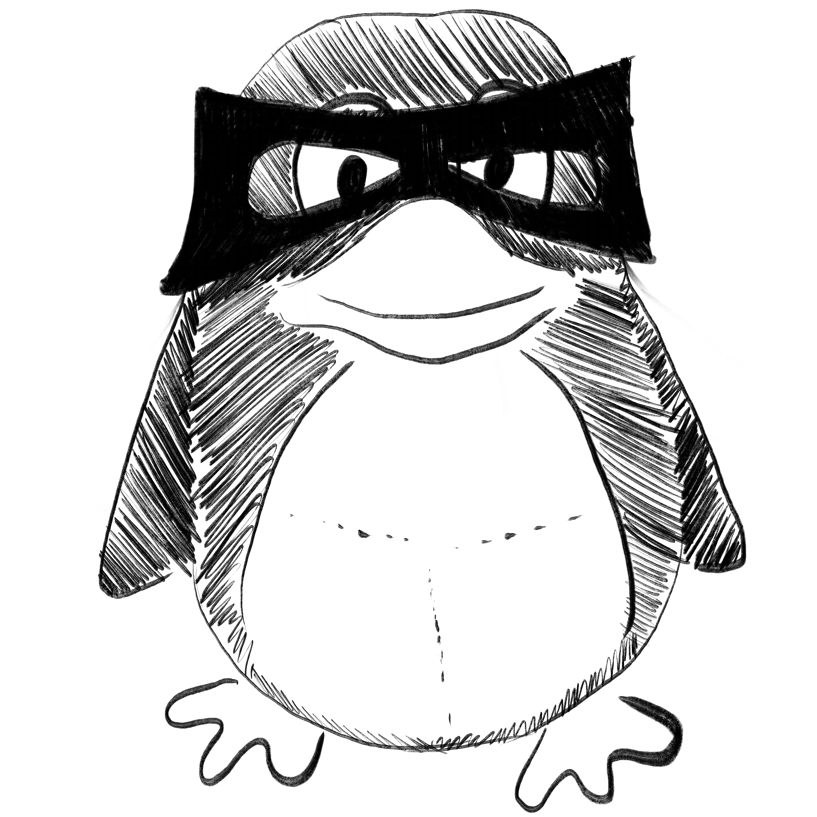
[CRISPR-based molecular diagnostics: a review].
In Sheng wu gong cheng xue bao = Chinese journal of biotechnology
Sun Wenjun, Huang Xingxu, Wang Xinjie
2023-Jan-25
CRISPR, CRISPR-based detection, gene editing, molecular detection, nucleic acid detection, point-of-care testing (POCT)
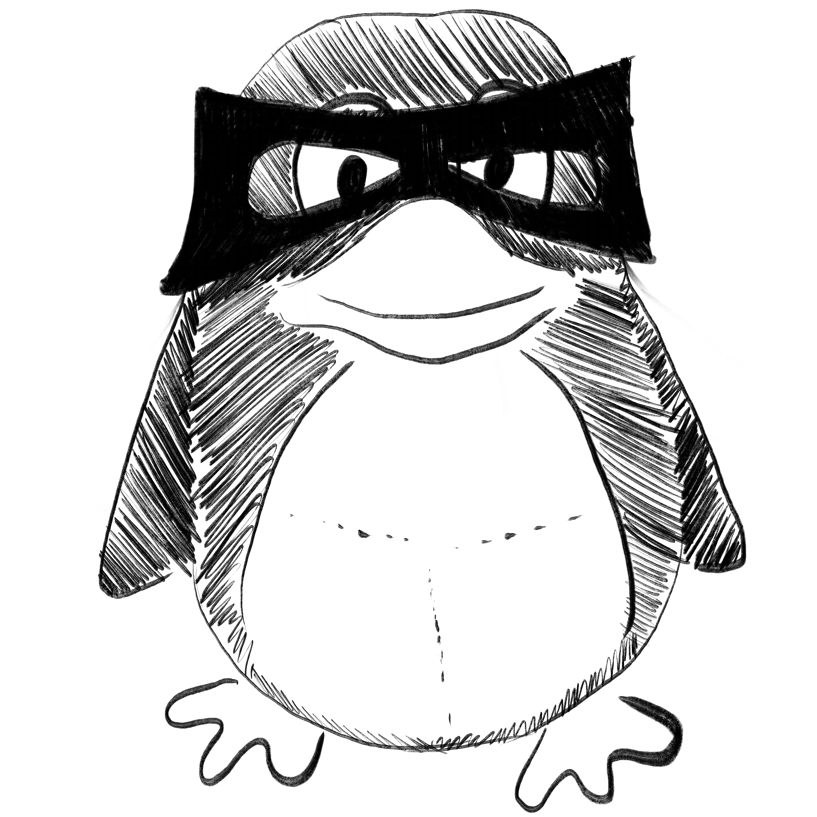
Post-COVID-19 interstitial lung disease: Insights from a machine learning radiographic model.
In Frontiers in medicine
INTRODUCTION :
METHODS :
RESULTS :
CONCLUSION :
Karampitsakos Theodoros, Sotiropoulou Vasilina, Katsaras Matthaios, Tsiri Panagiota, Georgakopoulou Vasiliki E, Papanikolaou Ilias C, Bibaki Eleni, Tomos Ioannis, Lambiri Irini, Papaioannou Ourania, Zarkadi Eirini, Antonakis Emmanouil, Pandi Aggeliki, Malakounidou Elli, Sampsonas Fotios, Makrodimitri Sotiria, Chrysikos Serafeim, Hillas Georgios, Dimakou Katerina, Tzanakis Nikolaos, Sipsas Nikolaos V, Antoniou Katerina, Tzouvelekis Argyris
2022
antifibrotics, interstitial lung disease, long COVID, machine learning, post-COVID-19
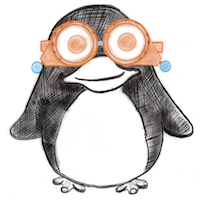
Tele-Glaucoma Using a New Smartphone-based Tool for Visual Field Assessment.
In Journal of glaucoma
PRECIS :
BACKGROUND :
METHODS :
RESULTS :
CONCLUSION :
Grau Elisabeth, Andrae Stefan, Horn Folkert, Hohberger Bettina, Ring Matthias, Michelson Georg
2022-Nov-29
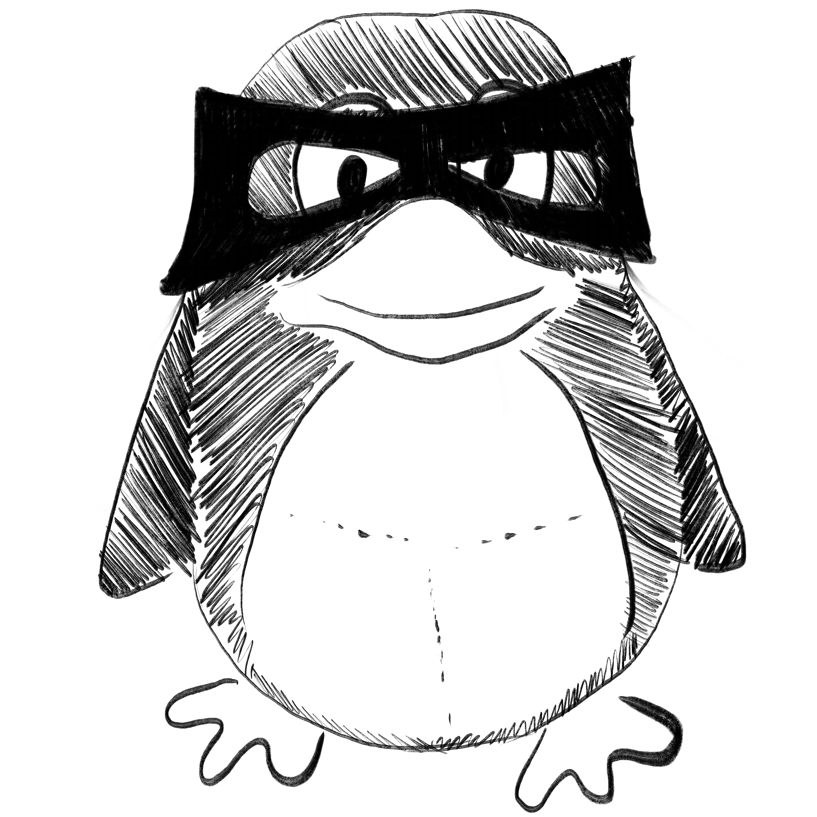
Methodological and Quality Flaws in the Use of Artificial Intelligence in Mental Health Research: Systematic Review.
In JMIR mental health
BACKGROUND :
OBJECTIVE :
METHODS :
RESULTS :
CONCLUSIONS :
Tornero-Costa Roberto, Martinez-Millana Antonio, Azzopardi-Muscat Natasha, Lazeri Ledia, Traver Vicente, Novillo-Ortiz David
2023-Feb-02
artificial intelligence, health research, mental health, research methodology, research quality, review methodology, systematic review, trial methodology
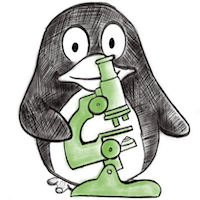
Host immunological responses facilitate development of SARS-CoV-2 mutations in patients receiving monoclonal antibody treatments.
In The Journal of clinical investigation ; h5-index 129.0
BACKGROUND :
METHODS :
RESULTS :
CONCLUSIONS :
Gupta Akshita, Konnova Angelina, Smet Mathias, Berkell Matilda, Savoldi Alessia, Morra Matteo, Van Averbeke Vincent, De Winter Fien Hr, Peserico Denise, Danese Elisa, Hotterbeekx An, Righi Elda, De Nardo Pasquale, Tacconelli Evelina, Malhotra-Kumar Surbhi, Kumar-Singh Samir
2023-Feb-09
COVID-19, Cellular immune response
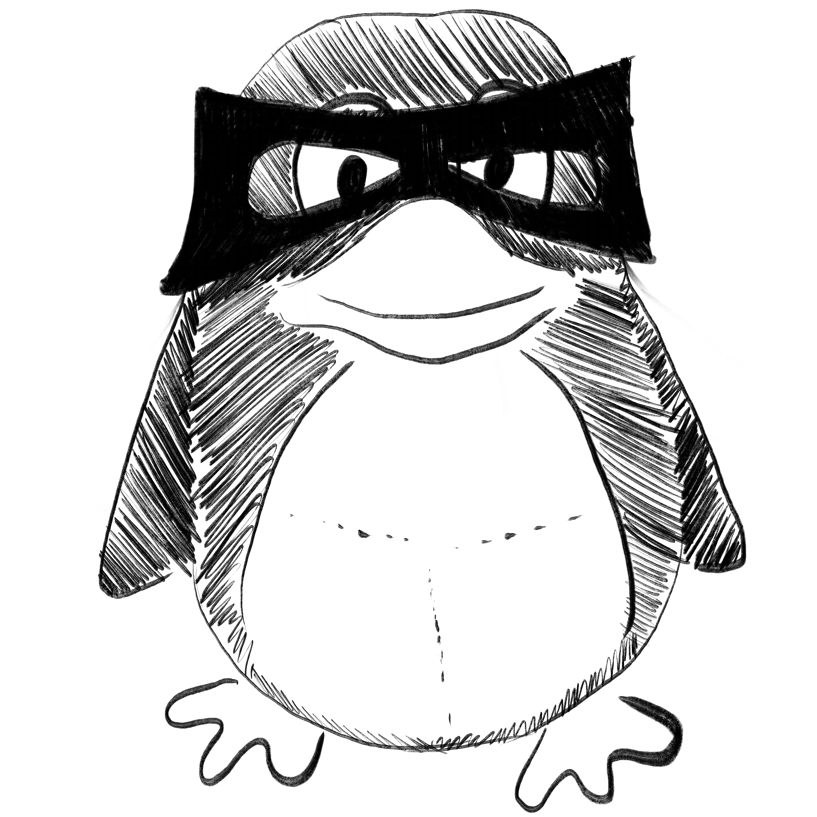
Improving effectiveness of online learning for higher education students during the COVID-19 pandemic.
In Frontiers in psychology ; h5-index 92.0
Li Xuelan, Pei Zhiqiang
2022
COVID-19, analytic hierarchy process, cluster analysis, group dynamics theory, online learning community
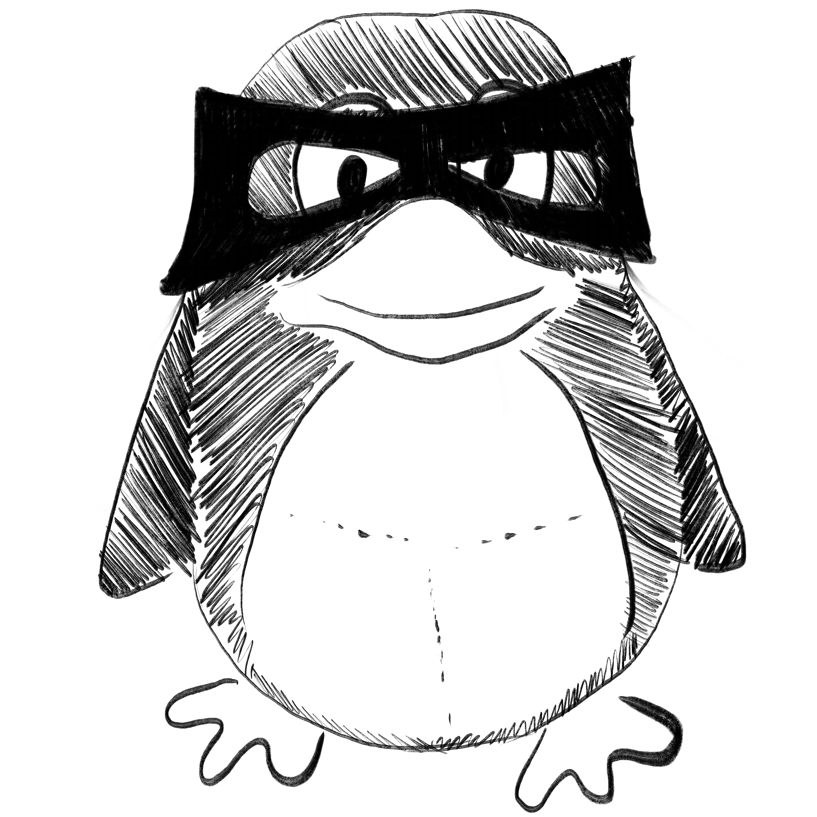
Interacting with chatbots later in life: A technology acceptance perspective in COVID-19 pandemic situation.
In Frontiers in psychology ; h5-index 92.0
INTRODUCTION :
METHODS :
RESULTS :
Iancu Ioana, Iancu Bogdan
2022
behavioral intention, chatbots, middle-aged and aging adults, perceived ease of use, perceived usefulness, technology acceptance model
Socioexposomics of COVID-19 across New Jersey: a comparison of geostatistical and machine learning approaches.
In Journal of exposure science & environmental epidemiology ; h5-index 34.0
BACKGROUND :
OBJECTIVE :
METHODS :
RESULTS :
SIGNIFICANCE :
Ren Xiang, Mi Zhongyuan, Georgopoulos Panos G
2023-Feb-01
Bayesian geospatial modeling, COVID-19, Explainable machine learning, Exposome and socioexposome, Social/environmental health disparities
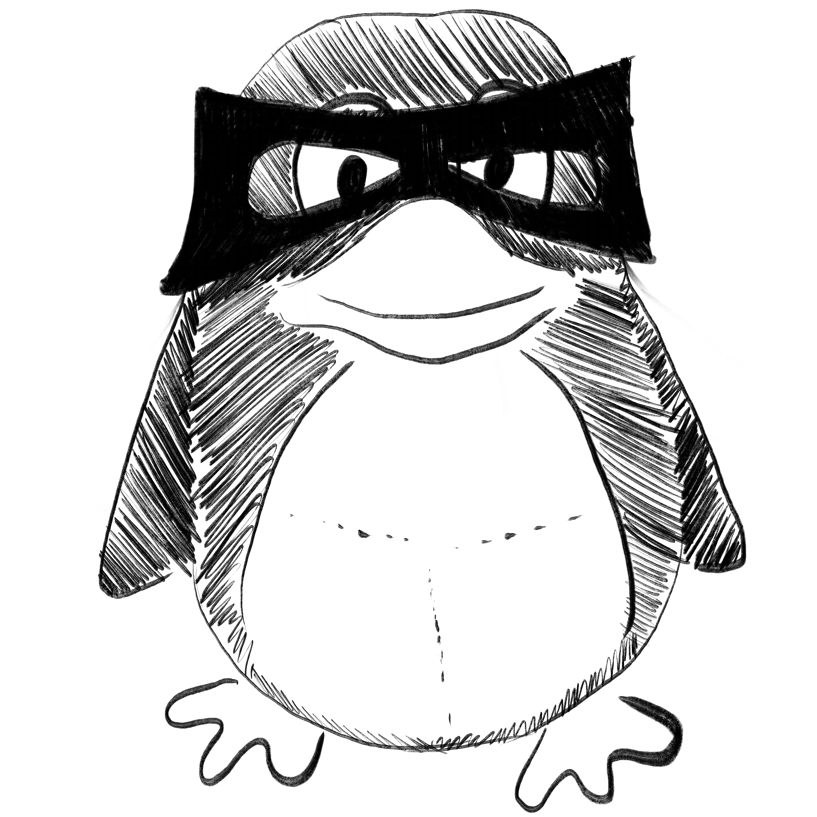
Future trajectory of respiratory infections following the COVID-19 pandemic in Hong Kong.
In Chaos (Woodbury, N.Y.)
Cheng Weibin, Zhou Hanchu, Ye Yang, Chen Yifan, Jing Fengshi, Cao Zhidong, Zeng Daniel Dajun, Zhang Qingpeng
2023-Jan
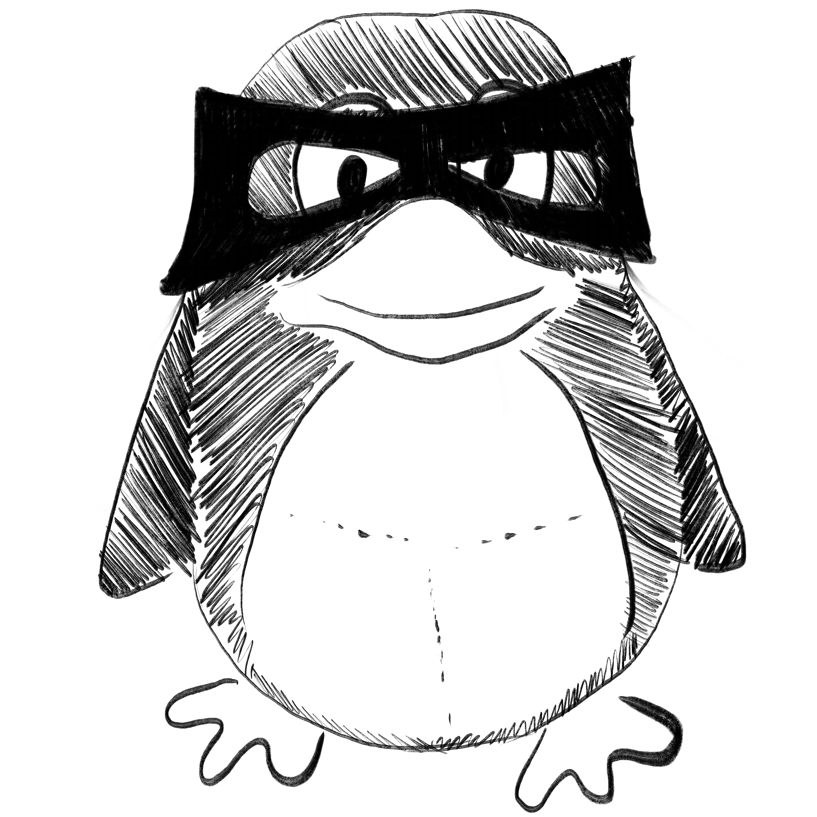
Recent Issues in Medical Journal Publishing and Editing Policies: Adoption of Artificial Intelligence, Preprints, Open Peer Review, Model Text Recycling Policies, Best Practice in Scholarly Publishing 4th Version, and Country Names in Titles.
In Neurointervention
Huh Sun
2023-Feb-01
Artificial intelligence, Culture, Peer review, Policy, Scholarly communication
Voice assistants' responses to questions about the COVID-19 vaccine: a national cross-sectional study.
In JMIR formative research
BACKGROUND :
OBJECTIVE :
METHODS :
RESULTS :
CONCLUSIONS :
Sossenheimer Philip, Hong Grace, Devon-Sand Anna, Lin Steven
2023-Jan-29
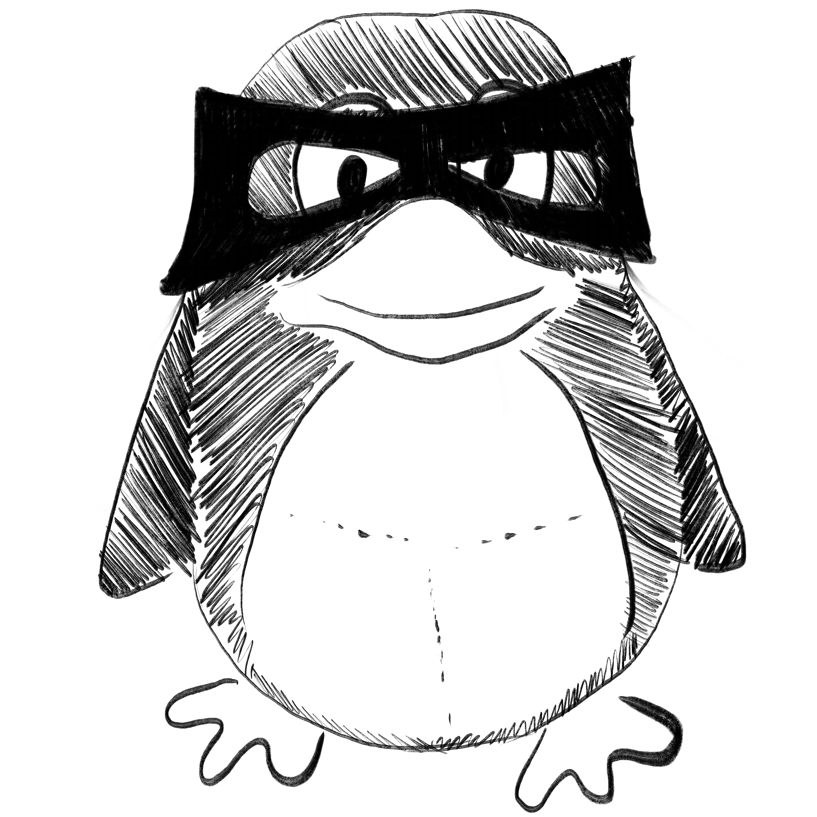
PneuNet: deep learning for COVID-19 pneumonia diagnosis on chest X-ray image analysis using Vision Transformer.
In Medical & biological engineering & computing ; h5-index 32.0
Wang Tianmu, Nie Zhenguo, Wang Ruijing, Xu Qingfeng, Huang Hongshi, Xu Handing, Xie Fugui, Liu Xin-Jun
2023-Jan-31
COVID-19, Deep learning, Multi-head attention, Pneumonia diagnosis, Vision Transformer
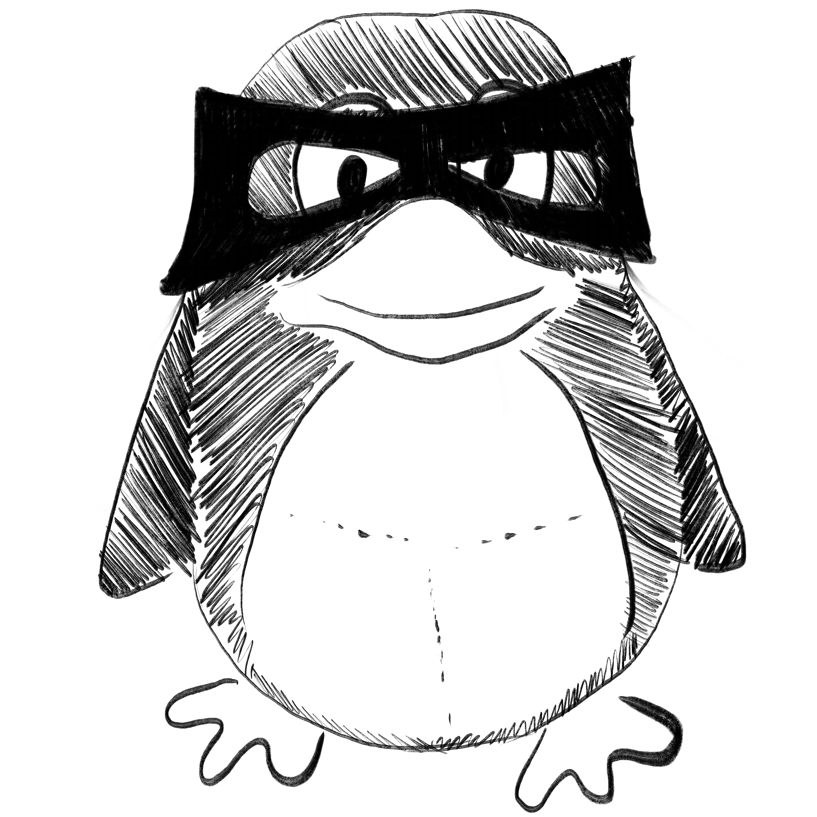
Data-driven analysis and predictive modeling on COVID-19.
In Concurrency and computation : practice & experience
Sharma Sonam, Alsmadi Izzat, Alkhawaldeh Rami S, Al-Ahmad Bilal
2022-Dec-25
COVID‐19, gender of patients, global growth rate, predictive modeling, social distancing
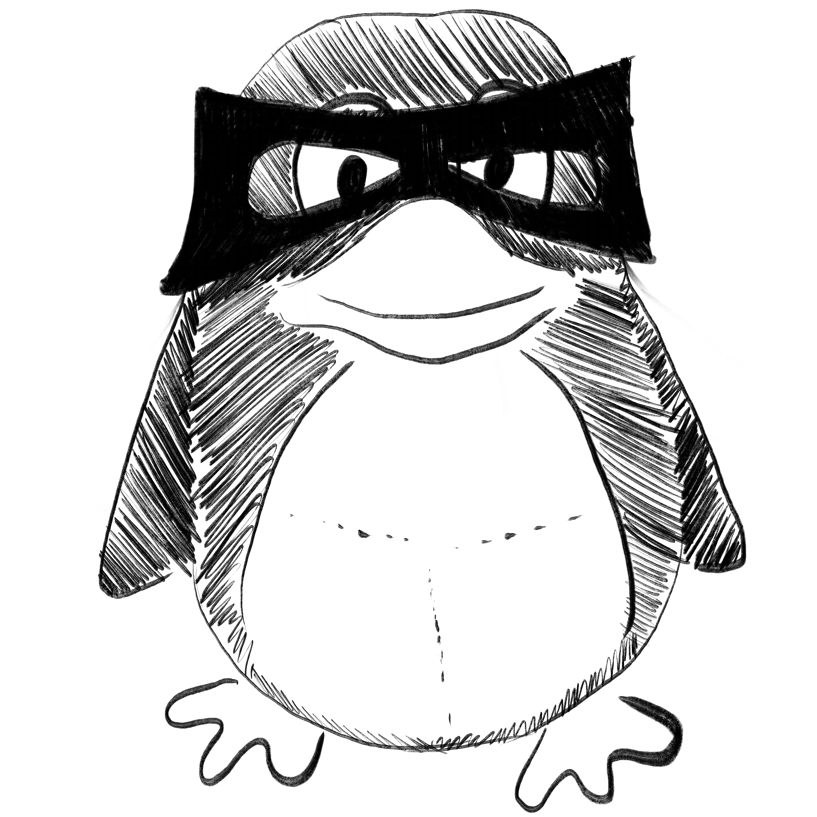
A face mask detection system: An approach to fight with COVID-19 scenario.
In Concurrency and computation : practice & experience
Jayaswal Ruchi, Dixit Manish
2022-Dec-25
3D‐face masks, CL‐SSDXcept, COVID‐19, DNN models, face mask detection, hyperparameters, optimizers
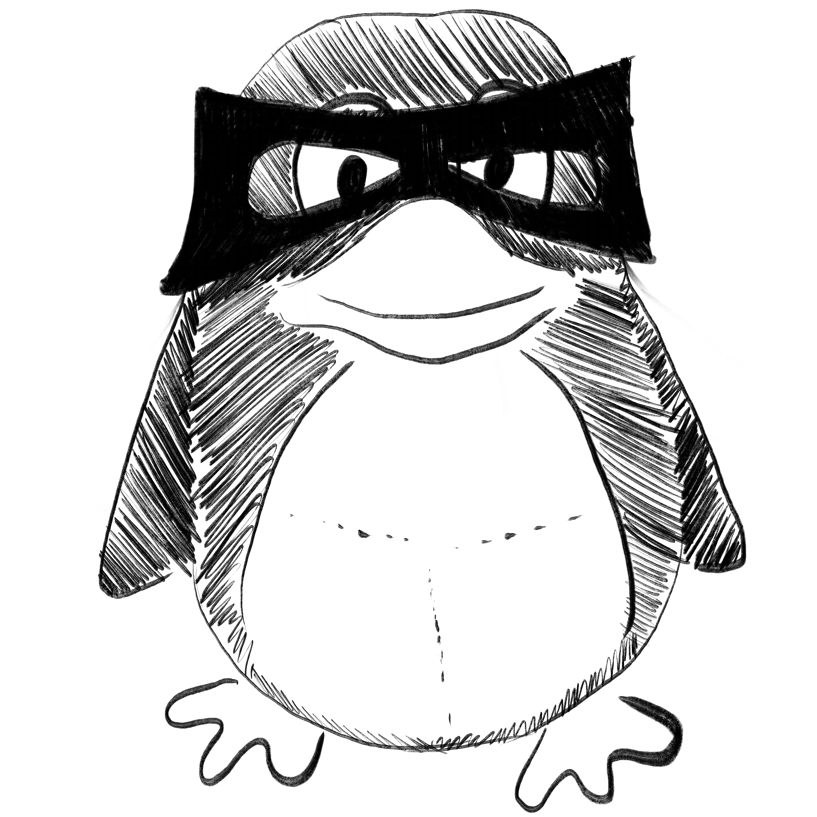
Rationing scarce healthcare capacity: A study of the ventilator allocation guidelines during the COVID-19 pandemic.
In Production and operations management
Anderson David R, Aydinliyim Tolga, Bjarnadóttir Margrét V, Çil Eren B, Anderson Michaela R
2023-Jan-22
COVID‐19, fairness, machine learning, multiclass queueing with abandonments, priority scheduling, resource allocation, scarce ventilator capacity
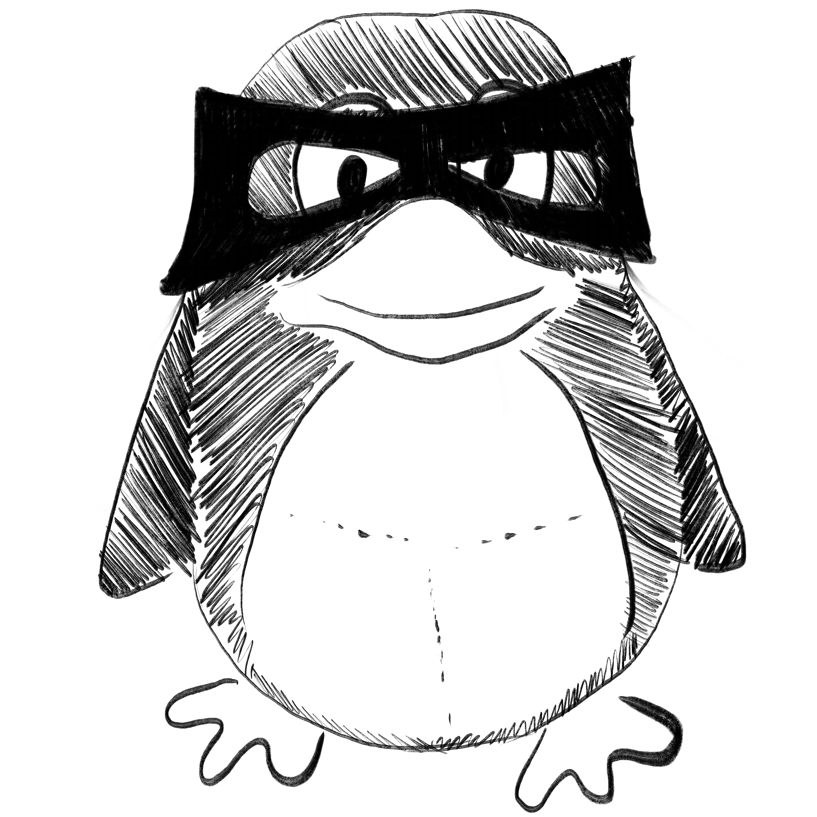
Federated learning based Covid-19 detection.
In Expert systems
Chowdhury Deepraj, Banerjee Soham, Sannigrahi Madhushree, Chakraborty Arka, Das Anik, Dey Ajoy, Dwivedi Ashutosh Dhar
2022-Nov-02
COVID‐19, CXR images, Internet of Medical Things (IoMT), Xception, cybersecurity, federated learning, privacy, transfer learning
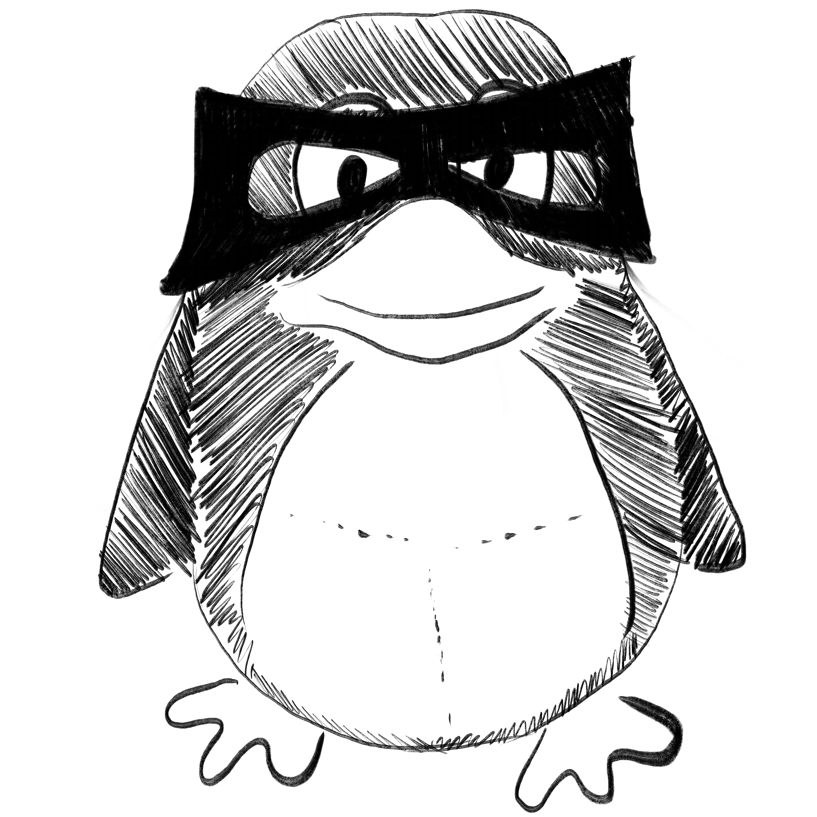
The RW3D: A multi-modal panel dataset to understand the psychological impact of the pandemic
ArXiv Preprint
Isabelle van der Vegt, Bennett Kleinberg
2023-02-01
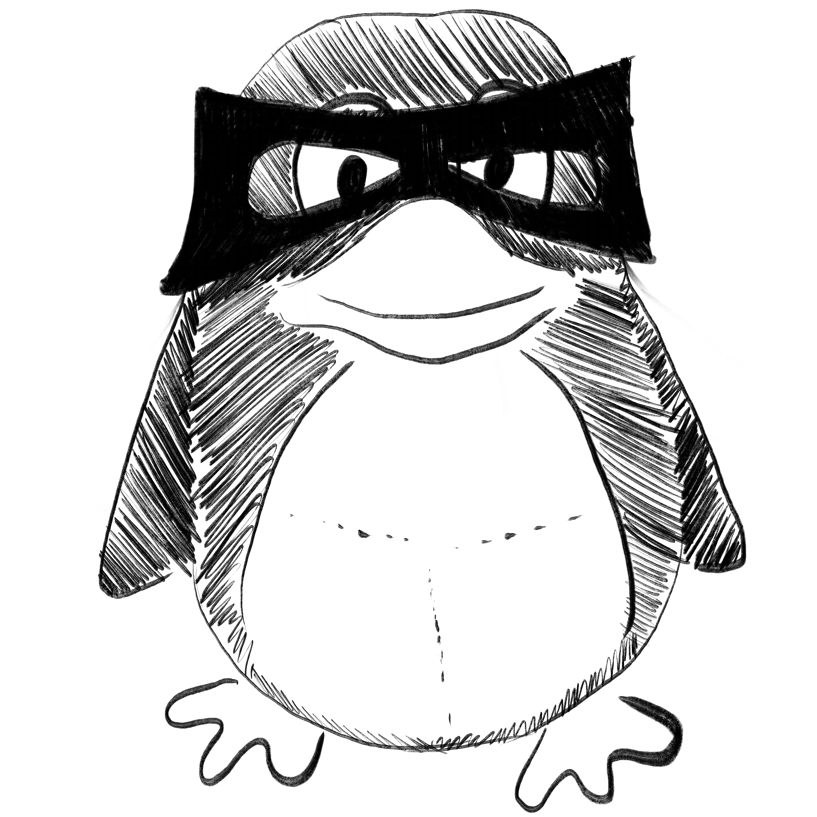
Dissociating COVID-19 from other respiratory infections based on acoustic, motor coordination, and phonemic patterns.
In Scientific reports ; h5-index 158.0
Talkar Tanya, Low Daniel M, Simpkin Andrew J, Ghosh Satrajit, O’Keeffe Derek T, Quatieri Thomas F
2023-Jan-28
Longitudinal changes in global structural brain connectivity and cognitive performance in former hospitalized COVID-19 survivors: an exploratory study.
In Experimental brain research
BACKGROUND :
OBJECTIVES :
METHODS :
RESULTS :
CONCLUSION :
Tassignon B, Radwan A, Blommaert J, Stas L, Allard S D, De Ridder F, De Waele E, Bulnes L C, Hoornaert N, Lacor P, Lathouwers E, Mertens R, Naeyaert M, Raeymaekers H, Seyler L, Van Binst A M, Van Imschoot L, Van Liedekerke L, Van Schependom J, Van Schuerbeek P, Vandekerckhove M, Meeusen R, Sunaert S, Nagels G, De Mey J, De Pauw K
2023-Jan-28
Magnetic resonance imaging, Recovery, SARS-CoV-2
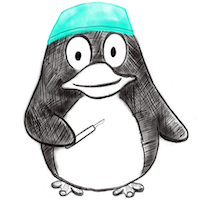
Virtual screening and molecular dynamics simulations provide insight into repurposing drugs against SARS-CoV-2 variants Spike protein/ACE2 interface.
In Scientific reports ; h5-index 158.0
Pirolli Davide, Righino Benedetta, Camponeschi Chiara, Ria Francesco, Di Sante Gabriele, De Rosa Maria Cristina
2023-Jan-27
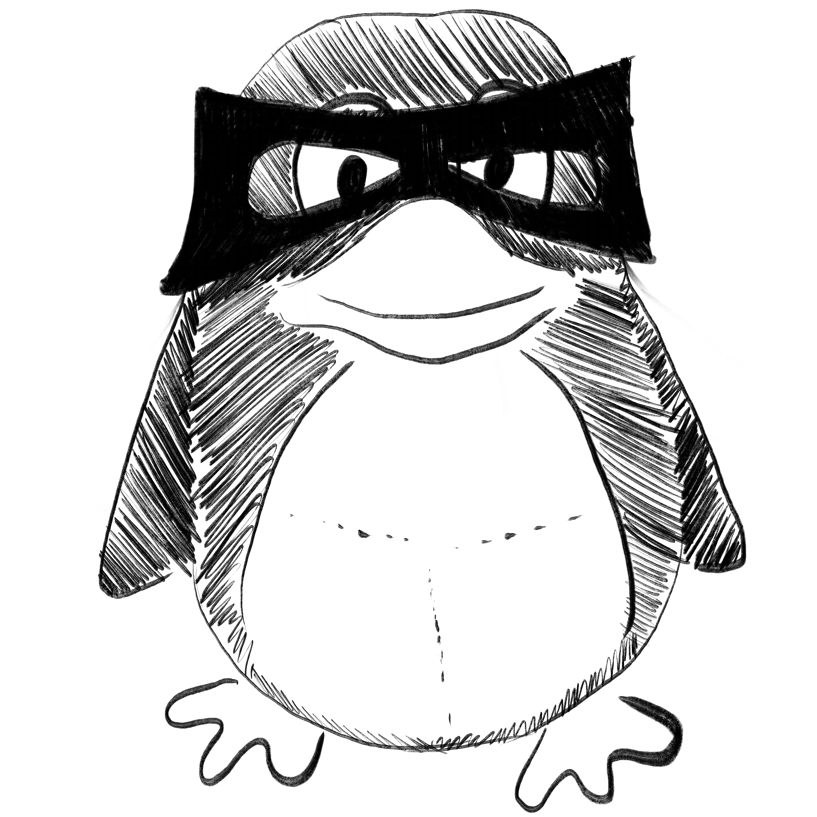
A survey of machine learning-based methods for COVID-19 medical image analysis.
In Medical & biological engineering & computing ; h5-index 32.0
Sailunaz Kashfia, Özyer Tansel, Rokne Jon, Alhajj Reda
2023-Jan-28
COVID-19, Computer tomography, Deep learning, Machine learning, Medical image analysis, Transfer learning
Development of an Artificial Intelligence-Guided Citizen-Centric Predictive Model for the Uptake of Maternal Health Services Among Pregnant Women Living in Urban Slum Settings in India: Protocol for a Cross-sectional Study With a Mixed Methods Design.
In JMIR research protocols ; h5-index 26.0
BACKGROUND :
OBJECTIVE :
METHODS :
RESULTS :
CONCLUSIONS :
INTERNATIONAL REGISTERED REPORT IDENTIFIER (IRRID) :
Shrivastava Rahul, Singhal Manmohan, Gupta Mansi, Joshi Ashish
2023-Jan-27
artificial intelligence, citizen centric, development, evaluation, informatics, machine learning, maternal health, predictive model
Application of artificial intelligence to the public health education.
In Frontiers in public health
Wang Xueyan, He Xiujing, Wei Jiawei, Liu Jianping, Li Yuanxi, Liu Xiaowei
2022
algorithm, artificial intelligence, big data, curriculum, education, public health
Entity and relation extraction from clinical case reports of COVID-19: a natural language processing approach.
In BMC medical informatics and decision making ; h5-index 38.0
BACKGROUND :
OBJECTIVE :
METHODS :
RESULTS :
CONCLUSIONS :
Raza Shaina, Schwartz Brian
2023-Jan-26
Artificial intelligence, COVID-19, Data cohort, Named entity, Natural language processing, Relation extraction, Transfer learning
Insights in paediatric virology during the COVID-19 era (Review).
In Medicine international
Mammas Ioannis N, Liston Maria, Koletsi Patra, Vitoratou Dimitra-Irinna, Koutsaftiki Chryssie, Papatheodoropoulou Alexia, Kornarou Helen, Theodoridou Maria, Kramvis Anna, Drysdale Simon B, Spandidos Demetrios A
2022
Institute of Paediatric Virology, ancient Mediterranean, artificial intelligence, coronavirus disease 2019, obesity, paediatric virology, severe acute respiratory syndrome coronavirus 2, viral infections, viral pandemics
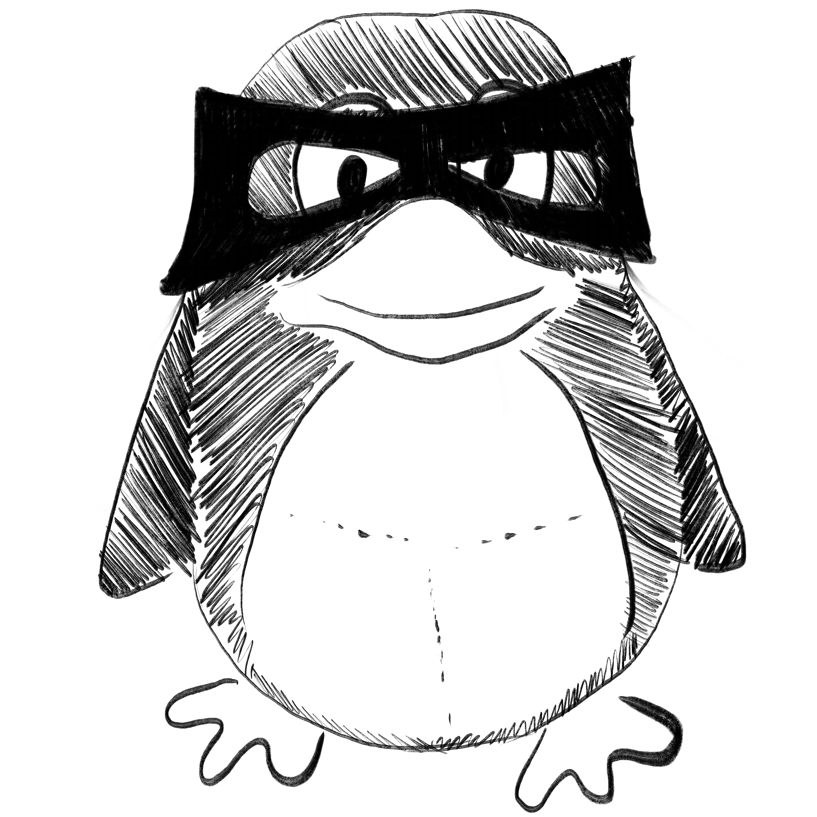
DeepMPF: deep learning framework for predicting drug-target interactions based on multi-modal representation with meta-path semantic analysis.
In Journal of translational medicine
BACKGROUND :
METHODS :
RESULTS :
CONCLUSIONS :
Ren Zhong-Hao, You Zhu-Hong, Zou Quan, Yu Chang-Qing, Ma Yan-Fang, Guan Yong-Jian, You Hai-Ru, Wang Xin-Fei, Pan Jie
2023-Jan-25
Drug–protein interactions, Joint learning, Meta-path, Multi-modal, Natural language processing, Sequence analysis
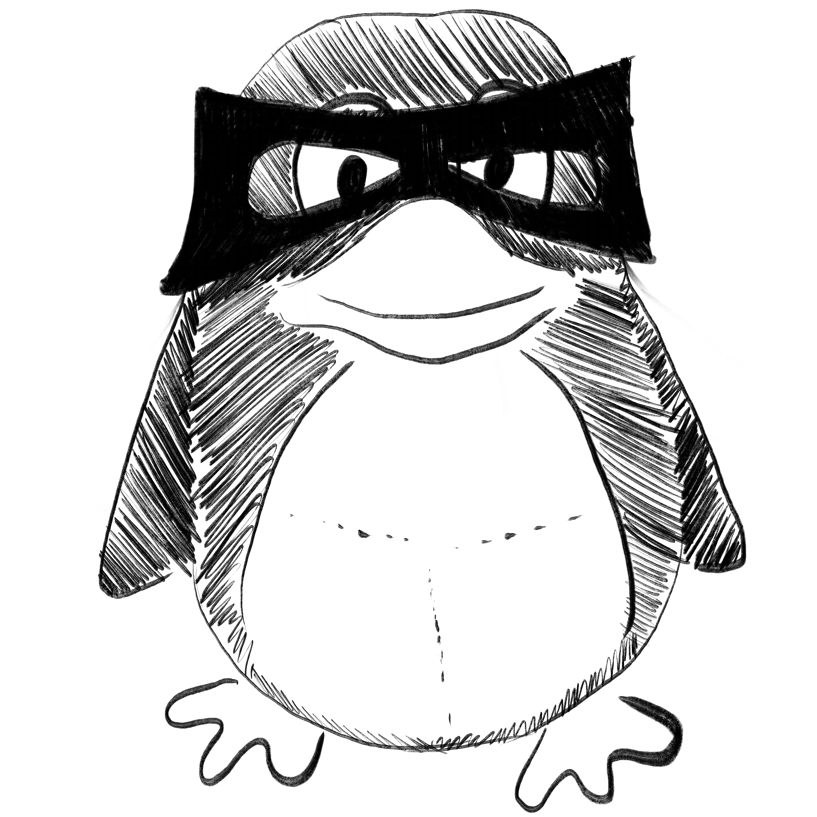
An open-source molecular builder and free energy preparation workflow.
In Communications chemistry
Bieniek Mateusz K, Cree Ben, Pirie Rachael, Horton Joshua T, Tatum Natalie J, Cole Daniel J
2022-Oct-27
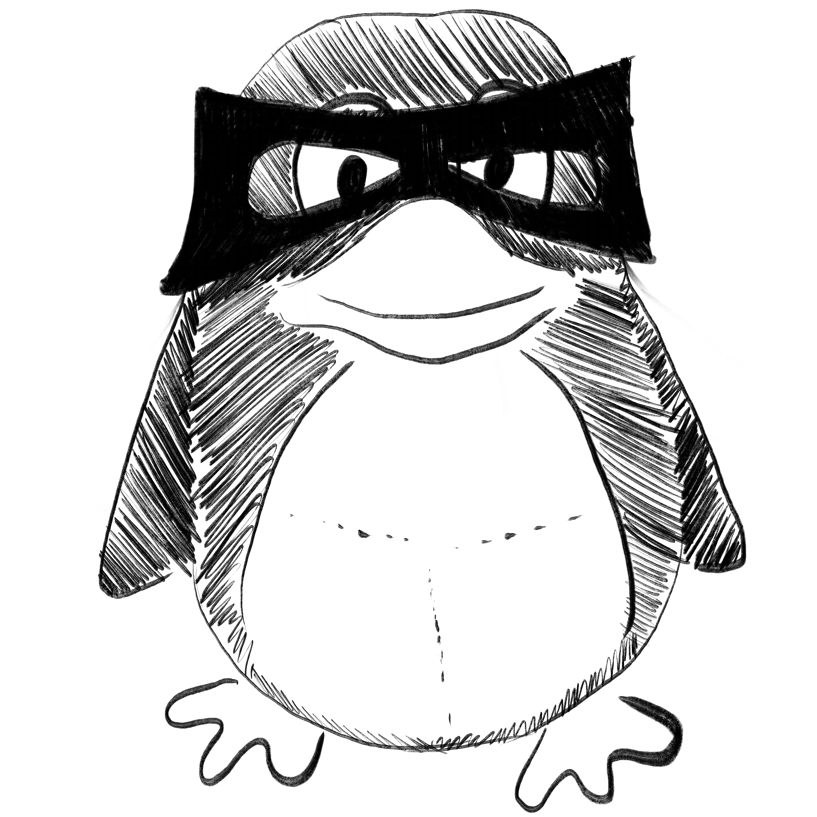
DeepGpgs: a novel deep learning framework for predicting arginine methylation sites combined with Gaussian prior and gated self-attention mechanism.
In Briefings in bioinformatics
Zhou Haiwei, Tan Wenxi, Shi Shaoping
2023-Jan-24
Gaussian prior, gated attention mechanism, methylation, residual network, weighted joint loss function
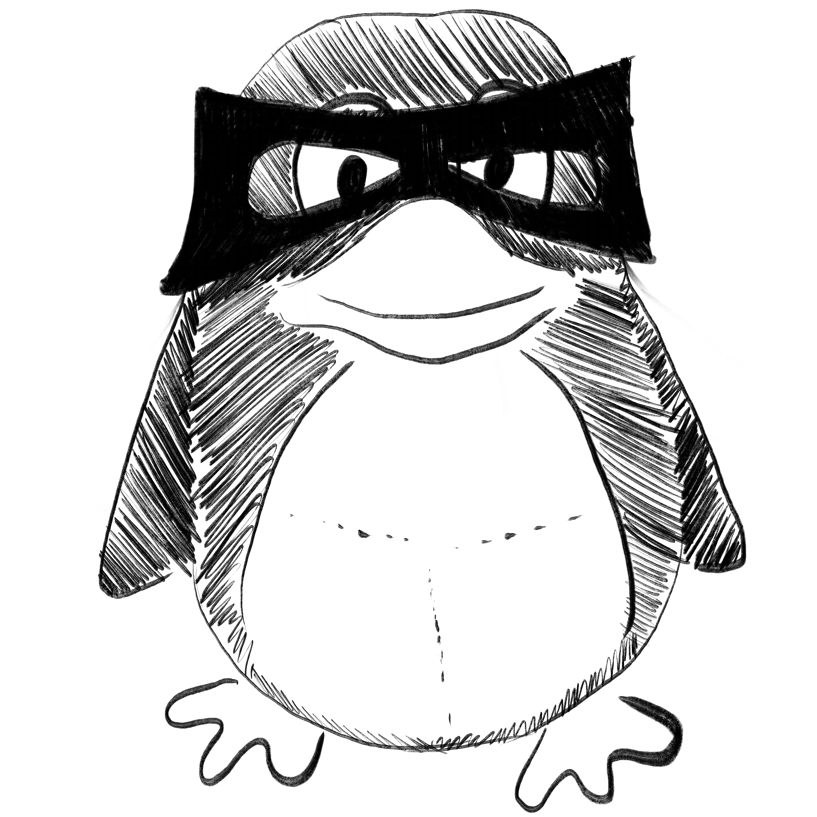
Executive protocol designed for new review study called: systematic review and artificial intelligence network meta-analysis (RAIN) with the first application for COVID-19.
In Biology methods & protocols
Salari Nader, Shohaimi Shamarina, Kiaei Aliakbar, Hosseinian-Far Amin, Mansouri Kamran, Ahmadi Arash, Mohammadi Masoud
2023
COVID-19, artificial intelligence, meta-analysis, network meta-analysis, protocol, systematic review
Conspiracy beliefs and COVID-19 guideline adherence in adolescent psychiatric outpatients: the predictive role of adverse childhood experiences.
In Child and adolescent psychiatry and mental health
BACKGROUND :
METHODS :
RESULTS :
CONCLUSION :
Goreis Andreas, Pfeffer Bettina, Zesch Heidi Elisabeth, Klinger Diana, Reiner Tamara, Bock Mercedes M, Ohmann Susanne, Sackl-Pammer Petra, Werneck-Rohrer Sonja, Eder Harald, Skala Katrin, Czernin Klara, Mairhofer Dunja, Rohringer Bernhard, Bedus Carolin, Lipp Ronja, Vesely Christine, Plener Paul L, Kothgassner Oswald D
2023-Jan-24
Adolescents, Adverse childhood experiences, COVID-19, Childhood Trauma, Conspiracy beliefs, Guideline adherence, Mental health
Generating simple classification rules to predict local surges in COVID-19 hospitalizations.
In Health care management science
Yaesoubi Reza, You Shiying, Xi Qin, Menzies Nicolas A, Tuite Ashleigh, Grad Yonatan H, Salomon Joshua A
2023-Jan-24
COVID-19, Decision tree, Machine learning, Prediction, Simulation, Surveillance
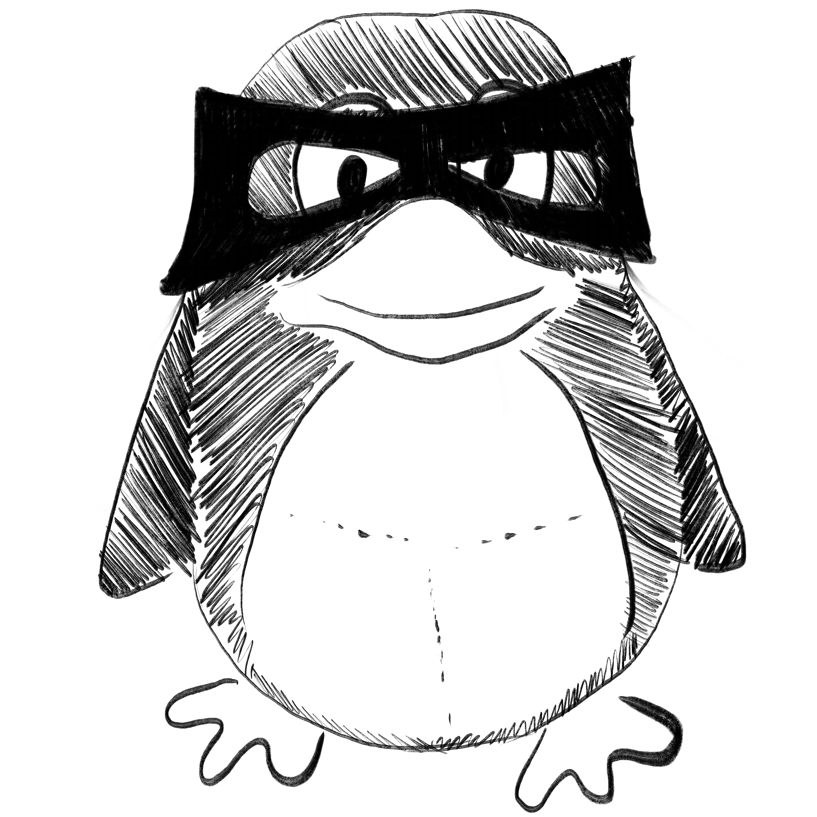
Deep learning identified genetic variants for COVID-19-related mortality among 28,097 affected cases in UK Biobank.
In Genetic epidemiology
Liu Zihuan, Dai Wei, Wang Shiying, Yao Yisha, Zhang Heping
2023-Jan-24
COVID-19, SARS-CoV-2, TAS2R1, UK Biobank, deep learning
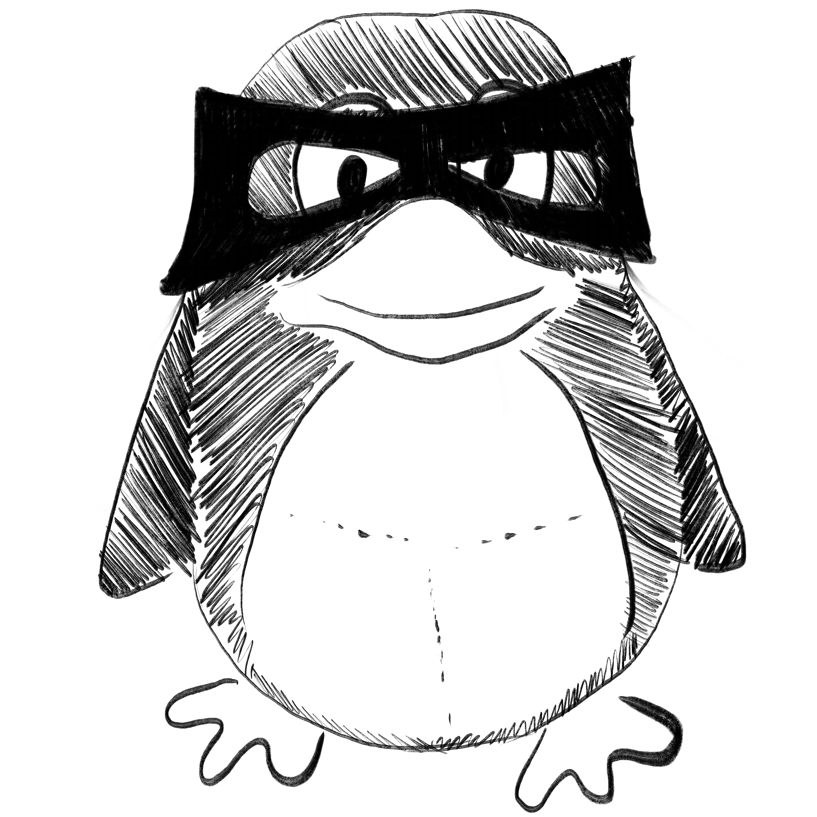
Deep learning approach to security enforcement in cloud workflow orchestration.
In Journal of cloud computing (Heidelberg, Germany)
El-Kassabi Hadeel T, Serhani Mohamed Adel, Masud Mohammad M, Shuaib Khaled, Khalil Khaled
2023
Anomaly detection, Cloud, Cloud workflow, Covid-19, Deep learning, Prediction, Security enforcement
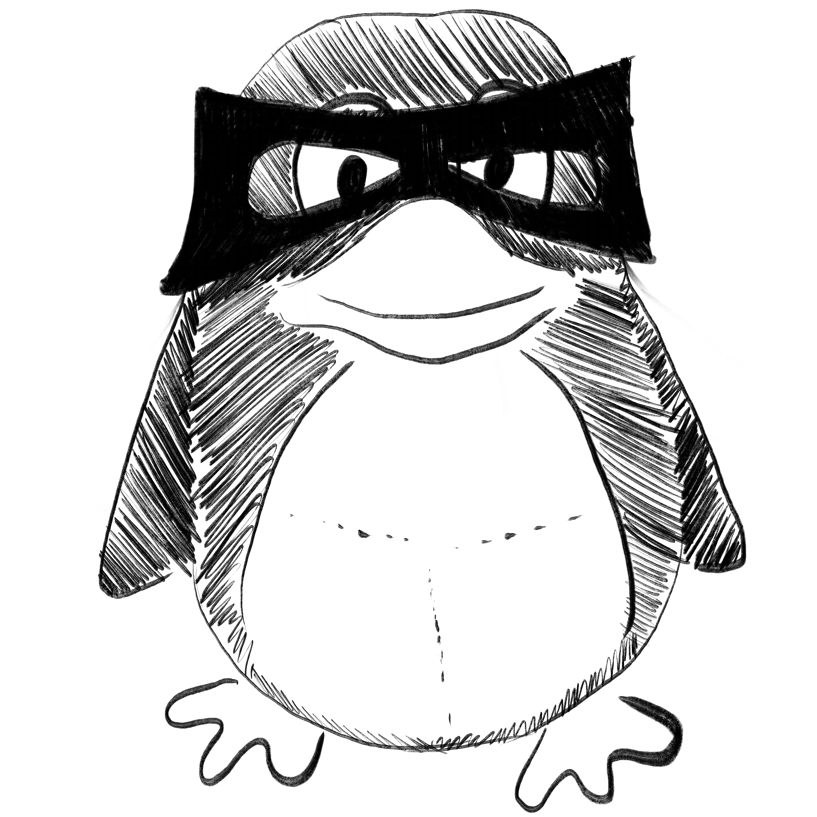
Protein-ligand binding affinity prediction with edge awareness and supervised attention.
In iScience
Gu Yuliang, Zhang Xiangzhou, Xu Anqi, Chen Weiqi, Liu Kang, Wu Lijuan, Mo Shenglong, Hu Yong, Liu Mei, Luo Qichao
2023-Jan-20
Biocomputational method, Classification of proteins, Molecular interaction
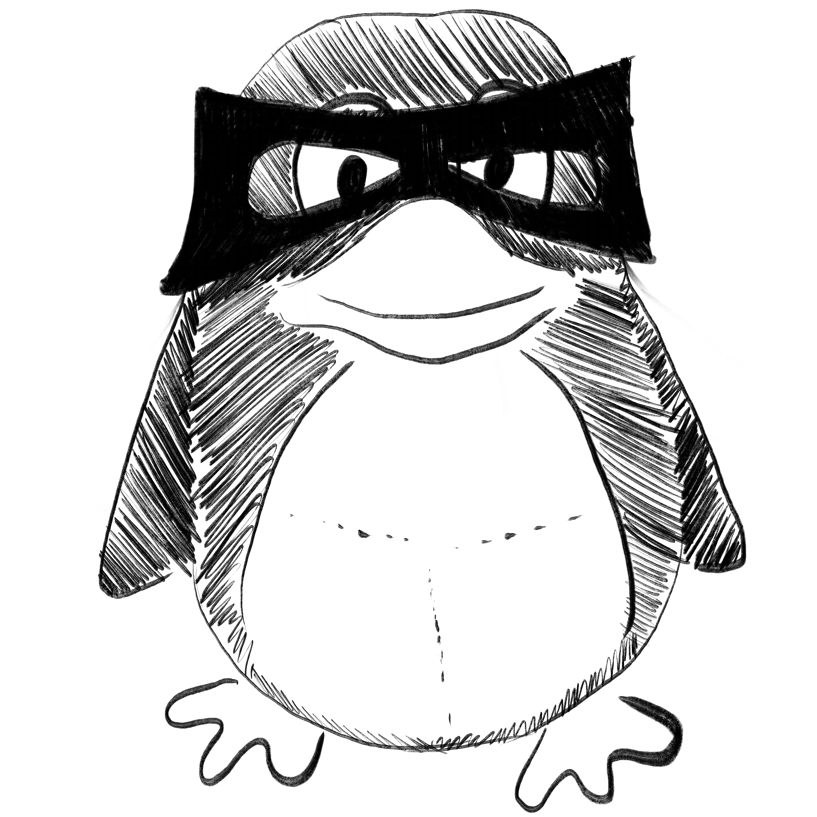
Estimation of the unemployment rate in Turkey: A comparison of the ARIMA and machine learning models including Covid-19 pandemic periods.
In Heliyon
Yamacli Dilek Surekci, Yamacli Serhan
2023-Jan
ANN, ARIMA, Estimation, Unemployment rate
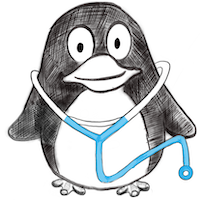
Statistical Analysis of Mortality Rates of Coronavirus Disease 2019 (COVID-19) Patients in Japan Across the 4C Mortality Score Risk Groups, Age Groups, and Epidemiological Waves: A Report From the Nationwide COVID-19 Cohort.
In Open forum infectious diseases
BACKGROUND :
METHODS :
RESULTS :
CONCLUSIONS :
Baba Hiroaki, Ikumi Saori, Aoyama Shotaro, Ishikawa Tetsuo, Asai Yusuke, Matsunaga Nobuaki, Ohmagari Norio, Kanamori Hajime, Tokuda Koichi, Ueda Takuya, Kawakami Eiryo
2023-Jan
4C mortality score, COVID-19, Japan, elderly, epidemic wave
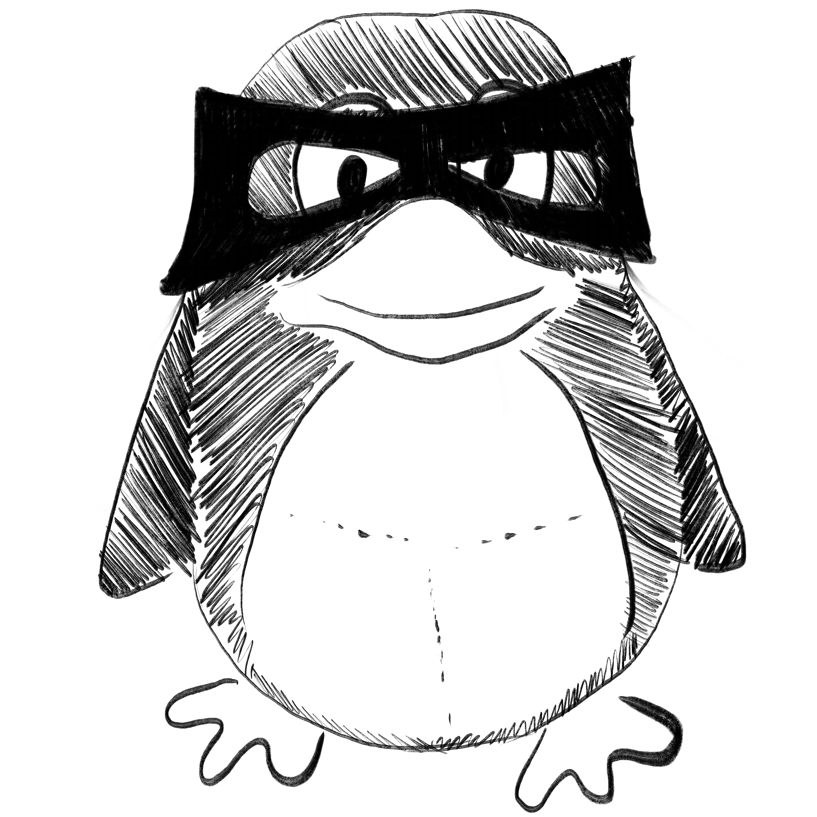
CovidExpert: A Triplet Siamese Neural Network framework for the detection of COVID-19.
In Informatics in medicine unlocked
Ornob Tareque Rahman, Roy Gourab, Hassan Enamul
2023
COVID-19 diagnosis, CT scan images, Ensemble CNN, Few-shot learning, Triplet siamese network
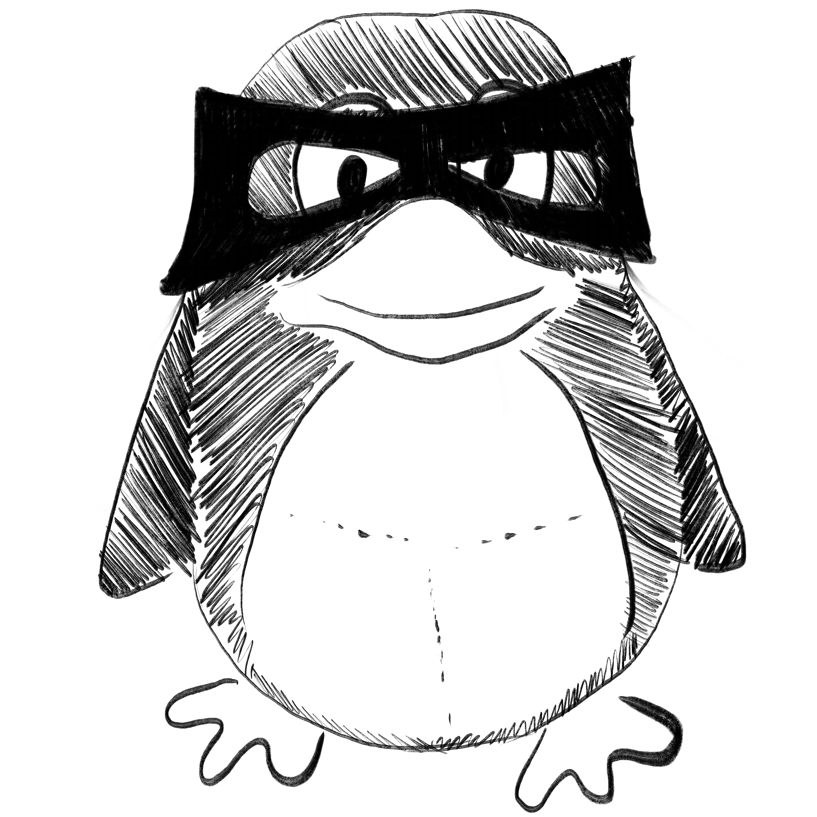
ELUCNN for explainable COVID-19 diagnosis.
In Soft computing
Wang Shui-Hua, Satapathy Suresh Chandra, Xie Man-Xia, Zhang Yu-Dong
2023-Jan-13
COVID-19, Cloud computing, Convolutional neural network, Cross validation, Deep learning, Exponential linear unit, Mobile app, Multiple-way data augmentation, SARS-CoV-2
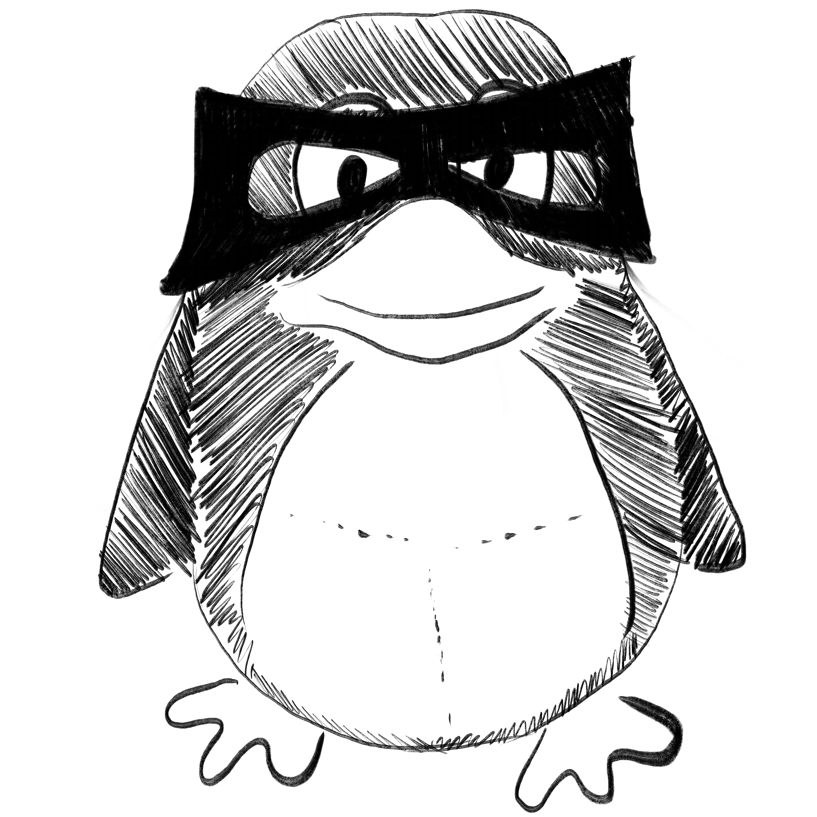
COVID-19-The Role of Artificial Intelligence, Machine Learning, and Deep Learning: A Newfangled.
In Archives of computational methods in engineering : state of the art reviews
Vinod Dasari Naga, Prabaharan S R S
2023-Jan-17
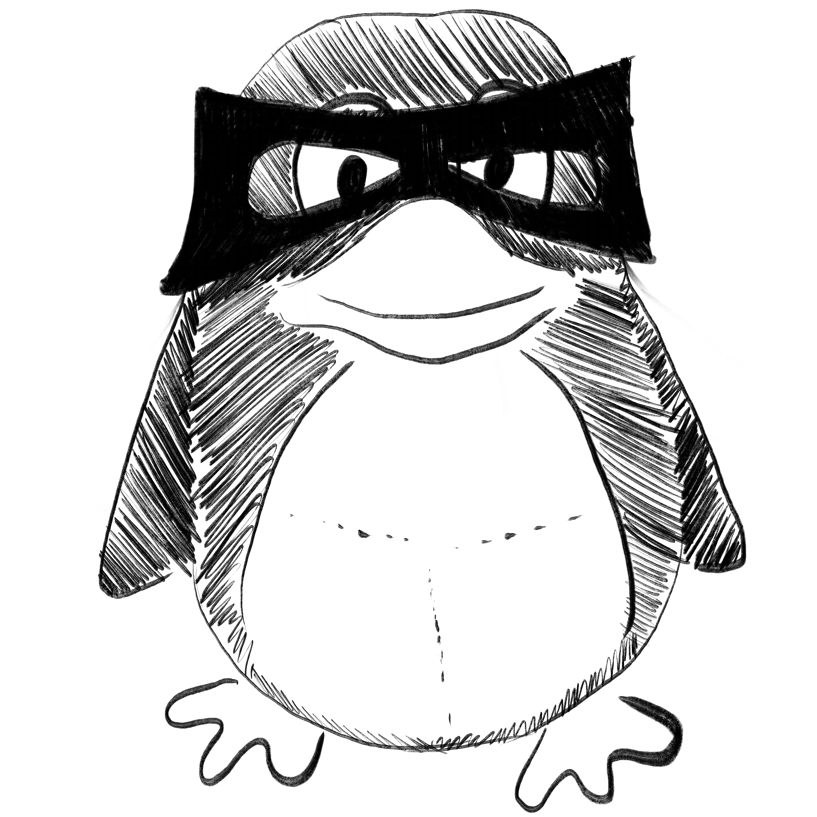
Development of a Fast Fourier Transform-based Analytical Method for COVID-19 Diagnosis from Chest X-Ray Images Using GNU Octave.
In Journal of medical physics
PURPOSE :
MATERIALS AND METHODS :
RESULTS :
CONCLUSION :
Majumder Durjoy
2022
COVID-19, Chest X-ray image, Fourier analysis, image analysis, pneumonia
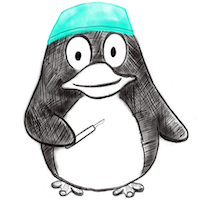
Machine learning-based integration develops biomarkers initial the crosstalk between inflammation and immune in acute myocardial infarction patients.
In Frontiers in cardiovascular medicine
Li Hongyu, Sun Xinti, Li Zesheng, Zhao Ruiping, Li Meng, Hu Taohong
2022
acute myocardial infarction, bioinformatics, immune infiltration, machine learning, prognosis
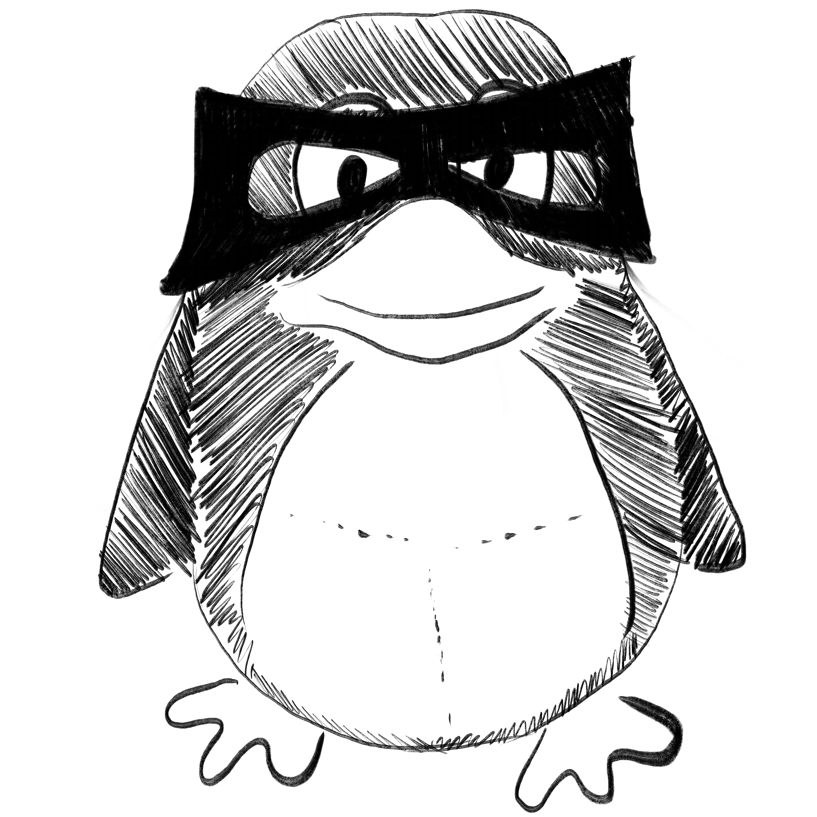
Analysis of individual characteristics influencing user polarization in COVID-19 vaccine hesitancy.
In Computers in human behavior ; h5-index 125.0
Xie Lei, Wang Dandan, Ma Feicheng
2023-Jan-17
Big five model of personality, COVID-19 pandemic, Curiosity-drive theory, User polarization, Vaccine hesitancy
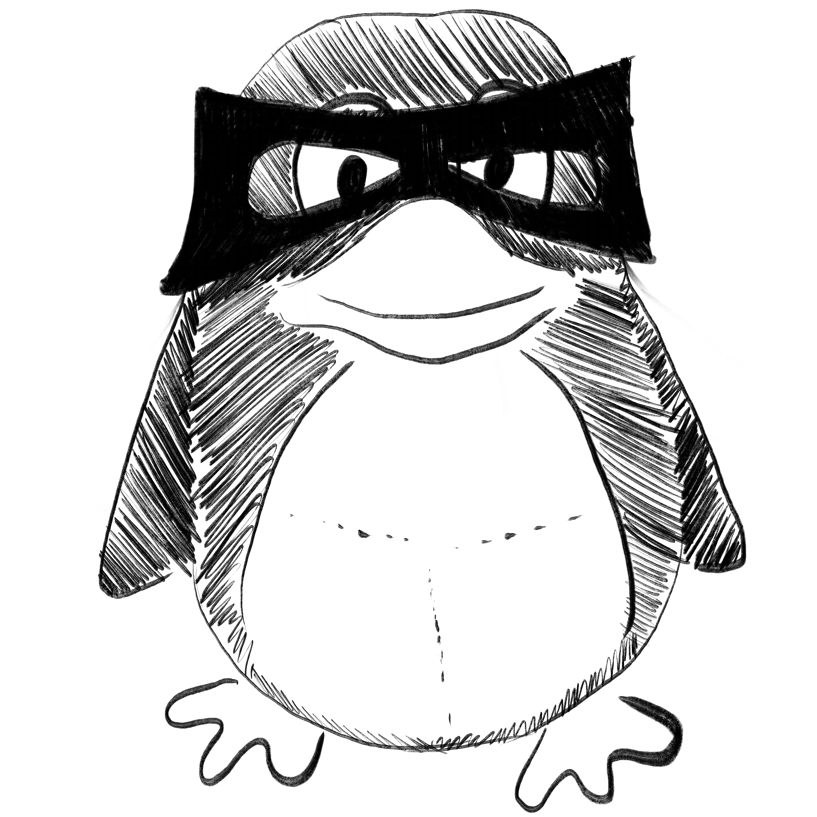
Safety, Tolerability and Pharmacokinetics of Half-Life Extended SARS-CoV-2 Neutralizing Monoclonal Antibodies AZD7442 (Tixagevimab/Cilgavimab) in Healthy Adults.
In The Journal of infectious diseases ; h5-index 82.0
BACKGROUND :
METHODS :
RESULTS :
CONCLUSIONS :
CLINICAL TRIALS REGISTRATION :
Forte-Soto Pablo, Albayaty Muna, Brooks Dennis, Arends Rosalinda H, Tillinghast John, Aksyuk Anastasia A, Bouquet Jerome, Chen Cecil, Gebre Asfiha, Kubiak Robert J, Pilla Reddy Venkatesh, Seegobin Seth, Streicher Katie, Templeton Alison, Esser Mark T
2023-Jan-23
COVID-19, SARS-CoV-2, monoclonal antibody, pharmacokinetics, phase 1, safety, tolerability
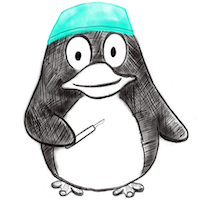
Essential elements of weight loss apps for a multi-ethnic population with high BMI: a qualitative study with practical recommendations.
In Translational behavioral medicine
Chew Han Shi Jocelyn, Lim Su Lin, Kim Guowei, Kayambu Geetha, So Bok Yan Jimmy, Shabbir Asim, Gao Yujia
2023-Jan-23
App, BMI, Behavior, Perceptions, Weight management, mHealth
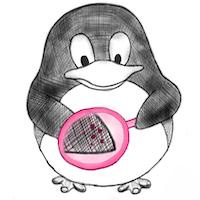
The role of mobile teledermoscopy in skin cancer triage and management during the COVID-19 pandemic.
In Indian journal of dermatology, venereology and leprology
Lee Claudia, Witkowski Alexander, Żychowska Magdalena, Ludzik Joanna
2022-Dec-08
COVID-19, Dermoscopy, melanoma, skin cancer, smartphone, teledermoscopy
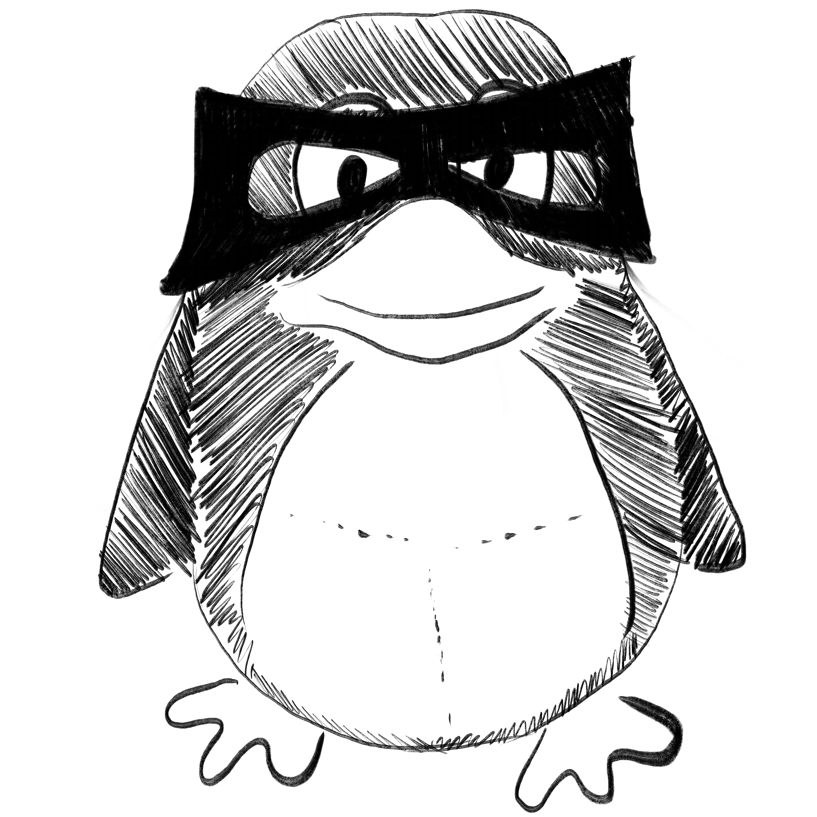
A framework for designing AI systems that support community wellbeing.
In Frontiers in psychology ; h5-index 92.0
INTRODUCTION :
METHODS :
RESULTS :
DISCUSSION :
van der Maden Willem, Lomas Derek, Hekkert Paul
2022
artificial intelligence, community wellbeing, cybernetics, feedback loop, human values, human-centered design, wellbeing economy
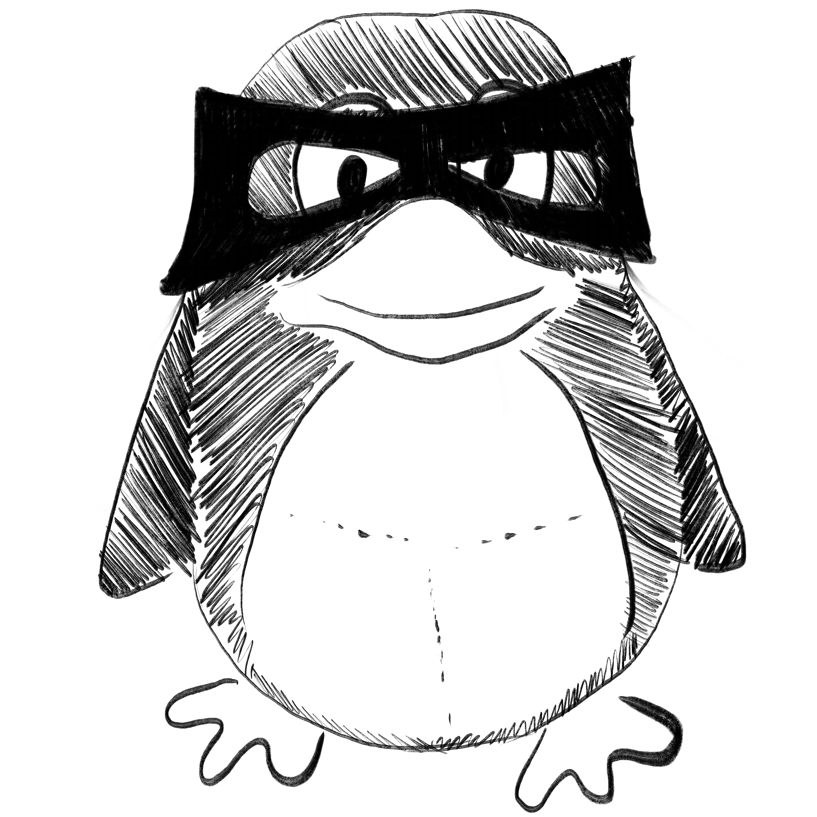
An Artificial Intelligence-as-a-Service Architecture for deep learning model embodiment on low-cost devices: A case study of COVID-19 diagnosis.
In Applied soft computing
Rodrigues Moreira Larissa Ferreira, Moreira Rodrigo, Travençolo Bruno Augusto Nassif, Backes André Ricardo
2023-Feb
Artificial intelligence, COVID-19, Convolutional neural network, Embedded, Low-cost device
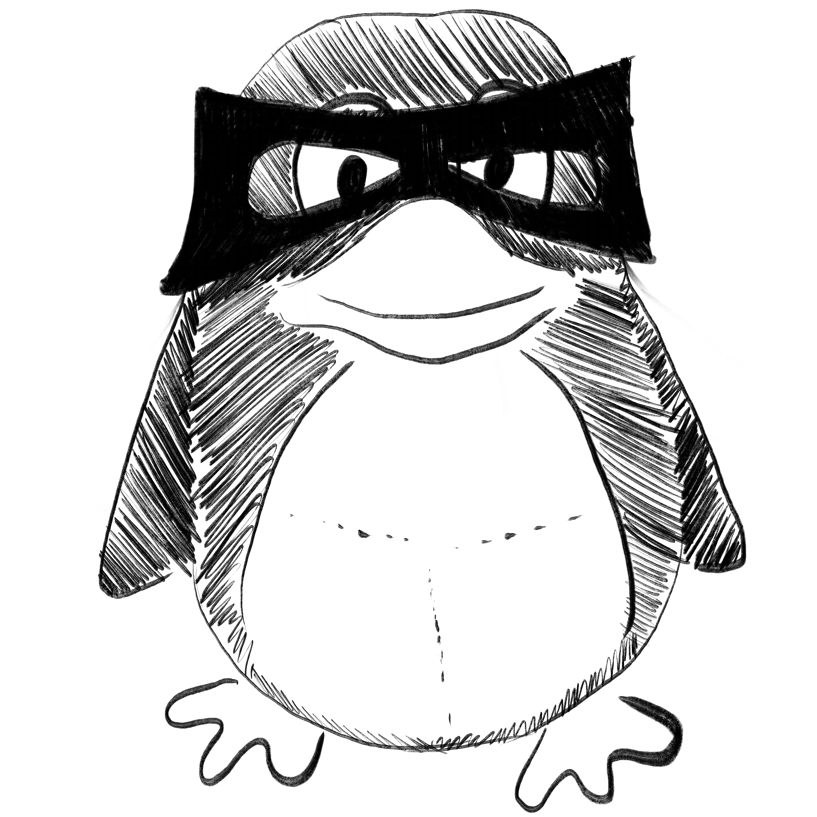
Deep Learning-Assisted Droplet Digital PCR for Quantitative Detection of Human Coronavirus.
In Biochip journal
Lee Young Suh, Choi Ji Wook, Kang Taewook, Chung Bong Geun
2023-Jan-17
Deep learning, GMM clustering, Image processing, Mask R-CNN, ddPCR
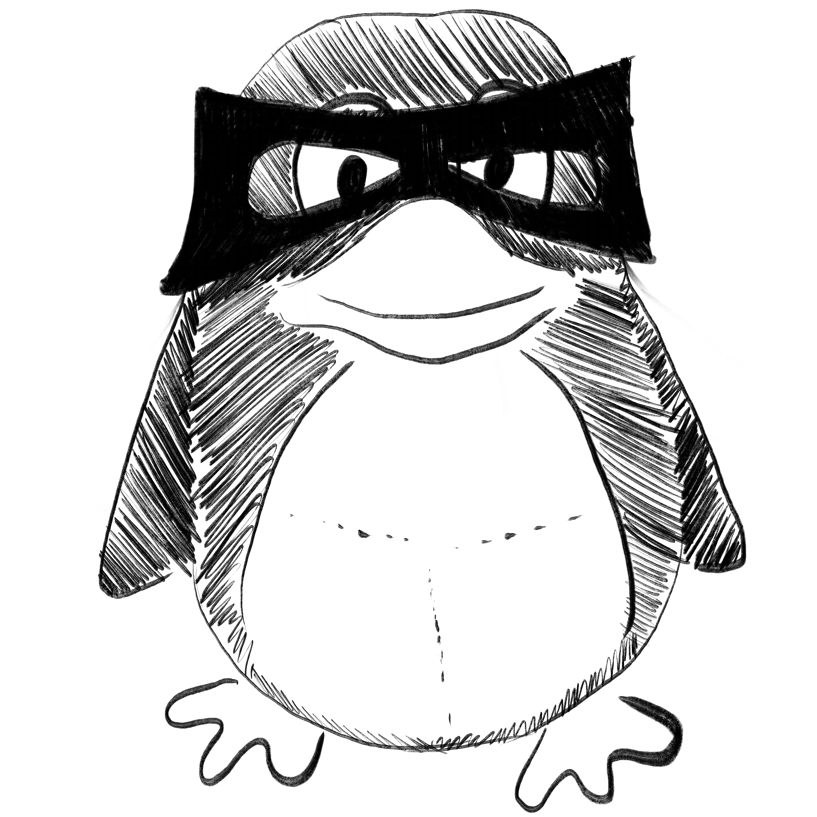
Machine learning sentiment analysis, COVID-19 news and stock market reactions.
In Research in international business and finance
Costola Michele, Hinz Oliver, Nofer Michael, Pelizzon Loriana
2023-Jan
COVID-19 news, Sentiment analysis, Stock markets
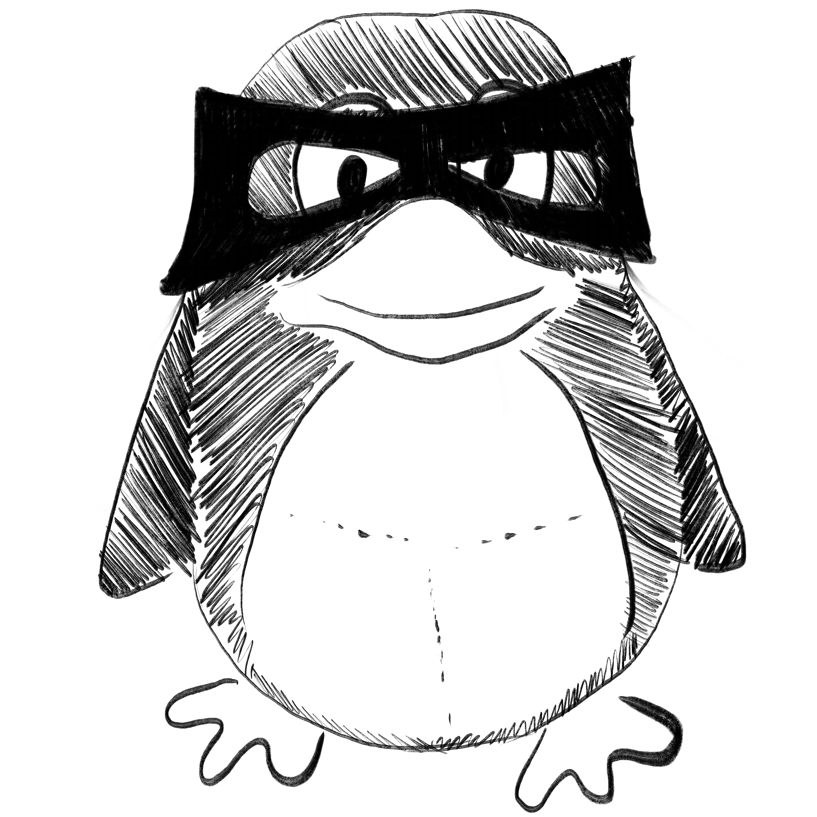
A review of advanced technologies available to improve the healthcare performance during COVID-19 pandemic.
In Procedia computer science
Ali Omar, AlAhmad Ahmad, Kahtan Hasan
2023
Artificial Intelligence, COVID-19, Cloud Computing, Healthcare, Informatization, Internet of Things
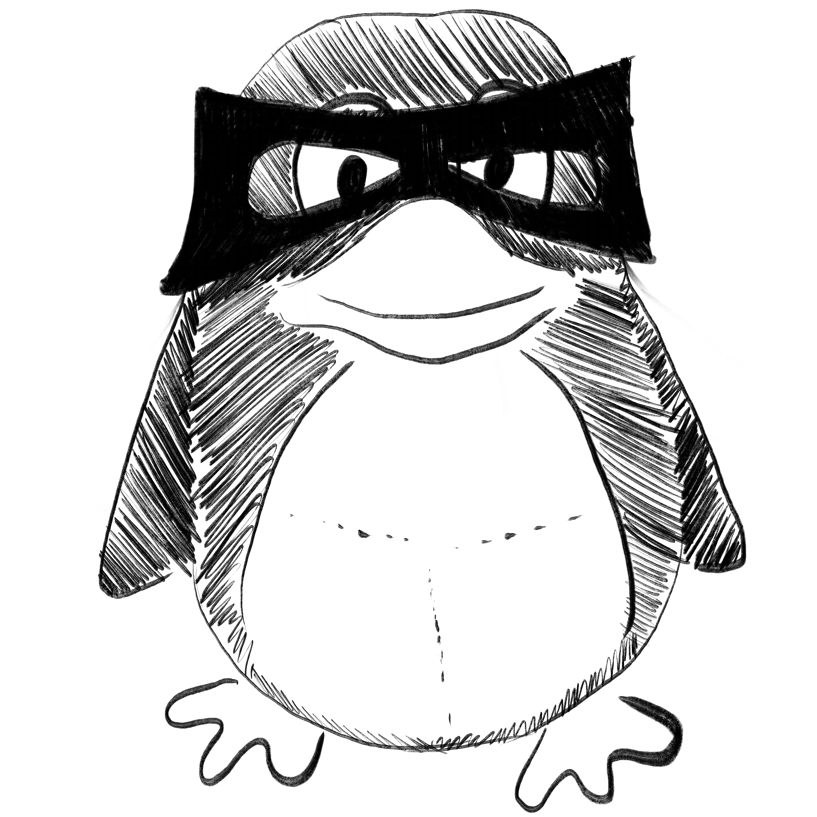
Towards precision medicine: Omics approach for COVID-19.
In Biosafety and health
Cen Xiaoping, Wang Fengao, Huang Xinhe, Jovic Dragomirka, Dubee Fred, Yang Huanming, Li Yixue
2023-Jan-18
COVID-19, artificial intelligence, multi-omics, precision medicine
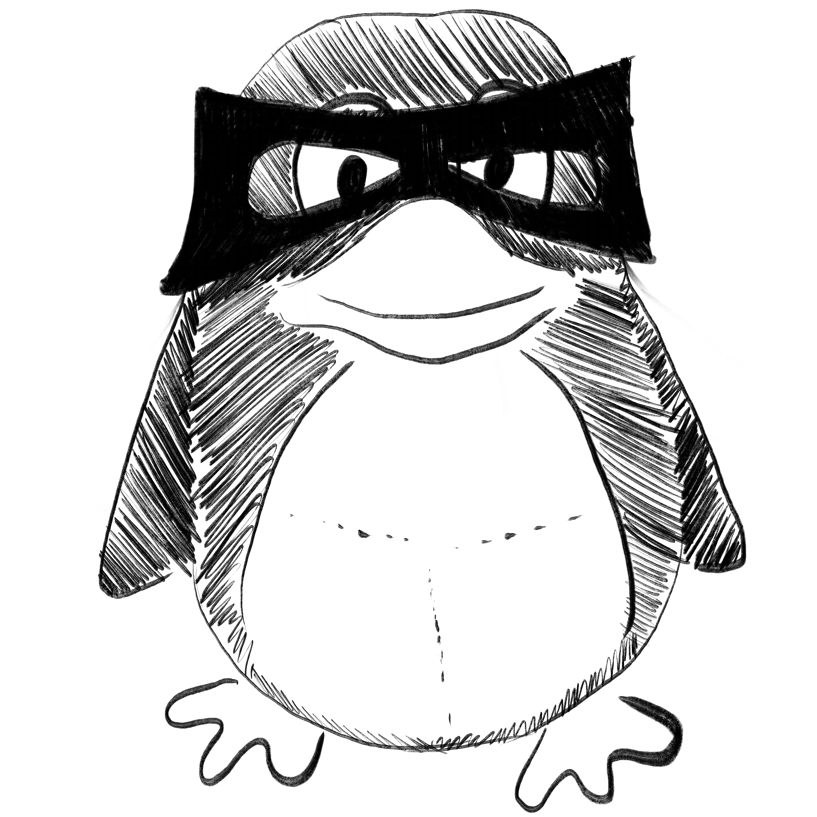
Speech phoneme and spectral smearing based non-invasive COVID-19 detection.
In Frontiers in artificial intelligence
Mishra Soumya, Dash Tusar Kanti, Panda Ganapati
2022
COVID-19, COVID-19 detection, machine learning, phoneme analysis, spectral smearing
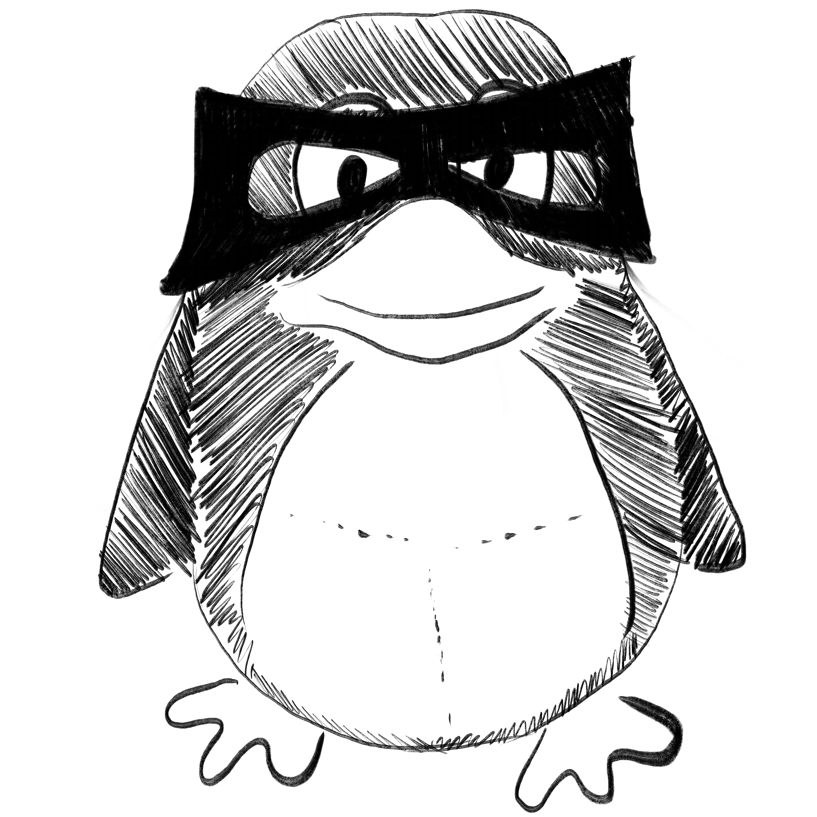
Curious thing, an artificial intelligence (AI)-based conversational agent for COVID-19 patient management.
In Australian journal of primary health ; h5-index 18.0
Chow Josephine Sau Fan, Blight Victoria, Brown Marian, Glynn Vanessa, Lane Brian, Larkin Amanda, Marshall Sonia, Matthews Prue, Rowles Mick, Warner Bradley
2023-Jan-23
Development and validation of a multivariable risk factor questionnaire to detect oesophageal cancer in 2-week wait patients.
In Clinics and research in hepatology and gastroenterology
INTRODUCTION :
METHODS :
RESULTS :
CONCLUSIONS :
Ho Kai Man Alexander, Rosenfeld Avi, Hogan Áine, McBain Hazel, Duku Margaret, Wolfson Paul Bd, Wilson Ashley, Cheung Sharon My, Hennelly Laura, Macabodbod Lester, Graham David G, Sehgal Vinay, Banerjee Amitava, Lovat Laurence B
2023-Jan-17
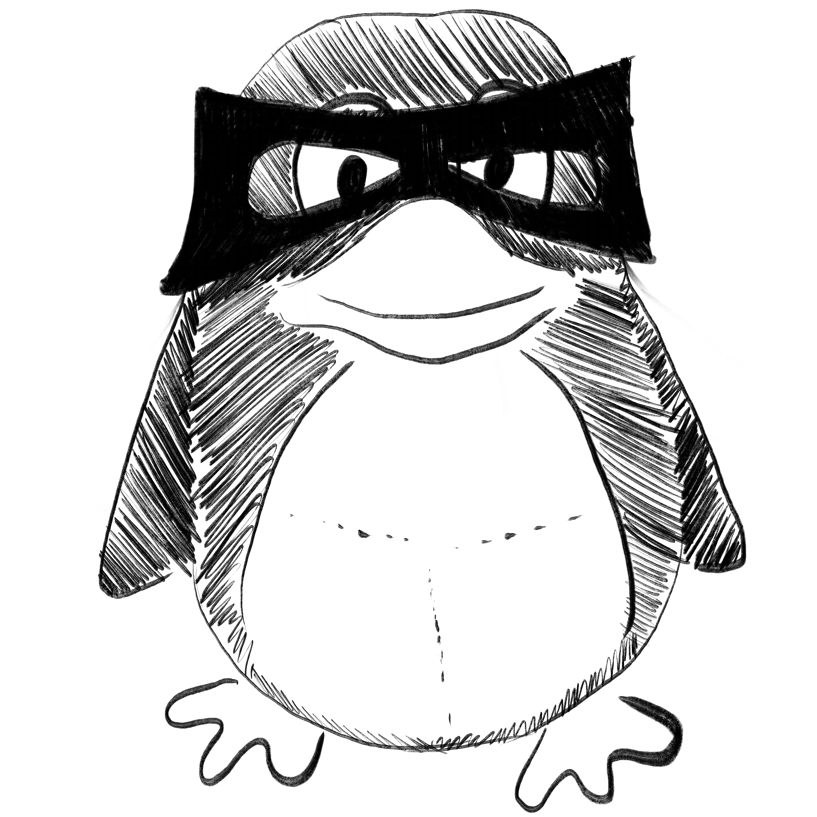
Mosaic RBD nanoparticles induce intergenus cross-reactive antibodies and protect against SARS-CoV-2 challenge.
In Proceedings of the National Academy of Sciences of the United States of America
Lee Dan Bi, Kim Hyojin, Jeong Ju Hwan, Jang Ui Soon, Jang Yuyeon, Roh Seokbeom, Jeon Hyunbum, Kim Eun Jeong, Han Su Yeon, Maeng Jin Young, Magez Stefan, Radwanska Magdalena, Mun Ji Young, Jun Hyun Sik, Lee Gyudo, Song Min-Suk, Lee Hye-Ra, Chung Mi Sook, Baek Yun Hee, Kim Kyung Hyun
2023-Jan-24
SARS-CoV-2, immune response, mosaic multivalent antigens, receptor-binding domain, spike
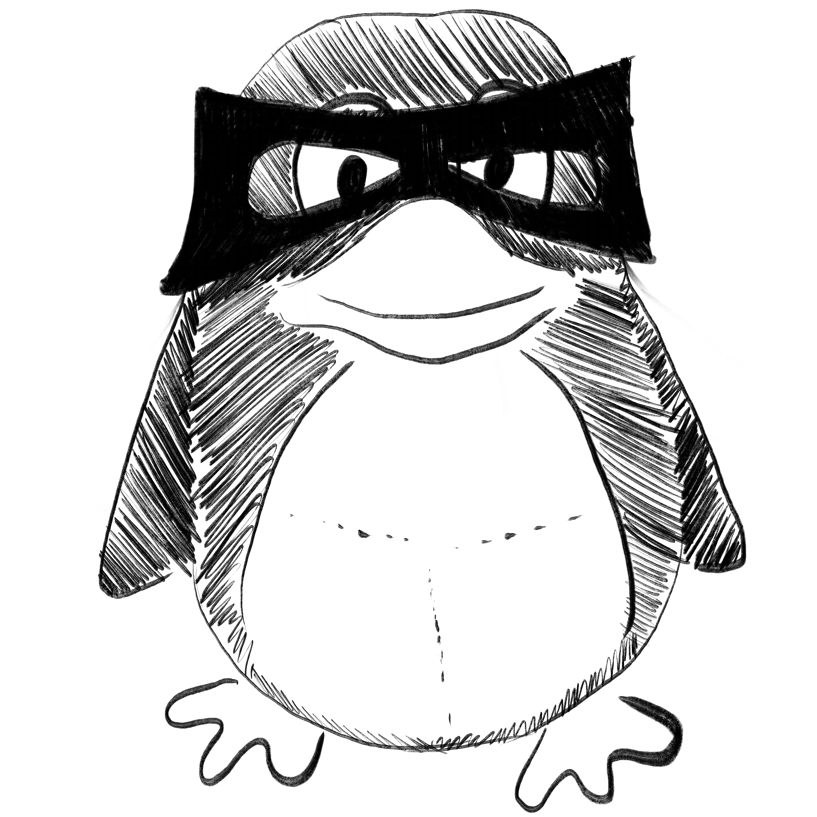
Multidimensional machine learning on 2173 COVID-19 patients in Vietnam: Retro-prospective Validation Study.
In JMIR formative research
BACKGROUND :
OBJECTIVE :
METHODS :
RESULTS :
CONCLUSIONS :
Nguyen Tue Trong, Ho Tu Cam, Bui Huong Thi Thu, Ho Lam Khanh, Ta Van Thanh
2023-Jan-18
Impact of the COVID-19 pandemic and corresponding control measures on long-term care facilities: a systematic review and meta-analysis.
In Age and ageing ; h5-index 55.0
BACKGROUND :
OBJECTIVE :
METHOD :
RESULTS :
CONCLUSION :
Zhang Jun, Yu Yushan, Petrovic Mirko, Pei Xiaomei, Tian Qing-Bao, Zhang Lei, Zhang Wei-Hong
2023-Jan-08
COVID-19, control measures, long-term care facilities, older people, systematic review
Long-term respiratory follow-up of ICU hospitalized COVID-19 patients: Prospective cohort study.
In PloS one ; h5-index 176.0
BACKGROUND :
METHODS :
RESULTS :
CONCLUSION :
Ribeiro Carvalho Carlos Roberto, Lamas Celina Almeida, Chate Rodrigo Caruso, Salge João Marcos, Sawamura Marcio Valente Yamada, de Albuquerque André L P, Toufen Junior Carlos, Lima Daniel Mario, Garcia Michelle Louvaes, Scudeller Paula Gobi, Nomura Cesar Higa, Gutierrez Marco Antonio, Baldi Bruno Guedes
2023
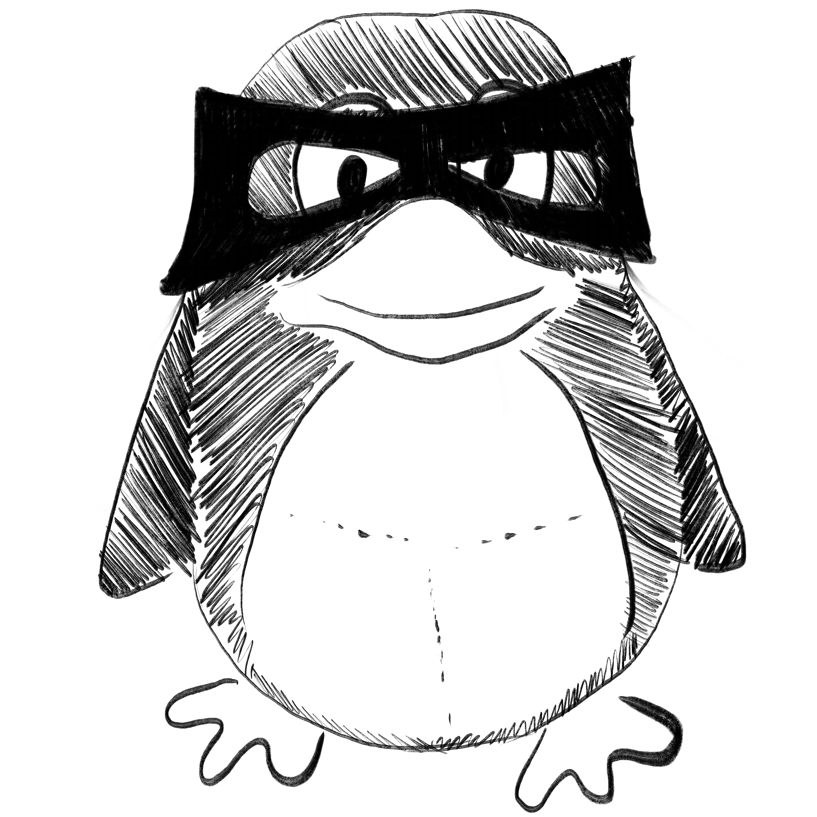
Sensing dynamic human activity zones using geo-tagged big data in Greater London, UK during the COVID-19 pandemic.
In PloS one ; h5-index 176.0
Chen Tongxin, Zhu Di, Cheng Tao, Gao Xiaowei, Chen Huanfa
2023
COVID-19's influence on cardiac function: a machine learning perspective on ECG analysis.
In Medical & biological engineering & computing ; h5-index 32.0
Gomes Juliana Carneiro, de Santana Maíra Araújo, Masood Aras Ismael, de Lima Clarisse Lins, Dos Santos Wellington Pinheiro
2023-Jan-20
COVID-19 clinical diagnosis, COVID-19 computer-aided diagnosis, Deep learning, Electrocardiography, Hybrid deep architectures
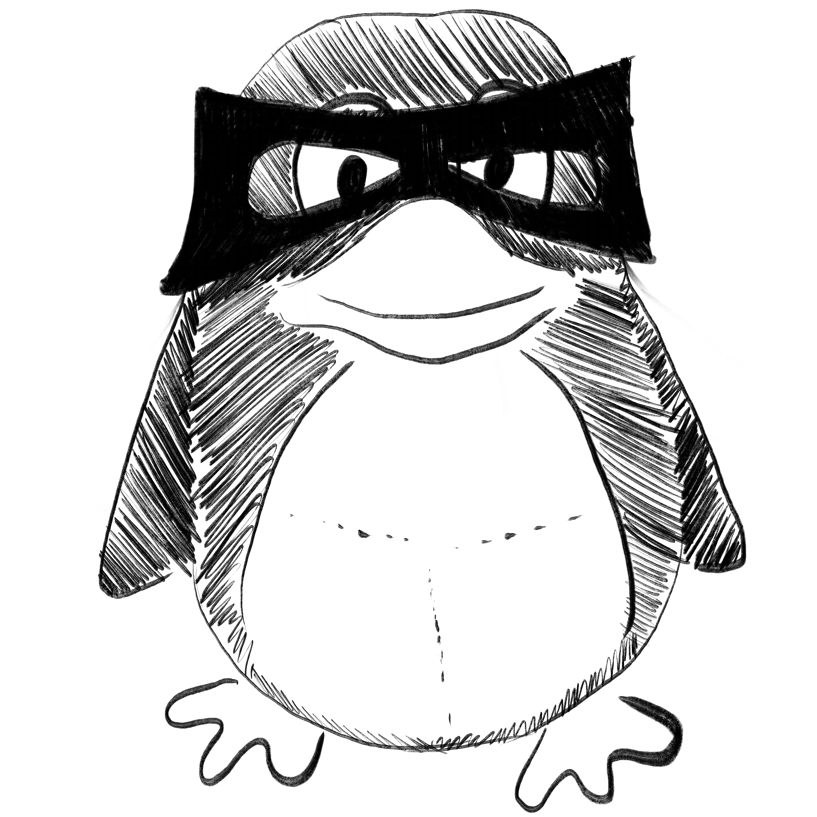
Social Media Devices' Influence on User Neck Pain during the COVID-19 Pandemic: Collaborating Vertebral-GLCM Extracted Features with a Decision Tree.
In Journal of imaging
Al-Naami Bassam, Badr Bashar E A, Rawash Yahia Z, Owida Hamza Abu, De Fazio Roberto, Visconti Paolo
2023-Jan-08
GLCM, decision tree algorithm, neck pain, smartphones, social media usage
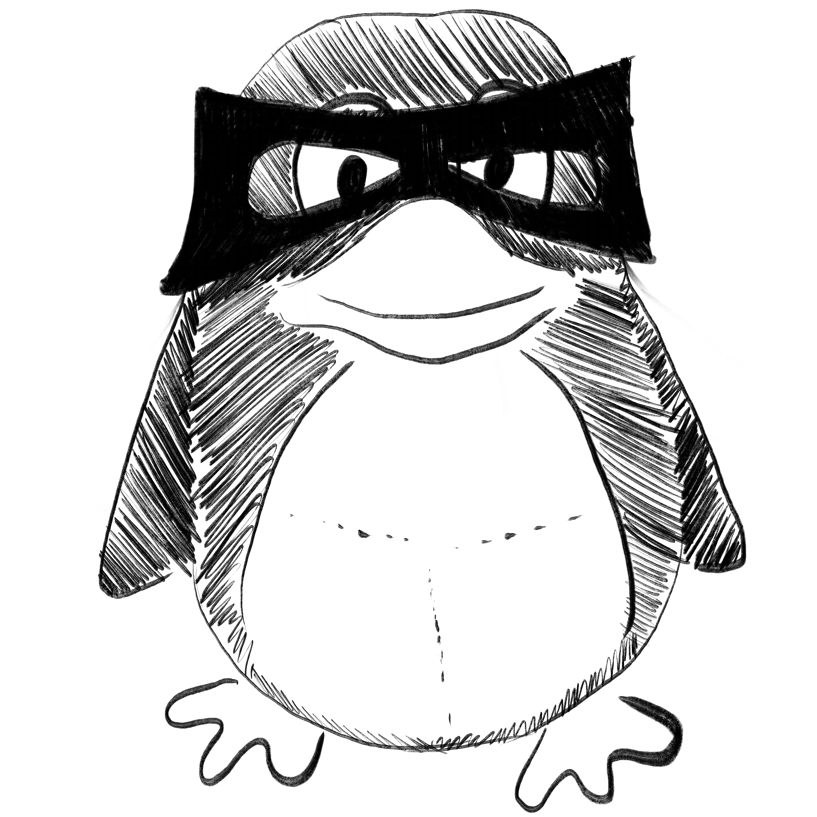
A Survey on Deep Learning in COVID-19 Diagnosis.
In Journal of imaging
Han Xue, Hu Zuojin, Wang Shuihua, Zhang Yudong
2022-Dec-20
COVID-19, CT images, X-ray images, classification, convolutional neural networks, deep learning, diagnosis, transfer learning
Machine learning identifies T cell receptor repertoire signatures associated with COVID-19 severity.
In Communications biology
Park Jonathan J, Lee Kyoung A V, Lam Stanley Z, Moon Katherine S, Fang Zhenhao, Chen Sidi
2023-Jan-20
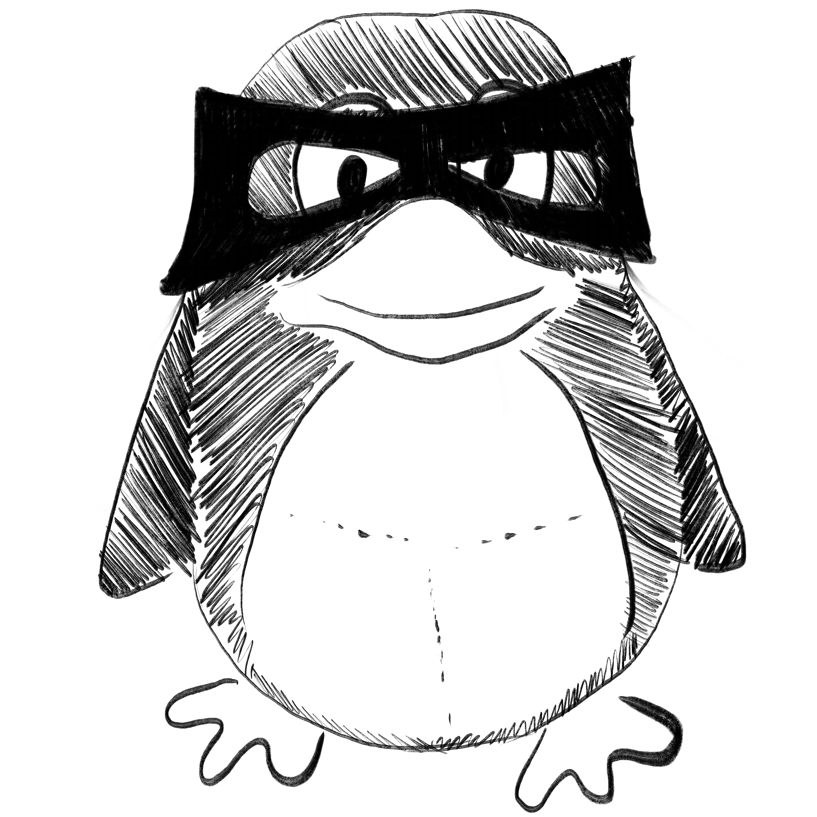
Fog-cloud architecture-driven Internet of Medical Things framework for healthcare monitoring.
In Medical & biological engineering & computing ; h5-index 32.0
Yıldırım Emre, Cicioğlu Murtaza, Çalhan Ali
2023-Jan-21
Cloud computing, Data analytics, Fog computing, IoMT, Machine learning, WBANs
Nesting the SIRV model with NAR, LSTM and statistical methods to fit and predict COVID-19 epidemic trend in Africa.
In BMC public health ; h5-index 82.0
OBJECTIVE :
METHODS :
RESULTS :
CONCLUSION :
Liu Xu-Dong, Wang Wei, Yang Yi, Hou Bo-Han, Olasehinde Toba Stephen, Feng Ning, Dong Xiao-Ping
2023-Jan-19
ARIMA, COVID-19, Epidemic, Functionalized β, Machine learning, Nested model, SIRV model
Improving the performance of machine learning algorithms for health outcomes predictions in multicentric cohorts.
In Scientific reports ; h5-index 158.0
Wichmann Roberta Moreira, Fernandes Fernando Timoteo, Chiavegatto Filho Alexandre Dias Porto
2023-Jan-19
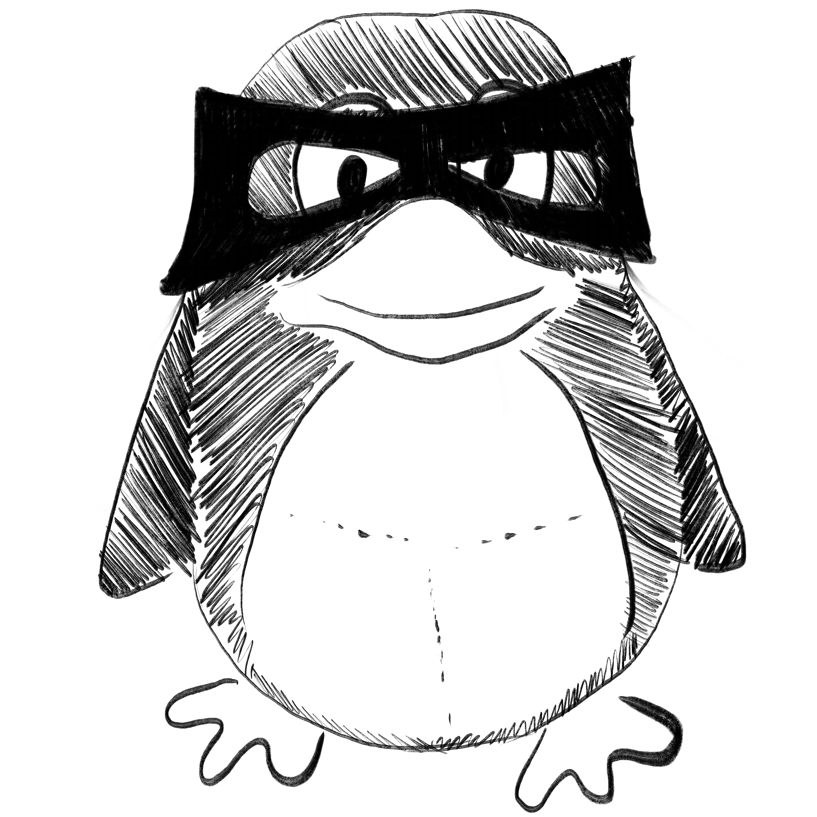
Role of different types of RNA molecules in the severity prediction of SARS-CoV-2 patients.
In Pathology, research and practice
Jeyananthan Pratheeba
2023-Jan-15
COVID-19 molecular data, Classification algorithm, Feature selection, Severity prediction, Treatment stage, lncRNA, miRNA and mRNA
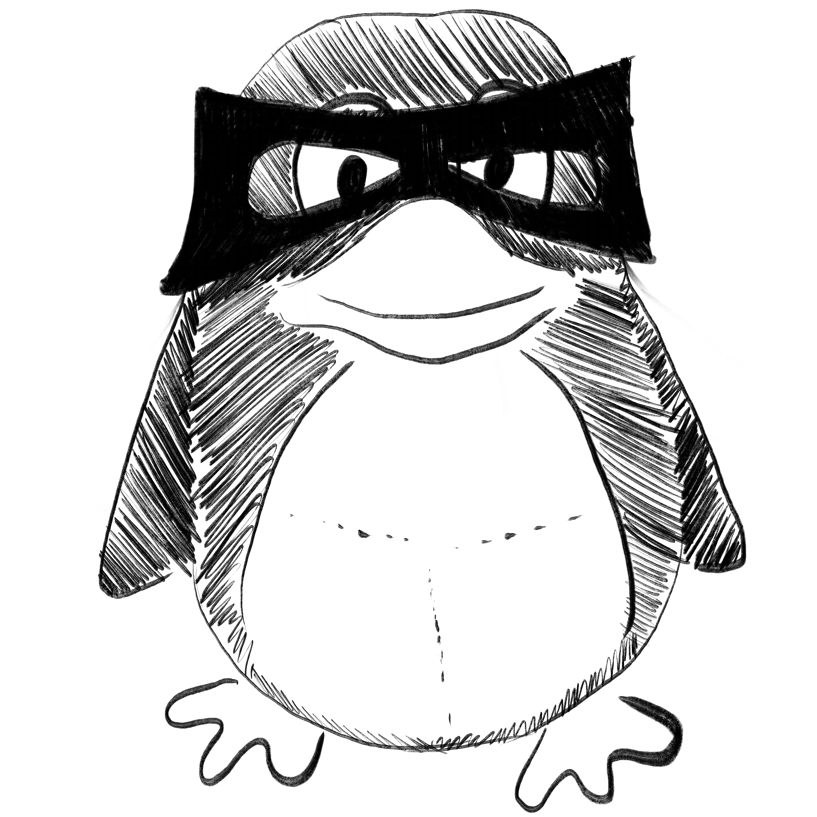
Characterizing SARS-CoV-2 Spike Sequences Based on Geographical Location.
In Journal of computational biology : a journal of computational molecular cell biology
Ali Sarwan, Bello Babatunde, Tayebi Zahra, Patterson Murray
2023-Jan-19
COVID-19, SARS-CoV-2, geographical location, k-mers, sequence classification
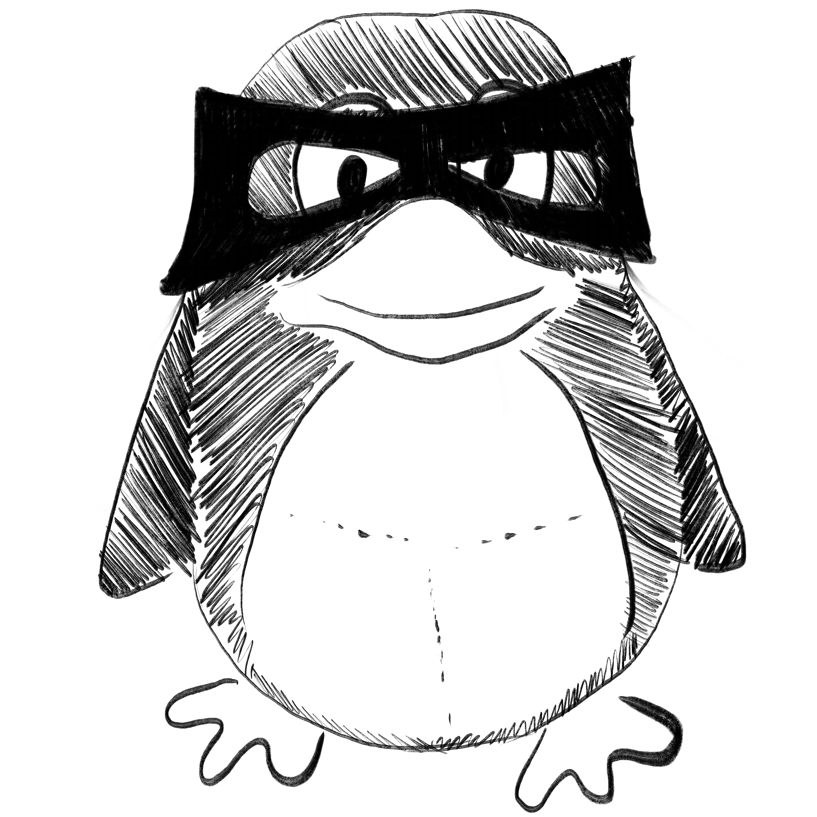
CNGOD-An improved convolution neural network with grasshopper optimization for detection of COVID-19.
In Mathematical biosciences and engineering : MBE
Singh Akansha, Singh Krishna Kant, Greguš Michal, Izonin Ivan
2022-Aug-26
** COVID-19 , Grasshopper Optimization , deep learning , diagnosis , machine learning **
Prehospital Cardiac Arrest should be considered when evaluating Covid-19 mortality in the United States.
In Methods of information in medicine
BACKGROUND :
OBJECTIVES :
METHODS :
RESULTS :
CONCLUSIONS :
Williams Nick
2023-Jan-18
Evolution of social mood in Spain throughout the COVID-19 vaccination process: a machine learning approach to tweets analysis.
In Public health
OBJECTIVES :
METHODS :
RESULTS :
CONCLUSIONS :
Turón A, Altuzarra A, Moreno-Jiménez J M, Navarro J
2022-Dec-14
COVID-19 vaccination process, Machine learning, Multivariate statistics, Sentiment analysis, Social mood, Tweets
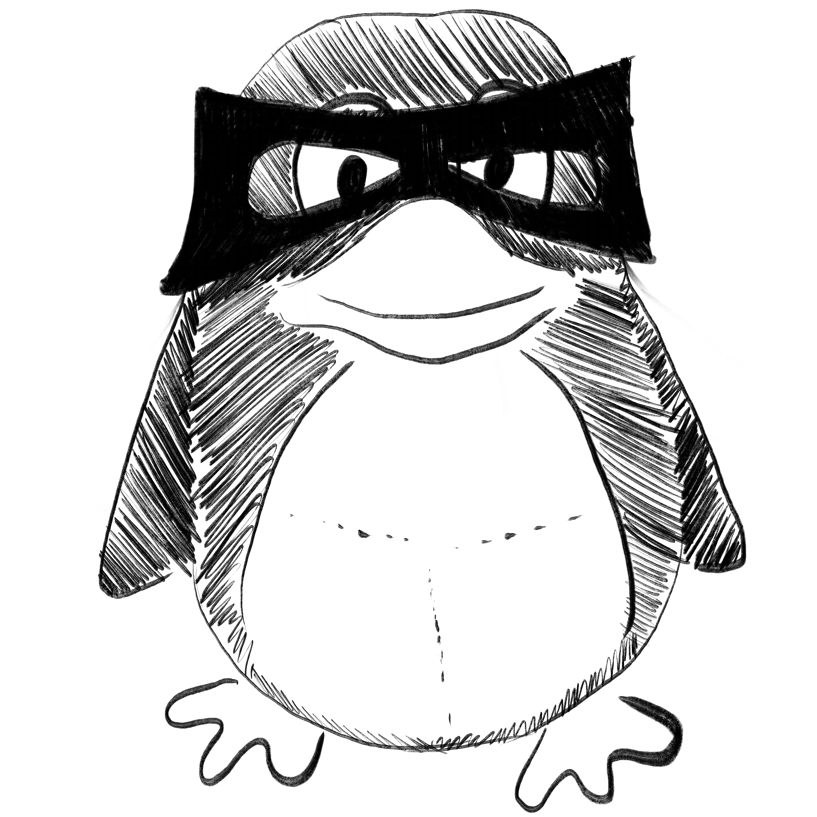
Estimating Remaining Lifespan from the Face
ArXiv Preprint
Amir Fekrazad
2023-01-19
Using digital traces to build prospective and real-time county-level early warning systems to anticipate COVID-19 outbreaks in the United States.
In Science advances
Stolerman Lucas M, Clemente Leonardo, Poirier Canelle, Parag Kris V, Majumder Atreyee, Masyn Serge, Resch Bernd, Santillana Mauricio
2023-Jan-18
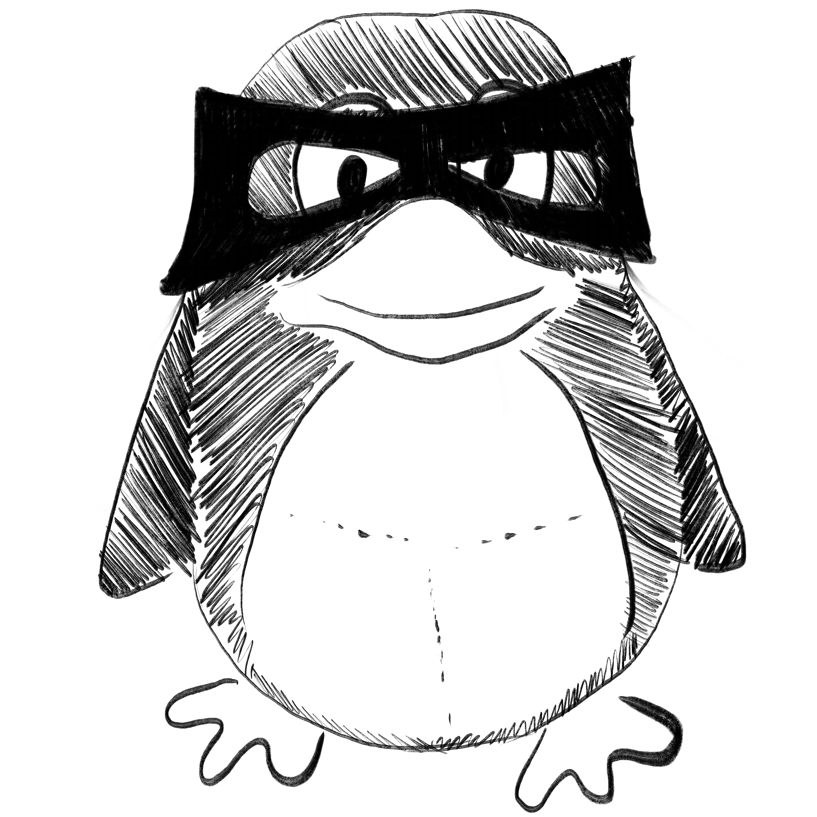
Diffusion-based Conditional ECG Generation with Structured State Space Models
ArXiv Preprint
Juan Miguel Lopez Alcaraz, Nils Strodthoff
2023-01-19
Is the generalizability of a developed artificial intelligence algorithm for COVID-19 on chest CT sufficient for clinical use? Results from the International Consortium for COVID-19 Imaging AI (ICOVAI).
In European radiology ; h5-index 62.0
OBJECTIVES :
METHODS :
RESULTS :
CONCLUSION :
KEY POINTS :
Topff Laurens, Groot Lipman Kevin B W, Guffens Frederic, Wittenberg Rianne, Bartels-Rutten Annemarieke, van Veenendaal Gerben, Hess Mirco, Lamerigts Kay, Wakkie Joris, Ranschaert Erik, Trebeschi Stefano, Visser Jacob J, Beets-Tan Regina G H
2023-Jan-18
Artificial intelligence, COVID-19, Computed tomography, Reproducibility of results, Validation study
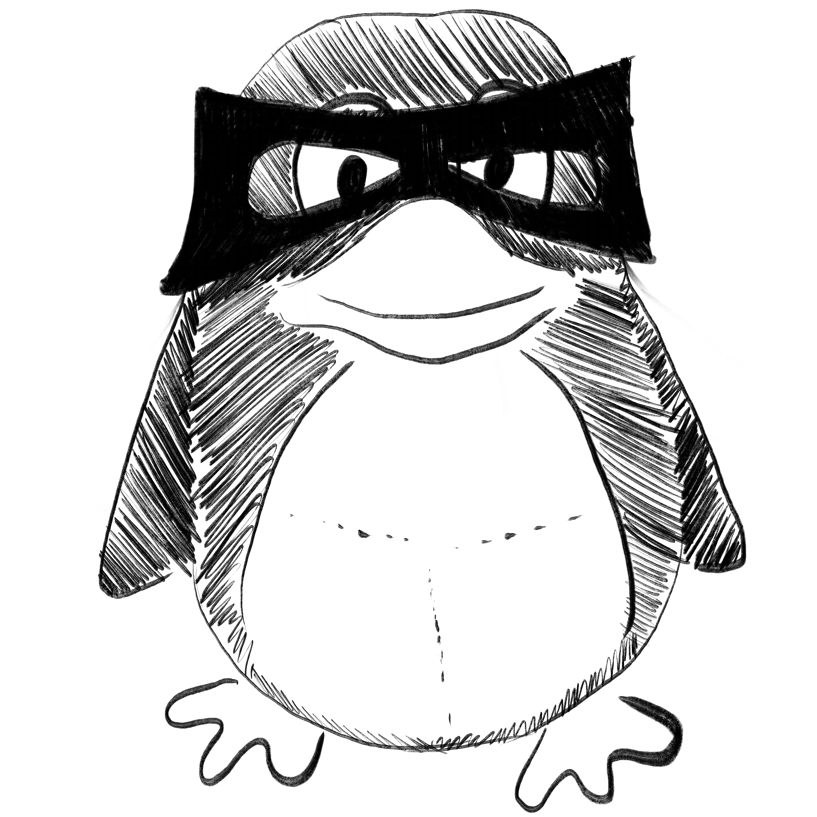
Improving Food Detection For Images From a Wearable Egocentric Camera
ArXiv Preprint
Yue Han, Sri Kalyan Yarlagadda, Tonmoy Ghosh, Fengqing Zhu, Edward Sazonov, Edward J. Delp
2023-01-19
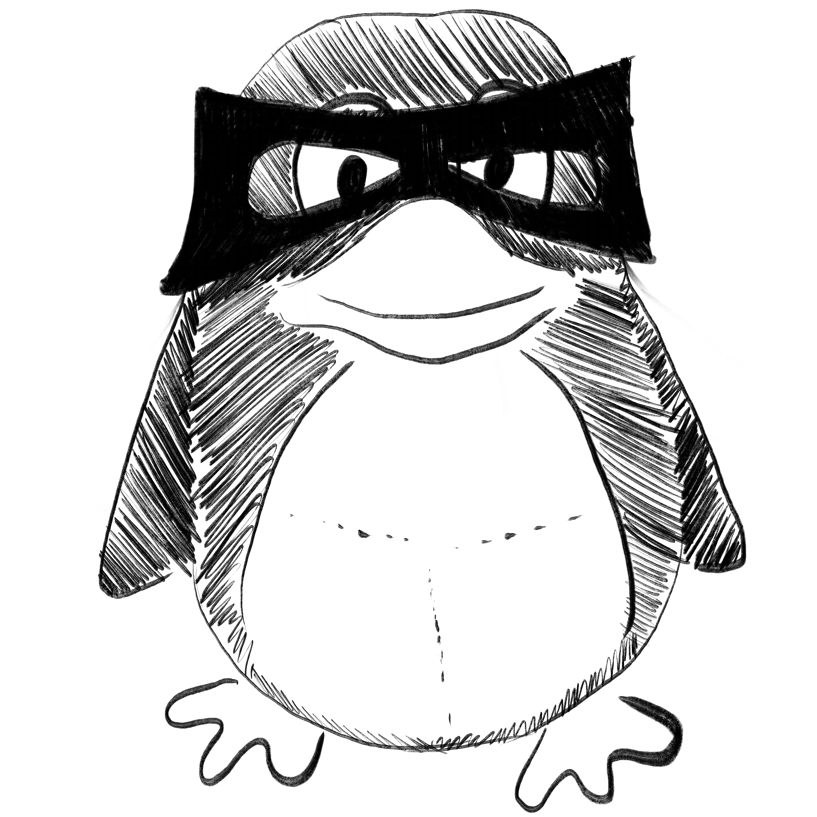
Pandemic disease detection through wireless communication using infrared image based on deep learning.
In Mathematical biosciences and engineering : MBE
Alhameed Mohammed, Jeribi Fathe, Elnaim Bushra Mohamed Elamin, Hossain Mohammad Alamgir, Abdelhag Mohammed Eltahir
2023-Jan
** convolution neural networks , deep learning , infrared image , machine intelligence , ten-folded-validation method **
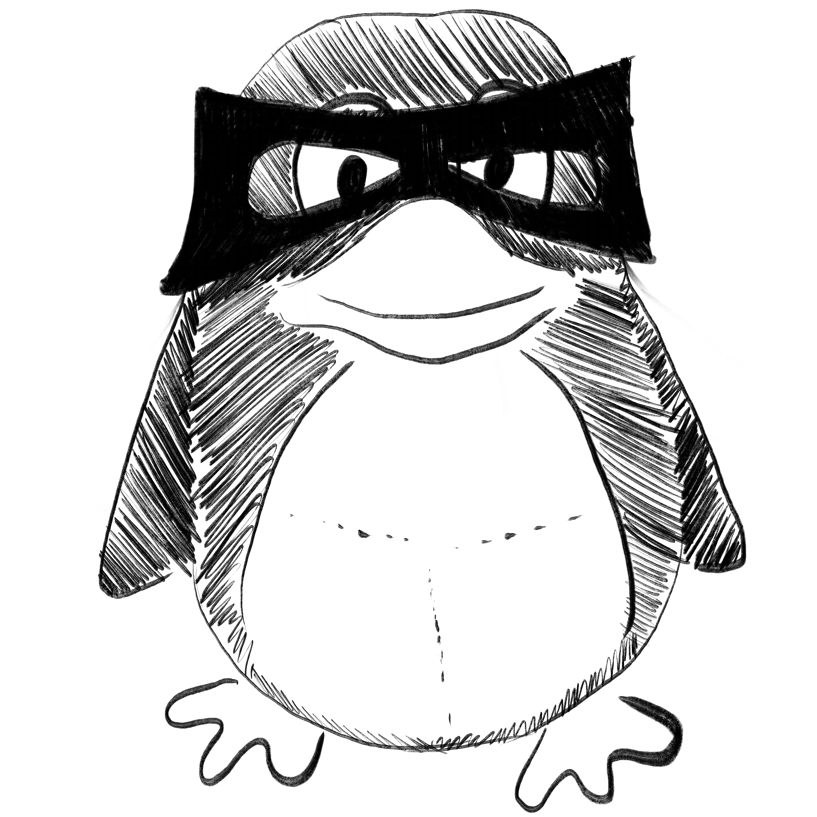
Causal conditional hidden Markov model for multimodal traffic prediction
ArXiv Preprint
Yu Zhao, Pan Deng, Junting Liu, Xiaofeng Jia, Mulan Wang
2023-01-19
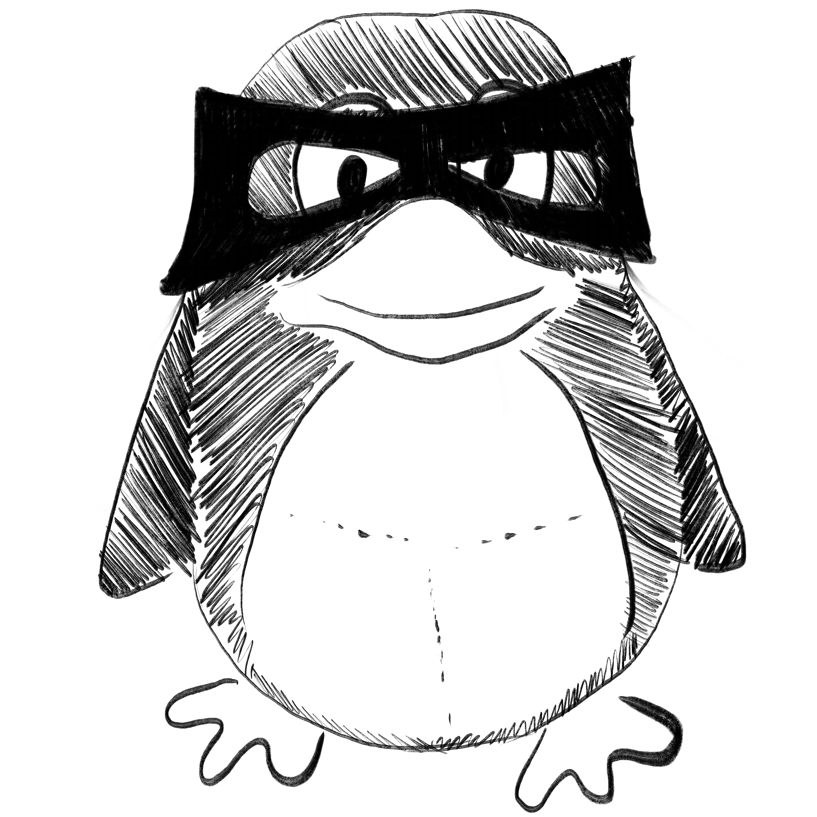
On the implementation of a new version of the Weibull distribution and machine learning approach to model the COVID-19 data.
In Mathematical biosciences and engineering : MBE
Zhou Yinghui, Ahmad Zubair, Almaspoor Zahra, Khan Faridoon, Tag-Eldin Elsayed, Iqbal Zahoor, El-Morshedy Mahmoud
2023-Jan
** family of distributions , healthcare sector , machine learning algorithms , mathematical properties , simulation , statistical modeling **
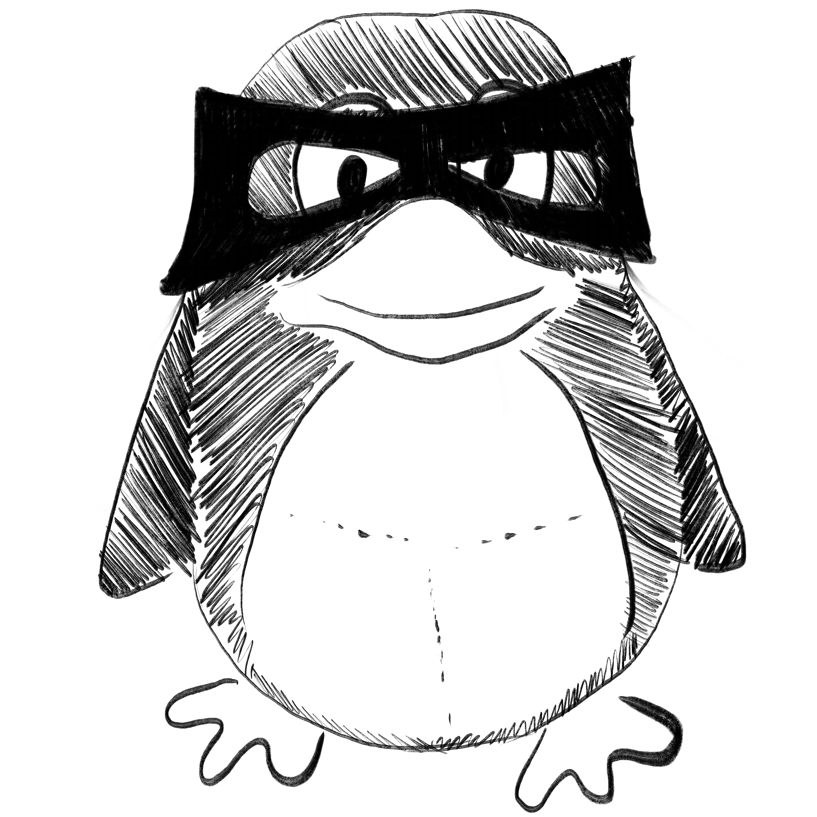
Ensemble of deep learning language models to support the creation of living systematic reviews for the COVID-19 literature: a retrospective study
bioRxiv Preprint
Knafou, J.; Haas, Q.; Borissov, N.; Counotte, M. J.; Low, N.; Imeri, H.; Ipekci, A. M.; Buitrago-Garcia, D.; Heron, L.; Amini, P.; Teodoro, D.
2023-01-19
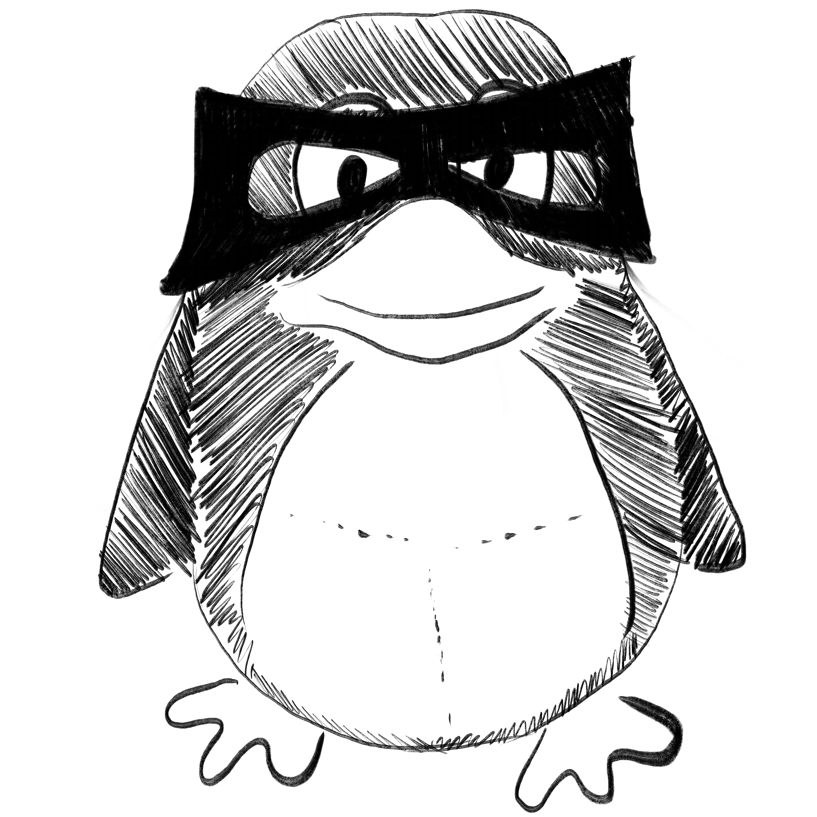
Decision-Focused Evaluation: Analyzing Performance of Deployed Restless Multi-Arm Bandits
ArXiv Preprint
Paritosh Verma, Shresth Verma, Aditya Mate, Aparna Taneja, Milind Tambe
2023-01-19
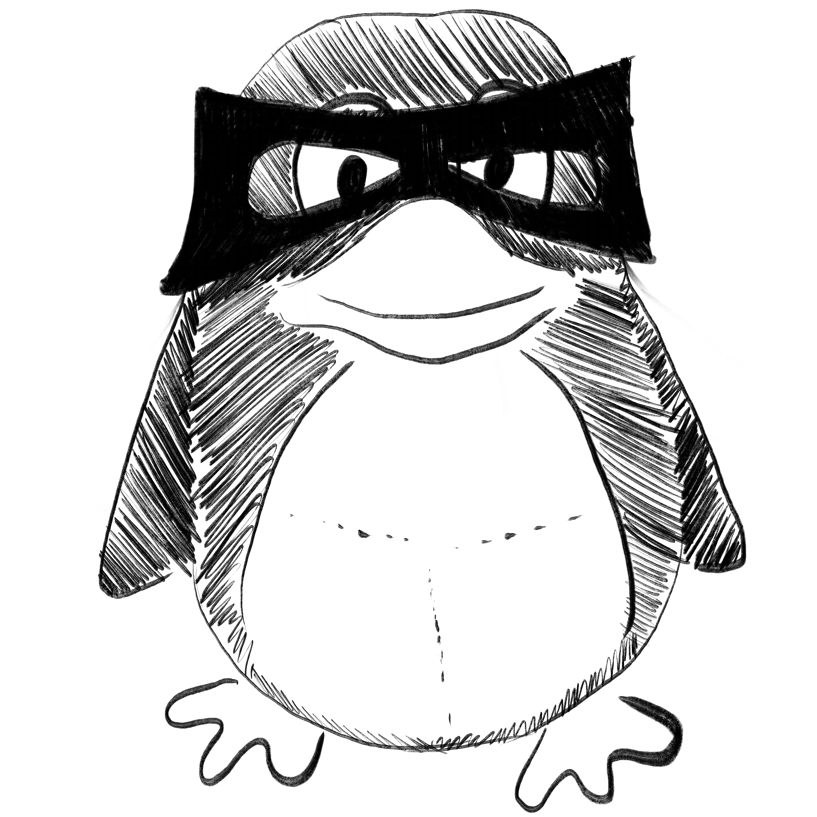
Automated grading of chest x-ray images for viral pneumonia with convolutional neural networks ensemble and region of interest localization.
In PloS one ; h5-index 176.0
Khan Asad, Akram Muhammad Usman, Nazir Sajid
2023
Applications of social media and digital technology in COVID-19 vaccination: a scoping review.
In Journal of medical Internet research ; h5-index 88.0
BACKGROUND :
OBJECTIVE :
METHODS :
RESULTS :
CONCLUSIONS :
CLINICALTRIAL :
Zang Shujie, Zhang Xu, Xing Yuting, Chen Jiaxian, Lin Leesa, Hou Zhiyuan
2023-Jan-13
Technology-Enabled Collaborative Care for Concurrent Diabetes and Distress Management During the COVID-19 Pandemic: Protocol for a Mixed Methods Feasibility Study.
In JMIR research protocols ; h5-index 26.0
BACKGROUND :
OBJECTIVE :
METHODS :
RESULTS :
CONCLUSIONS :
TRIAL REGISTRATION :
INTERNATIONAL REGISTERED REPORT IDENTIFIER (IRRID) :
Vojtila Lenka, Sherifali Diana, Dragonetti Rosa, Ashfaq Iqra, Veldhuizen Scott, Naeem Farooq, Agarwal Sri Mahavir, Melamed Osnat C, Crawford Allison, Gerretsen Philip, Hahn Margaret, Hill Sean, Kidd Sean, Mulsant Benoit, Serhal Eva, Tackaberry-Giddens Leah, Whitmore Carly, Marttila Jennifer, Tang Frank, Ramdass Seeta, Lourido Gloria, Sockalingam Sanjeev, Selby Peter
2023-Jan-17
coaching, collaborative care, diabetes, diabetic, digital health, eHealth, feasibility, health outcome, mental health, nurse, nursing, patient education, qualitative, satisfaction, substance use, technology, type 2 diabetes mellitus, virtual care
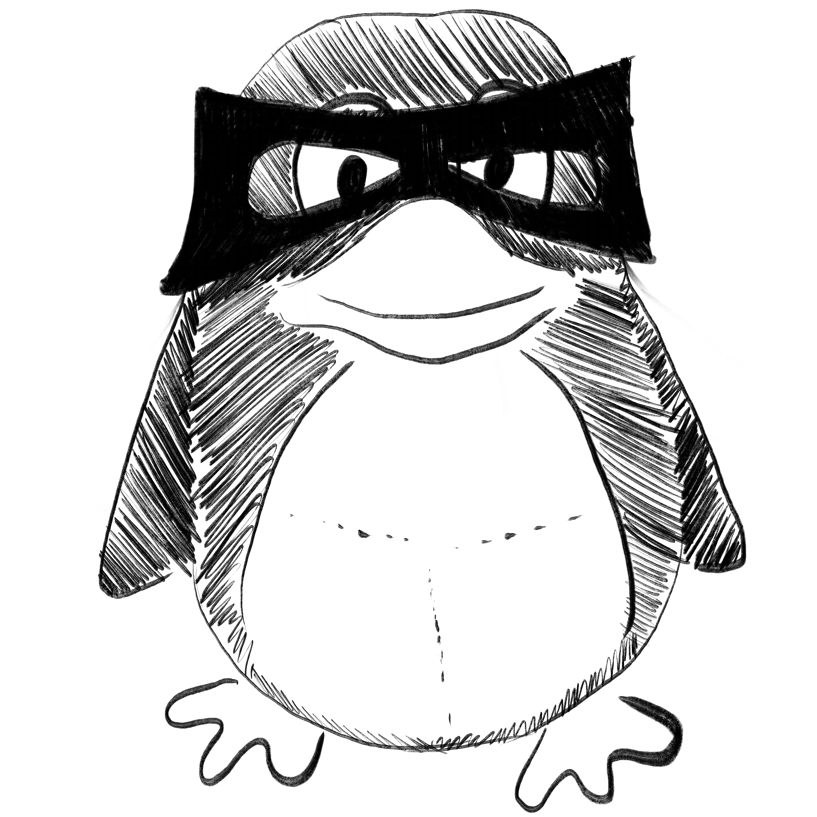
Predicting brain-regional gene regulatory networks from multi-omics for Alzheimer's disease phenotypes and Covid-19 severity.
In Human molecular genetics ; h5-index 81.0
Khullar Saniya, Wang Daifeng
2023-Jan-16
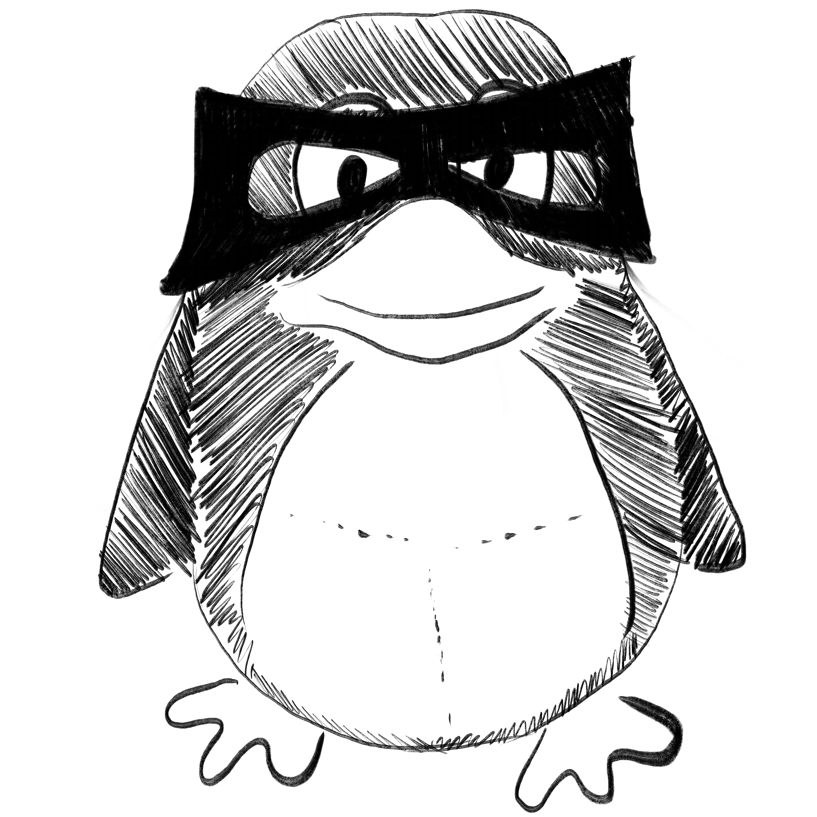
Towards an ML-Based Semantic IoT for Pandemic Management: A Survey of Enabling Technologies for COVID-19.
In Neurocomputing
Zgheib Rita, Chahbandarian Ghazar, Kamalov Firuz, Messiry Haythem El, Al-Gindy Ahmed
2023-Jan-12
COVID-19, Cloud architecture, Internet of Things, Machine Learning, Ontologies, Survey
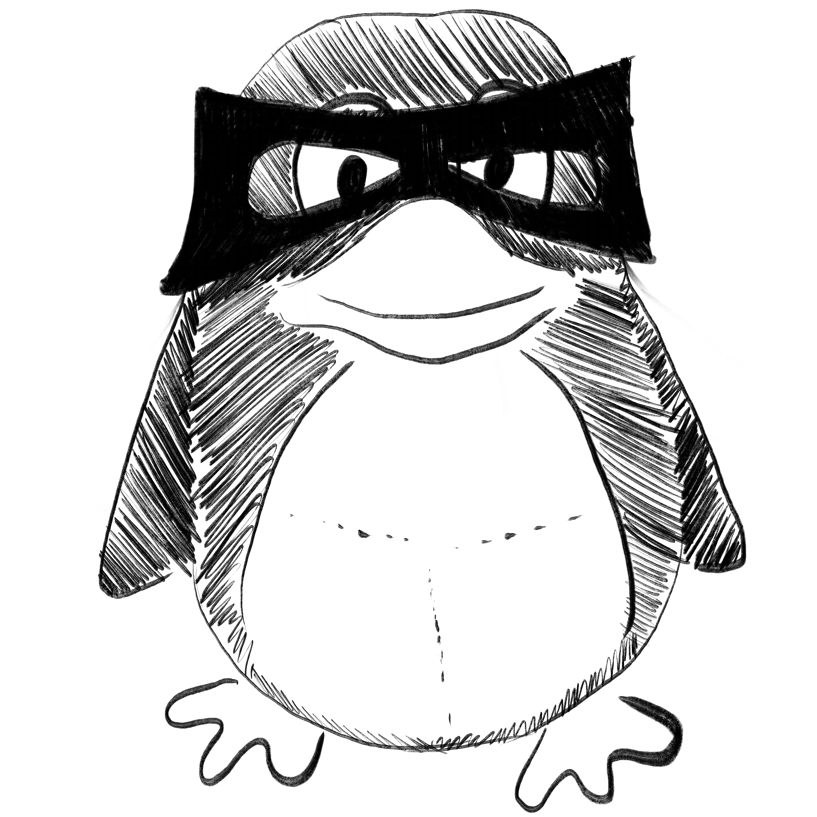
#COVIDisAirborne: AI-enabled multiscale computational microscopy of delta SARS-CoV-2 in a respiratory aerosol.
In The international journal of high performance computing applications
Dommer Abigail, Casalino Lorenzo, Kearns Fiona, Rosenfeld Mia, Wauer Nicholas, Ahn Surl-Hee, Russo John, Oliveira Sofia, Morris Clare, Bogetti Anthony, Trifan Anda, Brace Alexander, Sztain Terra, Clyde Austin, Ma Heng, Chennubhotla Chakra, Lee Hyungro, Turilli Matteo, Khalid Syma, Tamayo-Mendoza Teresa, Welborn Matthew, Christensen Anders, Smith Daniel Ga, Qiao Zhuoran, Sirumalla Sai K, O’Connor Michael, Manby Frederick, Anandkumar Anima, Hardy David, Phillips James, Stern Abraham, Romero Josh, Clark David, Dorrell Mitchell, Maiden Tom, Huang Lei, McCalpin John, Woods Christopher, Gray Alan, Williams Matt, Barker Bryan, Rajapaksha Harinda, Pitts Richard, Gibbs Tom, Stone John, Zuckerman Daniel M, Mulholland Adrian J, Miller Thomas, Jha Shantenu, Ramanathan Arvind, Chong Lillian, Amaro Rommie E
2023-Jan
AI, COVID-19, Delta, GPU, HPC, SARS-CoV-2, aerosols, computational virology, deep learning, molecular dynamics, multiscale simulation, weighted ensemble
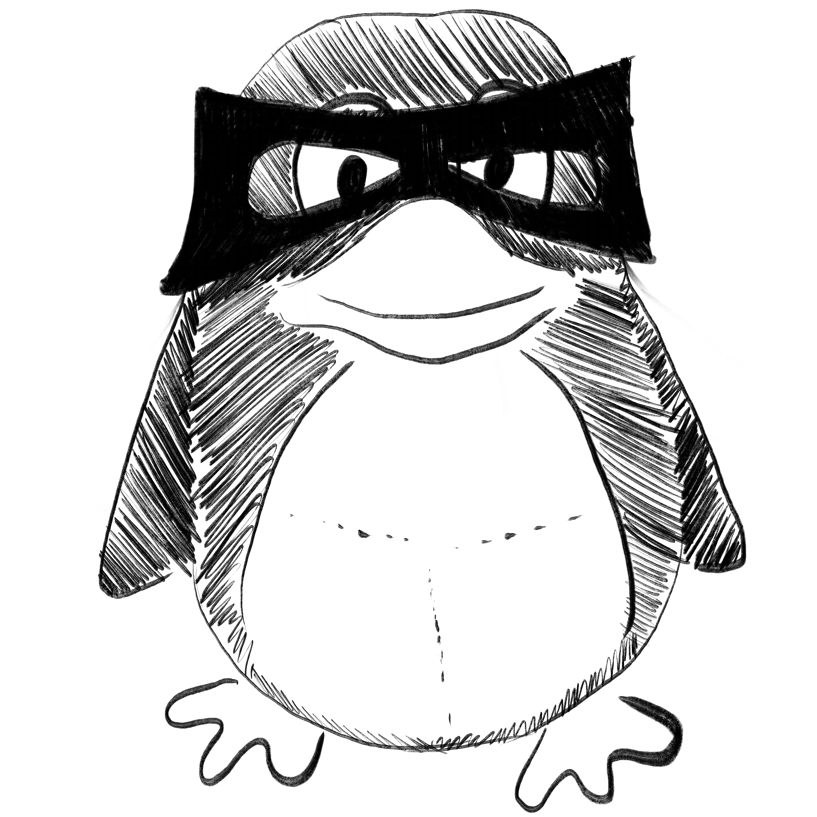
Benchmarking of analytical combinations for COVID-19 outcome prediction using single-cell RNA sequencing data
bioRxiv Preprint
Cao, Y.; Ghazanfar, S.; Yang, P.; Yang, J.
2023-01-18
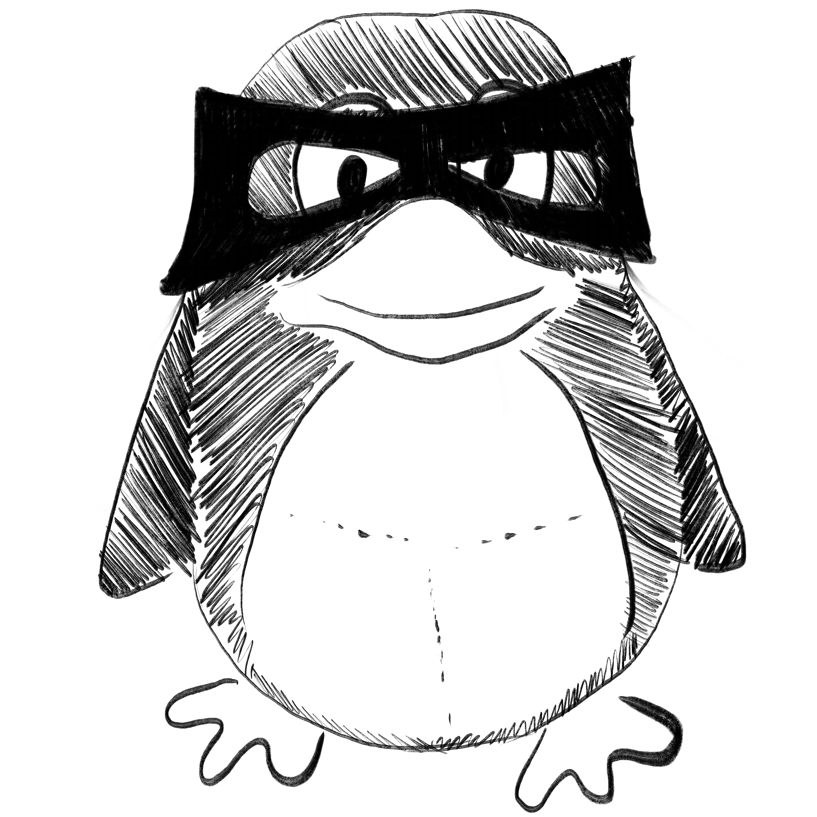
Use of Artificial Intelligence in the Search for New Information Through Routine Laboratory Tests: Systematic Review.
In JMIR bioinformatics and biotechnology
BACKGROUND :
OBJECTIVE :
METHODS :
RESULTS :
CONCLUSIONS :
Cardozo Glauco, Tirloni Salvador Francisco, Pereira Moro Antônio Renato, Marques Jefferson Luiz Brum
2022
COVID-19, diagnosis, laboratory tests, machine learning, prediction, review
Association of Neutralizing Anti-spike Monoclonal Antibody Treatment With COVID-19 Hospitalization and Assessment of the Monoclonal Antibody Screening Score.
In Mayo Clinic proceedings. Innovations, quality & outcomes
OBJECTIVE :
PATIENTS AND METHODS :
RESULTS :
CONCLUSIONS :
Johnson Patrick W, Kunze Katie L, Senefeld Jonathon W, Sinclair Jorge E, Isha Shahin, Satashia Parthkumar H, Bhakta Shivang, Cowart Jennifer B, Bosch Wendelyn, O’Horo Jack, Shah Sadia Z, Wadei Hani M, Edwards Michael A, Pollock Benjamin D, Edwards Alana J, Scheitel-Tulledge Sidna, Clune Caroline G, Hanson Sara N, Arndt Richard, Heyliger Alexander, Kudrna Cory, Bierle Dennis M, Buckmeier Jason R, Seville Maria Teresa A, Orenstein Robert, Libertin Claudia, Ganesh Ravindra, Franco Pablo Moreno, Razonable Raymund R, Carter Rickey E, Sanghavi Devang K, Speicher Leigh L
2023-Jan-11
CMH, Cochran Mantel Haenszel, COVID-19, Coronavirus Disease 2019, GBM, Gradient Boosting Machine, MASS, Monoclonal Antibody Screening Score, SARS-CoV-2, Severe Acute Respiratory Syndrome Corona Virus 2
The race to understand immunopathology in COVID-19: perspectives on the impact of quantitative approaches to understand within-host interactions.
In Immunoinformatics (Amsterdam, Netherlands)
Gazeau Sonia, Deng Xiaoyan, Ooi Hsu Kiang, Mostefai Fatima, Hussin Julie, Heffernan Jane, Jenner Adrianne L, Craig Morgan
2023-Jan-08
COVID-19, SARS-CoV-2, computational modelling, immunopathology, machine learning, mathematical modelling, population genetics, within-host dynamics
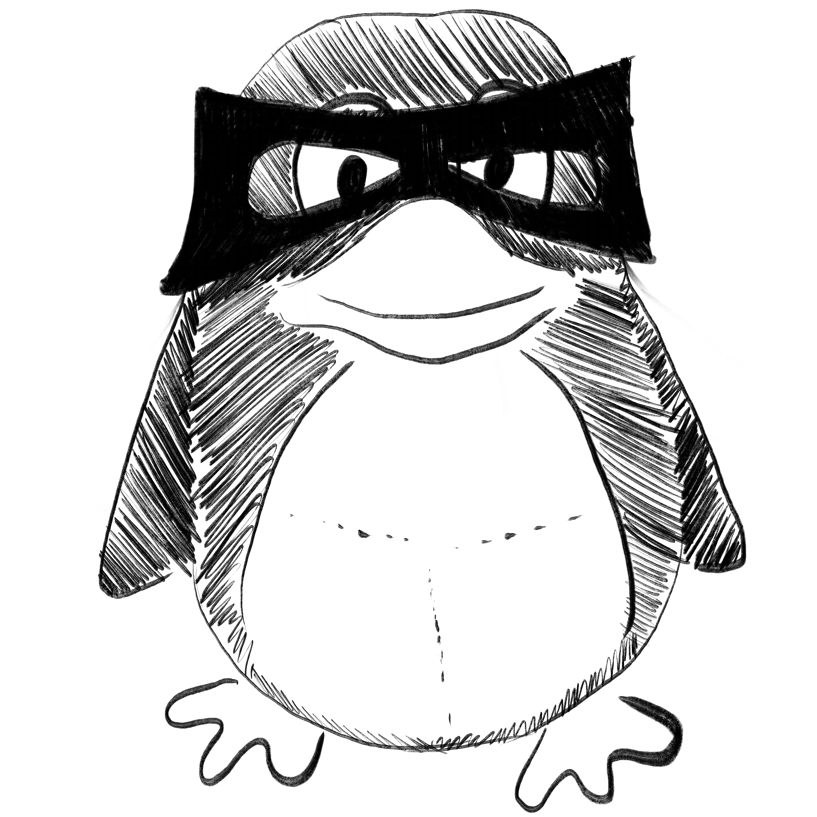
Deep learning-based user experience evaluation in distance learning.
In Cluster computing
Sadigov Rahim, Yıldırım Elif, Kocaçınar Büşra, Patlar Akbulut Fatma, Catal Cagatay
2023-Jan-08
Deep learning, Distance learning, NLP, Sentiment analysis
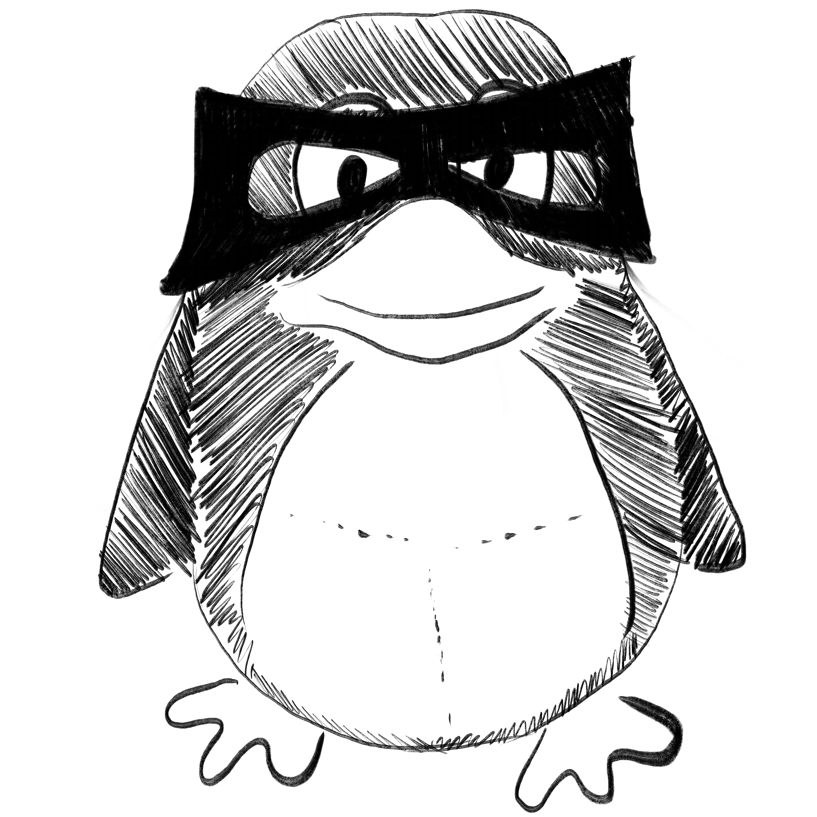
Bibliometric analysis of top-cited articles in Journal of Dental Sciences.
In Journal of dental sciences
BACKGROUND/PURPOSE :
MATERIALS AND METHODS :
RESULTS :
CONCLUSION :
Yang Li-Chiu, Liu Fu-Hsuan, Liu Chia-Min, Yu Chuan-Hang, Chang Yu-Chao
2023-Jan
Bibliometric analysis, Citation analysis, Journal of Dental Sciences, Web of Science
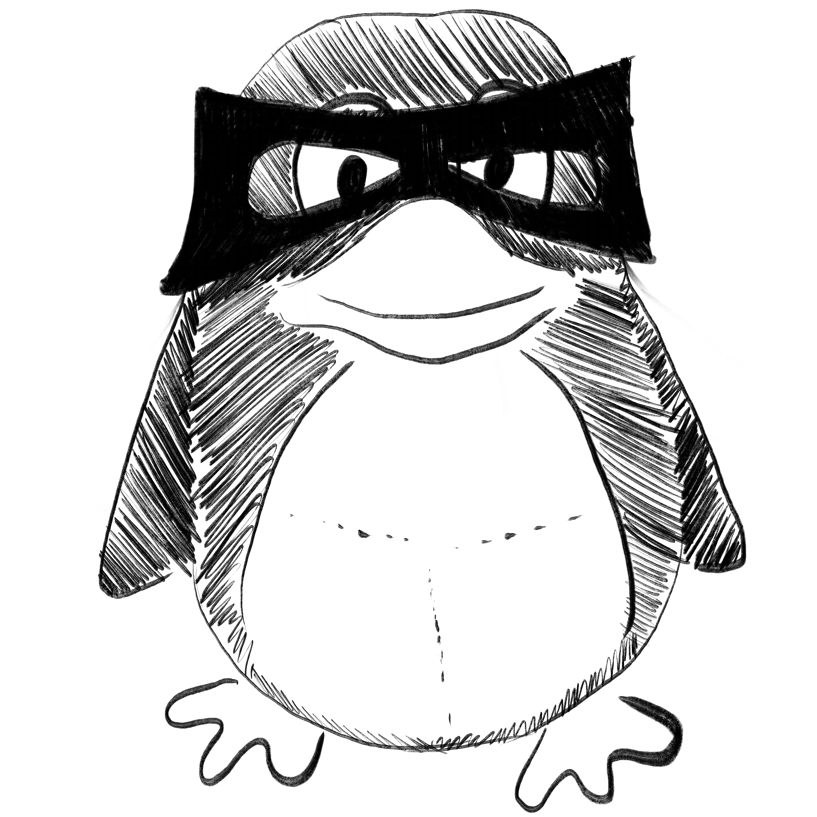
A systematic literature review of machine learning application in COVID-19 medical image classification.
In Procedia computer science
Daniel Cenggoro, Tjeng Wawan Pardamean
2023
COVID-19, classification, disease, machine learning, medical images
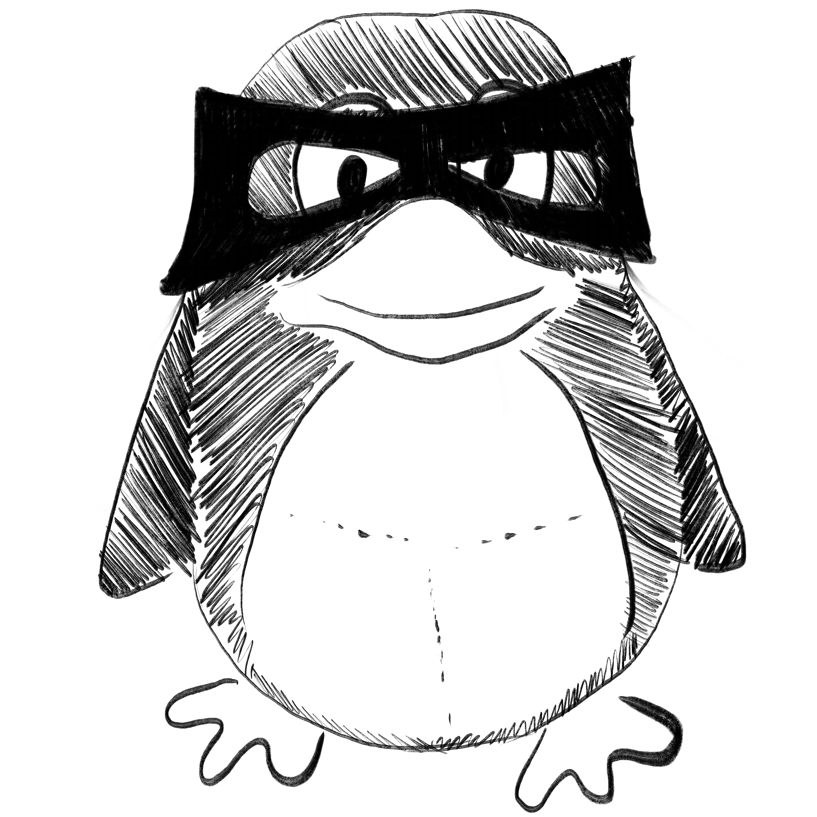
Towards a more general drug target interaction prediction model using transfer learning.
In Procedia computer science
Suhartono Derwin, Majiid Muhammad Rizki Nur, Handoyo Alif Tri, Wicaksono Pandu, Lucky Henry
2023
SMILES, deep learning, drug discovery, drug-target interaction, transfer learning
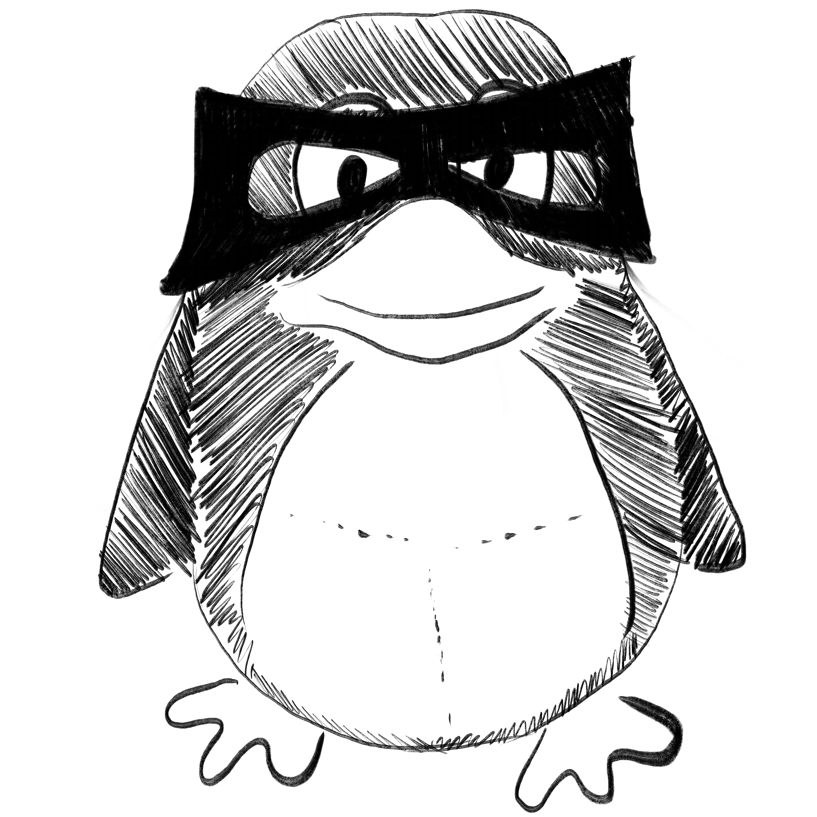
Comparative analysis of deep learning models for detecting face mask.
In Procedia computer science
Ramadhan M Vickya, Muchtar Kahlil, Nurdin Yudha, Oktiana Maulisa, Fitria Maya, Maulina Novi, Elwirehardja Gregorius Natanael, Pardamean Bens
2023
Binary Classification, Deep Learning, Face mask detection
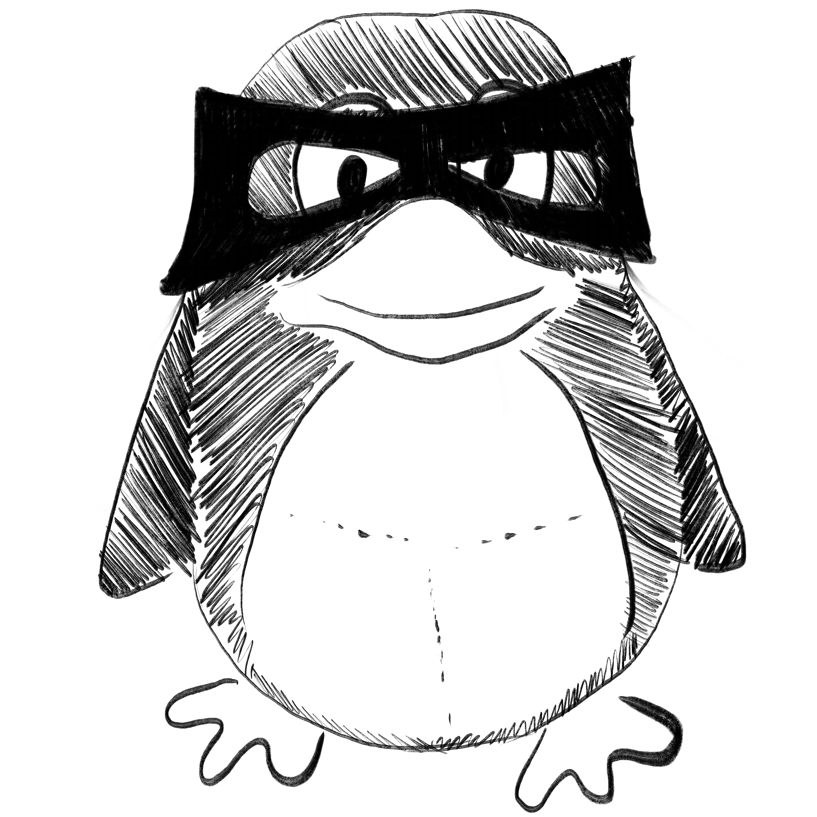
Development clustering system IDX company with k-means algorithm and DBSCAN based on fundamental indicator and ESG.
In Procedia computer science
Pranata Kevin Surya, Gunawan Alexander A S, Gaol Ford Lumban
2023
DBSCAN, ESG, Fundamental Indicator, IDX, K-Means Clustering, KOMPAS100, Machine Learning
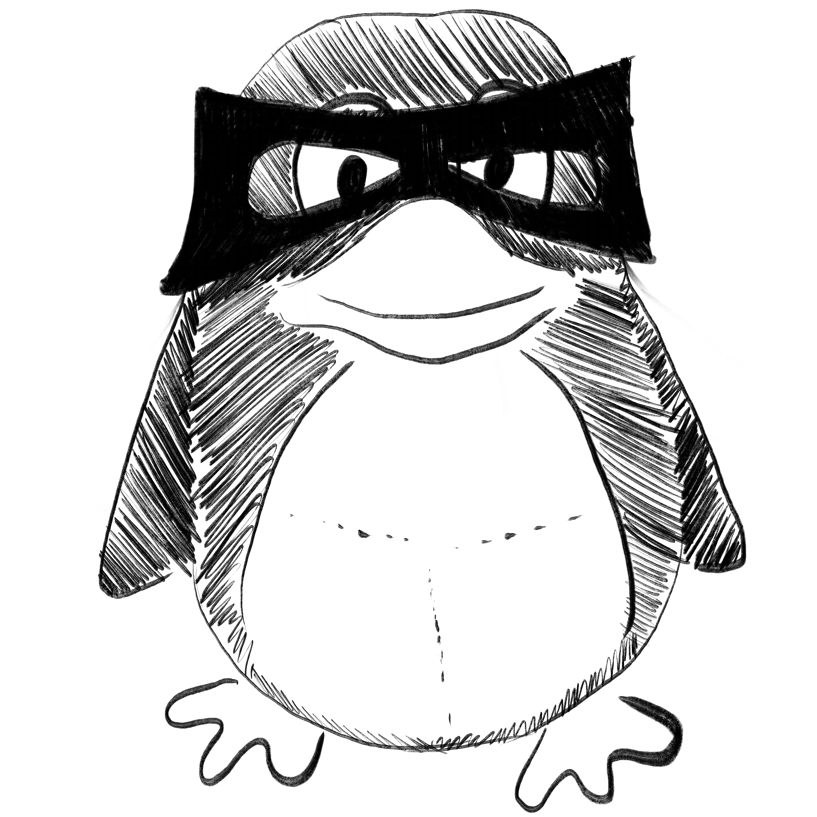
The next generation of evidence-based medicine.
In Nature medicine ; h5-index 170.0
Subbiah Vivek
2023-Jan-16
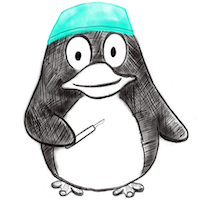
Perioperative Risk Assessment of Patients Using the MyRISK Digital Score Completed Before the Preanesthetic Consultation: Prospective Observational Study.
In JMIR perioperative medicine
BACKGROUND :
OBJECTIVE :
METHODS :
RESULTS :
CONCLUSIONS :
Ferré Fabrice, Laurent Rodolphe, Furelau Philippine, Doumard Emmanuel, Ferrier Anne, Bosch Laetitia, Ba Cyndie, Menut Rémi, Kurrek Matt, Geeraerts Thomas, Piau Antoine, Minville Vincent
2023-Jan-16
chatbot, digital health, machine learning, mobile phone, perioperative risk, preanesthetic consultation
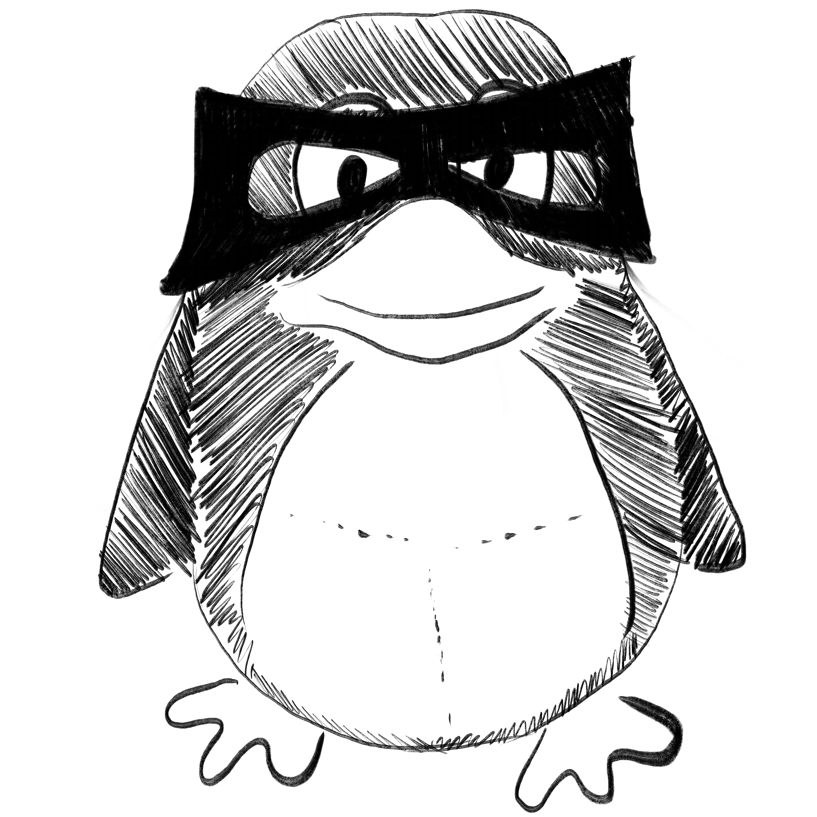
Re-envisioning the design of nanomedicines: Harnessing automation and artificial intelligence.
In Expert opinion on drug delivery
INTRODUCTION :
AREAS COVERED :
EXPERT OPINION :
Zaslavsky Jonathan, Bannigan Pauric, Allen Christine
2023-Jan-16
Artificial intelligence, Automation, Drug delivery, Machine learning, Nanomedicine, Pharmaceutical formulation development
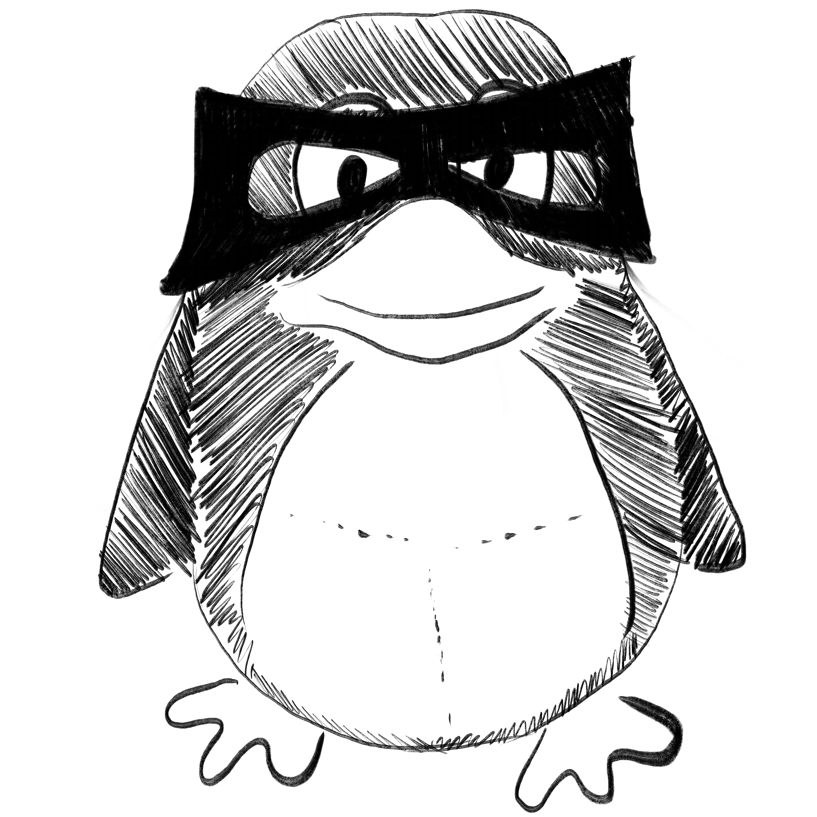
Transformer for one stop interpretable cell type annotation.
In Nature communications ; h5-index 260.0
Chen Jiawei, Xu Hao, Tao Wanyu, Chen Zhaoxiong, Zhao Yuxuan, Han Jing-Dong J
2023-Jan-14
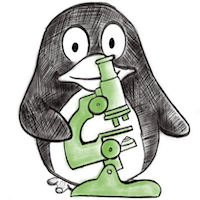
Optimizing variant-specific therapeutic SARS-CoV-2 decoys using deep-learning-guided molecular dynamics simulations.
In Scientific reports ; h5-index 158.0
Köchl Katharina, Schopper Tobias, Durmaz Vedat, Parigger Lena, Singh Amit, Krassnigg Andreas, Cespugli Marco, Wu Wei, Yang Xiaoli, Zhang Yanchong, Wang Welson Wen-Shang, Selluski Crystal, Zhao Tiehan, Zhang Xin, Bai Caihong, Lin Leon, Hu Yuxiang, Xie Zhiwei, Zhang Zaihui, Yan Jun, Zatloukal Kurt, Gruber Karl, Steinkellner Georg, Gruber Christian C
2023-Jan-14
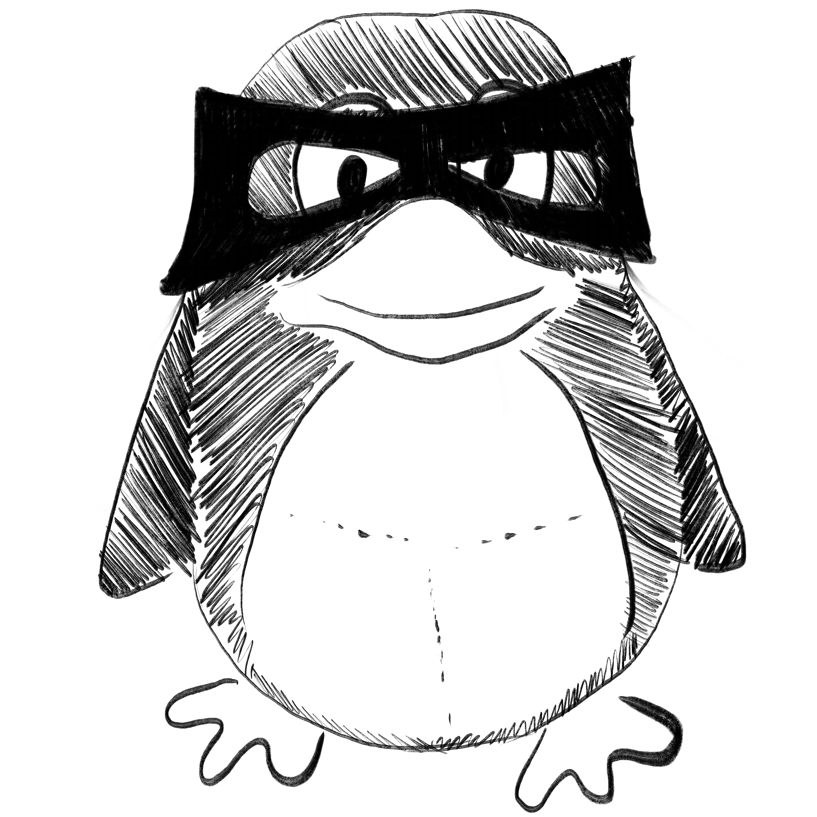
ACSN: Attention capsule sampling network for diagnosing COVID-19 based on chest CT scans.
In Computers in biology and medicine
Wen Cuihong, Liu Shaowu, Liu Shuai, Heidari Ali Asghar, Hijji Mohammad, Zarco Carmen, Muhammad Khan
2022-Nov-22
COVID-19 recognition, Capsule network, Chest CT scan, Deep learning, Feature sampling, Lung infections
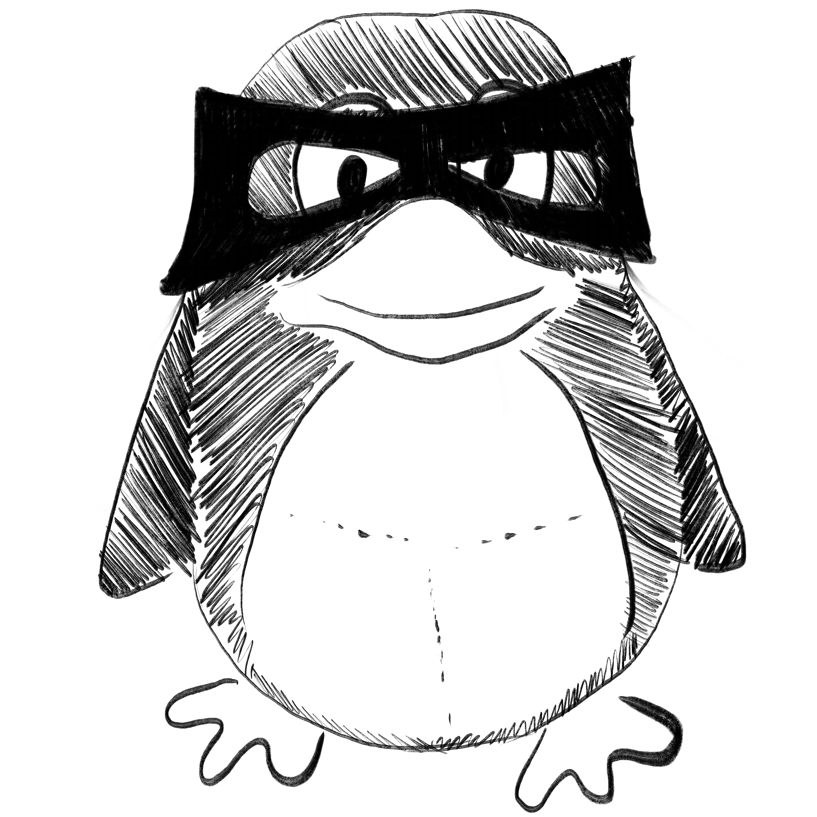
Utilisation of deep learning for COVID-19 diagnosis.
In Clinical radiology
Aslani S, Jacob J
2023-Feb
Necessity and challenges for the post-pandemic Hangzhou Asian Games: An interdisciplinary data science assessment.
In Frontiers in psychology ; h5-index 92.0
BACKGROUND :
METHODS :
RESULTS :
CONCLUSION :
Guo Jianwei, Zhang Xiangning, Cui Dandan
2022
Asian Games, COVID-19, Olympic Games, elite sport, public health
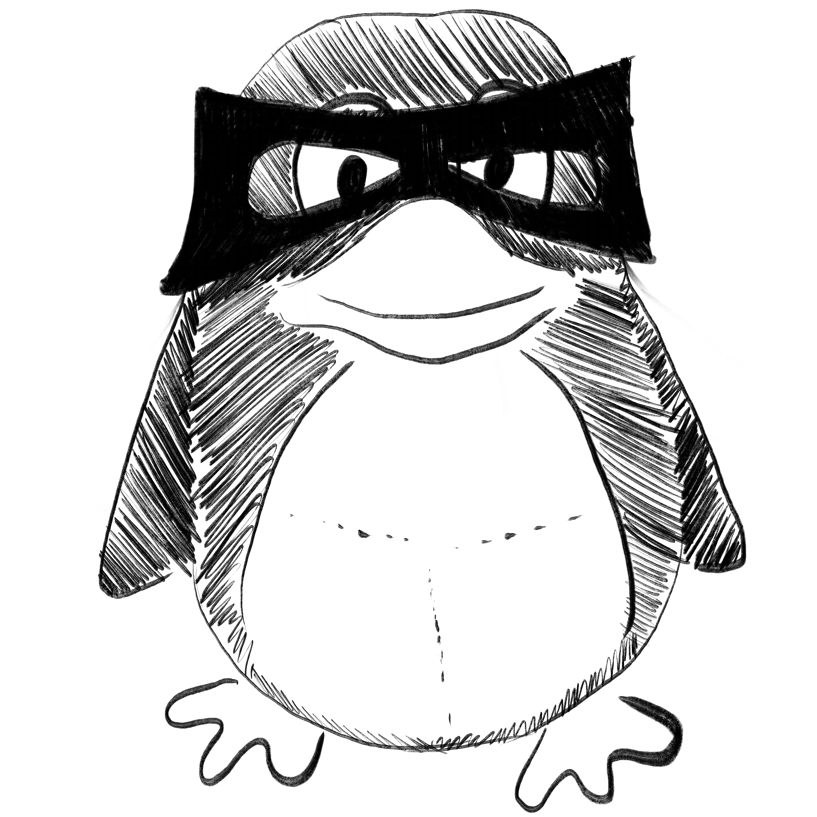
Primary Care Physicians' and Patients' Perspectives on Equity and Health Security of Infectious Disease Digital Surveillance.
In Annals of family medicine
PURPOSE :
METHODS :
RESULTS :
CONCLUSION :
Wong William Chi Wai, Zhao Ivy Yan, Ma Ye Xuan, Dong Wei Nan, Liu Jia, Pang Qin, Lu Xiao Qin, Molassiotis Alex, Holroyd Eleanor
2023-Jan-12
AI, artificial intelligence, disease outbreaks, disease survelillances, ethical issue
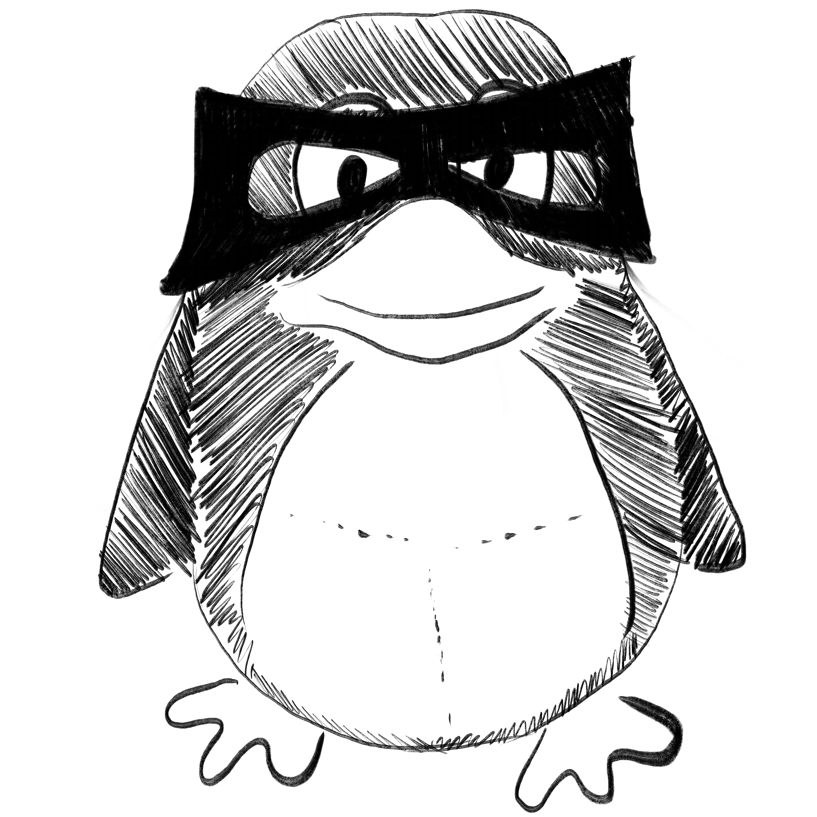
RNAdegformer: accurate prediction of mRNA degradation at nucleotide resolution with deep learning.
In Briefings in bioinformatics
He Shujun, Gao Baizhen, Sabnis Rushant, Sun Qing
2023-Jan-12
COVID-19 mRNA, bioinformatics, deep learning, mRNA vaccine degradation
Machine Learning-Assisted Real-Time Polymerase Chain Reaction and High-Resolution Melt Analysis for SARS-CoV-2 Variant Identification.
In Analytical chemistry
Promja Sutossarat, Puenpa Jiratchaya, Achakulvisut Titipat, Poovorawan Yong, Lee Su Yin, Athamanolap Pornpat, Lertanantawong Benchaporn
2023-Jan-12
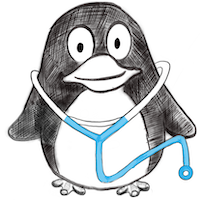
Digital Cough Monitoring - A Potential Predictive Acoustic Biomarker Of Clinical Outcomes in Hospitalized COVID-19 Patients.
In Journal of biomedical informatics ; h5-index 55.0
PURPOSE :
METHODS :
RESULTS :
INTERPRETATION :
Altshuler Ellery, Tannir Bouchra, Jolicoeur Gisèle, Rudd Matthew, Saleem Cyrus, Cherabuddi Kartikeya, Hélène Doré Dominique, Nagarsheth Parav, Brew Joe, Small Peter M, Glenn Morris J, Grandjean Lapierre Simon
2023-Jan-09
Artificial intelligence, Clinical decision making, Cough, Covid-19, Machine learning
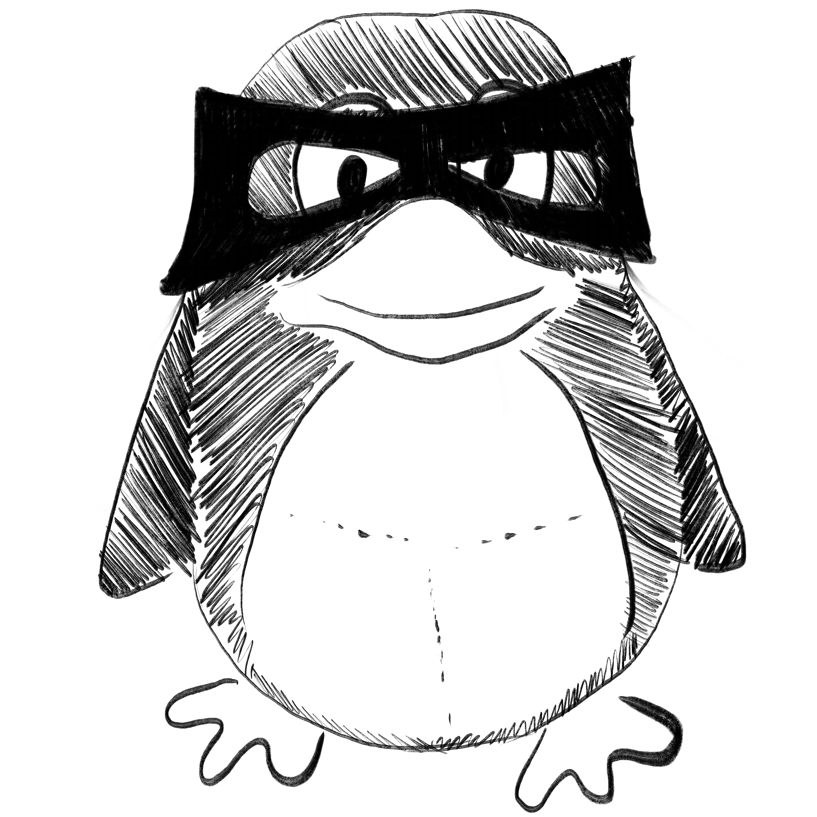
Lethality risk markers by sex and age-group for COVID-19 in Mexico: a cross-sectional study based on machine learning approach.
In BMC infectious diseases ; h5-index 58.0
BACKGROUND :
METHODS :
RESULTS :
CONCLUSIONS :
Rojas-García Mariano, Vázquez Blanca, Torres-Poveda Kirvis, Madrid-Marina Vicente
2023-Jan-11
COVID-19, Lethality risk markers, Machine learning, Mexico
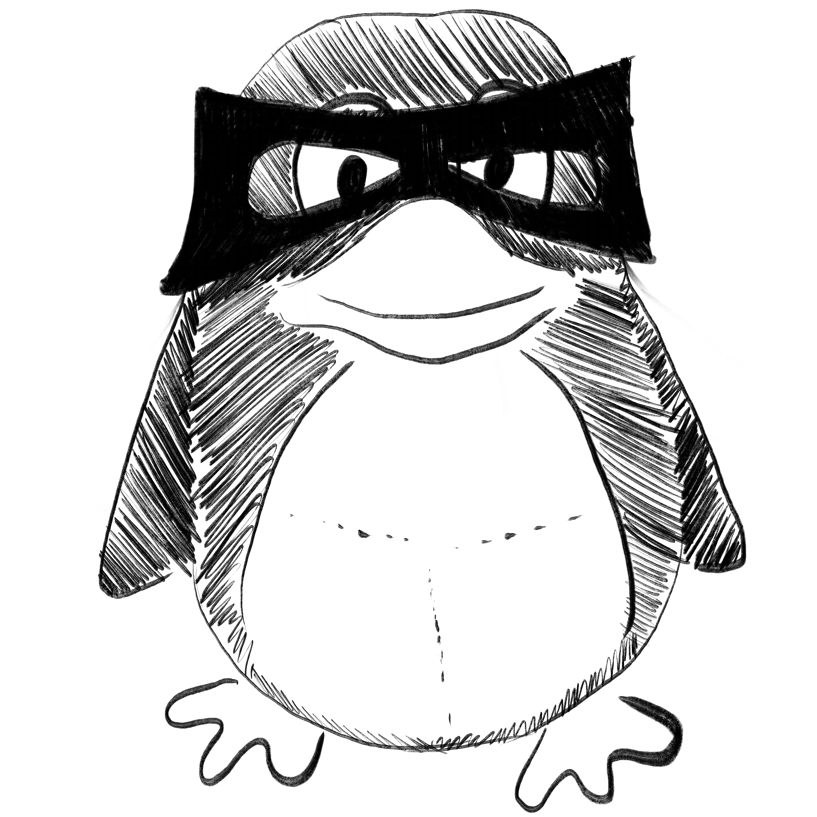
Machine learning for optimal test admission in the presence of resource constraints.
In Health care management science
Elitzur Ramy, Krass Dmitry, Zimlichman Eyal
2023-Jan-12
Data analytics, Machine learning, Optimal test admission policies, Predictive analytics
A Geo-AI-based ensemble mixed spatial prediction model with fine spatial-temporal resolution for estimating daytime/nighttime/daily average ozone concentrations variations in Taiwan.
In Journal of hazardous materials
Babaan Jennieveive, Hsu Fang-Tzu, Wong Pei-Yi, Chen Pau-Chung, Guo Yue-Leon, Lung Shih-Chun Candice, Chen Yu-Cheng, Wu Chih-Da
2023-Jan-07
Diurnal changes, Ensemble learning, Geospatial artificial intelligence, Land use regression, O(3)
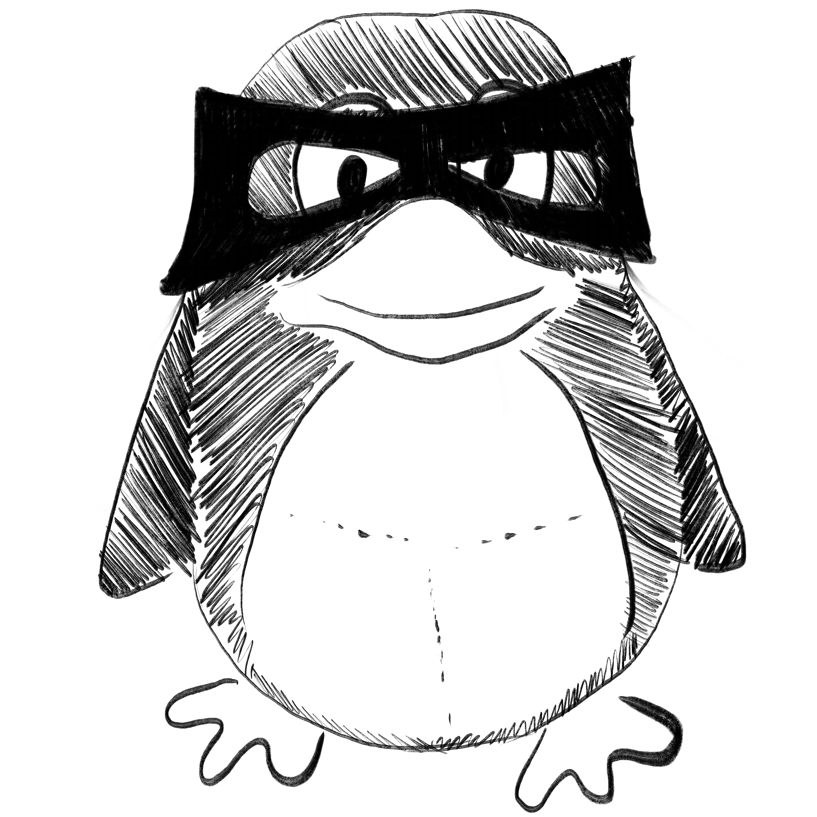
An integrated LSTM-HeteroRGNN model for interpretable opioid overdose risk prediction.
In Artificial intelligence in medicine ; h5-index 34.0
Dong Xinyu, Wong Rachel, Lyu Weimin, Abell-Hart Kayley, Deng Jianyuan, Liu Yinan, Hajagos Janos G, Rosenthal Richard N, Chen Chao, Wang Fusheng
2023-Jan
Clinical decision support, Deep learning, Electronic health records, Graph neural network, Long short-term memory, Opioid overdose, Opioid poisoning
Prognosis of COVID-19 patients using lab tests: A data mining approach.
In Health science reports
BACKGROUND :
METHODS :
RESULTS :
CONCLUSION :
Khounraz Fariba, Khodadoost Mahmood, Gholamzadeh Saeid, Pourhamidi Rashed, Baniasadi Tayebeh, Jafarbigloo Aida, Mohammadi Gohar, Ahmadi Mahnaz, Ayyoubzadeh Seyed Mohammad
2023-Jan
COVID‐19, Gradient Boosted Trees, artificial intelligence, data mining, machine learning
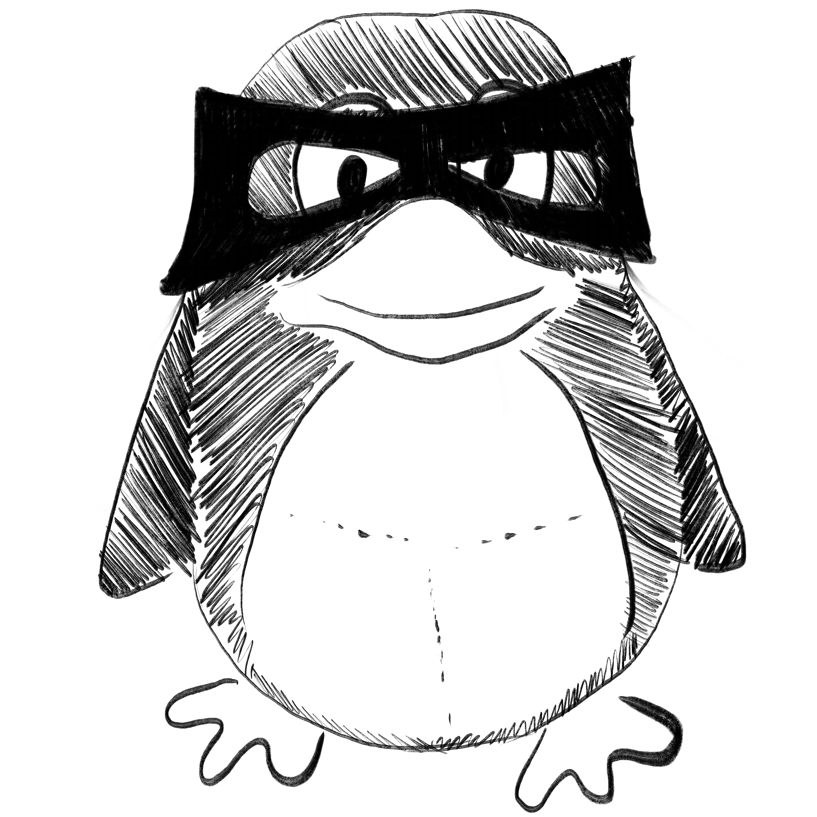
Does noncompliance with COVID-19 regulations impact the depressive symptoms of others?
In Economic modelling
Oyenubi Adeola, Kollamparambil Umakrishnan
2023-Mar
Causal inference, Double machine learning, Mental health, Negative externality, South Africa
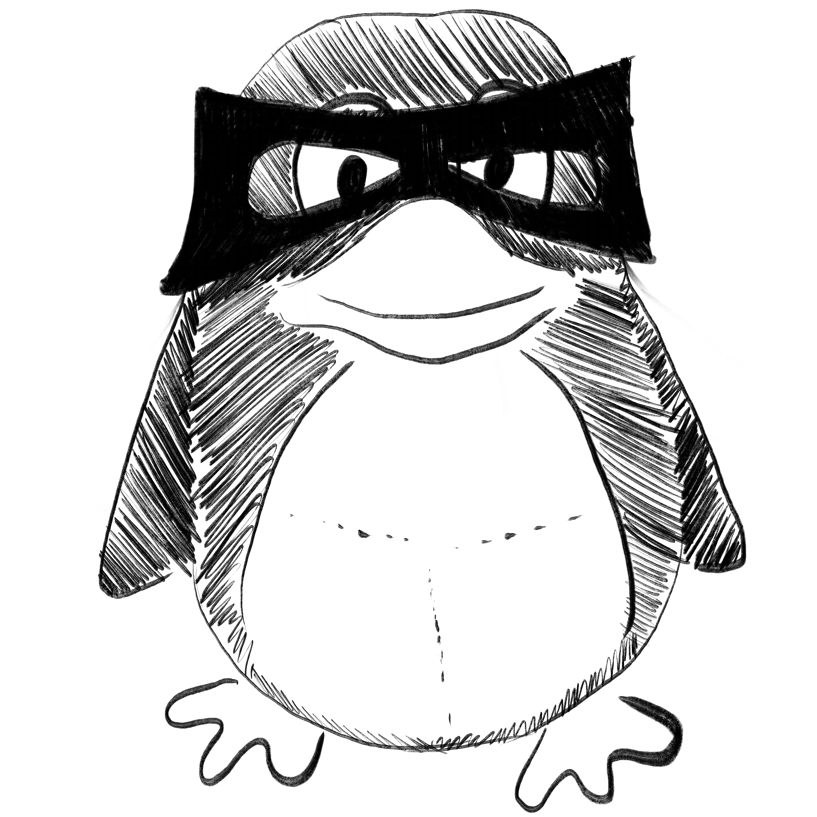
Independent regulation of Z-lines and M-linesduring sarcomere assembly in cardiac myocytesrevealed by the automatic image analysis software sarcApp
bioRxiv Preprint
Neininger-Castro, A. C.; Hayes, J. B.; Sanchez, Z. C.; Taneja, N.; Fenix, A. M.; Moparthi, S.; Vassilopoulos, S.; Burnette, D. T.
2023-01-12
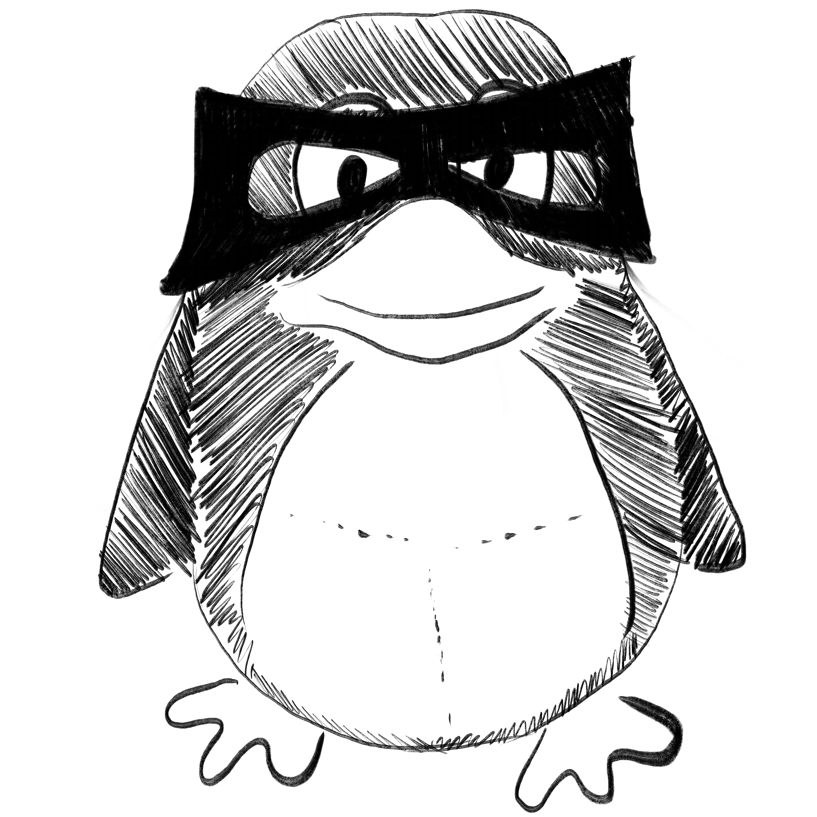
Coronavirus covid-19 detection by means of explainable deep learning.
In Scientific reports ; h5-index 158.0
Mercaldo Francesco, Belfiore Maria Paola, Reginelli Alfonso, Brunese Luca, Santone Antonella
2023-Jan-10
Innovations in Public Health Surveillance for Emerging Infections.
In Annual review of public health
Jia Peng, Liu Shiyong, Yang Shujuan
2022-Jan-10
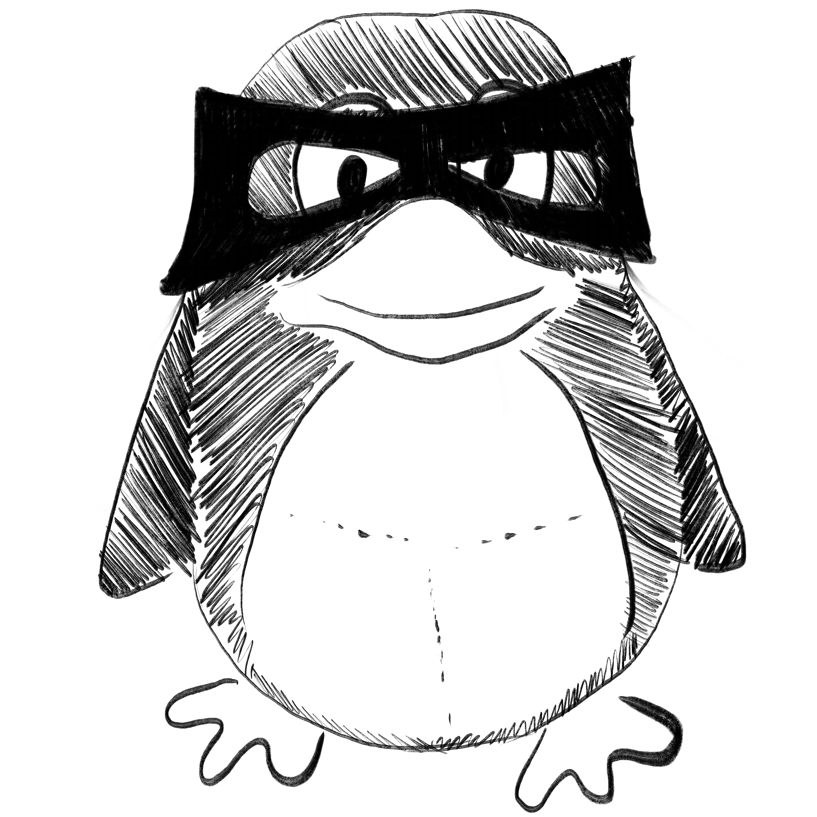
Big Data in Genomic Research for Big Questions with Examples from Covid-19 and Other Zoonoses.
In Journal of applied microbiology
Wassenaar Trudy M, Ussery David W, Rosel Adriana Cabal
2022-Dec-16
Covid-19, Salmonella, big data, genomics, omics, scientific publishing, zoonoses
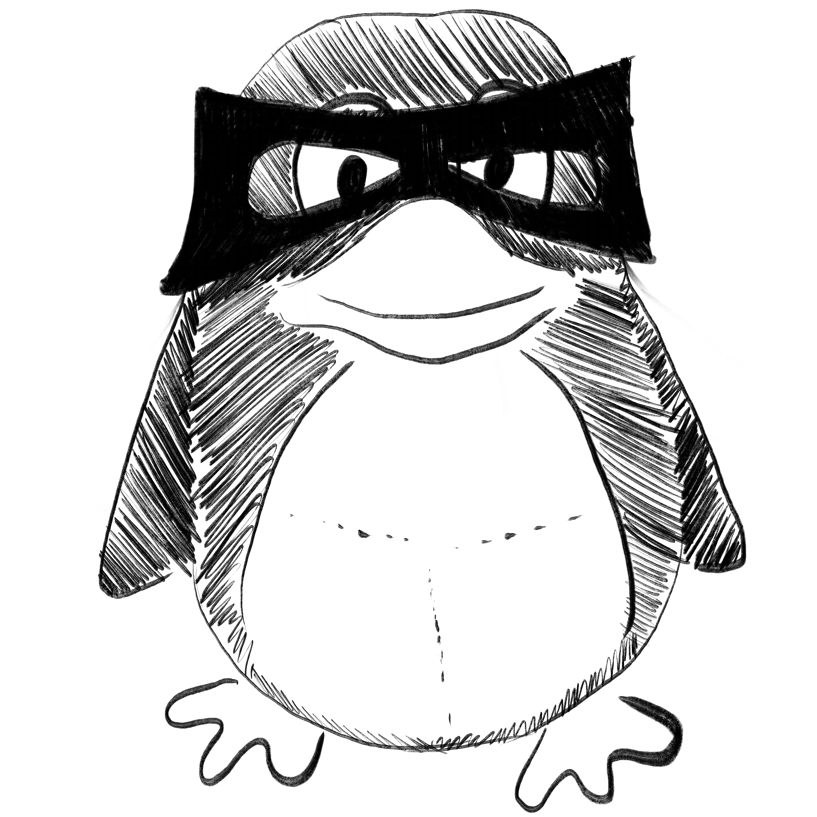
Machine learning-driven blood transcriptome-based discovery of SARS-CoV-2 specific severity biomarkers.
In Journal of medical virology
Krishnamoorthy Pandikannan, Raj Athira S, Kumar Himanshu
2023-Jan-10
Blood biomarker, Machine learning, Meta-analysis, SARS-CoV-2, Transcriptome
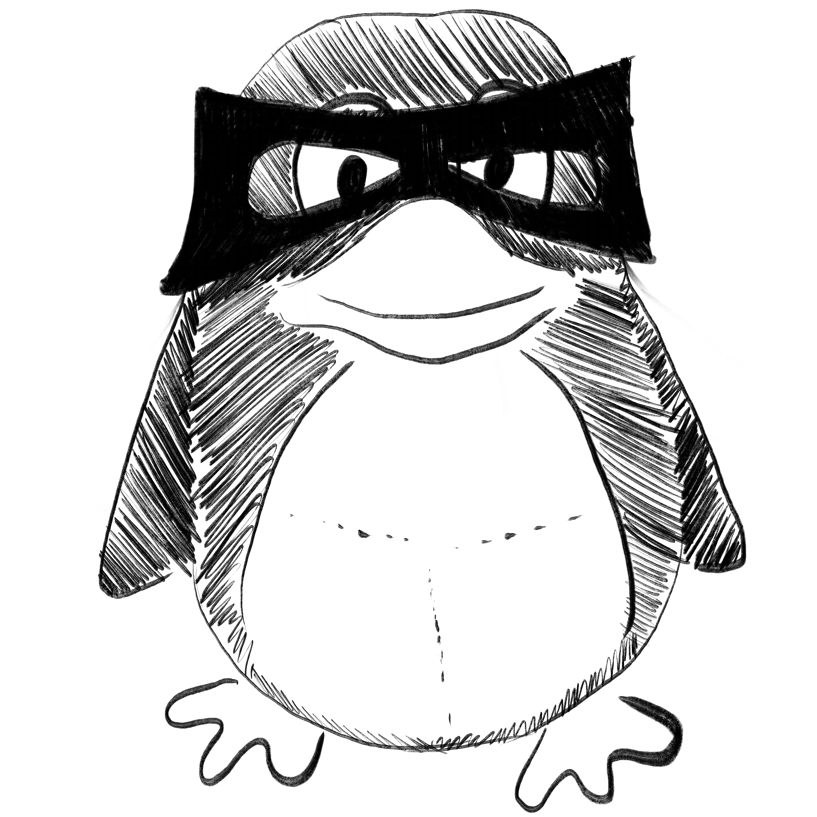
Research on interaction of innovation spillovers in the AI, Fin-Tech, and IoT industries: considering structural changes accelerated by COVID-19.
In Financial innovation
Ho Chi-Ming
2023
AI, Covid-19, Fin-Tech, Innovation spillover, IoT
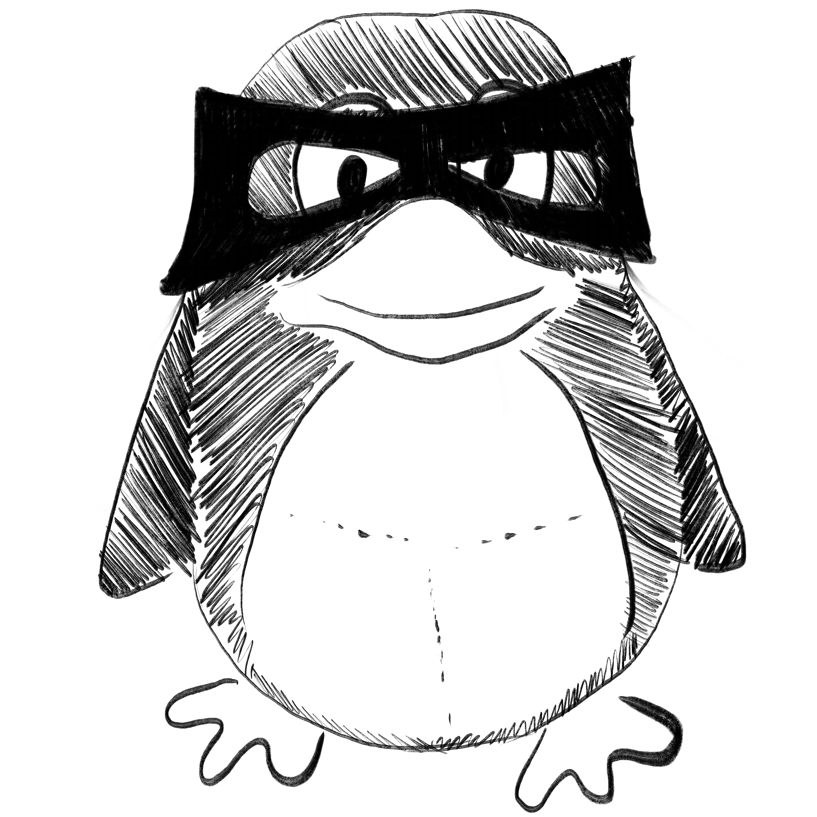
Using knowledge of, attitude toward, and daily preventive practices for COVID-19 to predict the level of post-traumatic stress and vaccine acceptance among adults in Hong Kong.
In Frontiers in psychology ; h5-index 92.0
INTRODUCTION :
METHODS :
RESULTS :
DISCUSSION :
Cao Yuan, Siu Judy Yuen-Man, Choi Kup-Sze, Ho Nick Cho-Lik, Wong Kai Chun, Shum David H K
2022
COVID-19, KAP, PTSD, knowledge – attitude – behavior, vaccine
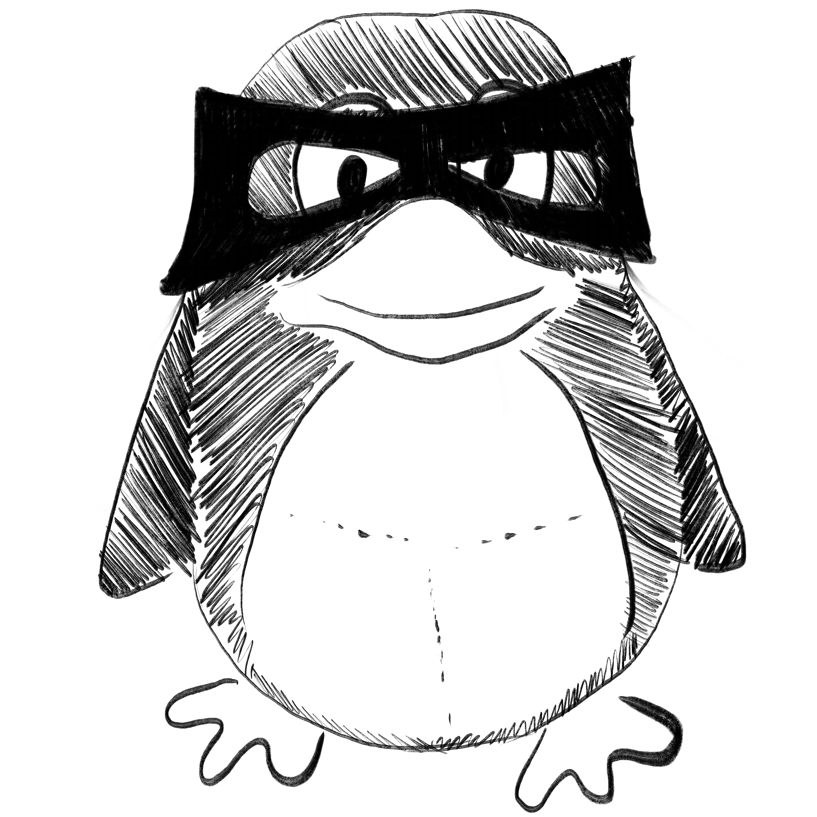
Drug repositioning based on heterogeneous networks and variational graph autoencoders.
In Frontiers in pharmacology
Lei Song, Lei Xiujuan, Liu Lian
2022
COVID-19, drug repositioning, graph representation learning, heterogeneous network, variational graph autoencoders
Swin-textural: A novel textural features-based image classification model for COVID-19 detection on chest computed tomography.
In Informatics in medicine unlocked
BACKGROUND :
MATERIAL AND METHOD :
RESULTS :
CONCLUSIONS :
Tuncer Ilknur, Barua Prabal Datta, Dogan Sengul, Baygin Mehmet, Tuncer Turker, Tan Ru-San, Yeong Chai Hong, Acharya U Rajendra
2023
COVID-19 classification, Computed tomography images, Swin, Swin-textural, Textural feature extraction
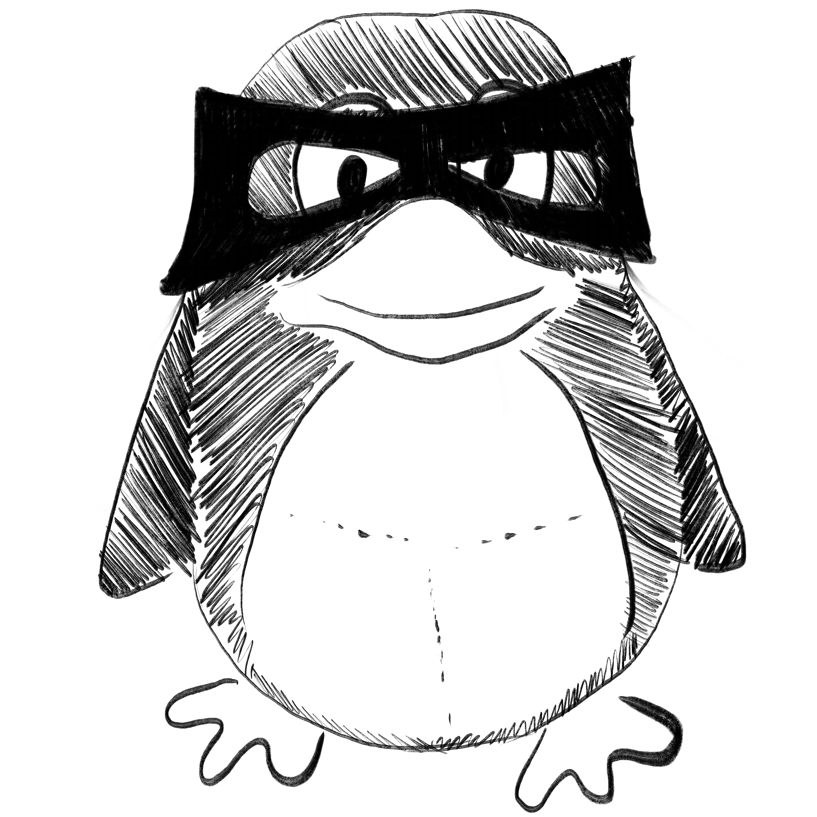
Cluster-based text mining for extracting drug candidates for the prevention of COVID-19 from the biomedical literature.
In Journal of Taibah University Medical Sciences
OBJECTIVE :
METHODS :
RESULTS :
CONCLUSIONS :
Supianto Ahmad Afif, Nurdiansyah Rizky, Weng Chia-Wei, Zilvan Vicky, Yuwana Raden Sandra, Arisal Andria, Pardede Hilman Ferdinandus, Lee Min-Min, Huang Chien-Hung, Ng Ka-Lok
2023-Jan-04
COVID-19, Coronavirus, Drug docking, Phytochemicals, SARS-CoV-2, Text mining
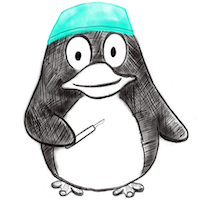
Literature analysis of artificial intelligence in biomedicine.
In Annals of translational medicine
Hulsen Tim
2022-Dec
Artificial intelligence (AI), Embase, PubMed, biomedicine, deep learning (DL), healthcare, literature, machine learning (ML), medicine, neural networks (NNs)
Predictive model for BNT162b2 vaccine response in cancer patients based on blood cytokines and growth factors.
In Frontiers in immunology ; h5-index 100.0
BACKGROUND :
METHODS :
RESULTS :
CONCLUSION :
Konnova Angelina, De Winter Fien H R, Gupta Akshita, Verbruggen Lise, Hotterbeekx An, Berkell Matilda, Teuwen Laure-Anne, Vanhoutte Greetje, Peeters Bart, Raats Silke, der Massen Isolde Van, De Keersmaecker Sven, Debie Yana, Huizing Manon, Pannus Pieter, Neven Kristof Y, Ariën Kevin K, Martens Geert A, Bulcke Marc Van Den, Roelant Ella, Desombere Isabelle, Anguille Sébastien, Berneman Zwi, Goossens Maria E, Goossens Herman, Malhotra-Kumar Surbhi, Tacconelli Evelina, Vandamme Timon, Peeters Marc, van Dam Peter, Kumar-Singh Samir
2022
BNT162b2, COVID-19 vaccine, SARS-CoV-2, chemokines, cytokines, growth factors, haematological malignancies, solid cancers
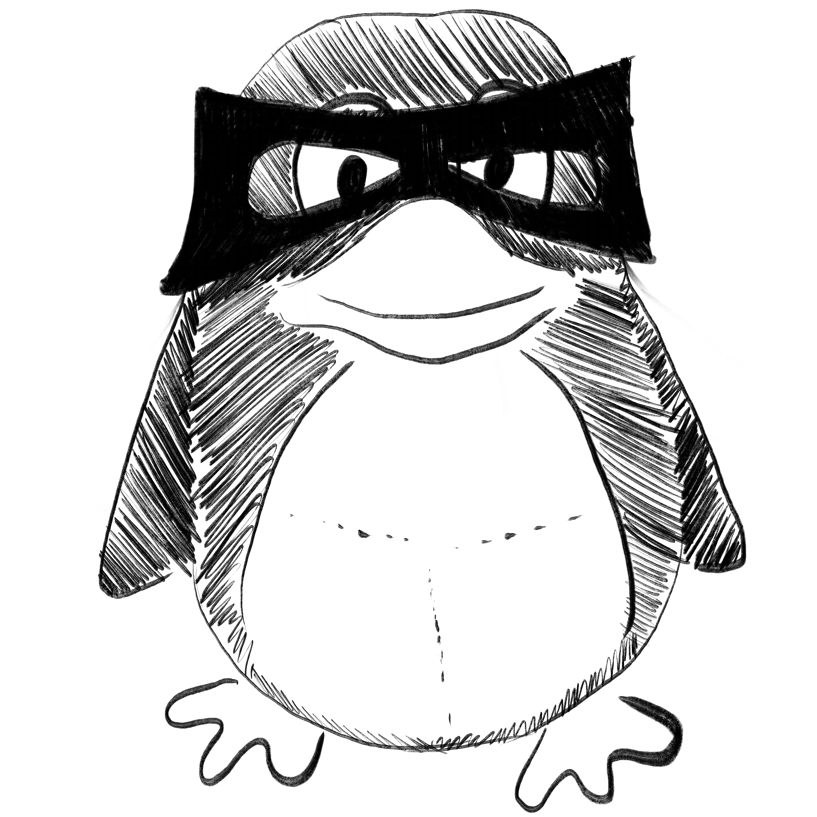
D3SENet: A hybrid deep feature extraction network for Covid-19 classification using chest X-ray images.
In Biomedical signal processing and control
Kaya Mustafa, Eris Mustafa
2023-Apr
Covid-19, Deep Ensemble Network, Deep Learning, Machine Learning, Medical Image Processing
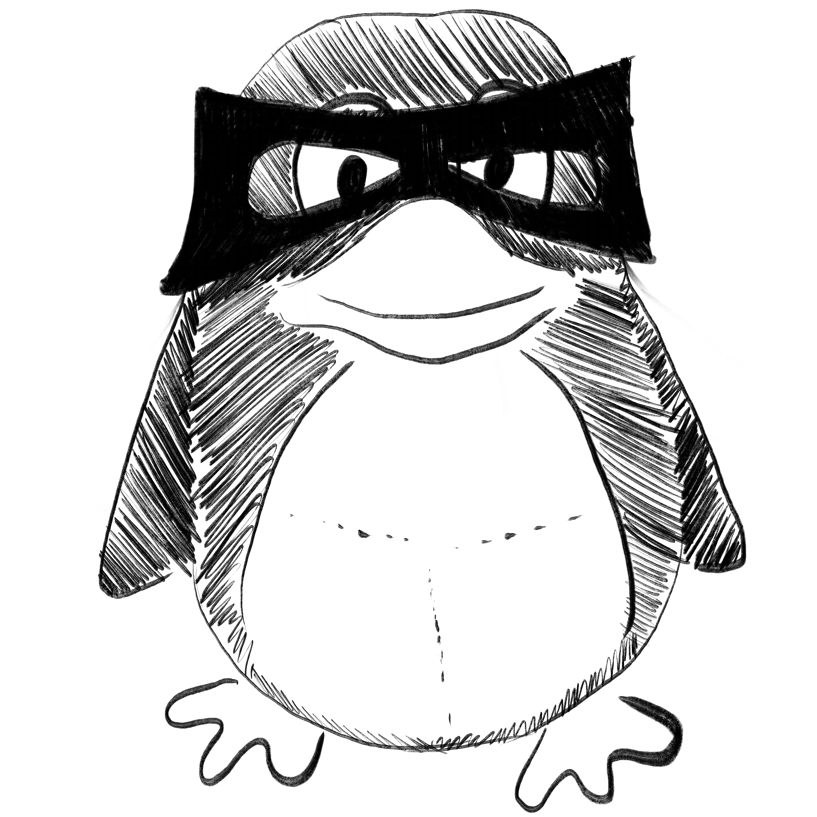
Locating frontline workers' position up against COVID-19.
In Journal of family medicine and primary care
Gupta Harish
2022-Oct
Artificial Intelligence, essential service providers, frontline workers, health-care professionals, modern warfare
A demonstration of Modified Treatment Policies to evaluate shifts in mobility and COVID-19 case rates in U.S. counties.
In American journal of epidemiology ; h5-index 65.0
Nugent Joshua R, Balzer Laura B
2023-Jan-09
COVID-19 Pandemic, Machine Learning, modified treatment policy, targeted learning
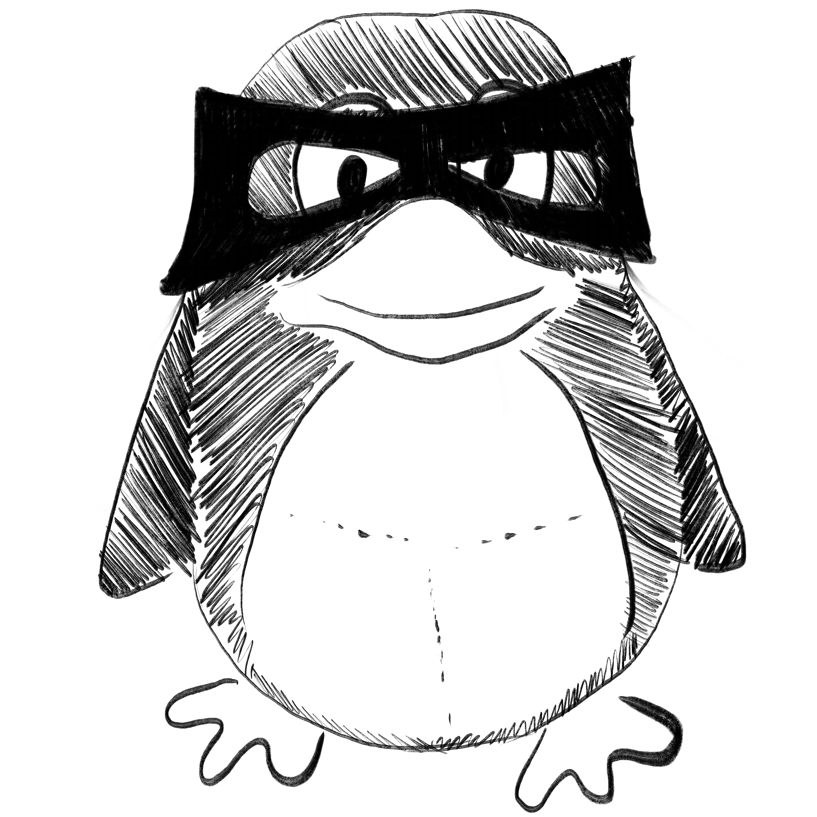
Intelligent speech technologies for transcription, disease diagnosis, and medical equipment interactive control in smart hospitals: A review.
In Computers in biology and medicine
Zhang Jun, Wu Jingyue, Qiu Yiyi, Song Aiguo, Li Weifeng, Li Xin, Liu Yecheng
2023-Jan-05
Automatic speech recognition, Diagnosis, Human-computer interaction, Machine learning, Smart hospital, Transcription
The Use of Digital Technology for COVID-19 Detection, and Response Management in Indonesia: A Mixed Methods Study.
In Interactive journal of medical research
BACKGROUND :
OBJECTIVE :
METHODS :
RESULTS :
CONCLUSIONS :
Nur Aisyah Dewi, Lokopessy Alfiano Fawwaz, Naman Maryan, Diva Haniena, Manikam Logan, Adisasmito Wiku, Kozlakidis Zisis
2023-Jan-09
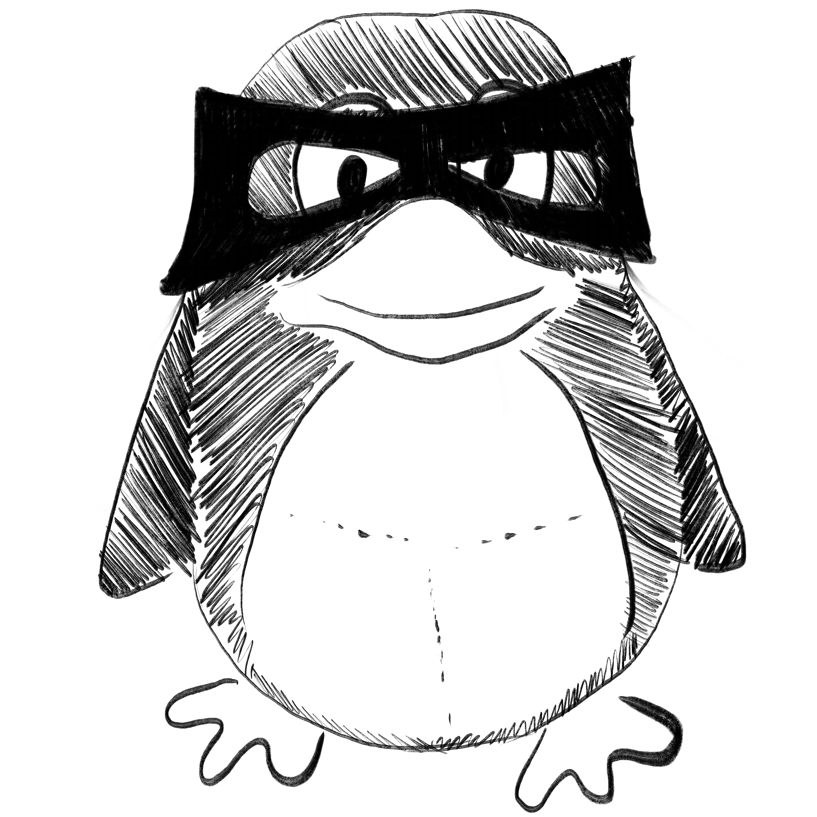
Deep Learning Models for Multiple Face Mask Detection under a Complex Big Data Environment.
In Procedia computer science
Rekha V, Manoharan J Samuel, Hemalatha R, Saravanan D
2022
Big Data, Complex Data analytics, Deep Learning models, Face Mask Detection, Multi – Sensor Data Acquisition
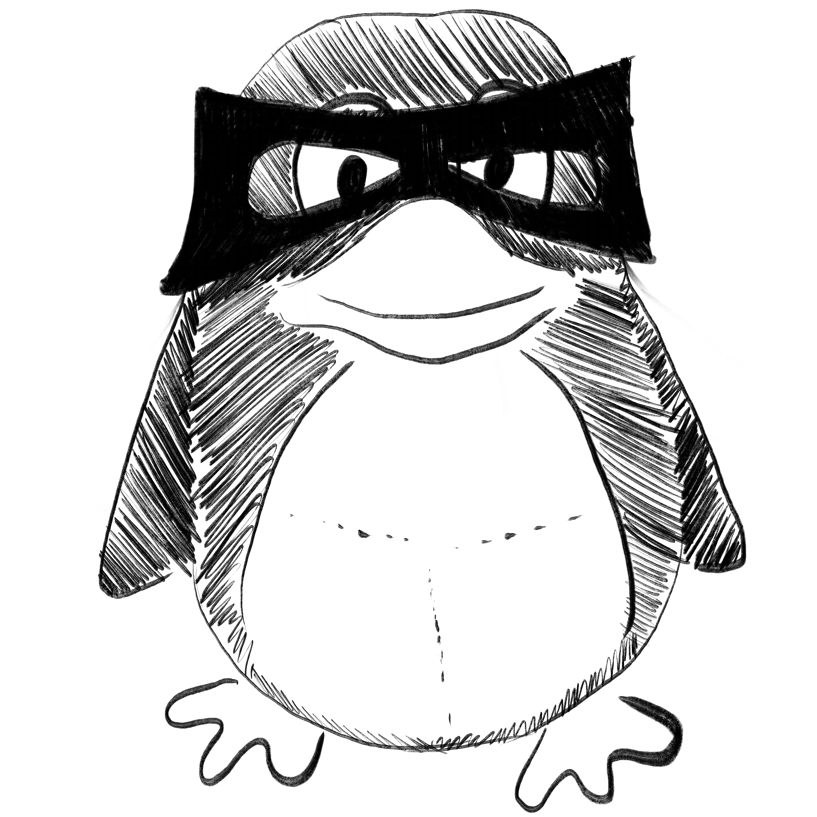
A fuzzy fine-tuned model for COVID-19 diagnosis.
In Computers in biology and medicine
Esmi Nima, Golshan Yasaman, Asadi Sara, Shahbahrami Asadollah, Gaydadjiev Georgi
2023-Jan-04
Blind/Referenceless image spatial quality evaluator, COVID-19, Deep learning, Fuzzy pooling, Weighted multi-class cross-entropy
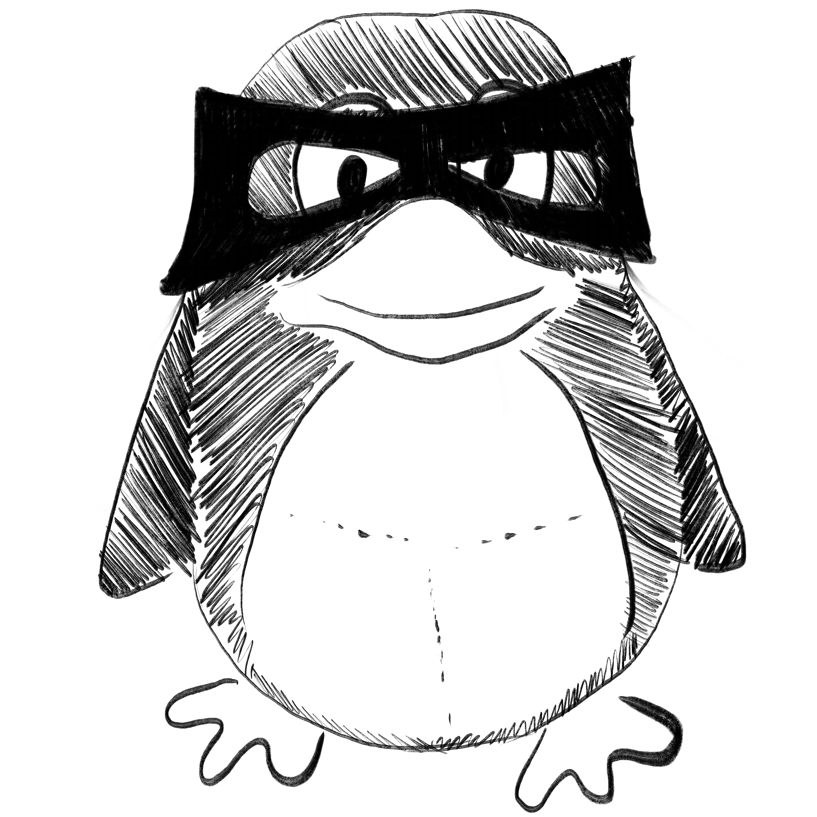
Disease X vaccine production and supply chains: risk assessing healthcare systems operating with artificial intelligence and industry 4.0.
In Health and technology
OBJECTIVE :
METHOD :
RESULTS :
CONCLUSION :
Radanliev Petar, De Roure David
2023-Jan-04
Artificial intelligence, Disease X, Healthcare systems, Industry 4.0, Risk assessment, Vaccine production and supply chains
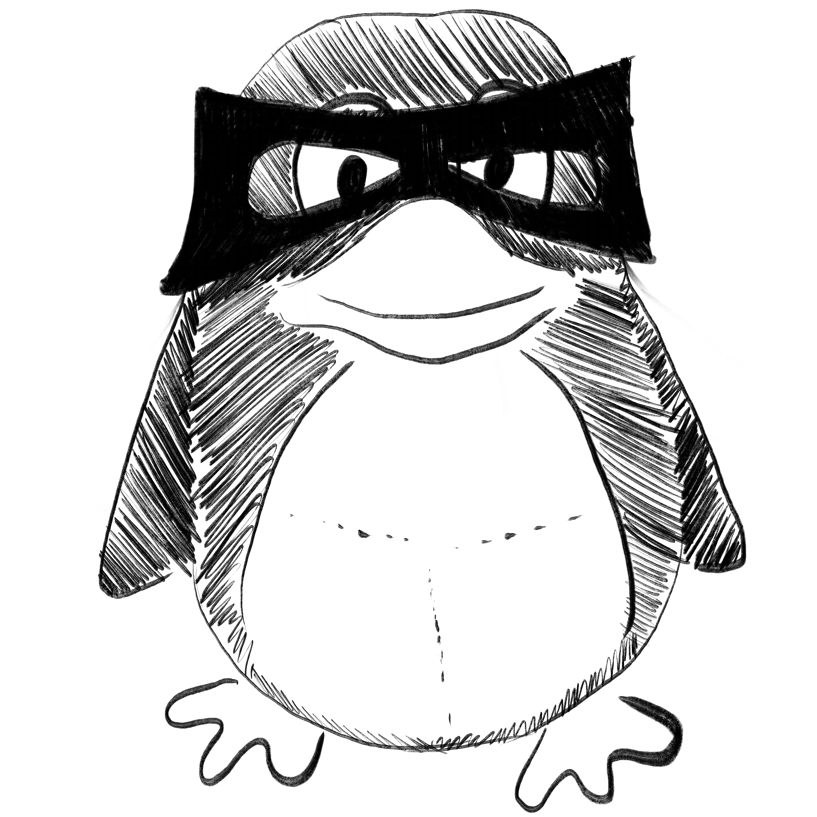
An Optimized Deep Learning Approach for the Prediction of Social Distance Among Individuals in Public Places During Pandemic.
In New generation computing
Sahoo Santosh Kumar, Palai G, Altahan Baraa Riyadh, Ahammad Sk Hasane, Priya P Poorna, Hossain Md Amzad, Rashed Ahmed Nabih Zaki
2023-Jan-02
Deep learning, Detectron2, Intersection over union, Object detection, Social distancing
A measurement method for mental health based on dynamic multimodal feature recognition.
In Frontiers in public health
INTRODUCTION :
METHODS :
RESULTS :
CONCLUSION :
Xu Haibo, Wu Xiang, Liu Xin
2022
deep learning, emotion recognition, interactive assessment scale, mental health assessment, video feature extraction
Artificial intelligence-assisted multistrategy image enhancement of chest X-rays for COVID-19 classification.
In Quantitative imaging in medicine and surgery
BACKGROUND :
METHODS :
RESULTS :
CONCLUSIONS :
Sun Hongfei, Ren Ge, Teng Xinzhi, Song Liming, Li Kang, Yang Jianhua, Hu Xiaofei, Zhan Yuefu, Wan Shiu Bun Nelson, Wong Man Fung Esther, Chan King Kwong, Tsang Hoi Ching Hailey, Xu Lu, Wu Tak Chiu, Kong Feng-Ming Spring, Wang Yi Xiang J, Qin Jing, Chan Wing Chi Lawrence, Ying Michael, Cai Jing
2023-Jan-01
Coronavirus disease 2019 (COVID-19), bone suppression, chest X-ray (CXR), super-resolution
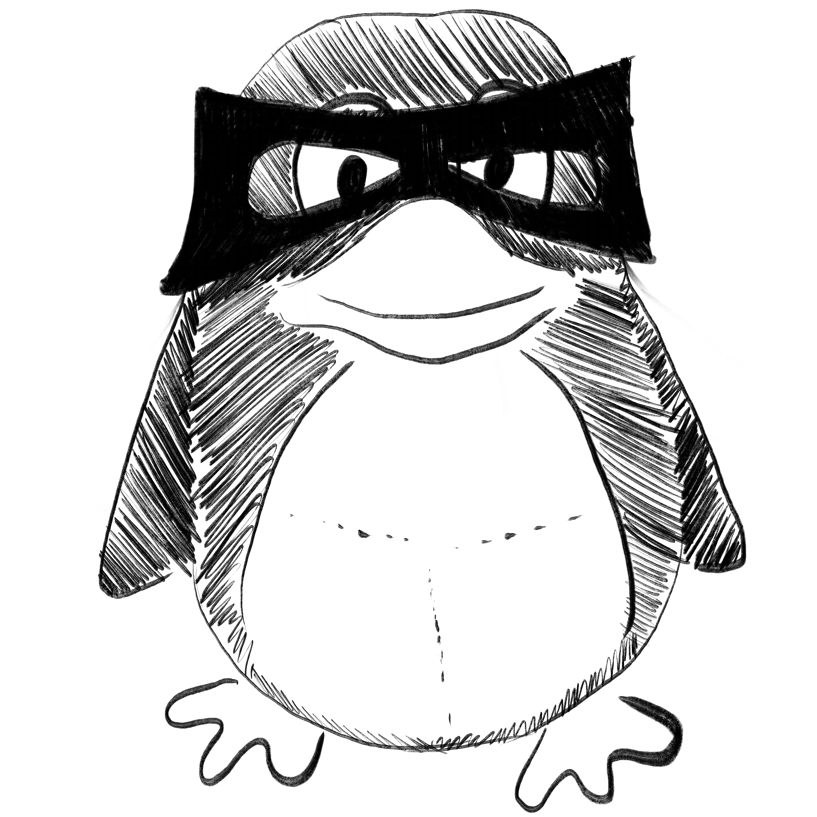
Genomic landscape of the SARS-CoV-2 pandemic in Brazil suggests an external P.1 variant origin.
In Frontiers in microbiology
Perico Camila P, De Pierri Camilla R, Neto Giuseppe Pasqualato, Fernandes Danrley R, Pedrosa Fabio O, de Souza Emanuel M, Raittz Roberto T
2022
SWeeP, big data, diversity, genomics and proteomics, machine learning, virus
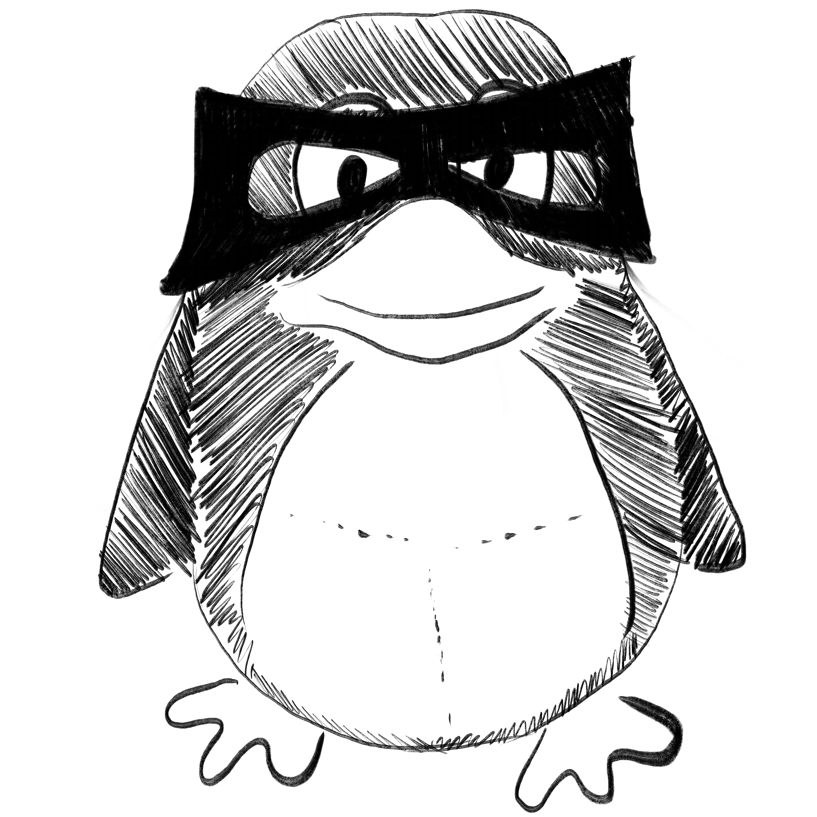
Multi-verse Optimizer with Rosenbrock and Diffusion Mechanisms for Multilevel Threshold Image Segmentation from COVID-19 Chest X-Ray Images.
In Journal of bionic engineering
Han Yan, Chen Weibin, Heidari Ali Asghar, Chen Huiling
2023-Jan-04
Bionic algorithm, COVID-19, Kapur’s entropy, Meta-heuristic algorithm, Multi-verse optimizer, Multilevel threshold image segmentation
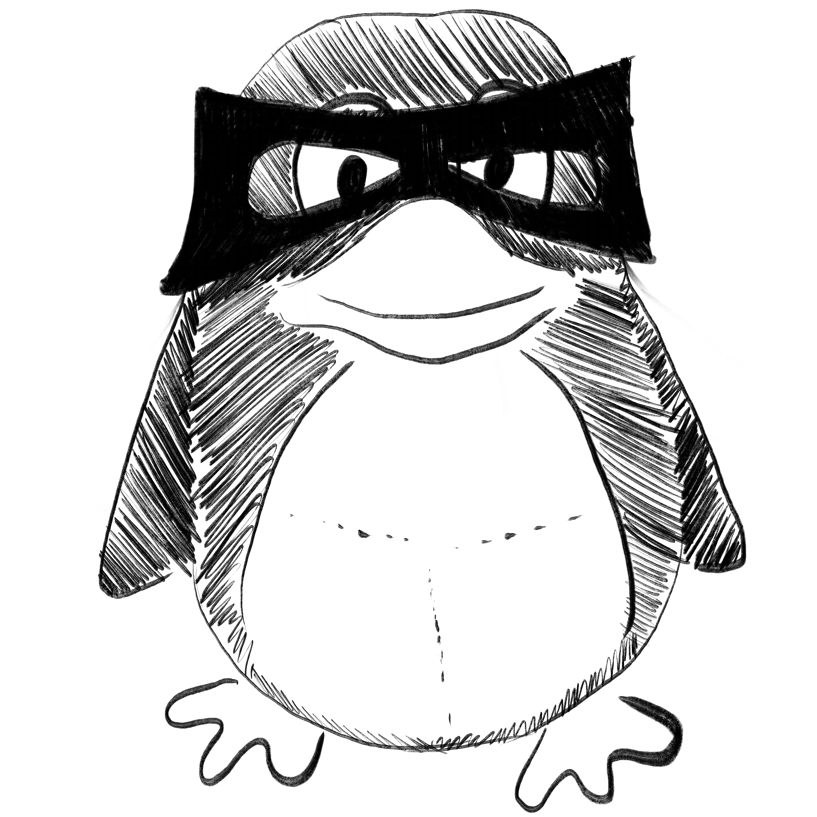
Integration of improved YOLOv5 for face mask detector and auto-labeling to generate dataset for fighting against COVID-19.
In The Journal of supercomputing
Pham Thi-Ngot, Nguyen Viet-Hoan, Huh Jun-Ho
2023-Jan-03
Auto-labeling, COVID-19, Coordinate attention, Deep learning, Face mask detection, YOLO, YOLOv5, You Only Look One
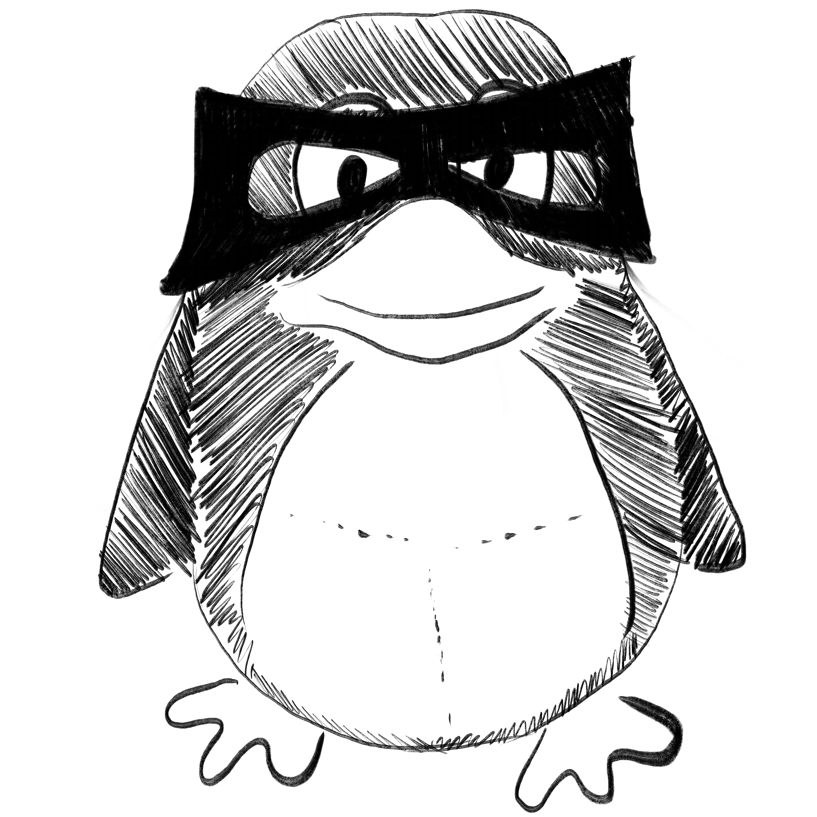
RADIC:A tool for diagnosing COVID-19 from chest CT and X-ray scans using deep learning and quad-radiomics.
In Chemometrics and intelligent laboratory systems : an international journal sponsored by the Chemometrics Society
Attallah Omneya
2023-Feb-15
COVID-19, Convolution neural networks (CNN), Deep learning, Discrete wavelet transform, Dual-tree complex wavelet transform, Texture analysis
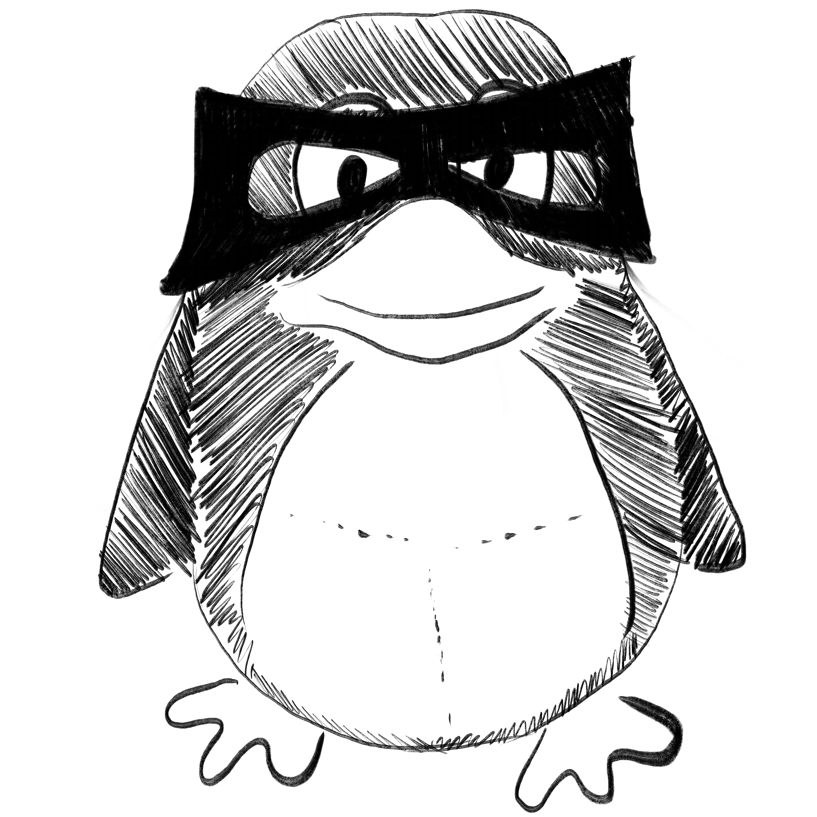
MTSS-AAE: Multi-task semi-supervised adversarial autoencoding for COVID-19 detection based on chest X-ray images.
In Expert systems with applications
Ullah Zahid, Usman Muhammad, Gwak Jeonghwan
2023-Apr-15
COVID-19, Deep learning, Multi-task learning, Representation learning, Semi-supervised adversarial learning
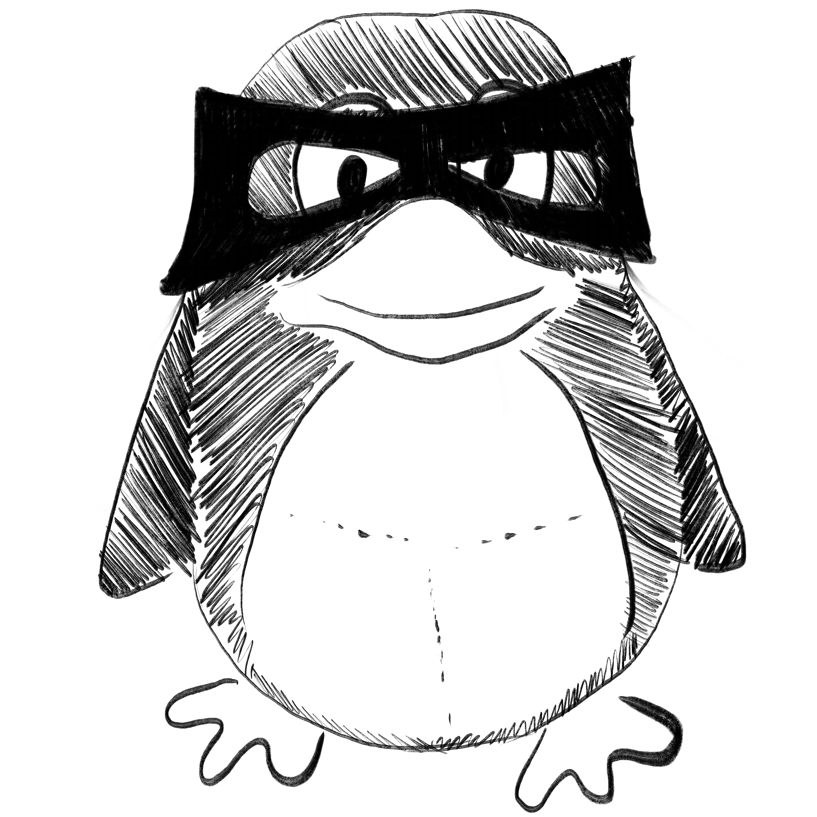
Critical review of conformational B-cell epitope prediction methods.
In Briefings in bioinformatics
Cia Gabriel, Pucci Fabrizio, Rooman Marianne
2023-Jan-05
Antibody-specific epitope prediction, Benchmarking, Conformational B-cell epitope prediction, Immunoinformatics
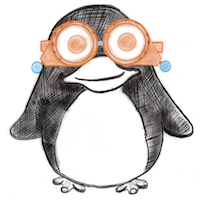
Can Tele-Neuro-Ophthalmology Be Useful Beyond the Pandemic?
In Current neurology and neuroscience reports
PURPOSE OF THE REVIEW :
RECENT FINDINGS :
Lai Kevin E, Ko Melissa W
2023-Jan-07
Artificial intelligence, Augmented reality, Neuro-ophthalmology, Pandemic, Telehealth, Telemedicine
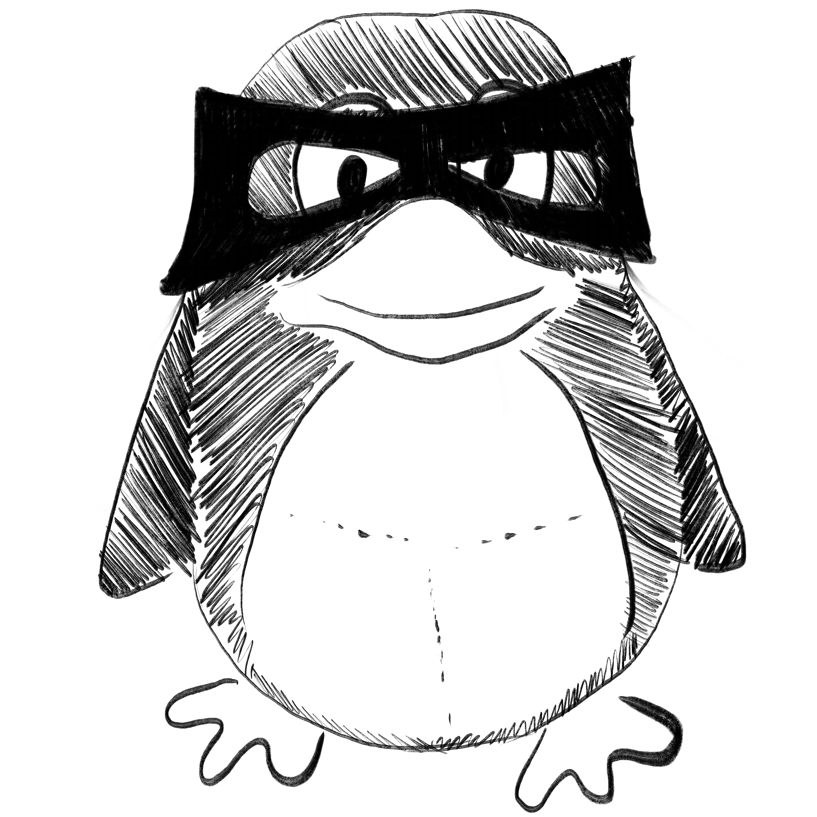
Authentication of Covid-19 Vaccines Using Synchronous Fluorescence Spectroscopy.
In Journal of fluorescence
Assi Sulaf, Abbas Ismail, Arafat Basel, Evans Kieran, Al-Jumeily Dhiya
2023-Jan-07
Covid-19, Gaussian mixture models, Principal component analysis, Self organising maps, Synchronous fluorescence, Vaccines
Machine learning to analyse omic-data for COVID-19 diagnosis and prognosis.
In BMC bioinformatics
BACKGROUND :
RESULTS :
CONCLUSIONS :
Liu Xuehan, Hasan Md Rakibul, Ahmed Khandaker Asif, Hossain Md Zakir
2023-Jan-06
Autoencoder, COVID-19 diagnosis, Machine learning, Multi-omics, Severity
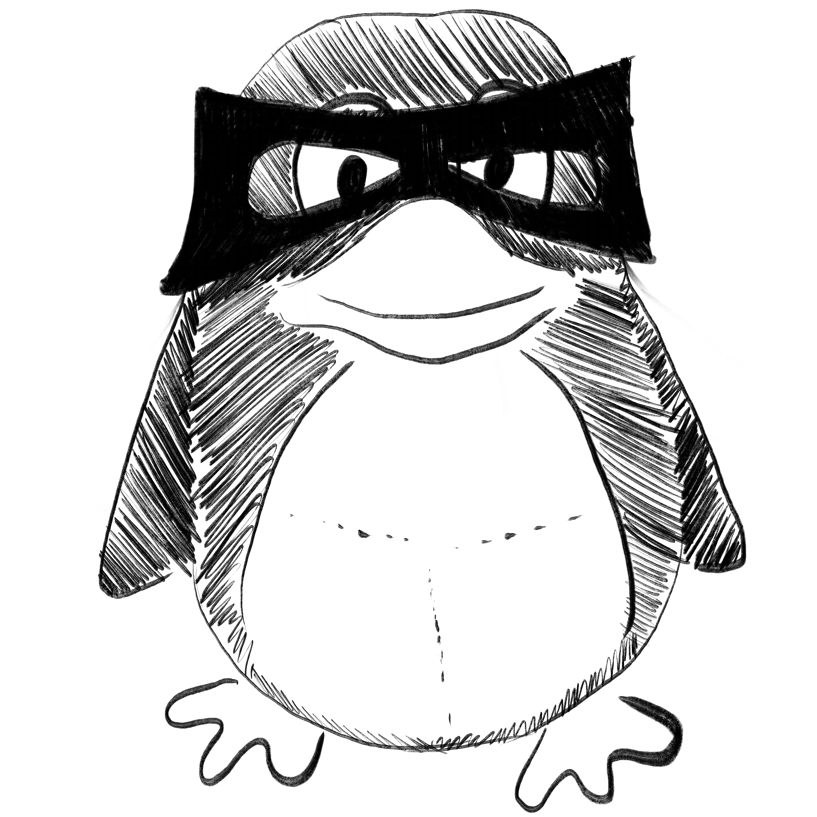
Nurses' Work Concerns and Disenchantment during the COVID-19 Pandemic: Machine Learning Analysis of Online Discussions.
In JMIR nursing
BACKGROUND :
OBJECTIVE :
METHODS :
RESULTS :
CONCLUSIONS :
Jiang Haoqiang, Castellanos Arturo, Castillo Alfred, Gomes Paulo J, Li Juanjuan, VanderMeer Debra
2023-Jan-03
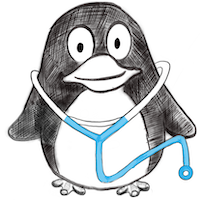
Severe COVID-19 Infection in Type 1 and Type 2 Diabetes During the First Three Waves in Sweden.
In Diabetes care ; h5-index 125.0
OBJECTIVE :
RESEARCH DESIGN AND METHODS :
RESULTS :
CONCLUSIONS :
Edqvist Jon, Lundberg Christina, Andreasson Karin, Björck Lena, Dikaiou Pigi, Ludvigsson Johnny, Lind Marcus, Adiels Martin, Rosengren Annika
2023-Jan-06
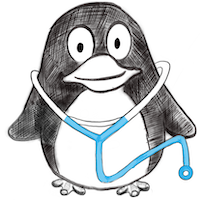
The 2000HIV study: Design, multi-omics methods and participant characteristics.
In Frontiers in immunology ; h5-index 100.0
BACKGROUND :
METHODS :
RESULTS :
CONCLUSION :
Vos Wilhelm A J W, Groenendijk Albert L, Blaauw Marc J T, van Eekeren Louise E, Navas Adriana, Cleophas Maartje C P, Vadaq Nadira, Matzaraki Vasiliki, Dos Santos Jéssica C, Meeder Elise M G, Fröberg Janeri, Weijers Gert, Zhang Yue, Fu Jingyuan, Ter Horst Rob, Bock Christoph, Knoll Rainer, Aschenbrenner Anna C, Schultze Joachim, Vanderkerckhove Linos, Hwandih Talent, Wonderlich Elizabeth R, Vemula Sai V, van der Kolk Mike, de Vet Sterre C P, Blok Willem L, Brinkman Kees, Rokx Casper, Schellekens Arnt F A, de Mast Quirijn, Joosten Leo A B, Berrevoets Marvin A H, Stalenhoef Janneke E, Verbon Annelies, van Lunzen Jan, Netea Mihai G, van der Ven Andre J A M
2022
COVID-19, HIV extreme phenotype, HIV reservoir, HIV-1, cardiovascular disease, hepatic disease, multi-omics, non-AIDS comorbidities
Improved predictions of antigen presentation and TCR recognition with MixMHCpred2.2 and PRIME2.0 reveal potent SARS-CoV-2 CD8+ T-cell epitopes.
In Cell systems
Gfeller David, Schmidt Julien, Croce Giancarlo, Guillaume Philippe, Bobisse Sara, Genolet Raphael, Queiroz Lise, Cesbron Julien, Racle Julien, Harari Alexandre
2022-Dec-23
CD8(+) T cell epitopes, HLA-I peptidomics, antigen presentation, computational biology, epitope predictions, immunology, machine learning
Analysis and forecasting of air quality index based on satellite data.
In Inhalation toxicology
OBJECTIVE :
MATERIALS AND METHODS :
RESULTS :
CONCLUSIONS :
Singh Tinku, Sharma Nikhil, Satakshi Kumar
2023-Jan-05
Google Earth Engine (GEE), beta distribution, pollutants, remote sensing, satellite data
Development and validation of a deep learning model to diagnose COVID-19 using time-series heart rate values before the onset of symptoms.
In Journal of medical virology
Chung Heewon, Ko Hoon, Lee Hooseok, Yon Dong Keon, Lee Won Hee, Kim Tae-Seong, Kim Kyung Won, Lee Jinseok
2023-Jan-05
COVID-19, deep learning, early diagnosis, heart rate, heart rate variability, smartwatch, transformer model
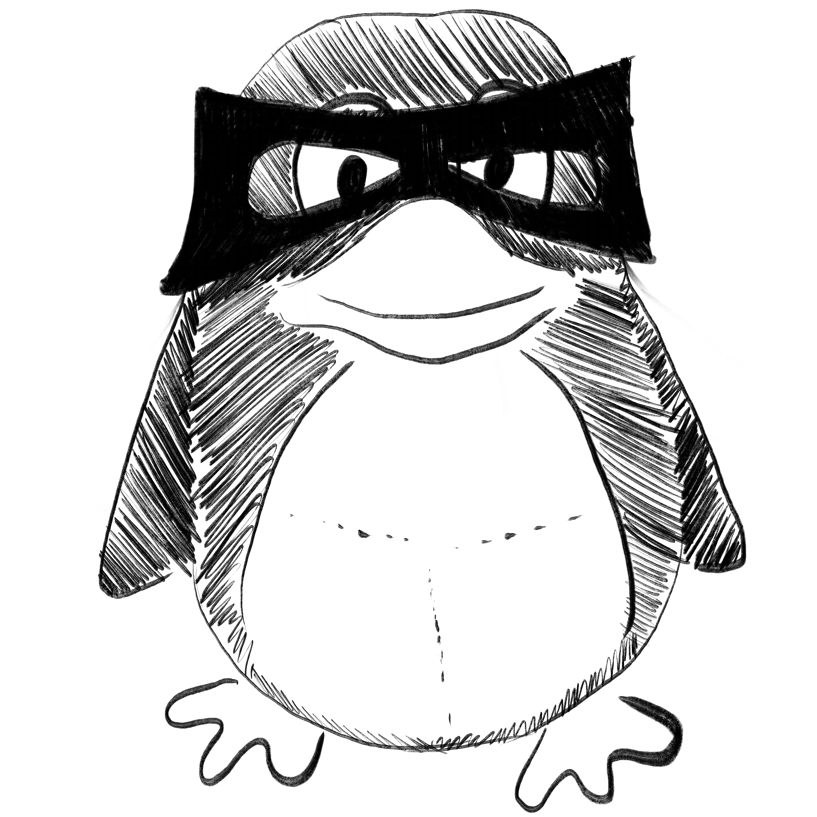
Single-cell multi-omics integration for unpaired data by a siamese network with graph-based contrastive loss.
In BMC bioinformatics
BACKGROUND :
RESULTS :
CONCLUSIONS :
Liu Chaozhong, Wang Linhua, Liu Zhandong
2023-Jan-04
COVID-19, Data integration, Deep learning, Single-cell sequencing analysis
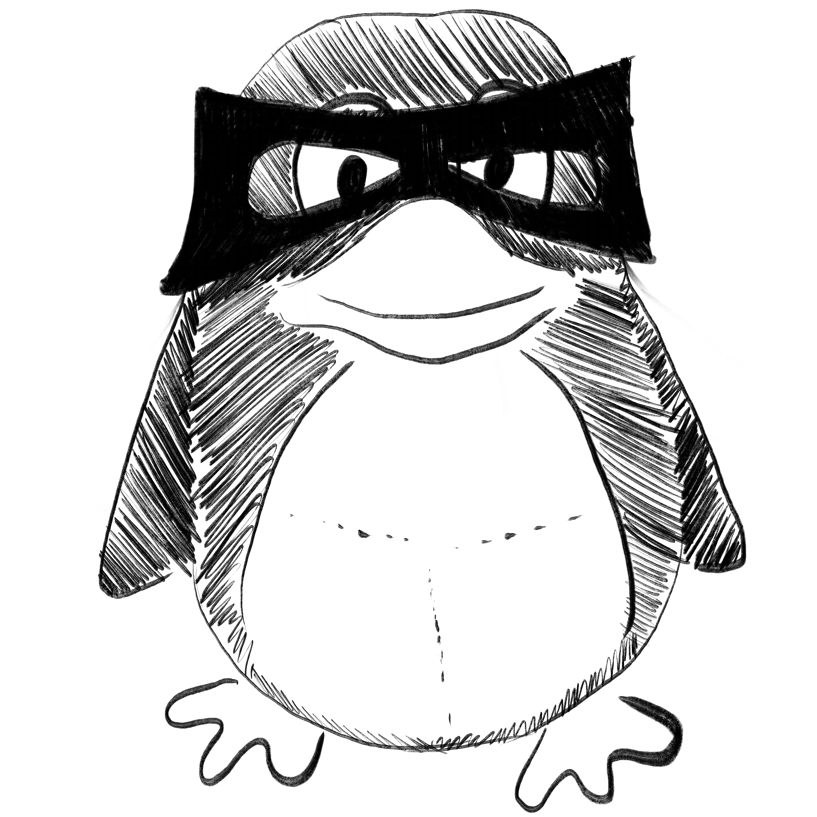
Neurophenotypes of COVID-19: risk factors and recovery trajectories.
In Research square
Prabhakaran Divya, Day Gregory, Munipalli Bala, Rush Beth, Pudalov Lauren, Niazi Shehzad, Brennan Emily, Powers Harry, Durvasula Ravi, Athreya Arjun, Blackmon Karen
2022-Dec-21
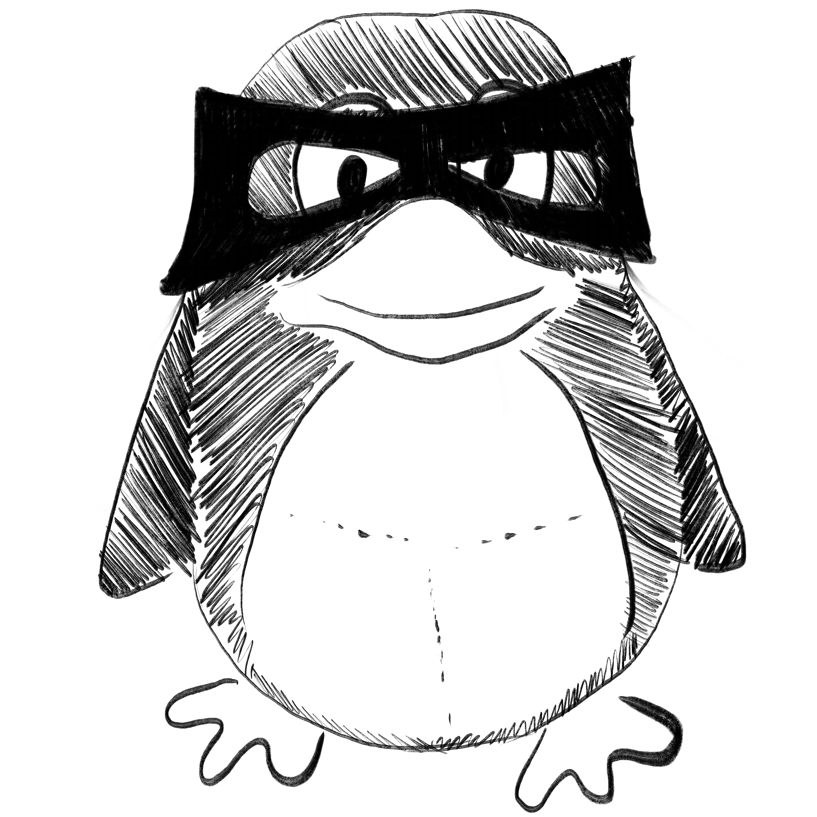
A machine learning-based phenotype for long COVID in children: an EHR-based study from the RECOVER program.
In medRxiv : the preprint server for health sciences
BACKGROUND :
METHODS AND FINDINGS :
CONCLUSIONS :
FUNDING SOURCE :
DISCLAIMER :
Lorman Vitaly, Razzaghi Hanieh, Song Xing, Morse Keith, Utidjian Levon, Allen Andrea J, Rao Suchitra, Rogerson Colin, Bennett Tellen D, Morizono Hiroki, Eckrich Daniel, Jhaveri Ravi, Huang Yungui, Ranade Daksha, Pajor Nathan, Lee Grace M, Forrest Christopher B, Bailey L Charles
2022-Dec-26
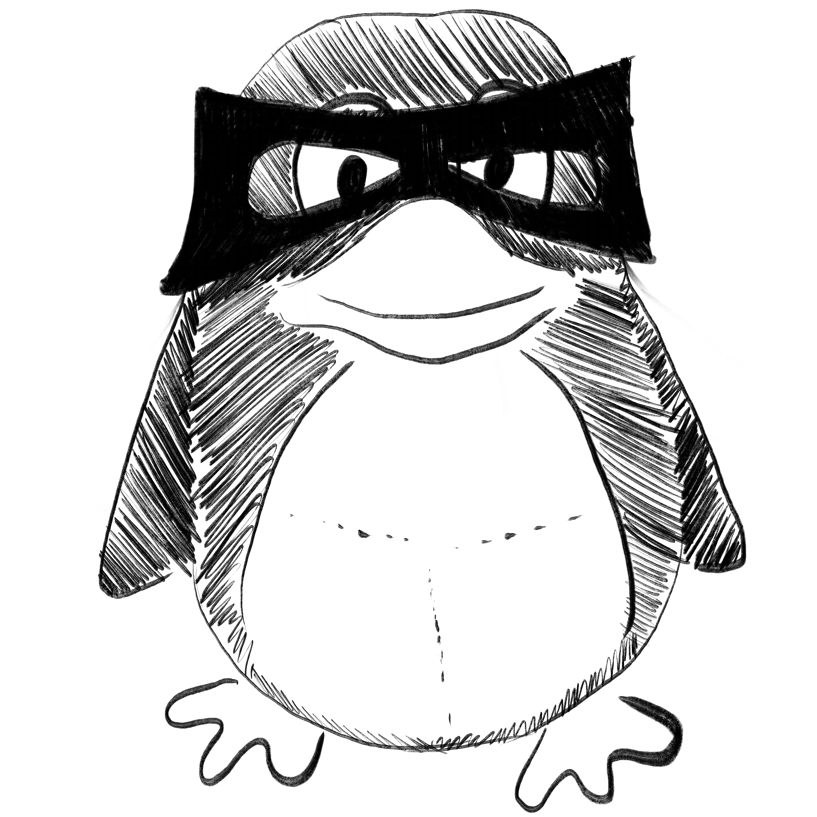
Diagnostic performance of artificial intelligence algorithms for detection of pulmonary involvement by COVID-19 based on portable radiography.
In Medicina clinica (English ed.)
INTRODUCTION AND OBJECTIVES :
MATERIAL AND METHODS :
RESULTS :
CONCLUSION :
Cobeñas Ricardo Luis, de Vedia María, Florez Juan, Jaramillo Daniela, Ferrari Luciana, Re Ricardo
2022-Dec-30
Artificial intelligence, COVID-19, Lung, Machine learning, Pneumonia, Thoracic RX
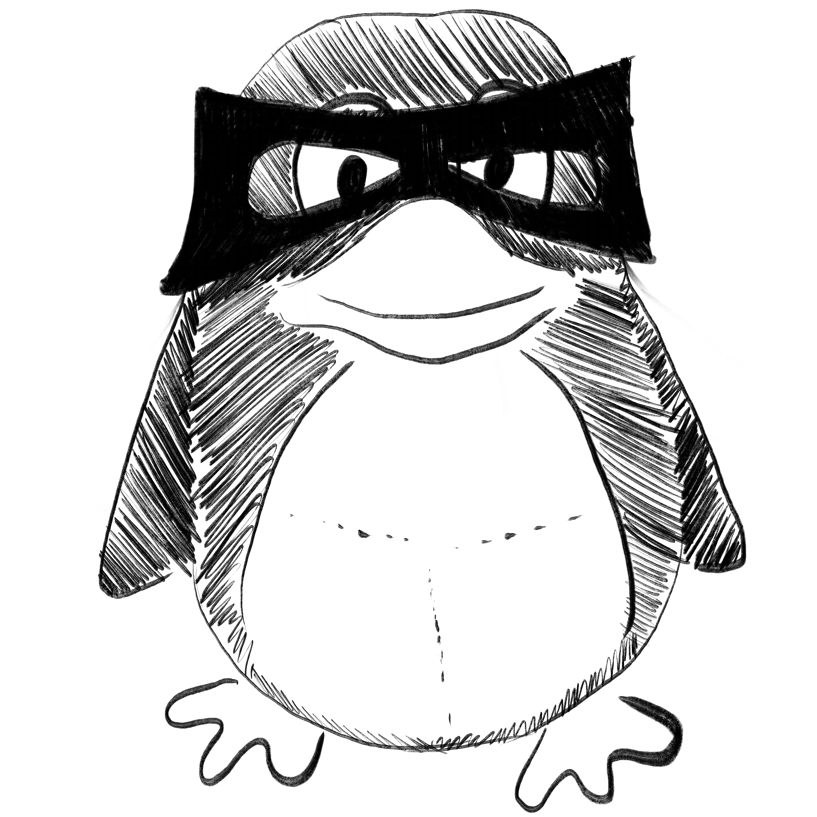
Fill in the blank for fashion complementary outfit product Retrieval: VISUM summer school competition.
In Machine vision and applications
Castro Eduardo, Ferreira Pedro M, Rebelo Ana, Rio-Torto Isabel, Capozzi Leonardo, Ferreira Mafalda Falcão, Gonçalves Tiago, Albuquerque Tomé, Silva Wilson, Afonso Carolina, Gamelas Sousa Ricardo, Cimarelli Claudio, Daoudi Nadia, Moreira Gabriel, Yang Hsiu-Yu, Hrga Ingrid, Ahmad Javed, Keswani Monish, Beco Sofia
2023
Computer vision, Deep learning, Fashion intelligence, Image retrieval, Summer school competition
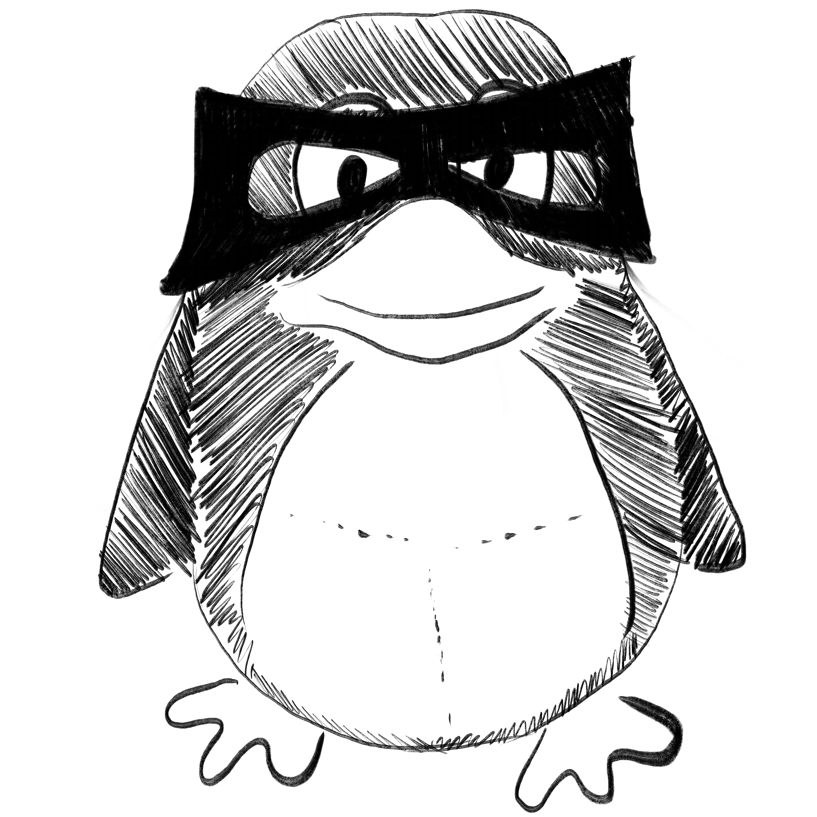
Identification of Asymptomatic COVID-19 Patients on Chest CT Images Using Transformer-Based or Convolutional Neural Network-Based Deep Learning Models.
In Journal of digital imaging
Yin Minyue, Liang Xiaolong, Wang Zilan, Zhou Yijia, He Yu, Xue Yuhan, Gao Jingwen, Lin Jiaxi, Yu Chenyan, Liu Lu, Liu Xiaolin, Xu Chao, Zhu Jinzhou
2023-Jan-03
Asymptomatic coronavirus-disease-2019 patients, Chest CT images, Convolutional neural networks, Deep learning, Transfer learning, Transformer
Cluster analysis of adults unvaccinated for COVID-19 based on behavioral and social factors, National Immunization Survey-Adult COVID Module, United States.
In Preventive medicine ; h5-index 62.0
Meng Lu, Masters Nina B, Lu Peng-Jun, Singleton James A, Kriss Jennifer L, Zhou Tianyi, Weiss Debora, Black Carla L
2022-Dec-31
COVID-19 vaccines, Cluster analysis, Health communication, Health policy, SARS-CoV-2, Vaccine hesitancy
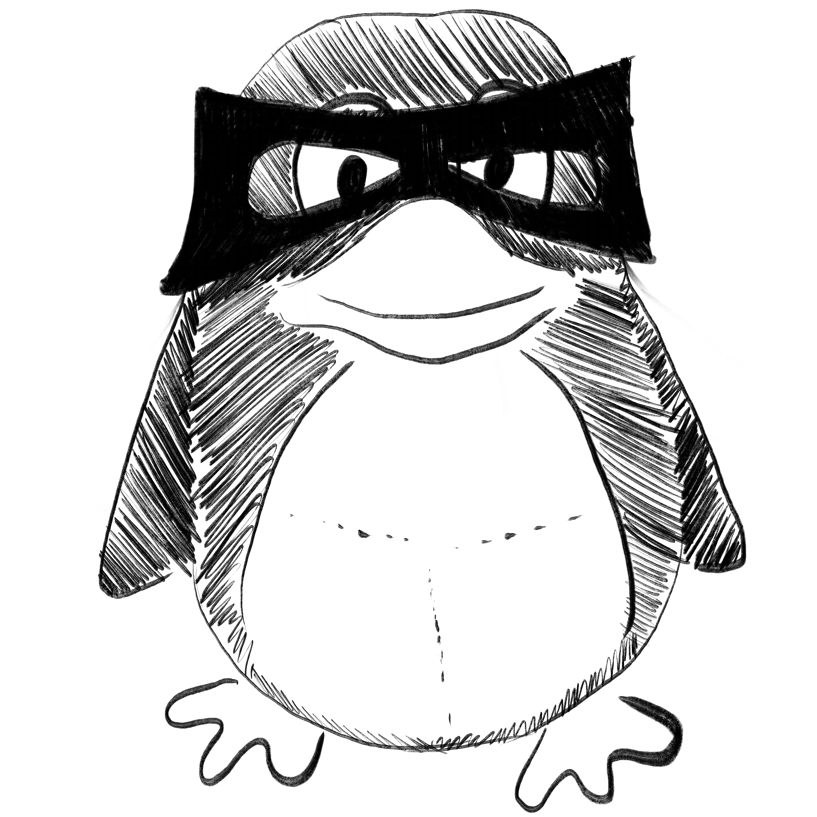
Investigating mental health outcomes of undergraduates and graduate students in Taiwan during the COVID-19 pandemic.
In Journal of American college health : J of ACH
Lin Ching-Hui, Lin Szu-Yin, Hu Bo-Hsien, Lo C Owen
2023-Jan-03
College students, Covid-19, mental health, postsecondary education
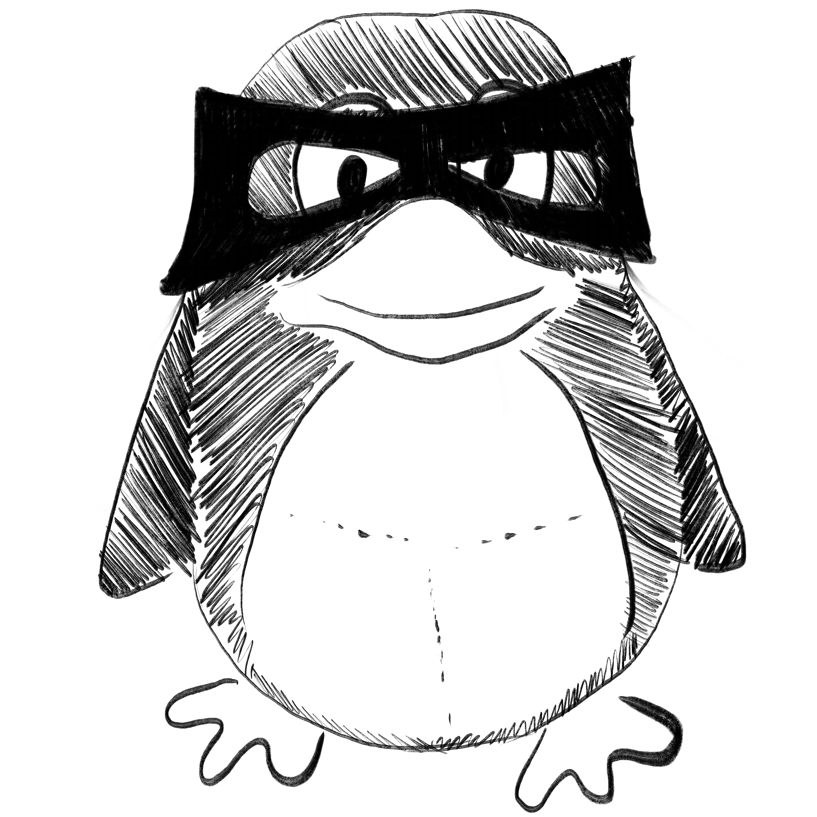
Users' Reactions on Announced Vaccines against COVID-19 Before Marketing in France: Analysis of Twitter posts.
In Journal of medical Internet research ; h5-index 88.0
BACKGROUND :
OBJECTIVE :
METHODS :
RESULTS :
CONCLUSIONS :
Dupuy-Zini Alexandre, Audeh Bissan, Gérardin Christel, Duclos Catherine, Gagneux-Brunon Amandine, Bousquet Cedric
2022-Aug-09
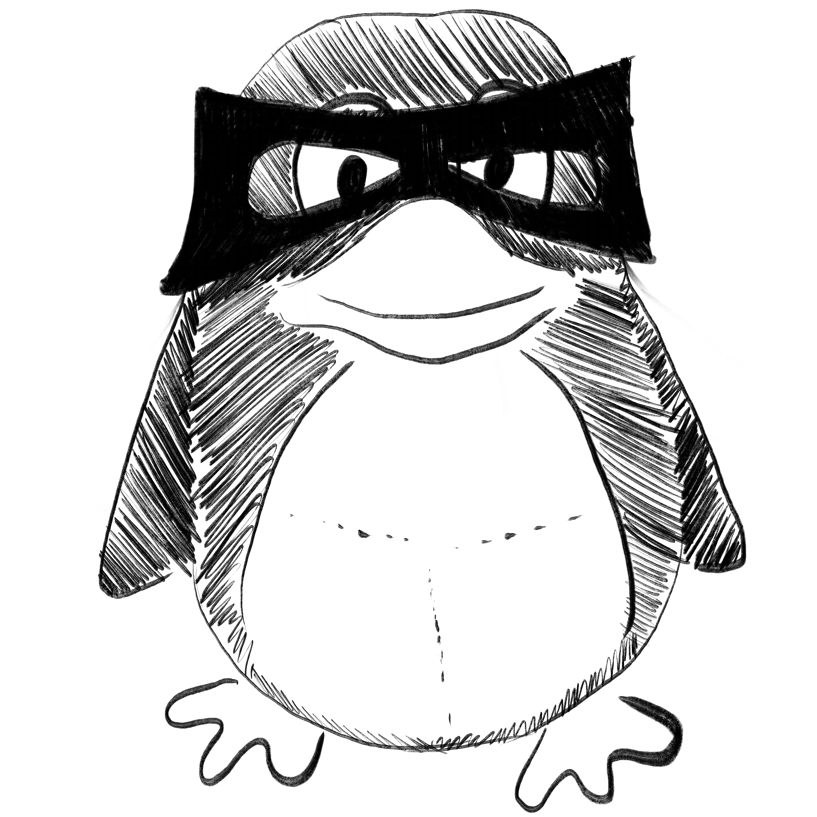
Weighted power Maxwell distribution: Statistical inference and COVID-19 applications.
In PloS one ; h5-index 176.0
Almuqrin Muqrin A, Almutlak Salemah A, Gemeay Ahmed M, Almetwally Ehab M, Karakaya Kadir, Makumi Nicholas, Hussam Eslam, Aldallal Ramy
2023
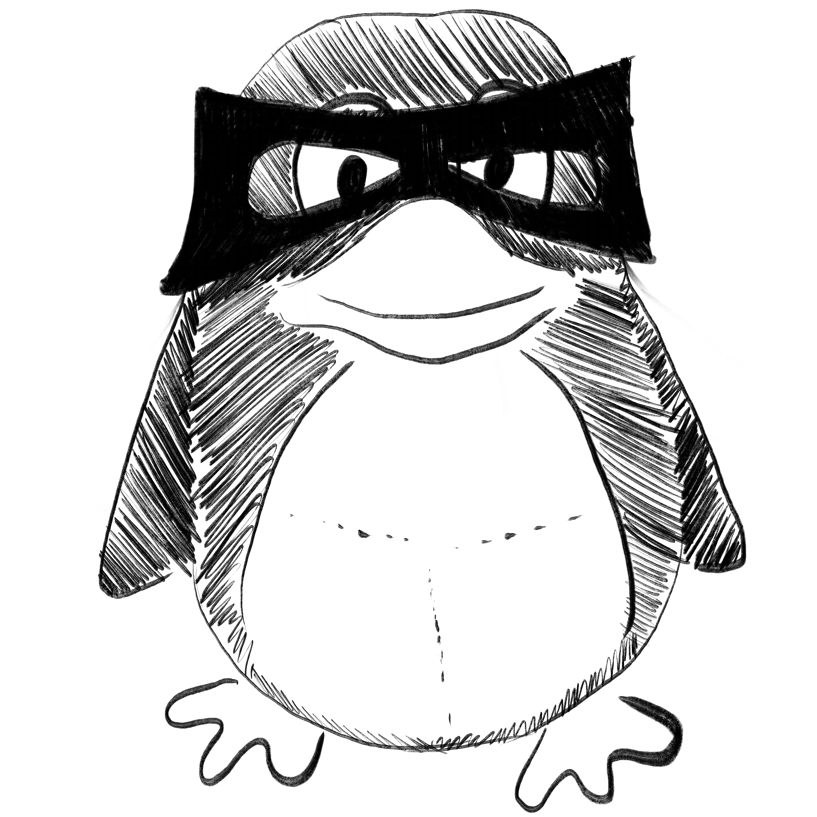
COVID-19 Detection: A Systematic Review of Machine and Deep Learning-Based Approaches Utilizing Chest X-Rays and CT Scans.
In Cognitive computation
Bhatele Kirti Raj, Jha Anand, Tiwari Devanshu, Bhatele Mukta, Sharma Sneha, Mithora Muktasha R, Singhal Stuti
2022-Dec-29
COVID-19, CT scan, Chest X-ray, Deep transfer learning, Machine learning
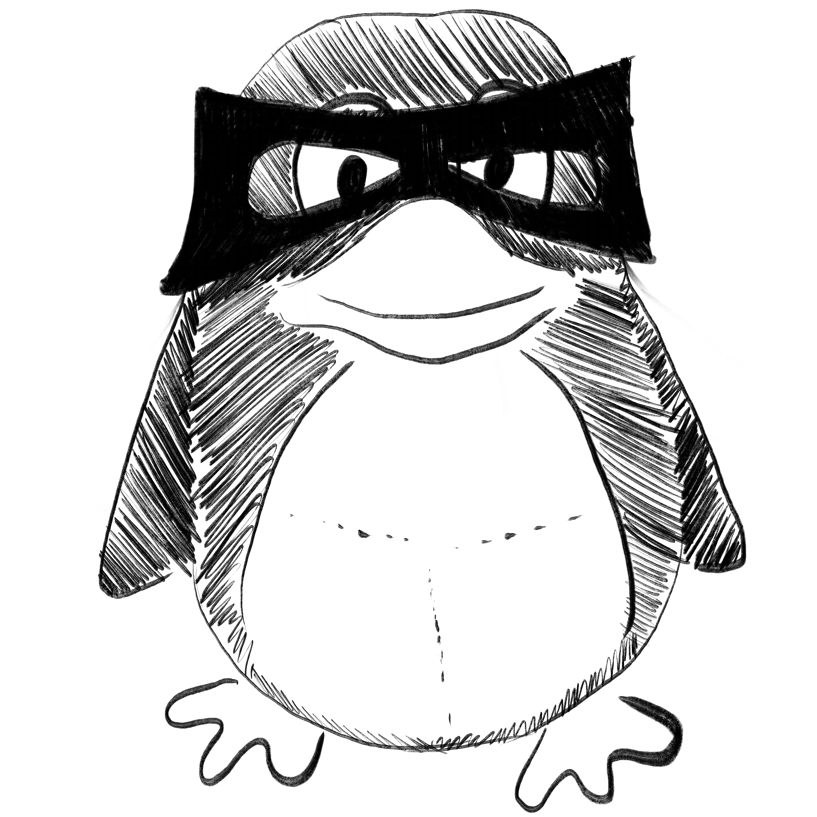
Screening of COVID-19 Based on GLCM Features from CT Images Using Machine Learning Classifiers.
In SN computer science
Godbin A Beena, Jasmine S Graceline
2023
COVID-19, Feature extraction, GLCM, LGBM, Machine learning, SVM
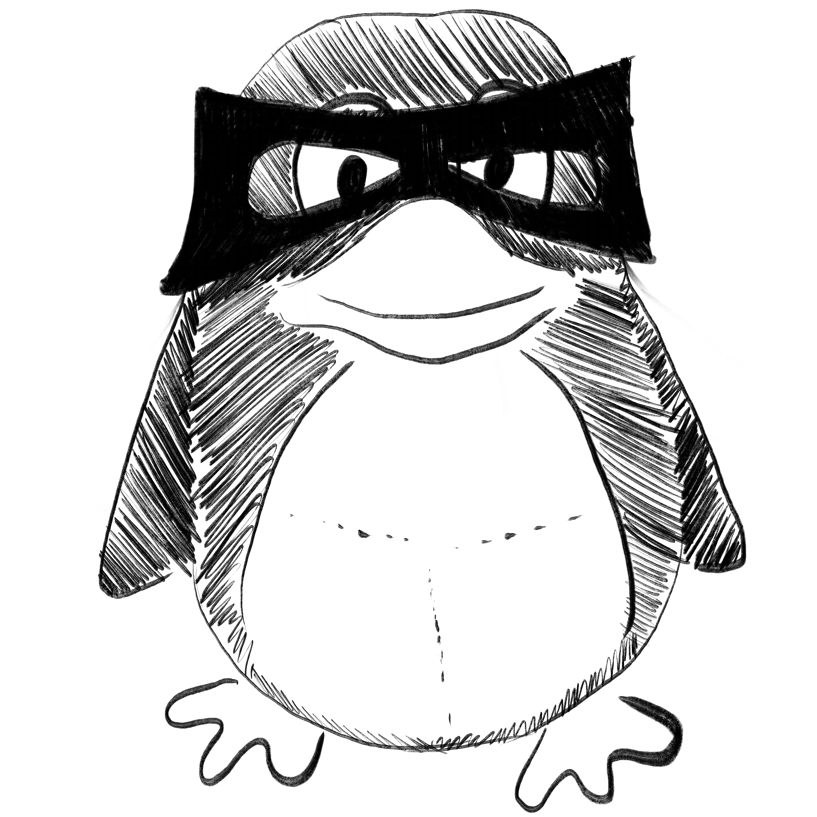
Predicting the evolution of COVID-19 mortality risk: A Recurrent Neural Network approach.
In Computer methods and programs in biomedicine update
BACKGROUND :
METHODS :
RESULTS :
CONCLUSIONS :
Villegas Marta, Gonzalez-Agirre Aitor, Gutiérrez-Fandiño Asier, Armengol-Estapé Jordi, Carrino Casimiro Pio, Fernández David Pérez, Soares Felipe, Serrano Pablo, Pedrera Miguel, García Noelia, Valencia Alfonso
2022-Dec-29
COVID-19, Mortality prediction, Recurrent Neural Network, Time series
Predicting emerging SARS-CoV-2 variants of concern through a One Class dynamic anomaly detection algorithm.
In BMJ health & care informatics
OBJECTIVES :
METHODS :
RESULTS :
DISCUSSION :
CONCLUSION :
Nicora Giovanna, Salemi Marco, Marini Simone, Bellazzi Riccardo
2022-Dec
COVID-19, data science, machine learning, public health informatics
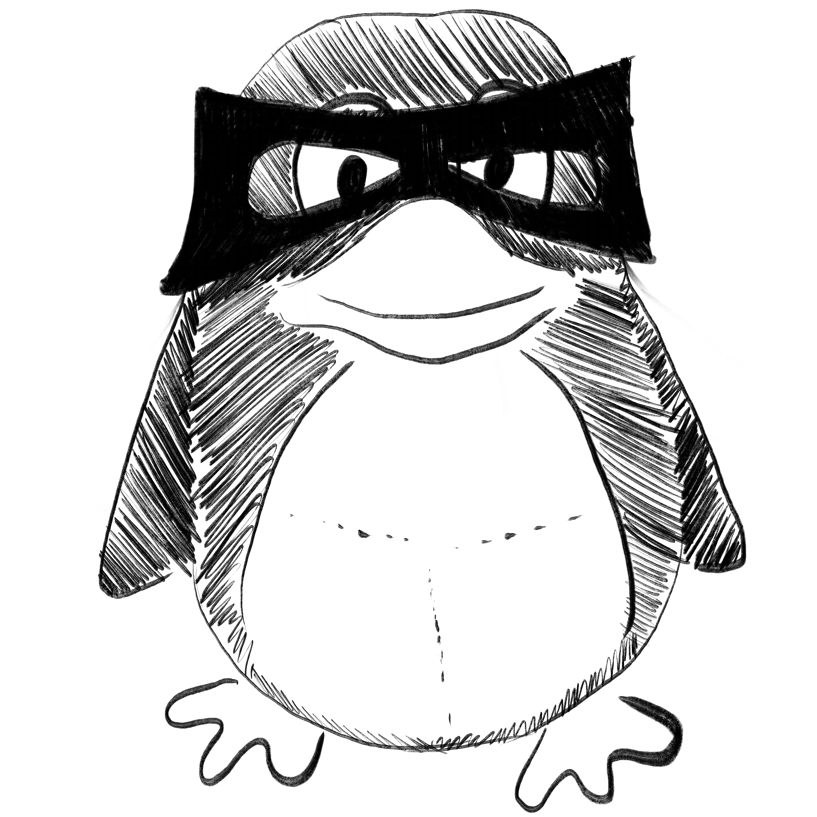
Fitness Dependent Optimizer with Neural Networks for COVID-19 patients.
In Computer methods and programs in biomedicine update
Abdulkhaleq Maryam T, Rashid Tarik A, Hassan Bryar A, Alsadoon Abeer, Bacanin Nebojsa, Chhabra Amit, Vimal S
2022-Dec-27
COVID 19, FDO, Fitness Dependent Optimizer, Machine Learning, Swarm Intelligence
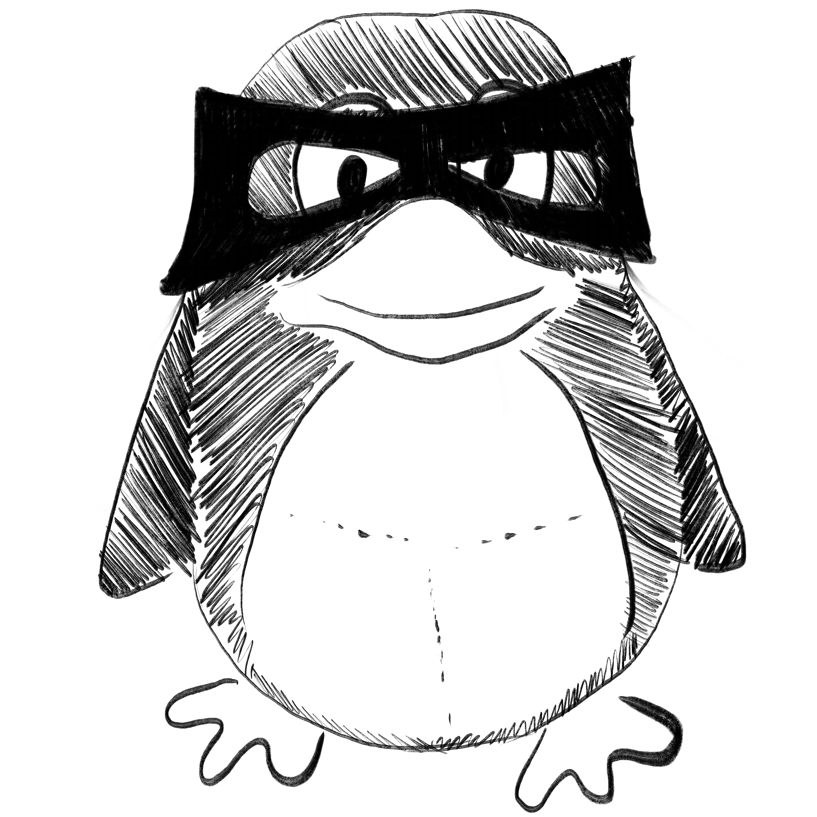
A novel deep neural network model based Xception and genetic algorithm for detection of COVID-19 from X-ray images.
In Annals of operations research
Gülmez Burak
2022-Dec-25
COVID-19, Convolutional neural network, Deep learning, Genetic algorithm, Xception
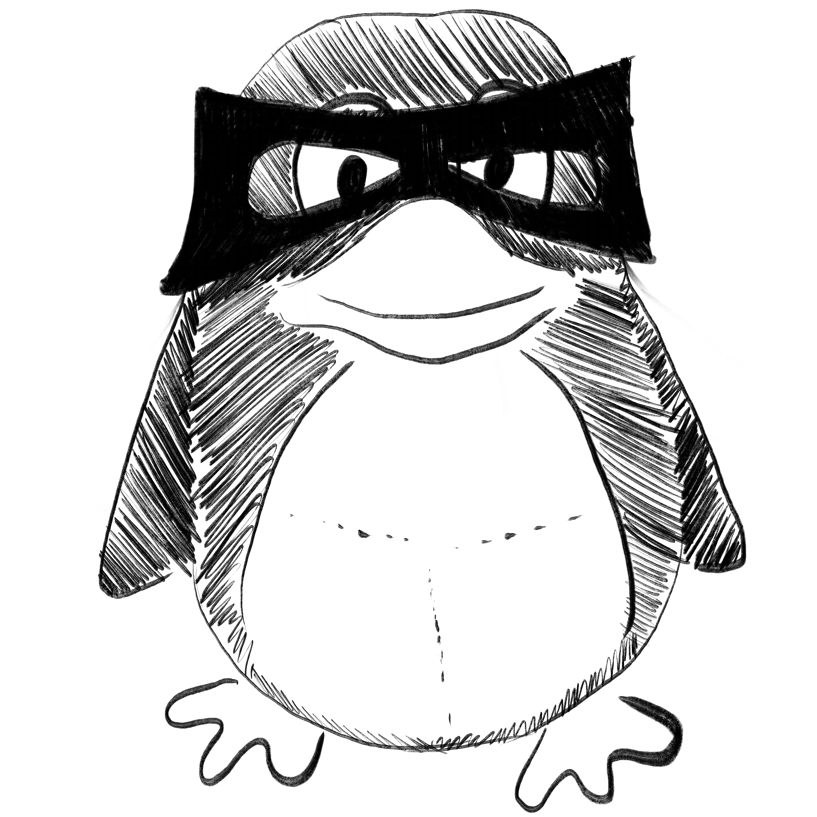
Multi-Objective deep learning framework for COVID-19 dataset problems.
In Journal of King Saud University. Science
BACKGROUND :
METHODS :
RESULTS :
CONCLUSIONS :
Mohammedqasem Roa’a, Mohammedqasim Hayder, Asad Ali Biabani Sardar, Ata Oguz, Alomary Mohammad N, Almehmadi Mazen, Amer Alsairi Ahad, Azam Ansari Mohammad
2022-Dec-28
Artificial intelligence, COVID-19, Deep learning, Hyperparameter optimization, Missing value
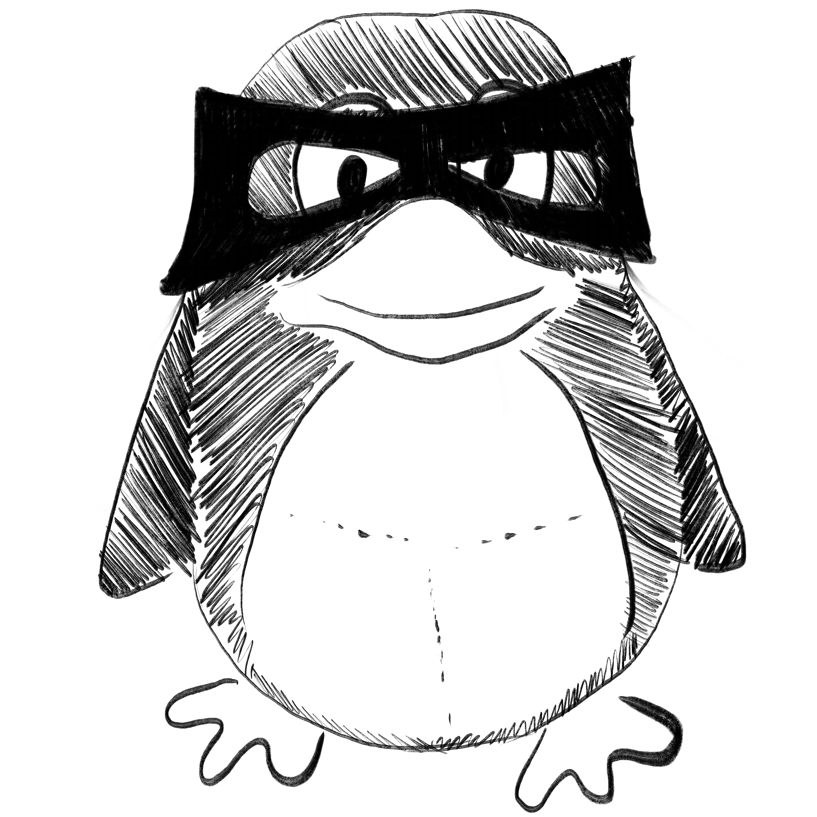
Development of CNN-LSTM combinational architecture for COVID-19 detection.
In Journal of ambient intelligence and humanized computing
Narula Abhinav, Vaegae Naveen Kumar
2022-Dec-24
COVID-19, Chest X-ray, Convolution neural network, Deep learning, Image processing, LSTM
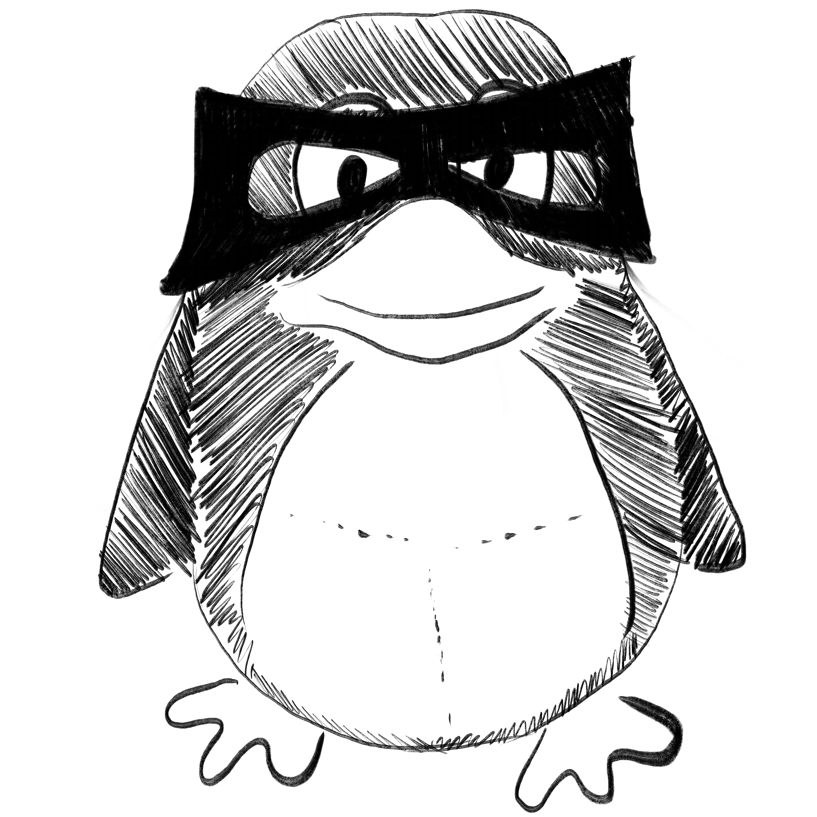
Can financial stress be anticipated and explained? Uncovering the hidden pattern using EEMD-LSTM, EEMD-prophet, and XAI methodologies.
In Complex & intelligent systems
Ghosh Indranil, Dragan Pamucar
2022-Dec-26
Ensemble empirical mode decomposition, Explainable artificial intelligence, Facebook’s prophet algorithm, Financial stress, Long short-term memory network, Technical indicators
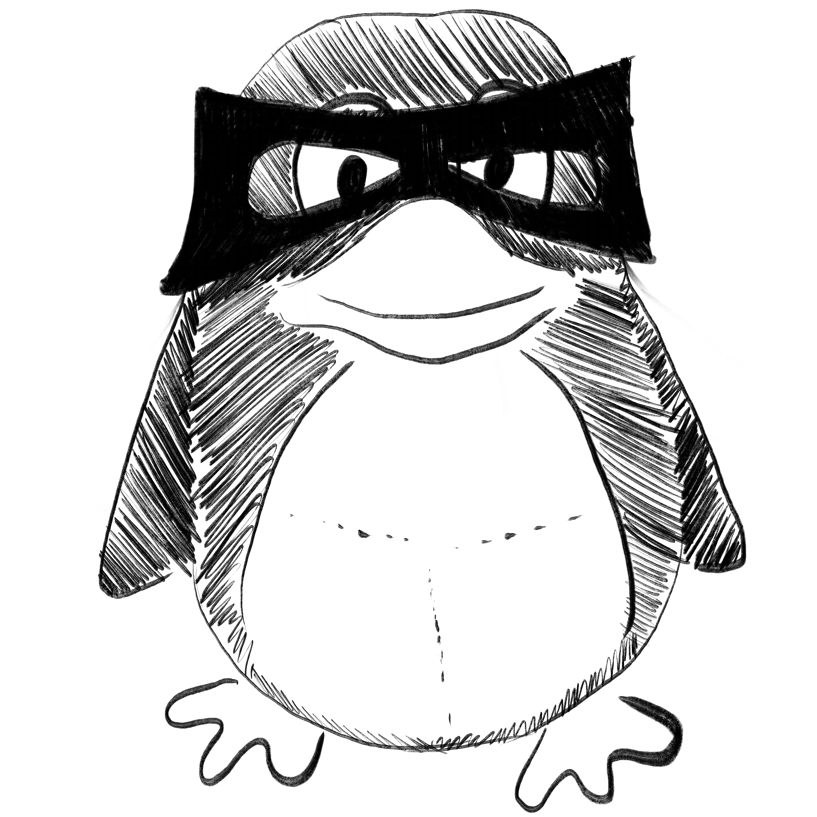
Cough Audio Analysis for COVID-19 Diagnosis.
In SN computer science
Kapoor Teghdeep, Pandhi Tanya, Gupta Bharat
2023
CNN, COVID-19, COVID-19 preliminary diagnosis, Cough diagnosis, Deep learning, LSTM, MLP, Machine learning, RNN, SVM
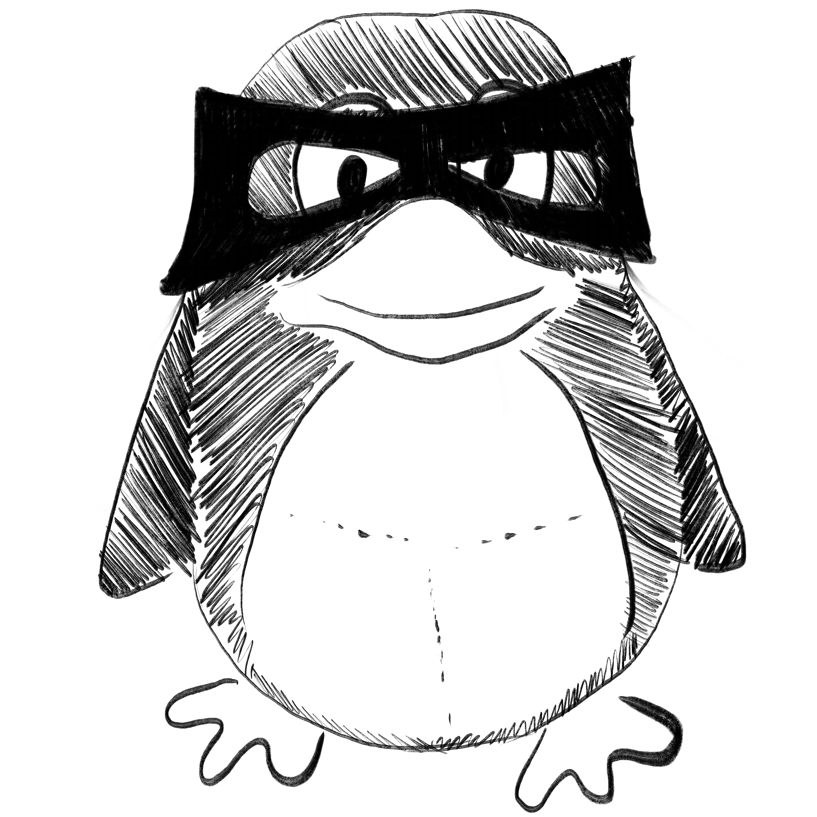
Think Twice: First for Tech, Then for Ed.
In SN computer science
Photopoulos Panos, Triantis Dimos
2023
Blended learning, COVID-19, Face-to-face education, Online learning, Technological determinism, Technology driven change
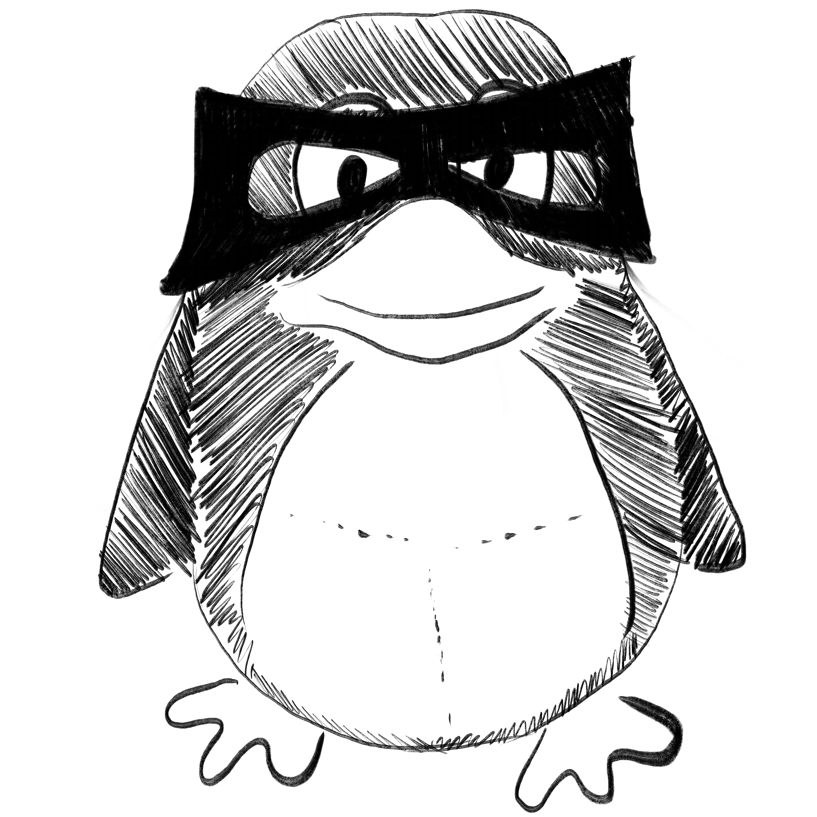
Data Assimilation Predictive GAN (DA-PredGAN) Applied to a Spatio-Temporal Compartmental Model in Epidemiology.
In Journal of scientific computing
Silva Vinicius L S, Heaney Claire E, Li Yaqi, Pain Christopher C
2023
COVID-19, Compartmental model, Data assimilation, Deep learning, Epidemiology, Generative adversarial networks, Reduced-order model, Spatio-temporal prediction
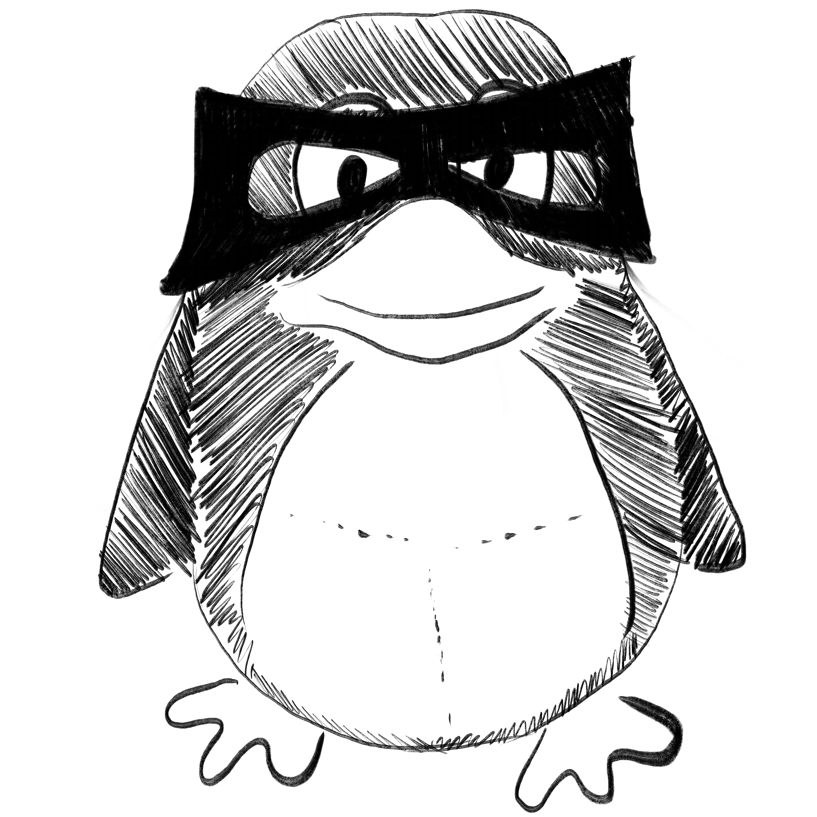
Chest X-Ray Images to Differentiate COVID-19 from Pneumonia with Artificial Intelligence Techniques.
In International journal of biomedical imaging
Islam Rumana, Tarique Mohammed
2022
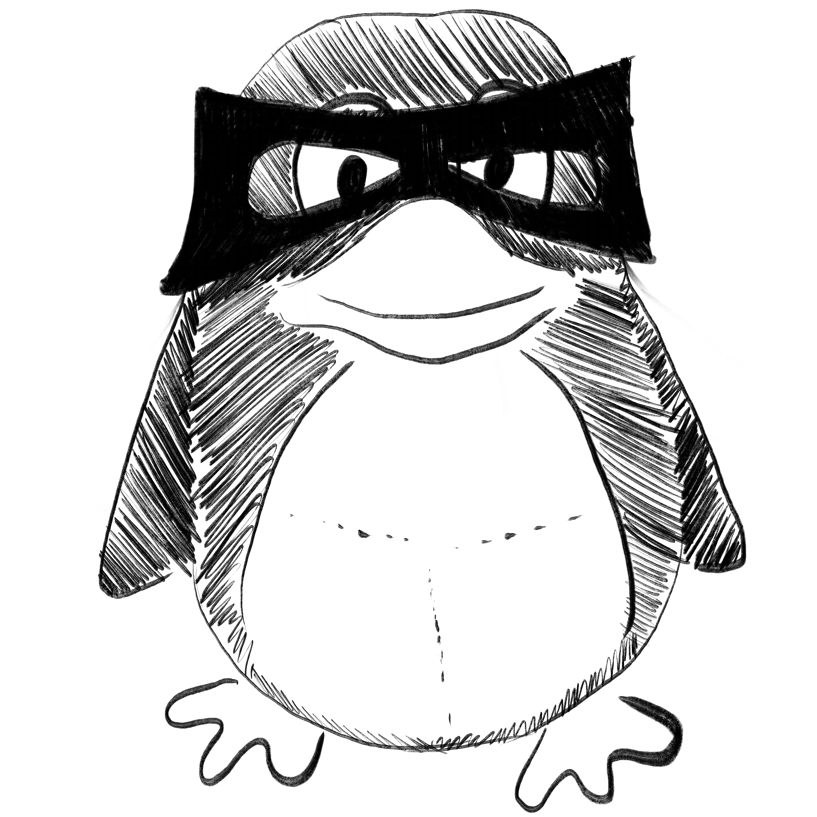
De novo design of site-specific protein interactions with learned surface fingerprints
bioRxiv Preprint
Gainza, P.; Wehrle, S.; Van Hall-Beauvais, A.; Marchand, A.; Scheck, A.; Harteveld, Z.; Ni, D.; Tan, S.; Sverrisson, F.; Goverde, C.; Turelli, P.; Raclot, C.; Teslenko, A.; Pacesa, M.; Rosset, S.; Buckley, S.; Georgeon, S.; Marsden, J.; Petruzzella, A.; Liu, K.; Xu, Z.; Chai, Y.; Han, P.; Gao, G. F.; Oricchio, E.; Fierz, B.; Trono, D.; Stahlberg, H.; Bronstein, M.; Correia, B. E.
2023-01-03
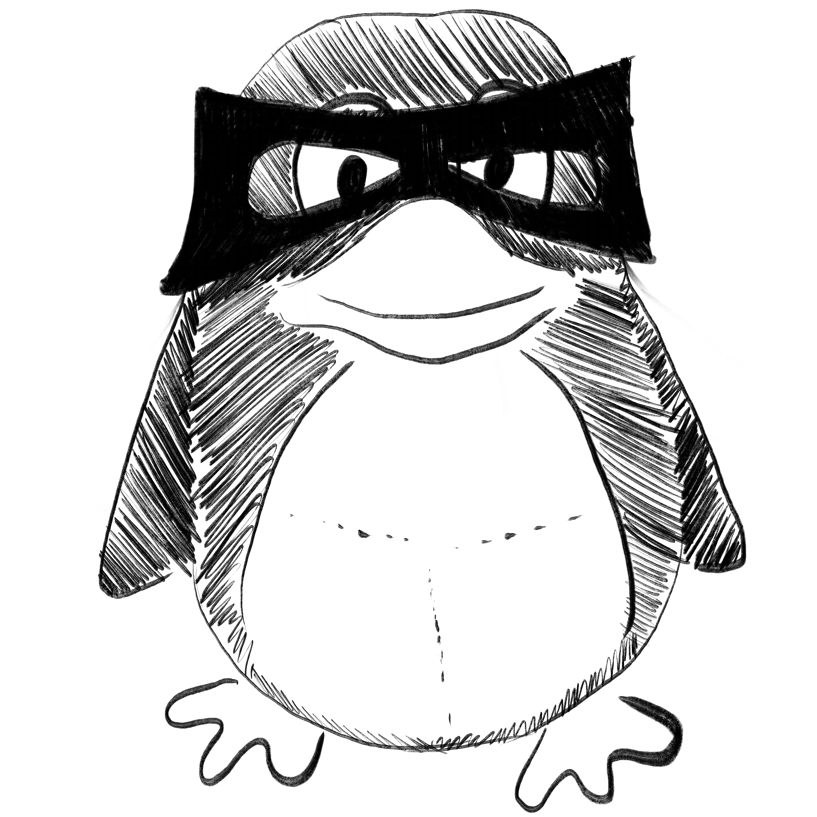
Biosensors - A Miraculous Detecting Tool in Combating the War against COVID-19.
In Current pharmaceutical biotechnology
Deshmukh Rohitas, Mishra Sakshi, Singh Rajesh
2023-Jan-02
Biosensors, COVID-19, CRISPR-Cas9., SARS-CoV-2, Virus, respiratory, syndrome
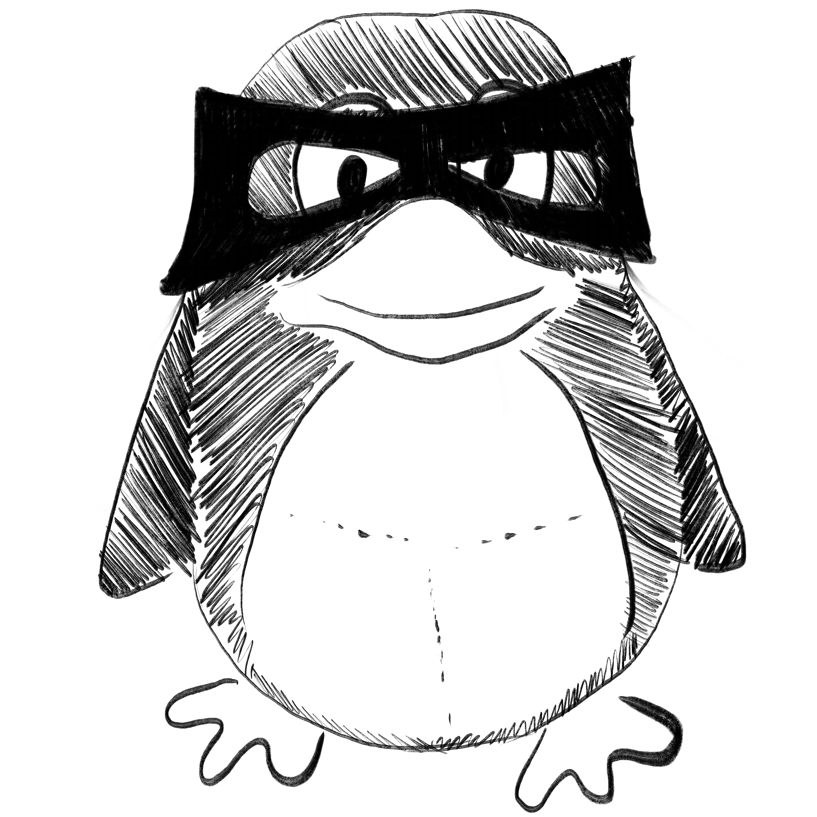
A statistical analysis of tweets on covid-19 vaccine hesitancy utilizing opinion mining: an Indian perspective.
In Social network analysis and mining
Verma Ravi, Chhabra Amit, Gupta Ankit
2023
COVID-19 vaccine, Coronavirus, Latent dirichlet allocation (LDA), Natural language processing (NLP), Opinion mining, Sentiment analysis
Did Australia's COVID-19 restrictions impact statin incidence, prevalence or adherence?
In Current problems in cardiology
OBJECTIVE :
METHODS :
RESULTS :
CONCLUSIONS :
Livori Adam C, Lukose Dickson, Bell J Simon, Webb Geoffrey I, Ilomäki Jenni
2022-Dec-28
Statin, cardiology, cardiovascular, drug utilisation, medication adherence
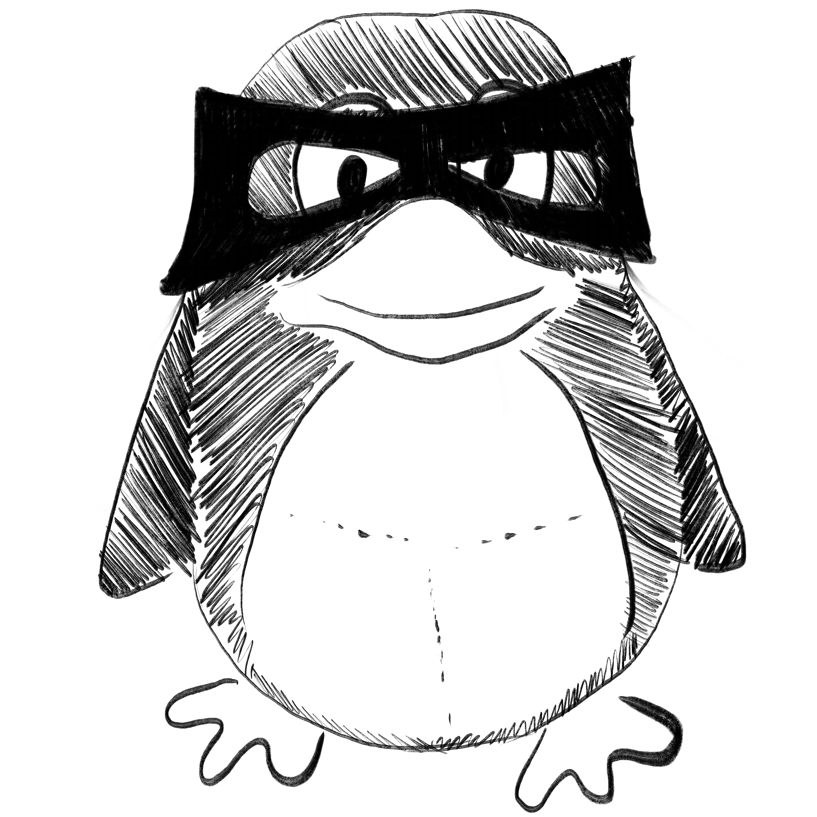
Development and validation of a machine learning-based vocal predictive model for major depressive disorder.
In Journal of affective disorders ; h5-index 79.0
BACKGROUND :
METHODS :
RESULTS :
LIMITATIONS :
CONCLUSIONS :
Wasserzug Yael, Degani Yoav, Bar-Shaked Mili, Binyamin Milana, Klein Amit, Hershko Shani, Levkovitch Yechiel
2022-Dec-28
Depression screening, Machine learning, Predictive analytics, Remote patient monitoring, Speech prosody, Voice analysis
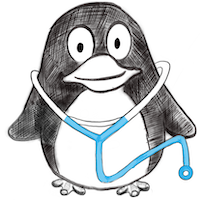
Early prediction of COVID-19 outcome using artificial intelligence techniques and only five laboratory indices.
In Clinical immunology (Orlando, Fla.)
Asteris Panagiotis G, Kokoris Styliani, Gavriilaki Eleni, Tsoukalas Markos Z, Houpas Panagiotis, Paneta Maria, Koutzas Andreas, Argyropoulos Theodoros, Alkayem Nizar Faisal, Armaghani Danial J, Bardhan Abidhan, Cavaleri Liborio, Cao Maosen, Mansouri Iman, Mohammed Ahmed Salih, Samui Pijush, Gerber Gloria, Boumpas Dimitrios T, Tsantes Argyrios, Terpos Evangelos, Dimopoulos Meletios A
2022-Dec-28
Artificial intelligence, Artificial neural networks, COVID-19, Laboratory indices, SARS-CoV2
Interpretable generalized neural additive models for mortality prediction of COVID-19 hospitalized patients in Hamadan, Iran.
In BMC medical research methodology
BACKGROUND :
METHODS :
RESULTS :
CONCLUSIONS :
Moslehi Samad, Mahjub Hossein, Farhadian Maryam, Soltanian Ali Reza, Mamani Mojgan
2022-Dec-31
COVID-19, Feature selection, Generalized neural additive, Laboratory markers, Machine learning, Prediction
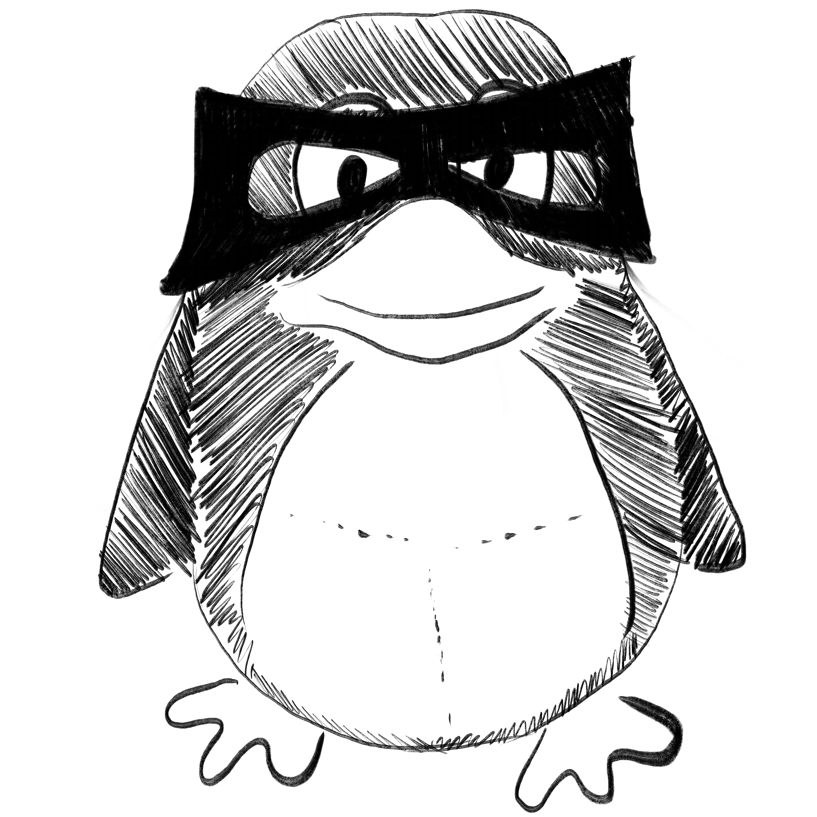
Student adaptation to college and coping in relation to adjustment during COVID-19: A machine learning approach.
In PloS one ; h5-index 176.0
Zhao Yijun, Ding Yi, Chekired Hayet, Wu Ying
2022
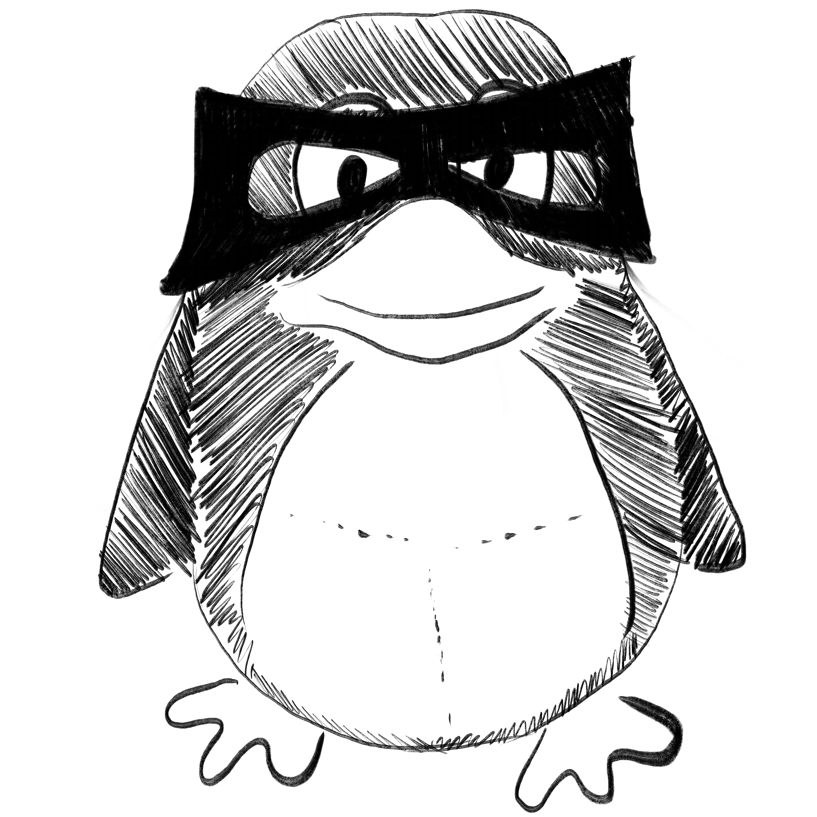
Testing the Acceptability and Usability of an AI-Enabled COVID-19 Diagnostic Tool Among Diverse Adult Populations in the United States.
In Quality management in health care
BACKGROUND AND OBJECTIVES :
METHODS :
RESULTS :
CONCLUSION :
Schilling Josh, Moeller F Gerard, Peterson Rachele, Beltz Brandon, Joshi Deepti, Gartner Danielle, Vang Jee, Jain Praduman
COVID-19 Diagnosis on Chest Radiograph Using Artificial Intelligence.
In Cureus
BACKGROUND :
MATERIALS AND METHODS :
RESULTS :
CONCLUSION :
Baruah Dhiraj, Runge Louis, Jones Richard H, Collins Heather R, Kabakus Ismail M, McBee Morgan P
2022-Nov
artificial intelligence, chest radiography, covid, receiver operating characteristic (roc) analysis, rt-pcr
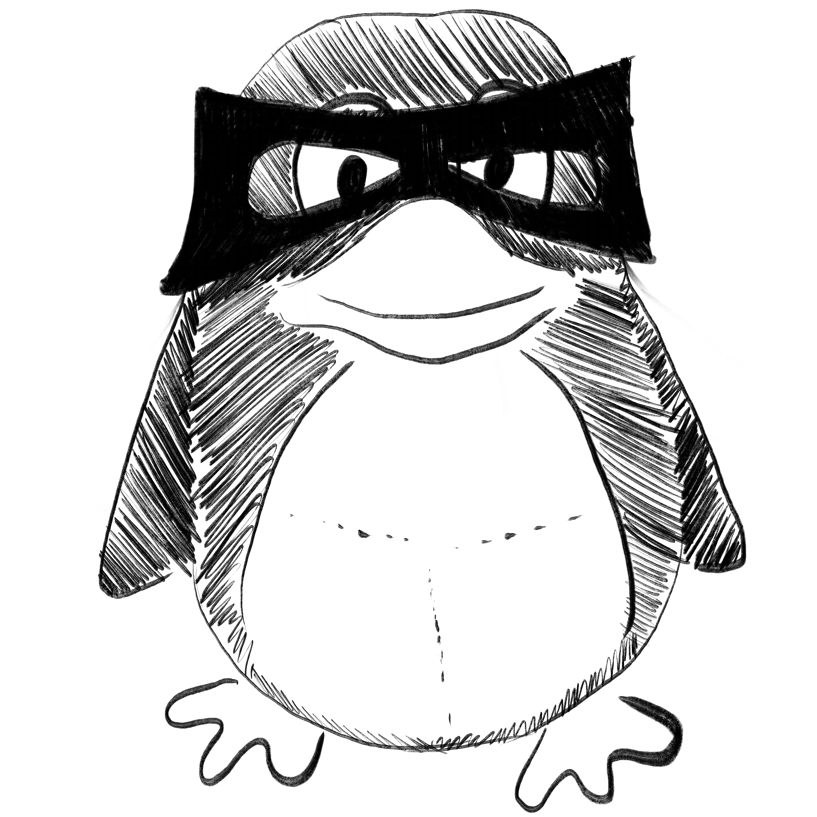
The role of artificial intelligence technology in the care of diabetic foot ulcers: the past, the present, and the future.
In World journal of diabetes ; h5-index 53.0
Pappachan Joseph M, Cassidy Bill, Fernandez Cornelius James, Chandrabalan Vishnu, Yap Moi Hoon
2022-Dec-15
Artificial intelligence technology, COVID-19 pandemic, Diabetic foot ulcers, Digital photography, Mobile app, Photographic monitoring
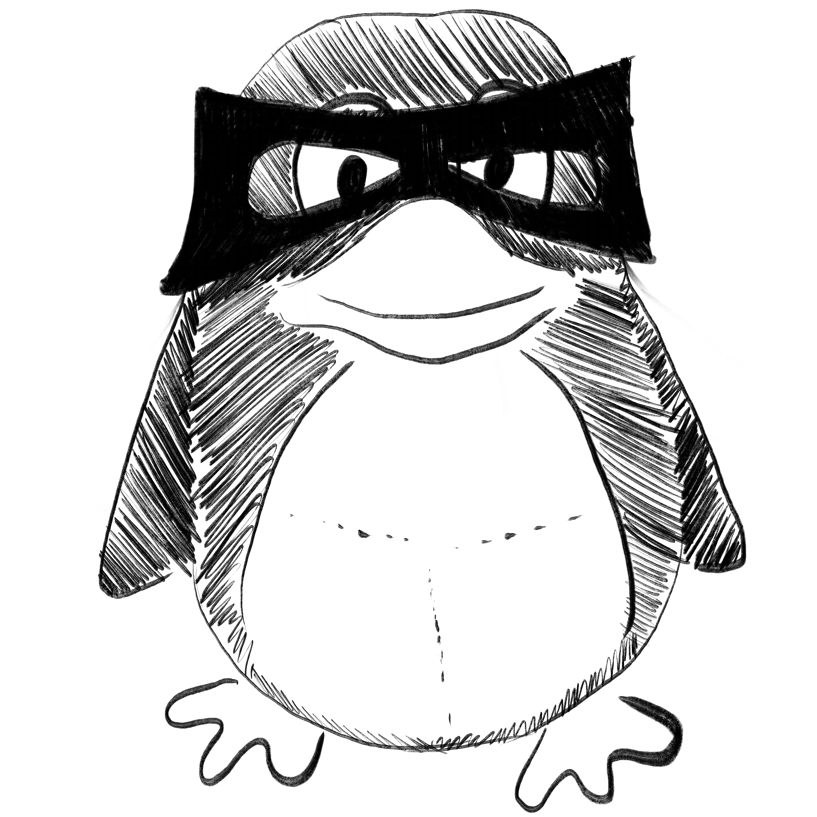
What factors can support students' deep learning in the online environment: The mediating role of learning self-efficacy and positive academic emotions?
In Frontiers in psychology ; h5-index 92.0
INTRODUCTION :
METHODS :
RESULTS :
DISCUSSION :
Zhao Jingxian, Liu Enyun
2022
deep learning, learning self-efficacy, perceived TPACK support, perceived peer support, perceived technical usefulness and ease of use, positive academic emotions
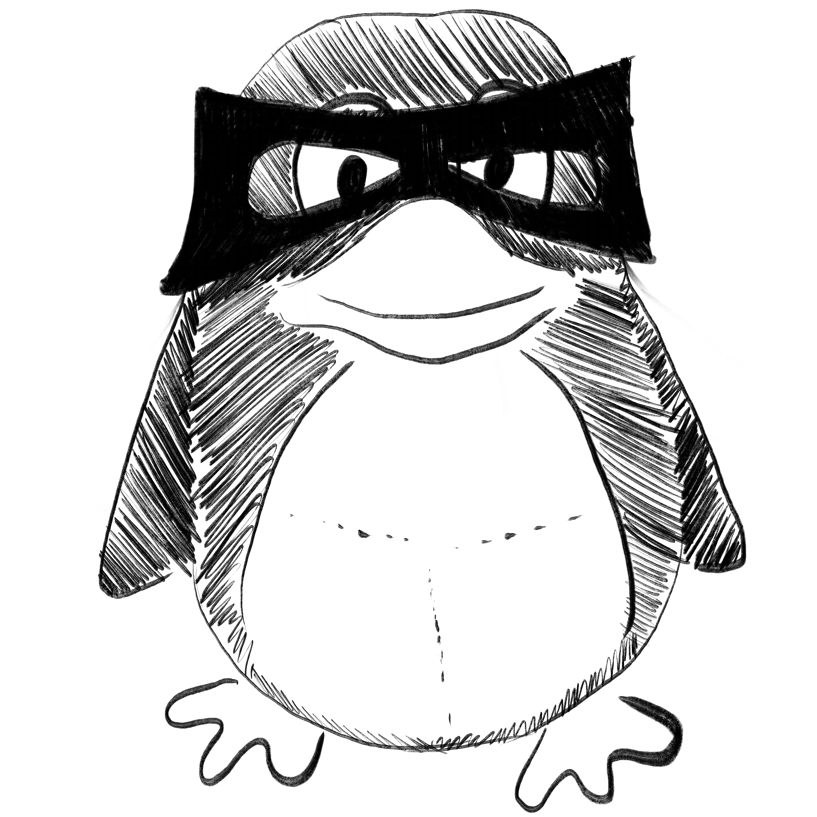
A machine learning approach for predicting high risk hospitalized patients with COVID-19 SARS-Cov-2.
In BMC medical informatics and decision making ; h5-index 38.0
BACKGROUND :
METHODS :
RESULTS :
CONCLUSIONS :
Bottrighi Alessio, Pennisi Marzio, Roveta Annalisa, Massarino Costanza, Cassinari Antonella, Betti Marta, Bolgeo Tatiana, Bertolotti Marinella, Rava Emanuele, Maconi Antonio
2022-Dec-28
COVID-19, Explainability, Machine learning, Patient risk prediction
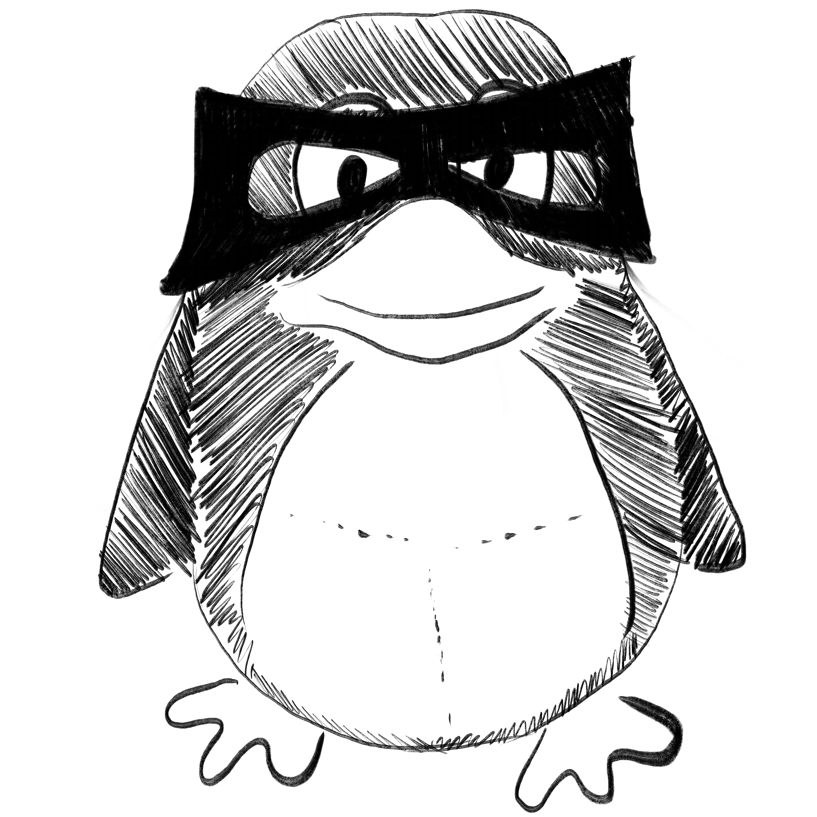
Emerging Dominant SARS-CoV-2 Variants.
In Journal of chemical information and modeling
Chen Jiahui, Wang Rui, Hozumi Yuta, Liu Gengzhuo, Qiu Yuchi, Wei Xiaoqi, Wei Guo-Wei
2022-Dec-28
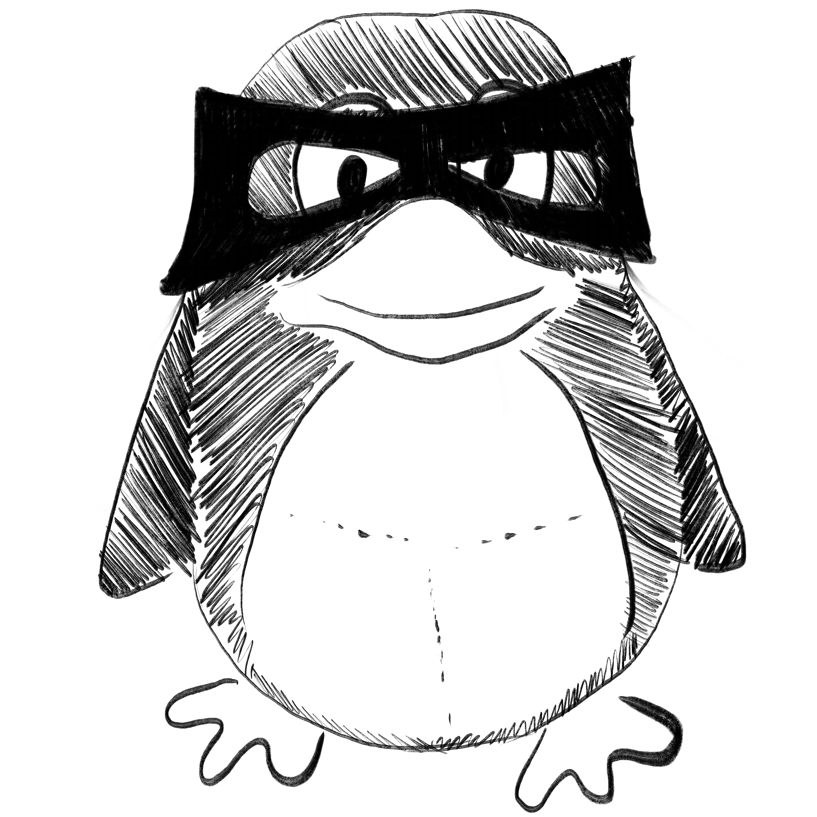
Accurate and fast clade assignment via deep learning and frequency chaos game representation.
In GigaScience
BACKGROUND :
RESULTS :
CONCLUSIONS :
AVAILABILITY :
Avila Cartes Jorge, Anand Santosh, Ciccolella Simone, Bonizzoni Paola, Della Vedova Gianluca
2022-Dec-28
, GISAID clades, SARS-CoV-2, chaos game representation, classification of genome sequences, convolutional neural networks, deep learning
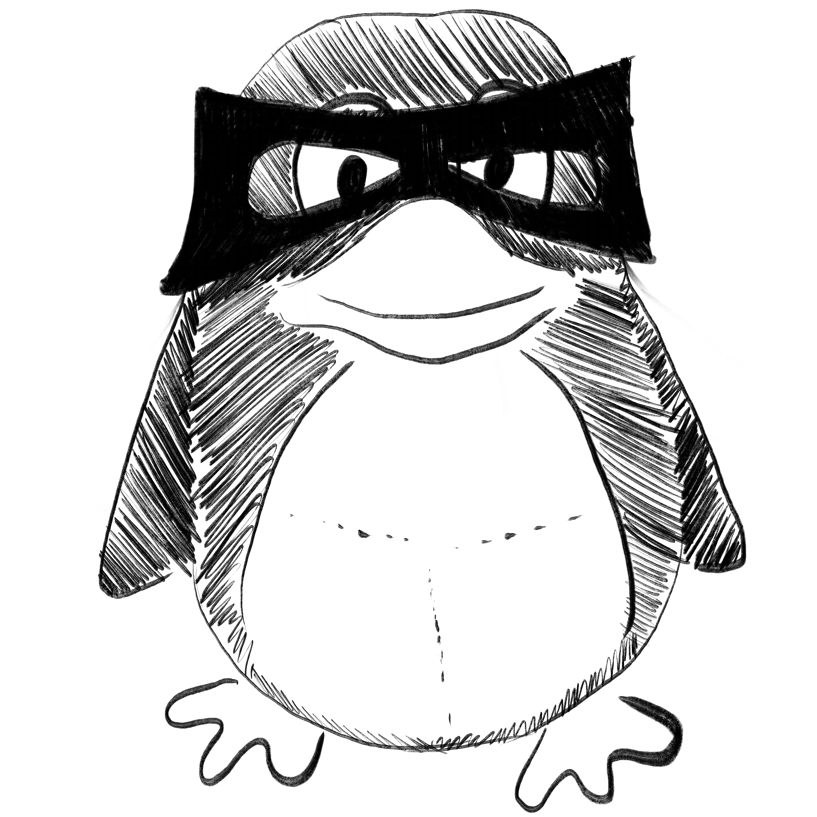
Multi-horizon predictive models for guiding extracorporeal resource allocation in critically ill COVID-19 patients.
In Journal of the American Medical Informatics Association : JAMIA
OBJECTIVE :
MATERIAL AND METHODS :
RESULTS :
DISCUSSION AND CONCLUSION :
Xue Bing, Shah Neel, Yang Hanqing, Kannampallil Thomas, Payne Philip Richard Orrin, Lu Chenyang, Said Ahmed Sameh
2022-Dec-28
COVID-19, ECMO, early alert, machine learning, prediction, resource allocation
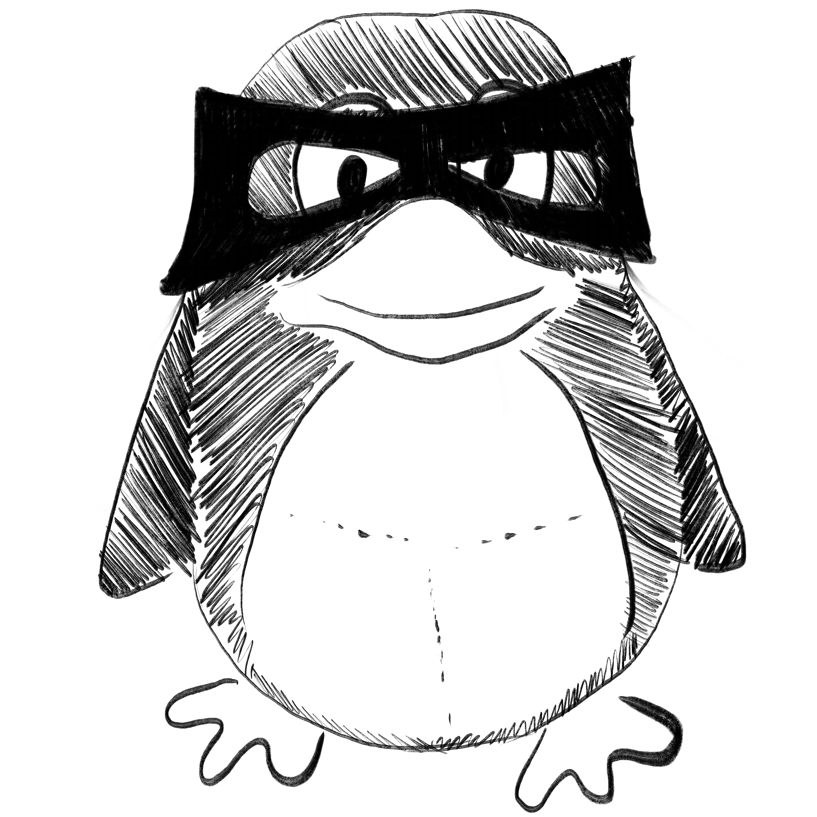
Towards a soft three-level voting model (Soft T-LVM) for fake news detection.
In Journal of intelligent information systems
Jlifi Boutheina, Sakrani Chayma, Duvallet Claude
2022-Dec-23
COVID-19., Ensemble learning models, Fake news detection, Machine learning algorithms, Natural Language Processing (NLP), Social media
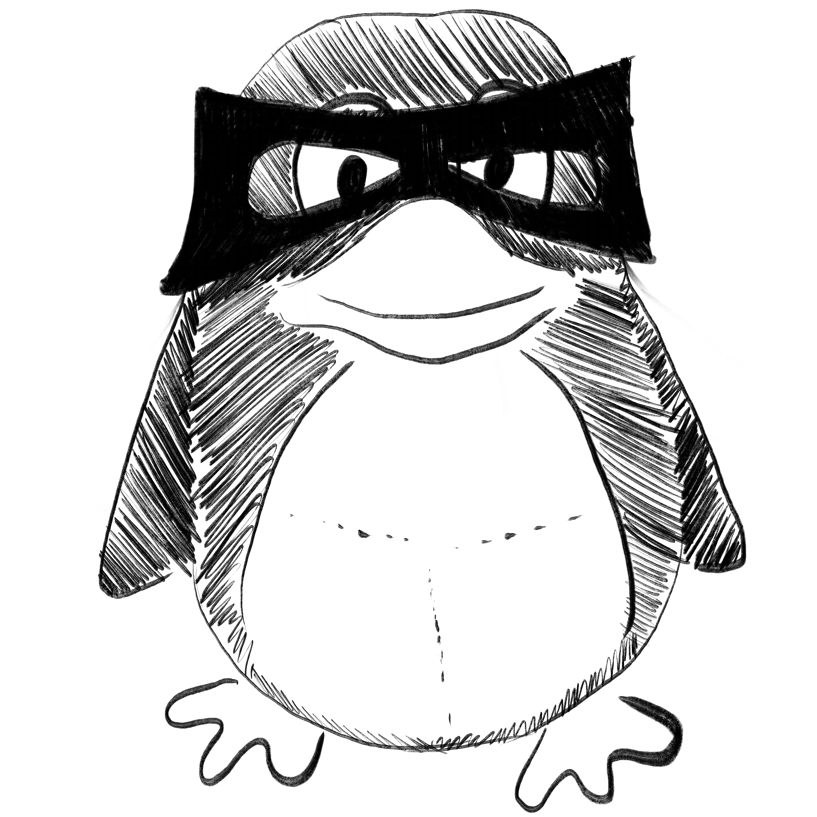
AI Assisted Attention Mechanism for Hybrid Neural Model to Assess Online Attitudes About COVID-19.
In Neural processing letters
Kour Harnain, Gupta Manoj K
2022-Dec-23
BERT, COVID-19, LSTM, Sentiment analysis, Transfer learning, Tweets
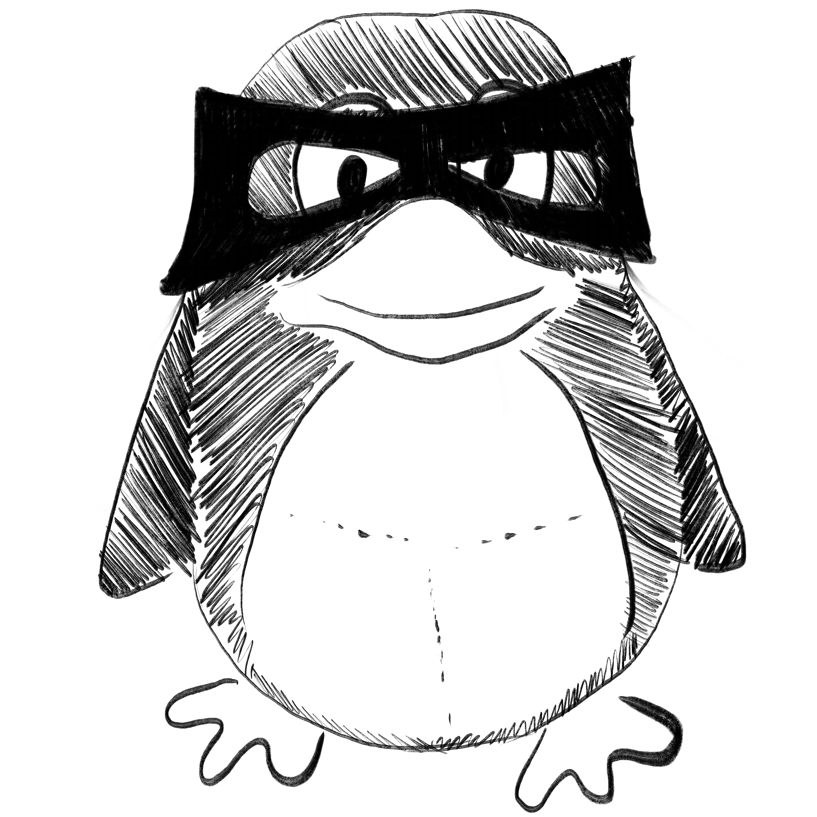
Enhancing the classification metrics of spectroscopy spectrums using neural network based low dimensional space.
In Earth science informatics
Yousuff Mohamed, Babu Rajasekhara
2022-Dec-23
COVID-19, Chemometrics, Dimensionality reduction, Machine learning, Random Forest, Spectroscopy
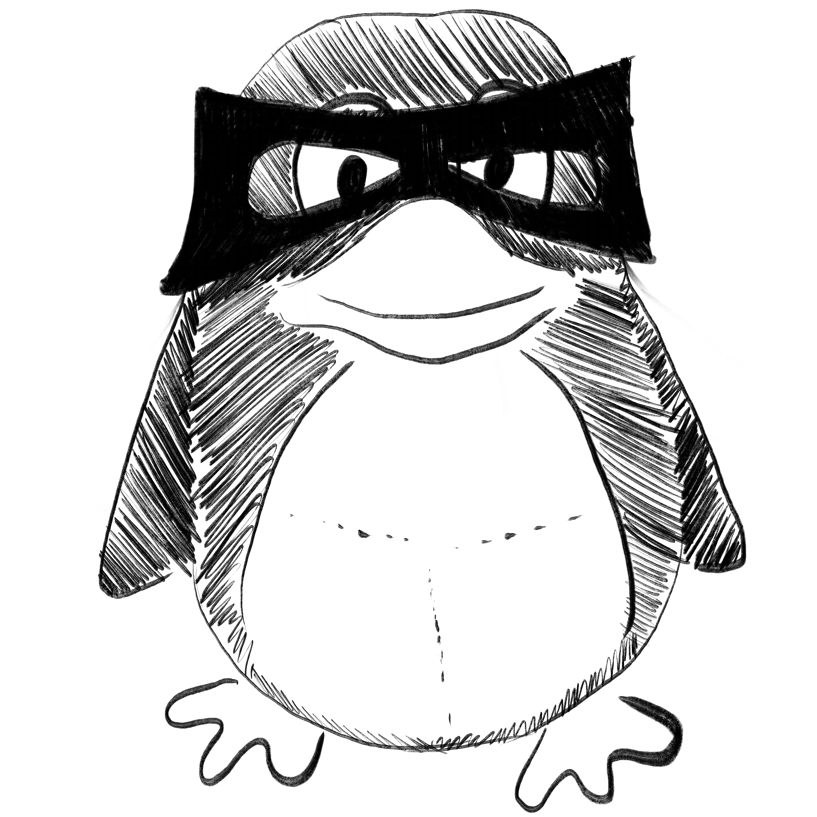
A robust deep learning platform to predict CD8+ T-cell epitopes
bioRxiv Preprint
Lee, C. H.-J.; Huh, J.; Buckley, P. R.; Jang, M. J.; Pereira Pinho, M.; Fernandes, R.; Antanaviciute, A.; Simmons, A.; Koohy, H.
2022-12-29
Prevalence of Asymptomatic SARS-CoV-2 Infection in Japan.
In JAMA network open
IMPORTANCE :
OBJECTIVE :
DESIGN, SETTING, AND PARTICIPANTS :
MAIN OUTCOMES AND MEASURES :
RESULTS :
CONCLUSIONS AND RELEVANCE :
Suzuki Toru, Aizawa Kenichi, Shibuya Kenji, Yamanaka Shinya, Anzai Yuichiro, Kurokawa Kiyoshi, Nagai Ryozo
2022-Dec-01
Depression and anxiety on Twitter during the COVID-19 stay-at-home period in seven major US cities.
In AJPM focus
INTRODUCTION :
METHODS :
RESULTS :
CONCLUSIONS :
Levanti Danielle, Monastero Rebecca N, Zamani Mohammadzaman, Eichstaedt Johannes C, Giorgi Salvatore, Schwartz H Andrew, Meliker Jaymie R
2022-Dec-22
coronavirus, mental health, social media, stay-at-home order
Assessing Trustworthy AI in Times of COVID-19: Deep Learning for Predicting a Multiregional Score Conveying the Degree of Lung Compromise in COVID-19 Patients.
In IEEE transactions on technology and society
Allahabadi Himanshi, Amann Julia, Balot Isabelle, Beretta Andrea, Binkley Charles, Bozenhard Jonas, Bruneault Frederick, Brusseau James, Candemir Sema, Cappellini Luca Alessandro, Chakraborty Subrata, Cherciu Nicoleta, Cociancig Christina, Coffee Megan, Ek Irene, Espinosa-Leal Leonardo, Farina Davide, Fieux-Castagnet Genevieve, Frauenfelder Thomas, Gallucci Alessio, Giuliani Guya, Golda Adam, van Halem Irmhild, Hildt Elisabeth, Holm Sune, Kararigas Georgios, Krier Sebastien A, Kuhne Ulrich, Lizzi Francesca, Madai Vince I, Markus Aniek F, Masis Serg, Mathez Emilie Wiinblad, Mureddu Francesco, Neri Emanuele, Osika Walter, Ozols Matiss, Panigutti Cecilia, Parent Brendan, Pratesi Francesca, Moreno-Sanchez Pedro A, Sartor Giovanni, Savardi Mattia, Signoroni Alberto, Sormunen Hanna-Maria, Spezzatti Andy, Srivastava Adarsh, Stephansen Annette F, Theng Lau Bee, Tithi Jesmin Jahan, Tuominen Jarno, Umbrello Steven, Vaccher Filippo, Vetter Dennis, Westerlund Magnus, Wurth Renee, Zicari Roberto V
2022-Dec
Artificial intelligence, COVID-19, Z-Inspection®, case study, ethical tradeoff, ethics, explainable AI, healthcare, pandemic, radiology, trust, trustworthy AI
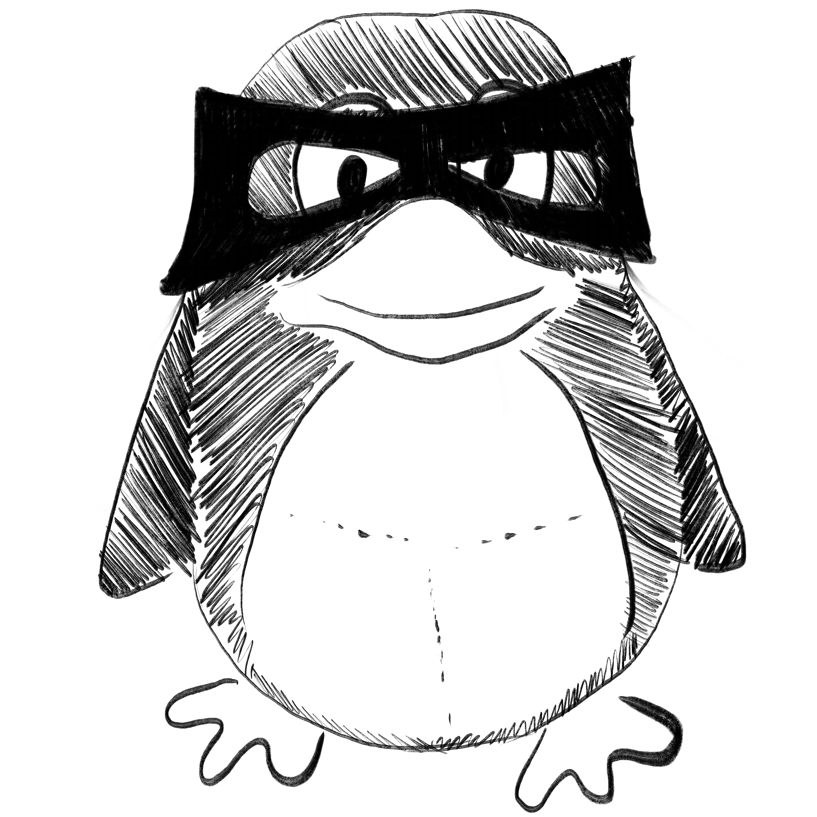
Automatic cough detection from realistic audio recordings using C-BiLSTM with boundary regression.
In Biomedical signal processing and control
You Mingyu, Wang Weihao, Li You, Liu Jiaming, Xu Xianghuai, Qiu Zhongmin
2022-Feb
BiLSTM, Boundary regression, C-BiLSTM, Cough detection, Deep learning
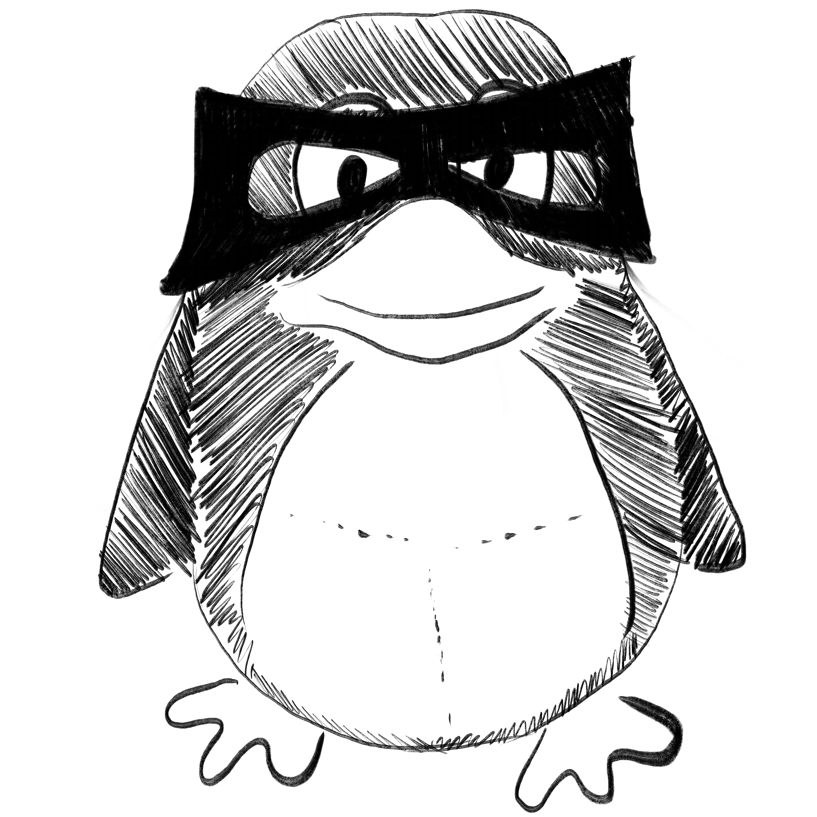
Impact of COVID-19 pandemic on oil consumption in the United States: A new estimation approach.
In Energy (Oxford, England)
Wang Qiang, Li Shuyu, Zhang Min, Li Rongrong
2022-Jan-15
COVID-19, Pandemic-free scenario, Simulation, U.S. petroleum
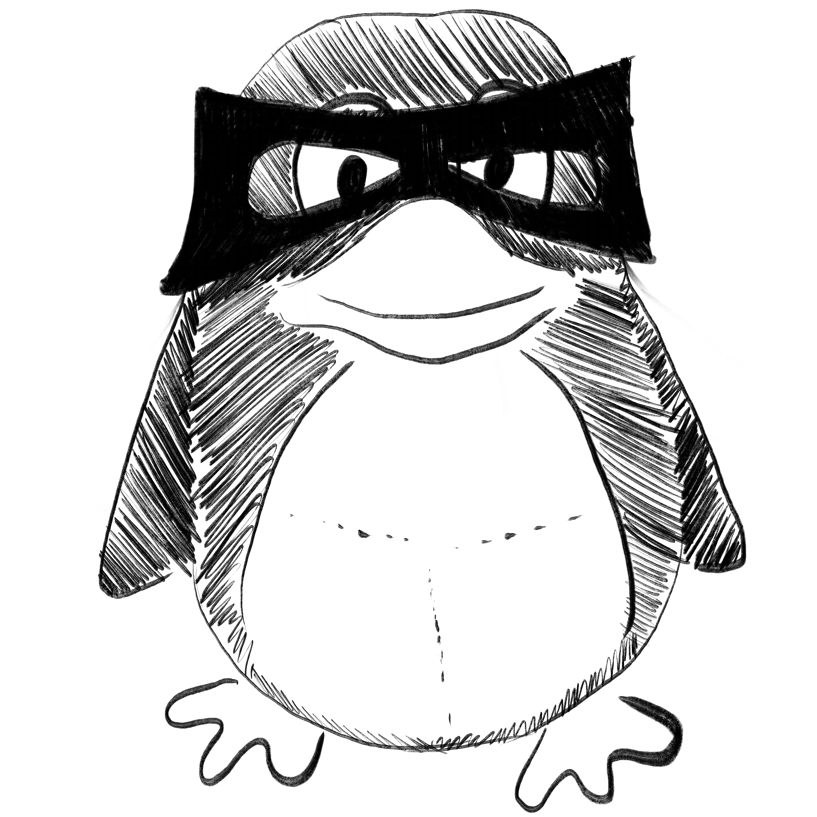
Visualizing the historical COVID-19 shock in the US airline industry: A Data Mining approach for dynamic market surveillance.
In Journal of air transport management
Pérez-Campuzano Darío, Rubio Andrada Luis, Morcillo Ortega Patricio, López-Lázaro Antonio
2022-Jun
Airlines, COVID-19, Data mining (DM), K-means, Self-organizing map (SOM), Unsupervised learning
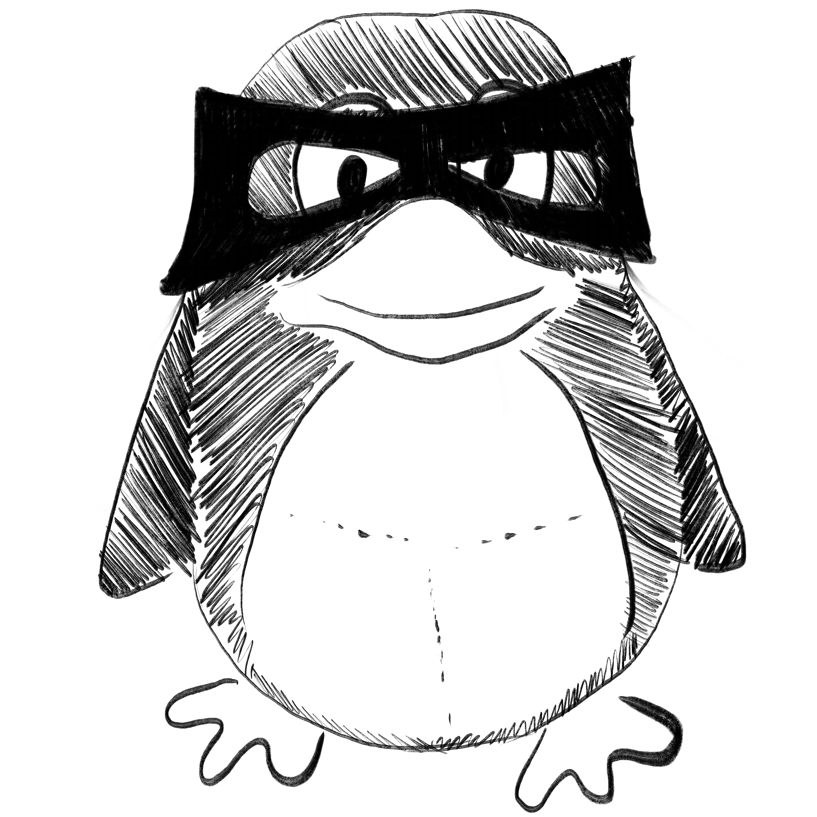
Chang impact analysis of level 3 COVID-19 alert on air pollution indicators using artificial neural network.
In Ecological informatics
Lin Guan-Yu, Chen Wei-Yea, Chieh Shao-Heng, Yang Yi-Tsung
2022-Jul
And SO42− prediction, Artificial neural network, Level 3 COVID-19 alert, Meteorological effect factors, NO3−, NOx, O3
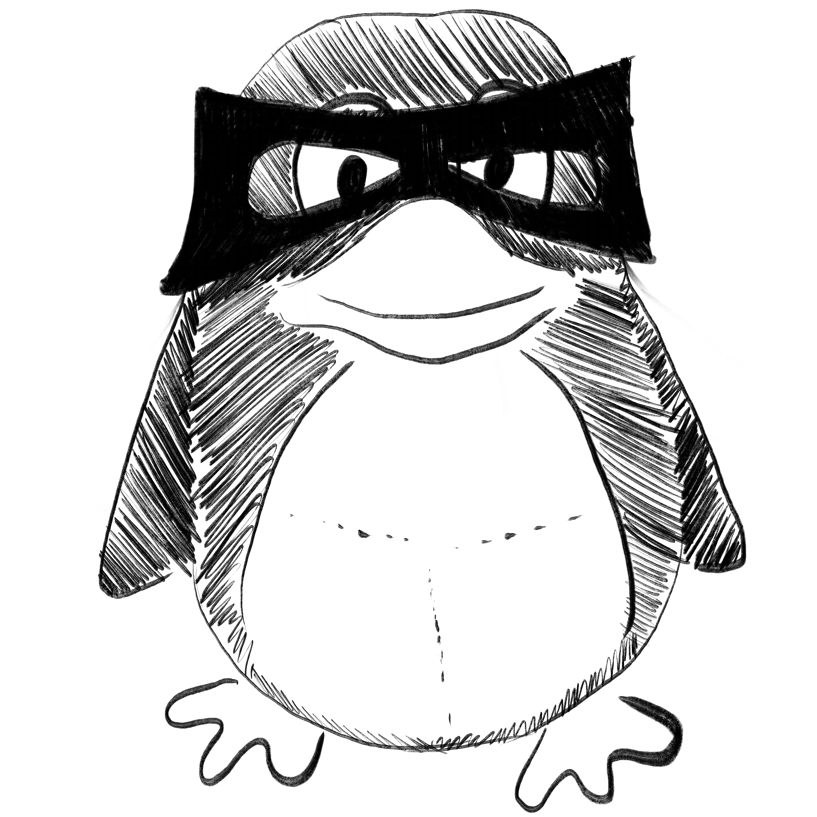
Covid-19 Diagnosis by WE-SAJ.
In Systems science & control engineering
Wang Wei, Zhang Xin, Wang Shui-Hua, Zhang Yu-Dong
2022-Dec-31
COVID-19, Deep Learning, Diagnosis, Jaya, Self-adaptive Jaya, Wavelet Entropy
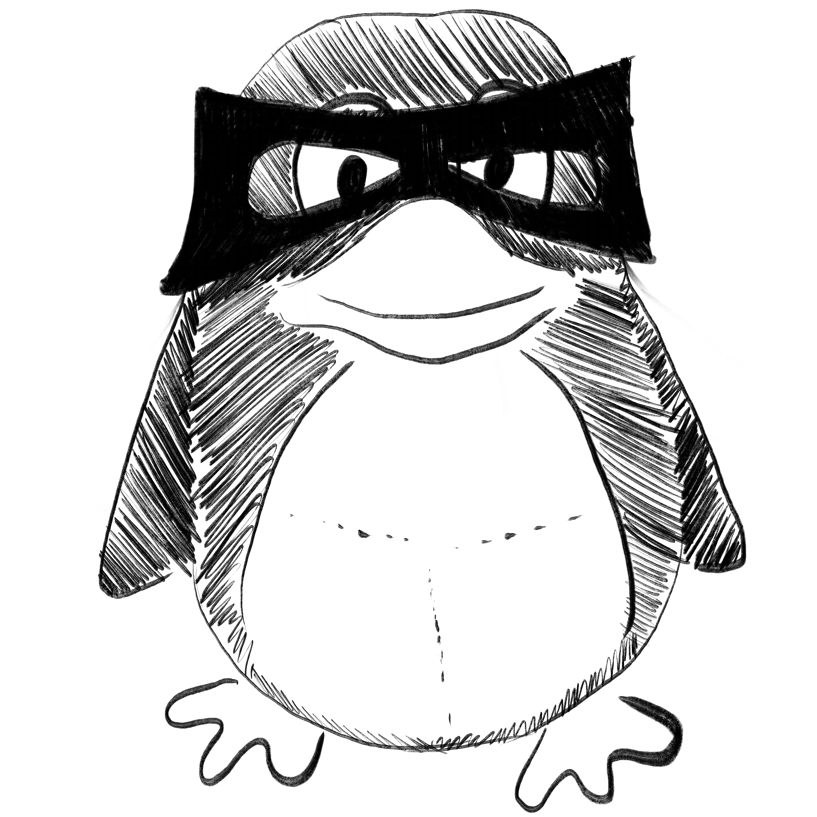
Optimizing deep neural networks to predict the effect of social distancing on COVID-19 spread.
In Computers & industrial engineering
Liu Dixizi, Ding Weiping, Dong Zhijie Sasha, Pedrycz Witold
2022-Apr
COVID-19 Pandemic, Deep Neural Network, Evolutionary Algorithm, Generalized Opposition-Based Learning, Particle Swarm Optimization, Social Distancing
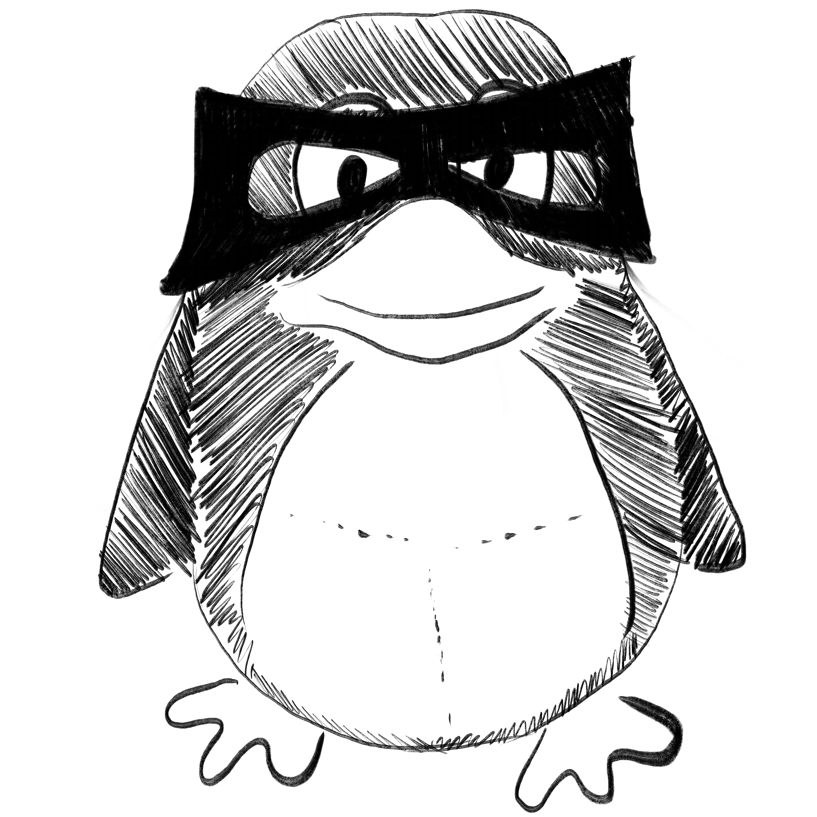
Modeling the social influence of COVID-19 via personalized propagation with deep learning.
In World wide web
Liu Yufei, Cao Jie, Wu Jia, Pi Dechang
2022-Dec-17
COVID-19, Deep learning, Personalized propagation, Social influence
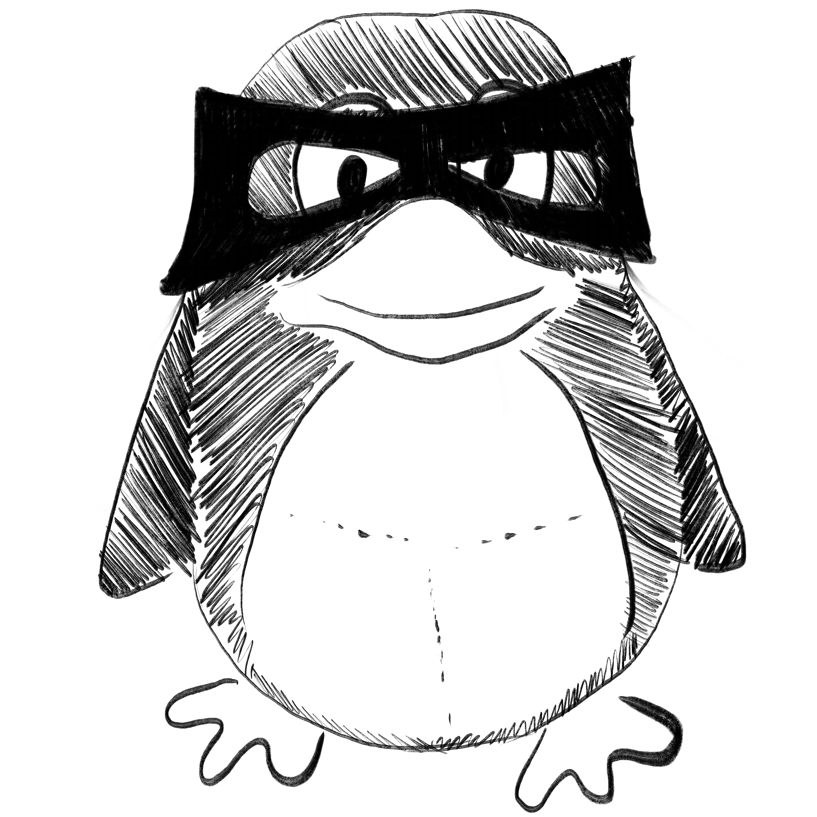
Forecasting seasonal electricity generation in European countries under Covid-19-induced lockdown using fractional grey prediction models and machine learning methods.
In Applied energy ; h5-index 131.0
Şahin Utkucan, Ballı Serkan, Chen Yan
2021-Nov-15
Covid-19, Electricity generation, Forecasting, Fractional grey model, Machine learning, Seasonal fluctuations
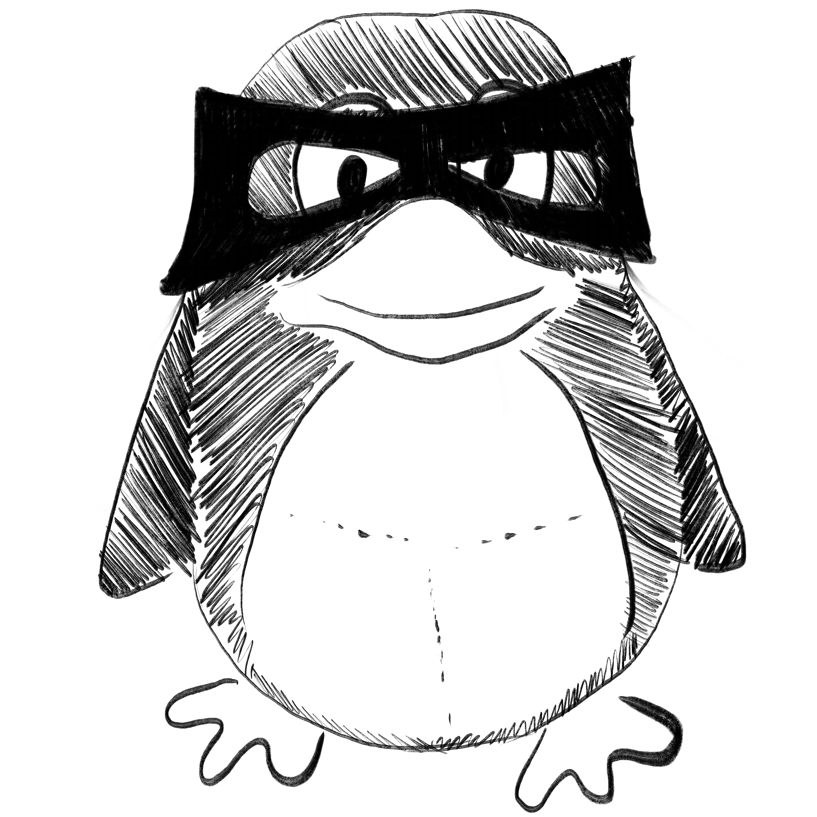
A physiological signal compression approach using optimized Spindle Convolutional Auto-encoder in mHealth applications.
In Biomedical signal processing and control
BACKGROUND AND OBJECTIVES :
RESULTS :
CONCLUSION :
Barot Vishal, Patel Dr Ritesh
2022-Mar
Data compression, Energy efficiency, Physiological signal compression, Spindle Convolutional Auto-encoder, mHealth applications
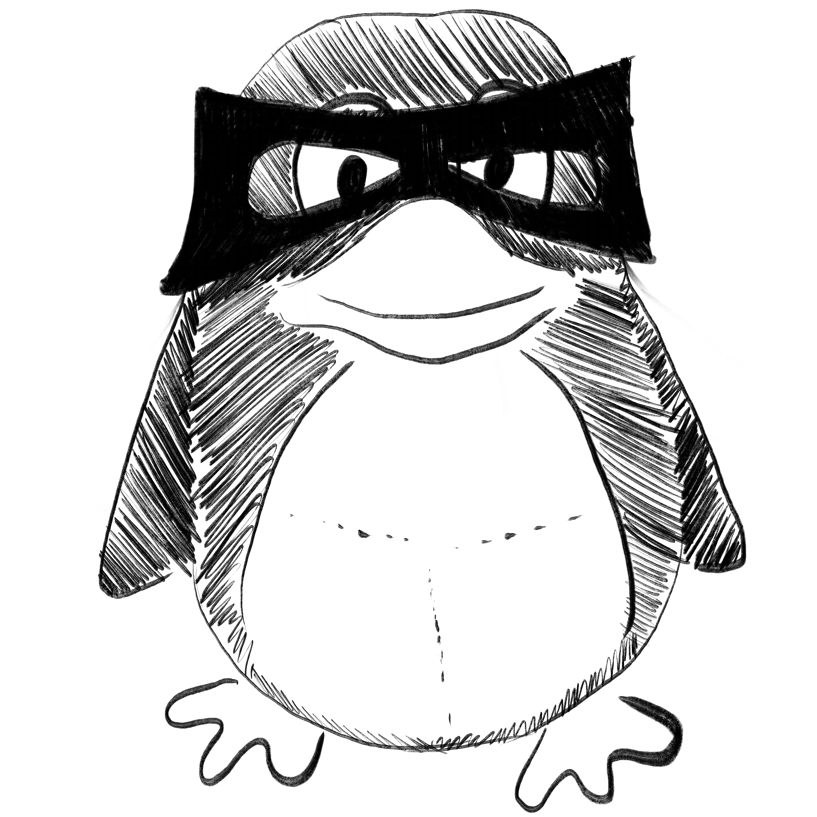
Techniques for Developing Reliable Machine Learning Classifiers Applied to Understanding and Predicting Protein:Protein Interaction Hot Spots
bioRxiv Preprint
Chen, J.; Kuhn, L. A.; Raschka, S.
2022-12-27
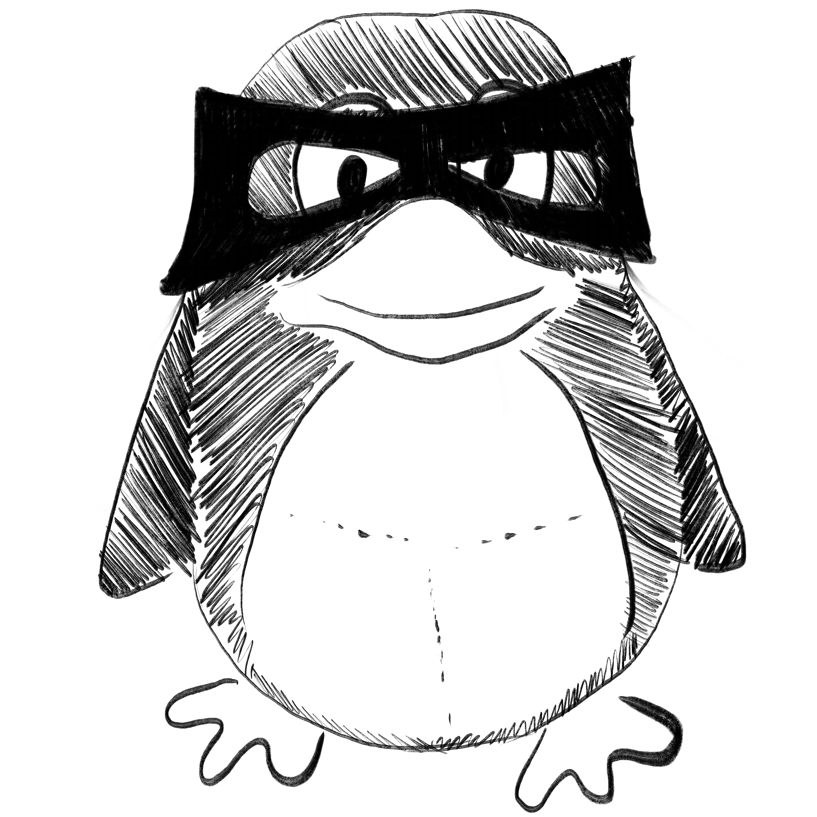
A comparative bibliometric analysis of Omicron and Delta variants during the COVID-19 pandemic.
In Annals of palliative medicine ; h5-index 20.0
BACKGROUND :
METHODS :
RESULTS :
CONCLUSIONS :
Liu Yang-Xi, Wang Li-Hui, Zhu Cheng, Zha Qiong-Fang, Yu Yue-Tian
2022-Dec-14
Bibliometric analysis, Delta variant, Omicron variant, coronavirus disease (COVID), research trends
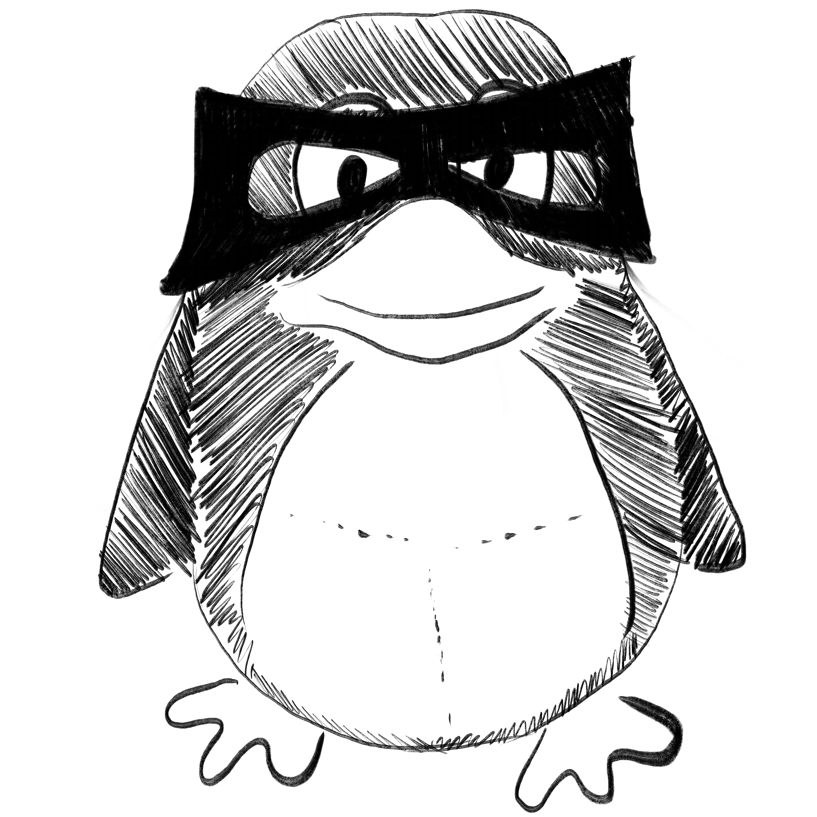
PSTCNN: Explainable COVID-19 diagnosis using PSO-guided self-tuning CNN.
In Biocell : official journal of the Sociedades Latinoamericanas de Microscopia Electronica ... et. al
Wang Wei, Pei Yanrong, Wang Shui-Hua, Gorrz Juan Manuel, Zhang Yu-Dong
2023
COVID-19, Convolutional Neural Network, Hyperparameters Tuning, Particle Swarm Optimisation, SARS-CoV-2
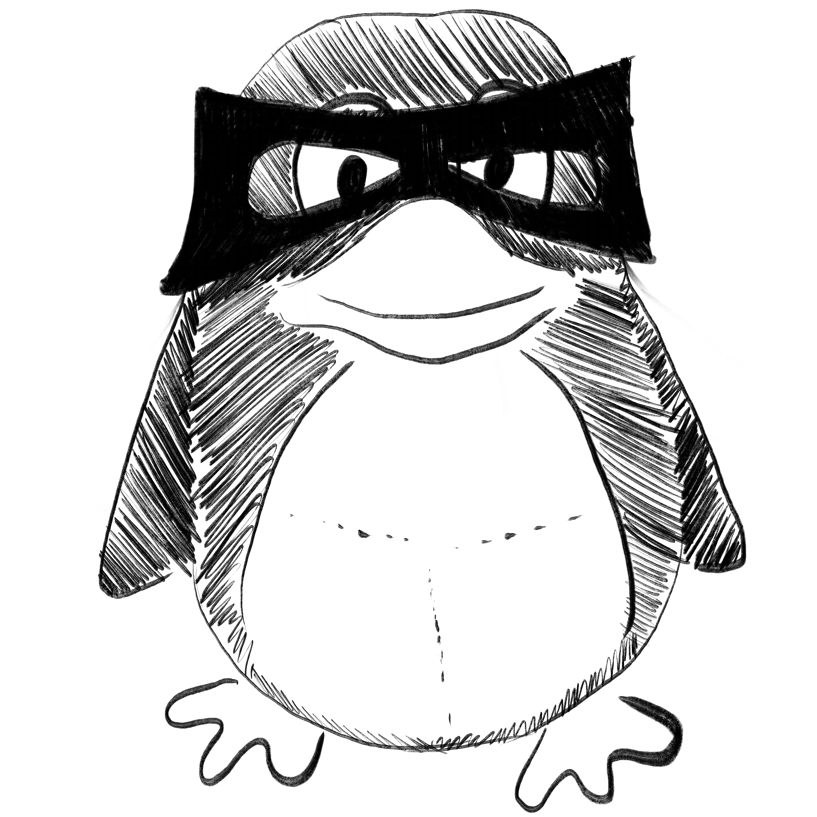
Biphasic majority voting-based comparative COVID-19 diagnosis using chest X-ray images.
In Expert systems with applications
Sunnetci Kubilay Muhammed, Alkan Ahmet
2023-Apr-15
Bag of features, COVID-19, Deep learning, Machine learning, Majority voting
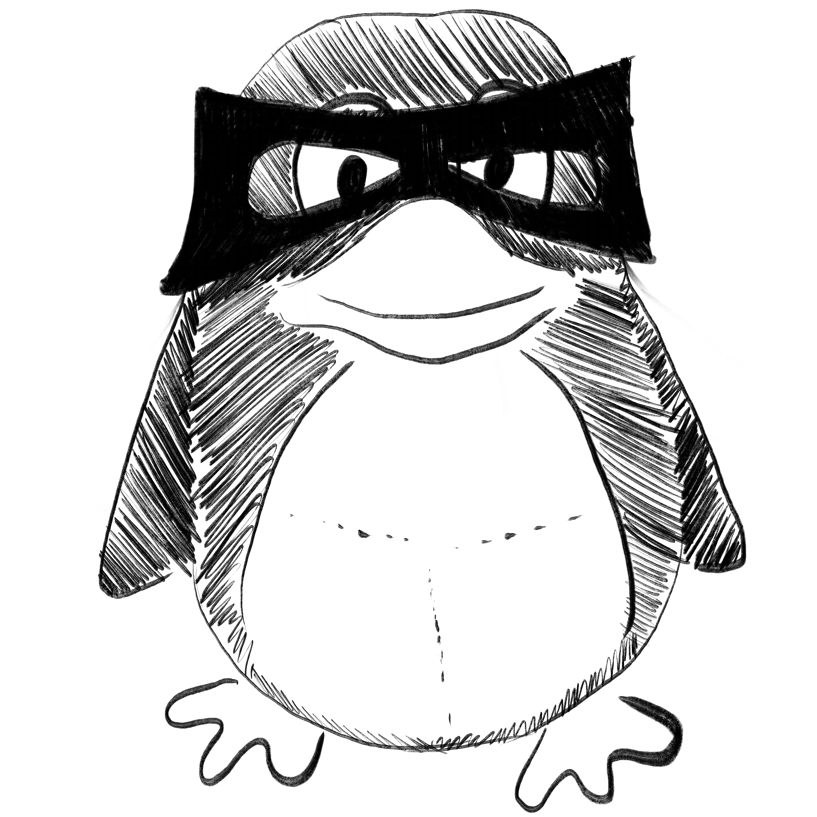
Post-pandemic healthcare for COVID-19 vaccine: Tissue-aware diagnosis of cervical lymphadenopathy via multi-modal ultrasound semantic segmentation.
In Applied soft computing
Gao Yue, Fu Xiangling, Chen Yuepeng, Guo Chenyi, Wu Ji
2023-Jan
Attention mechanism, COVID-19, Cervical lymphadenopathy, Computer-aided diagnosis, Healthcare administration, Human-in-the-loop, Image classification, Multi-modal, Semantic segmentation
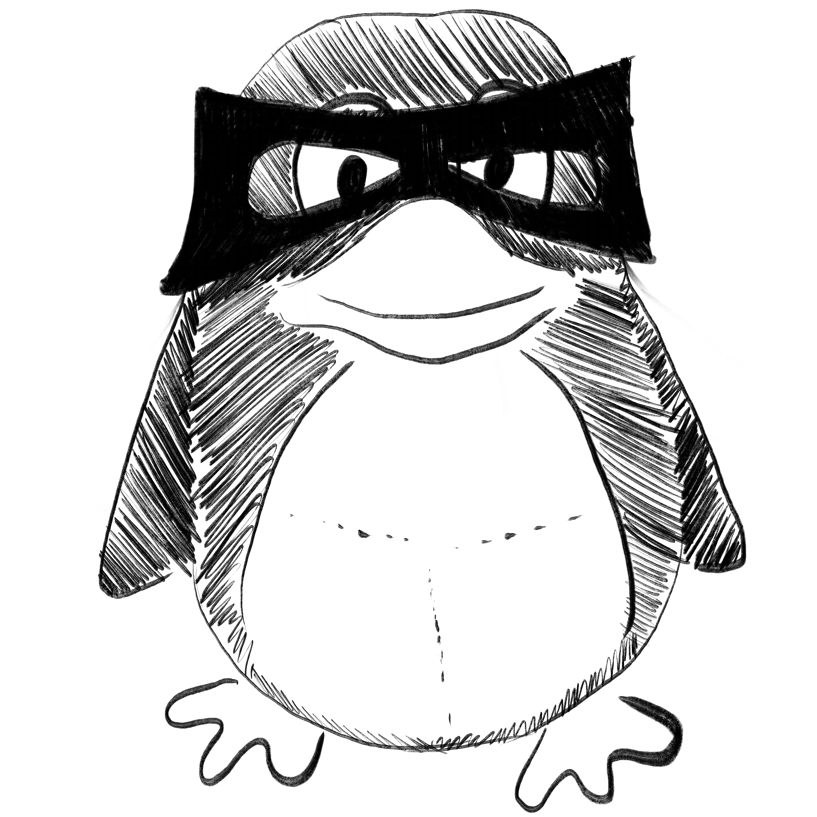
On the improvement of heart rate prediction using the combination of singular spectrum analysis and copula-based analysis approach.
In PeerJ
Namazi Asieh
2022
Artificial intelligence, Heart rate, Machine learning, Prediction, Wearable sensors
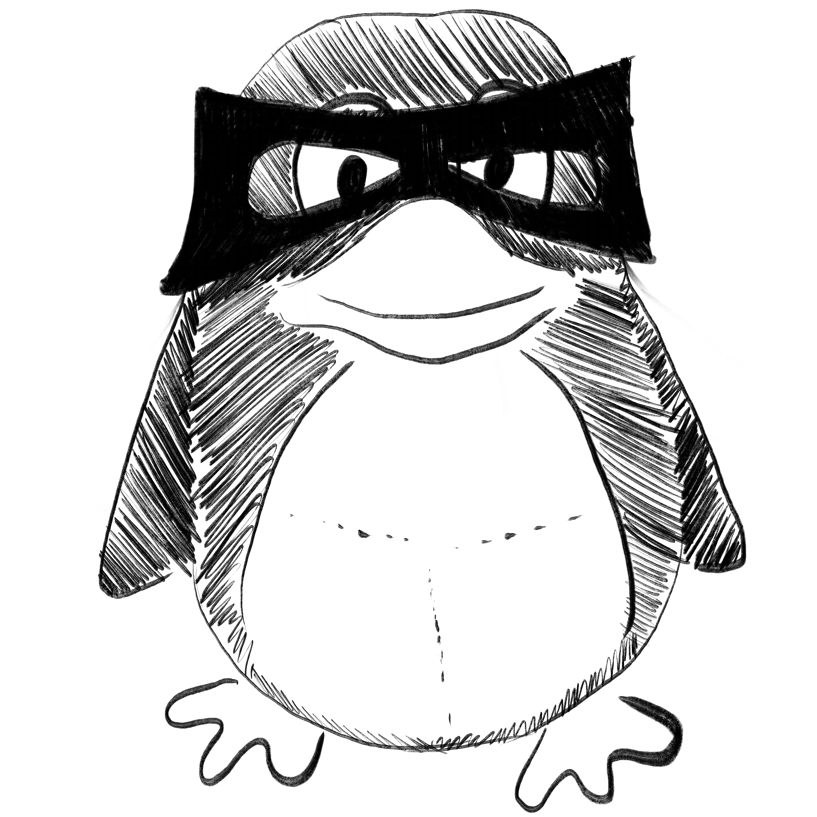
Predicting Risk of Mortality in COVID-19 Hospitalized Patients using Hybrid Machine Learning Algorithms.
In Journal of biomedical physics & engineering
BACKGROUND :
OBJECTIVE :
MATERIAL AND METHODS :
RESULTS :
CONCLUSION :
Afrash Mohammad Reza, Shanbehzadeh Mostafa, Kazemi-Arpanahi Hadi
2022-Dec
** Artificial Intelligence, Coronavirus (COVID-19), Data Mining, Machine Learning, Mortality**
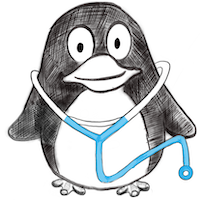
Prediction of COVID-19 Patients' Survival by Deep Learning Approaches.
In Medical journal of the Islamic Republic of Iran
Taheriyan Moloud, Ayyoubzadeh Seyed Mehdi, Ebrahimi Mehdi, R Niakan Kalhori Sharareh, Abooei Amir Hossien, Gholamzadeh Marsa, Ayyoubzadeh Seyed Mohammad
2022
COVID-19, Deep Learning, Prediction, Survival Analysis, Triage
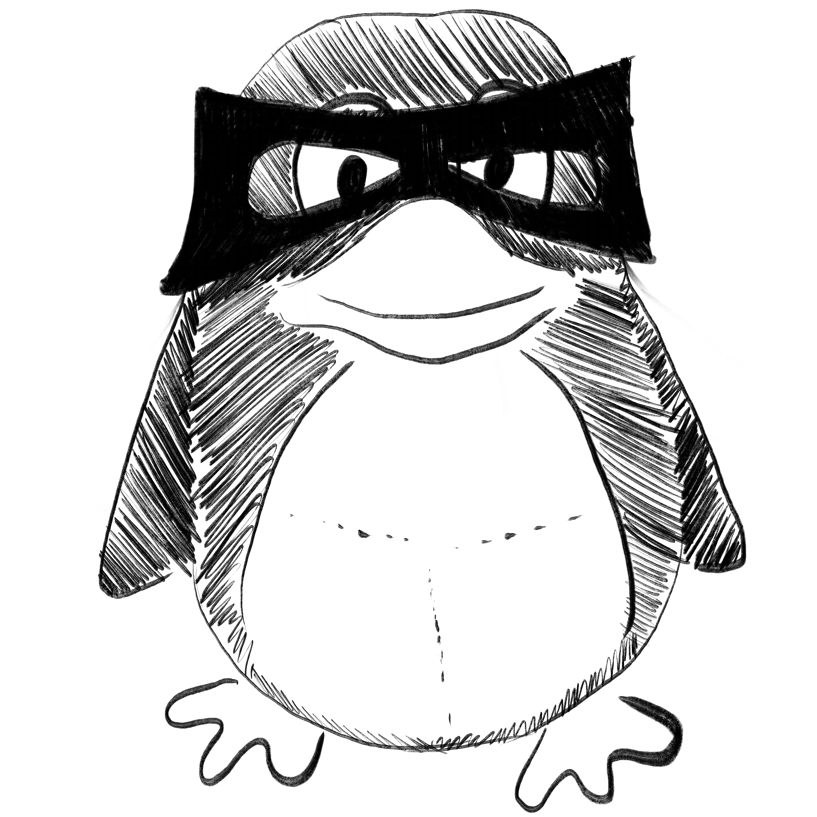
On sparse ensemble methods: An application to short-term predictions of the evolution of COVID-19.
In European journal of operational research
Benítez-Peña Sandra, Carrizosa Emilio, Guerrero Vanesa, Jiménez-Gamero M Dolores, Martín-Barragán Belén, Molero-Río Cristina, Ramírez-Cobo Pepa, Romero Morales Dolores, Sillero-Denamiel M Remedios
2021-Dec-01
COVID-19, Ensemble Method, Machine Learning, Mathematical Optimization, Selective Sparsity
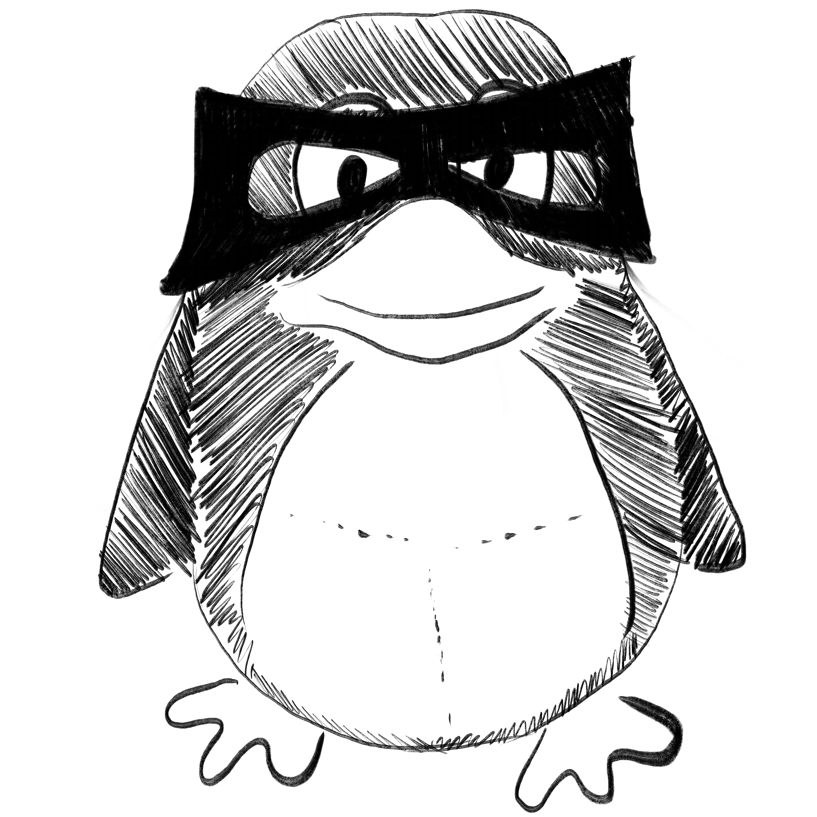
Optimism and pessimism analysis using deep learning on COVID-19 related twitter conversations.
In Information processing & management
Blanco Guillermo, Lourenço Anália
2022-May
Conversation, Covid-19 pandemic, Emotion classification, Emotion shift, Sociome
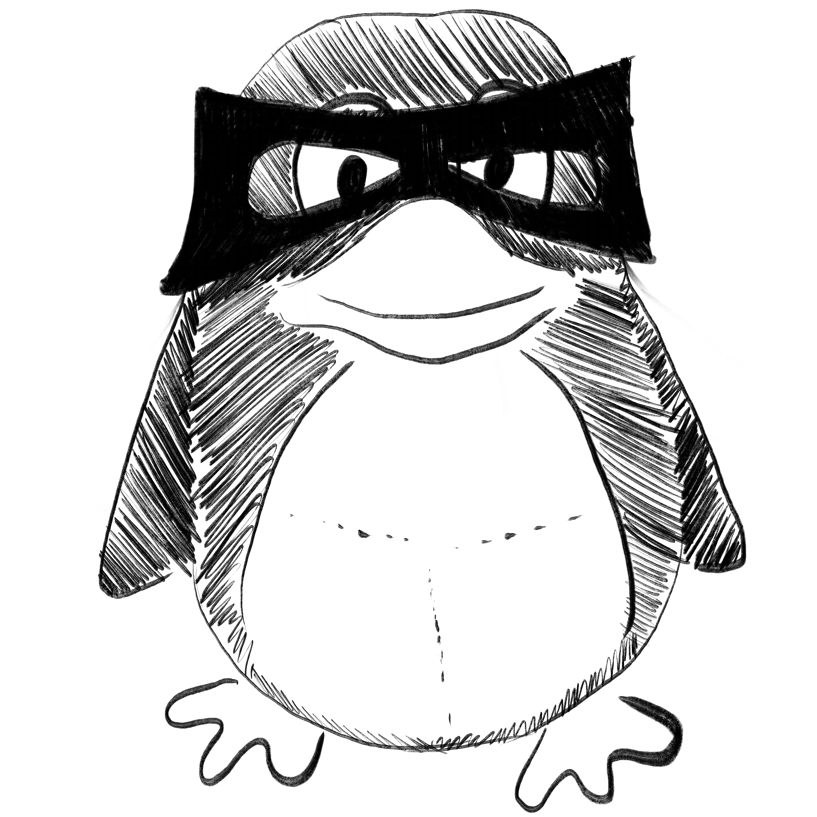
A deep learning based steganography integration framework for ad-hoc cloud computing data security augmentation using the V-BOINC system.
In Journal of cloud computing (Heidelberg, Germany)
Mawgoud Ahmed A, Taha Mohamed Hamed N, Abu-Talleb Amr, Kotb Amira
2022
Ad-hoc system, Cloud computing, Cloud security, Deep learning, Encryption, Steganography
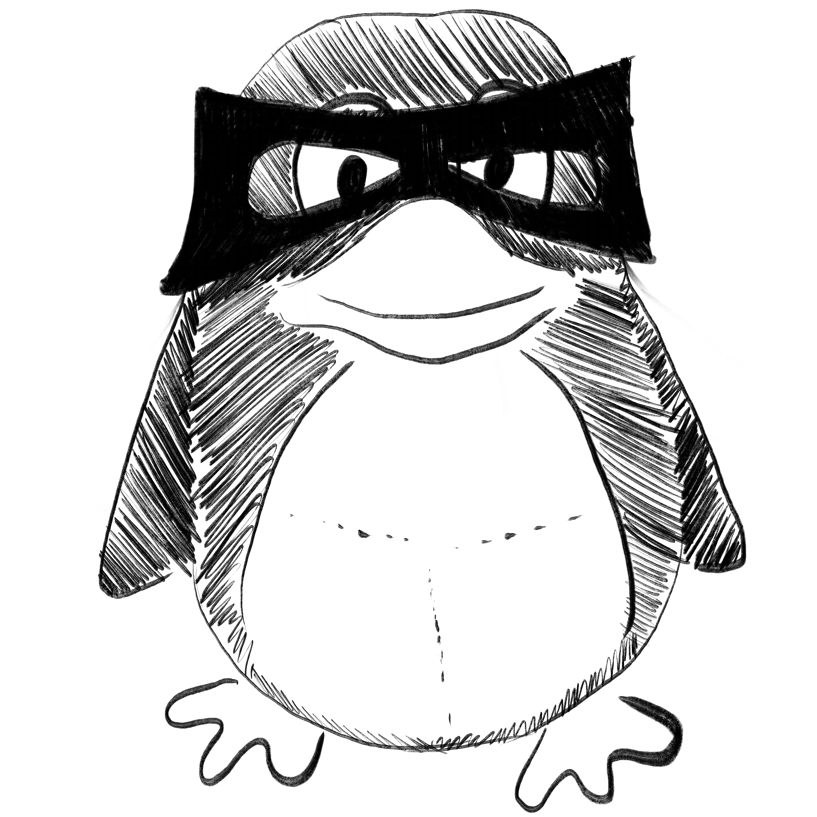
Interpretable artificial intelligence model for accurate identification of medical conditions using immune repertoire.
In Briefings in bioinformatics
Zhao Yu, He Bing, Xu Zhimeng, Zhang Yidan, Zhao Xuan, Huang Zhi-An, Yang Fan, Wang Liang, Duan Lei, Song Jiangning, Yao Jianhua
2022-Dec-24
COVID-19, TCR repertoire, artificial intelligence, diagnosis, prognosis
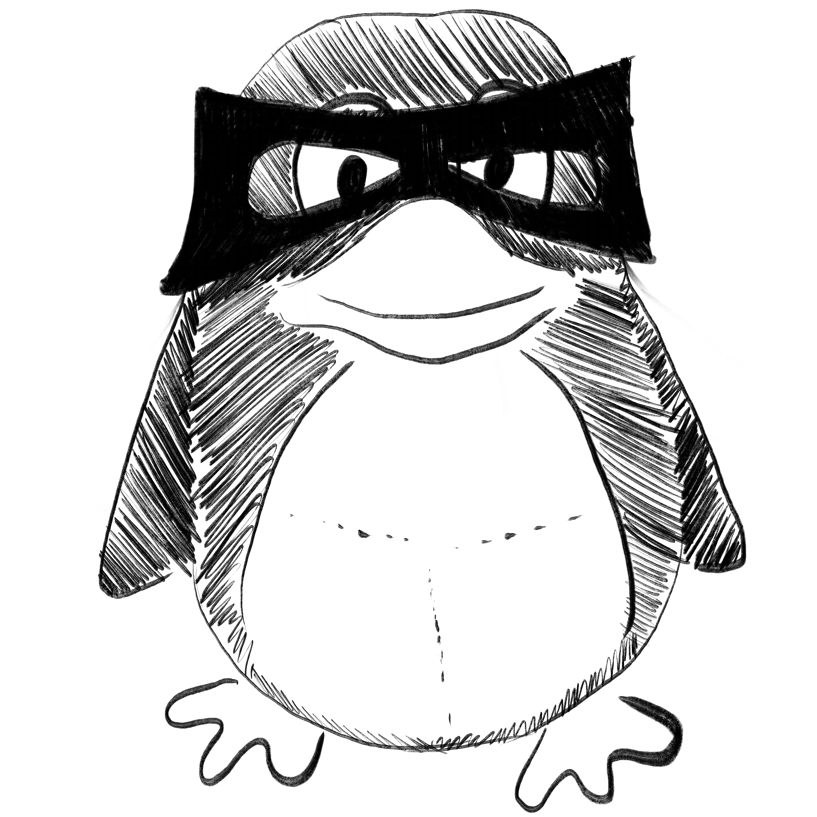
New advances in prediction and surveillance of preeclampsia: role of machine learning approaches and remote monitoring.
In Archives of gynecology and obstetrics ; h5-index 44.0
Hackelöer Max, Schmidt Leon, Verlohren Stefan
2022-Dec-25
Angiogenic factors, Artificial intelligence, Decision trees, Hypertensive pregnancy disorders, Machine learning, Multivariable modeling, Preeclampsia, Remote monitoring
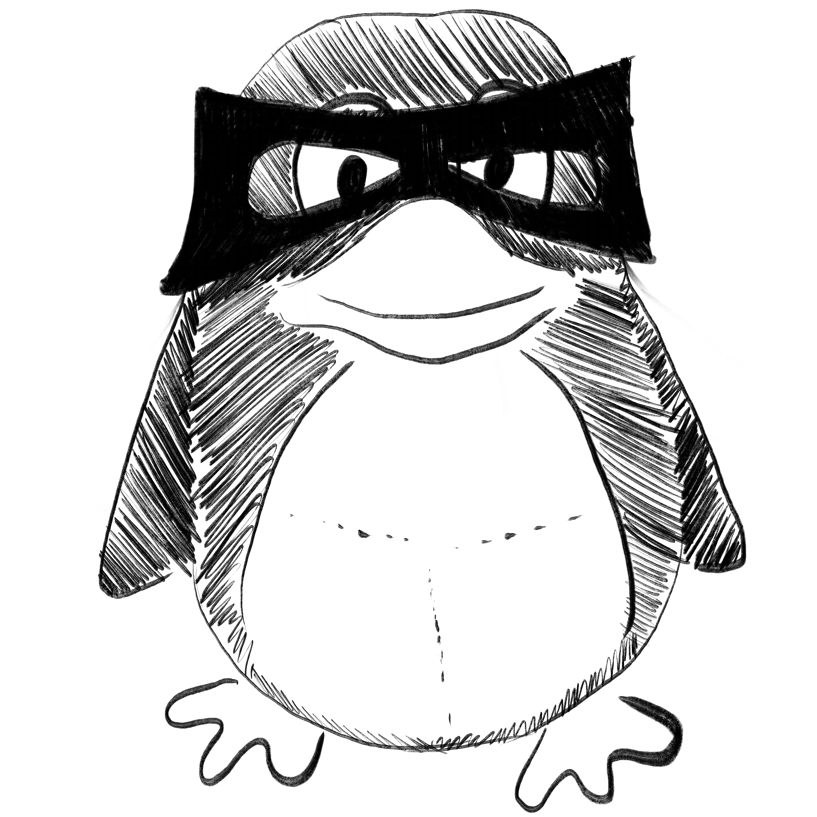
Comparing Short-Term Univariate and Multivariate Time-Series Forecasting Models in Infectious Disease Outbreak.
In Bulletin of mathematical biology
Assad Daniel Bouzon Nagem, Cara Javier, Ortega-Mier Miguel
2022-Dec-24
COVID-19, Forecasting, Multivariate approach, Outbreak disease, Univariate approach
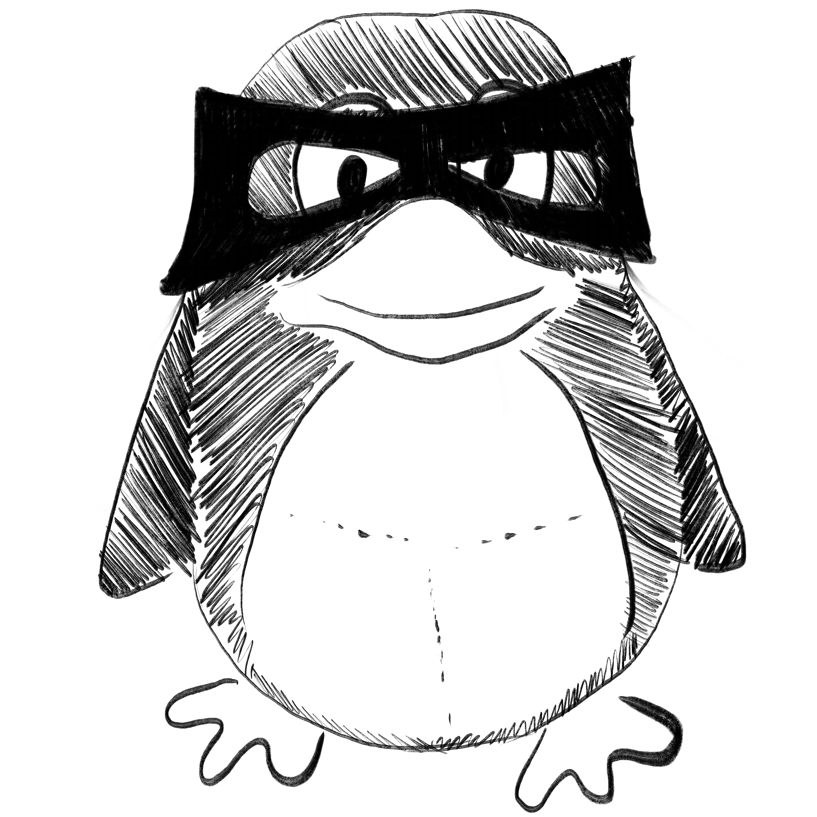
Measuring daily-life fear perception change: A computational study in the context of COVID-19.
In PloS one ; h5-index 176.0
Chai Yuchen, Palacios Juan, Wang Jianghao, Fan Yichun, Zheng Siqi
2022
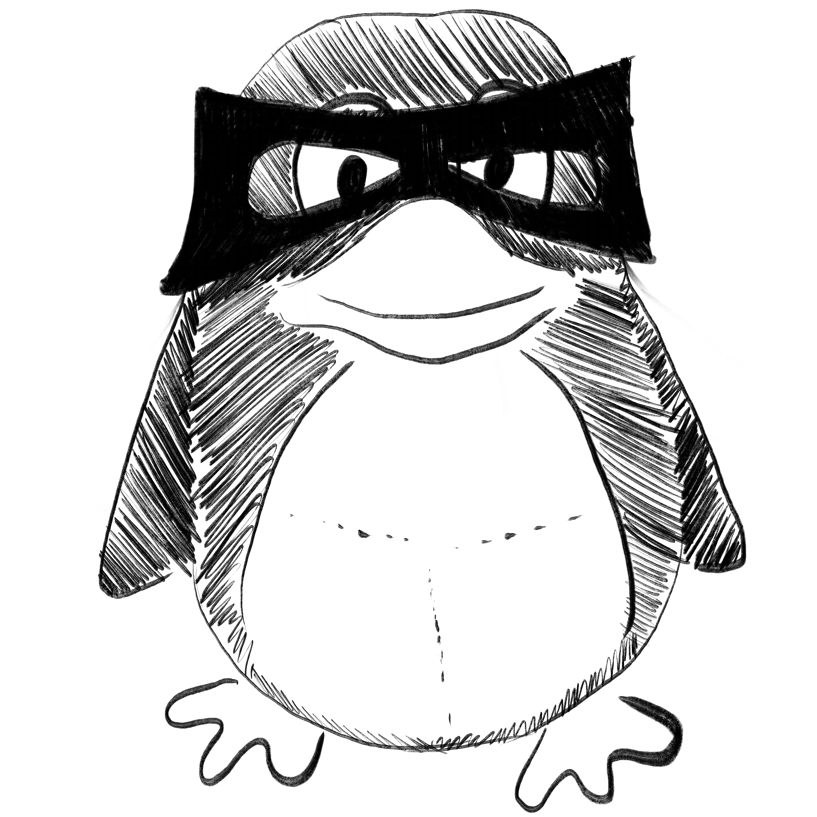
Interactive framework for Covid-19 detection and segmentation with feedback facility for dynamically improved accuracy and trust.
In PloS one ; h5-index 176.0
Sailunaz Kashfia, Bestepe Deniz, Özyer Tansel, Rokne Jon, Alhajj Reda
2022
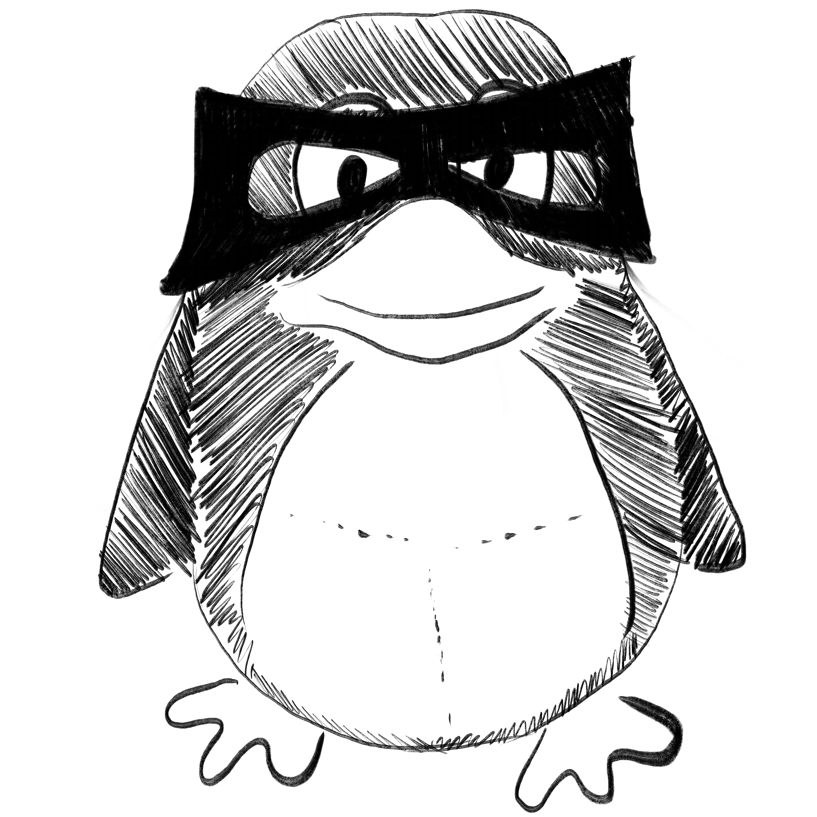
The effects of department of Veterans Affairs medical centers on socio-economic outcomes: Evidence from the Paycheck Protection Program.
In PloS one ; h5-index 176.0
Makridis Christos A, Kelly J D, Alterovitz Gil
2022
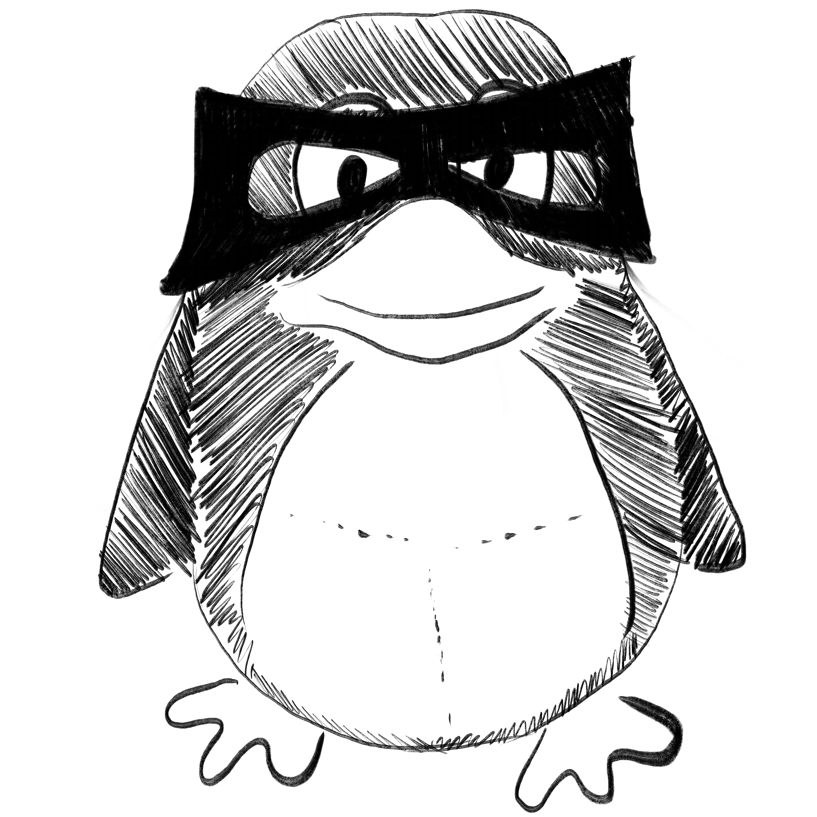
Disease Recognition in X-ray Images with Doctor Consultation-Inspired Model.
In Journal of imaging
Phung Kim Anh, Nguyen Thuan Trong, Wangad Nileshkumar, Baraheem Samah, Vo Nguyen D, Nguyen Khang
2022-Dec-05
disease recognition, doctor consultation-inspired, medical image processing
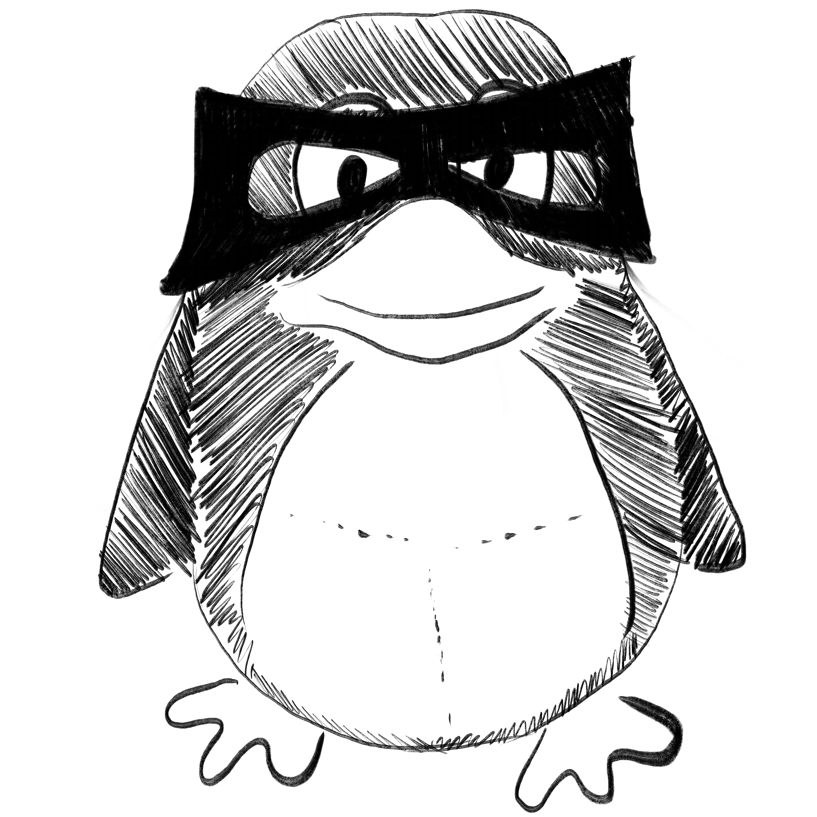
COVID-19 Outcome Prediction by Integrating Clinical and Metabolic Data using Machine Learning Algorithms.
In Revista de investigacion clinica; organo del Hospital de Enfermedades de la Nutricion
BACKGROUND :
OBJECTIVES :
METHODS :
RESULTS :
CONCLUSIONS :
Villagrana-Bañuelos Karen E, Maeda-Gutiérrez Valeria, Alcalá-Rmz Vanessa, Oropeza-Valdez Juan J, Herrera-Van Oostdam Ana S, Castañeda-Delgado Julio E, López Jesús Adrián, Borrego Moreno Juan C, Galván-Tejada Carlos E, Galván-Tejeda Jorge I, Gamboa-Rosales Hamurabi, Luna-García Huizilopoztli, Celaya-Padilla José M, López-Hernández Yamilé
2022
Biomarker, COVID-19, Genetic algorithm, LC-MS, Machine learning, Metabolomics, Random forest
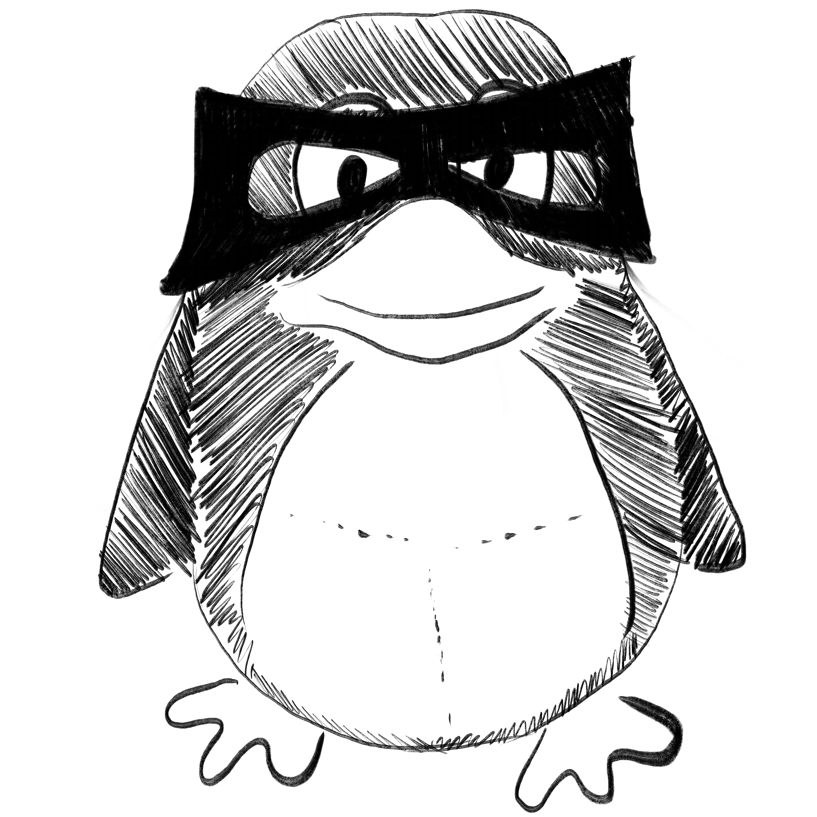
The role of advanced technologies against COVID-19: Prevention, Detection, and Treatments.
In Current stem cell research & therapy
Hasanzadeh Elham, Rafati Adele, Tamijani Seyedeh Masoumeh Seyed Hosseini, Rafaiee Raheleh, Golchin Ali, Abasi Mozhgan
2022-Dec-21
Artificial intelligence, COVID-19, Nanotechnology, Nerve stimulation, Stem cell therapy, Tissue engineering
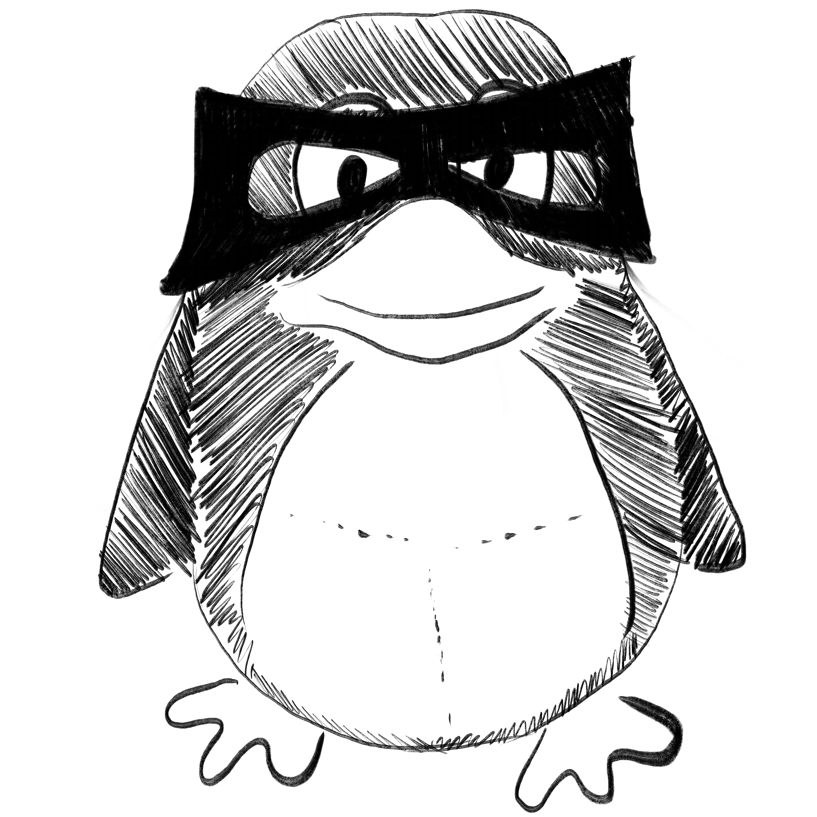
Physical fitness changes in adolescents due to social distancing during the coronavirus disease pandemic in Korea.
In PeerJ
BACKGROUND :
METHODS :
RESULTS :
CONCLUSIONS :
Lee Kwang-Jin, Seon Se-Young, Noh Byungjoo, An Keun-Ok
2022
Middle school students, Physical activity, Physical fitness level
Mediating Role of Fine Particles Abatement on Pediatric Respiratory Health During COVID-19 Stay-at-Home Order in San Diego County, California.
In GeoHealth
Aguilera Rosana, Leibel Sydney, Corringham Thomas, Bialostozky Mario, Nguyen Margaret B, Gershunov Alexander, Benmarhnia Tarik
2022-Sep
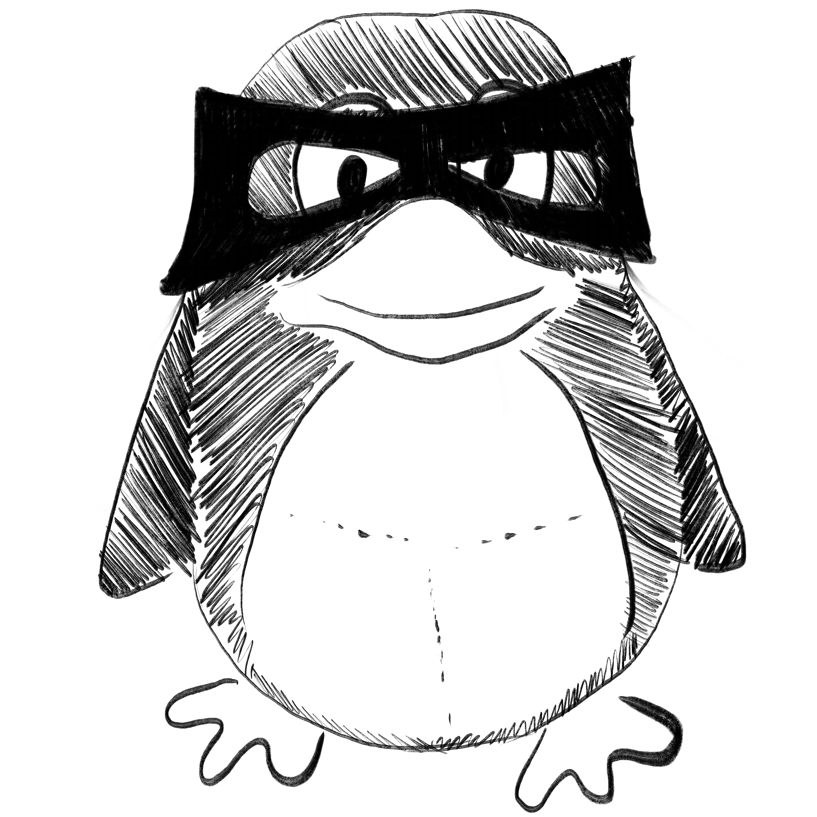
Drug repositioning for SARS-CoV-2 by Gaussian kernel similarity bilinear matrix factorization.
In Frontiers in microbiology
Wang Yibai, Xiang Ju, Liu Cuicui, Tang Min, Hou Rui, Bao Meihua, Tian Geng, He Jianjun, He Binsheng
2022
SARS-CoV-2, bilinear matrix factorization, drug repositioning, machine learning, molecular docking
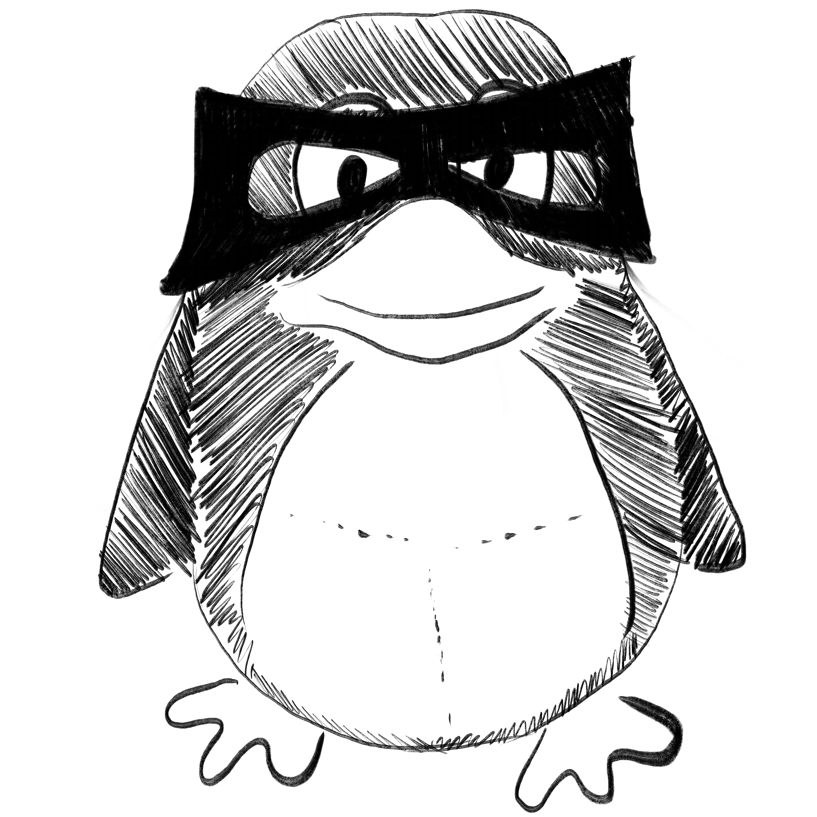
Artificial Neural Networks for the Prediction of Monkeypox Outbreak.
In Tropical medicine and infectious disease
Manohar Balakrishnama, Das Raja
2022-Dec-08
COVID-19, Hessian matrix, K-fold cross-validation, Levenberg–Marquardt model, machine learning, regression analysis, sigmoid function
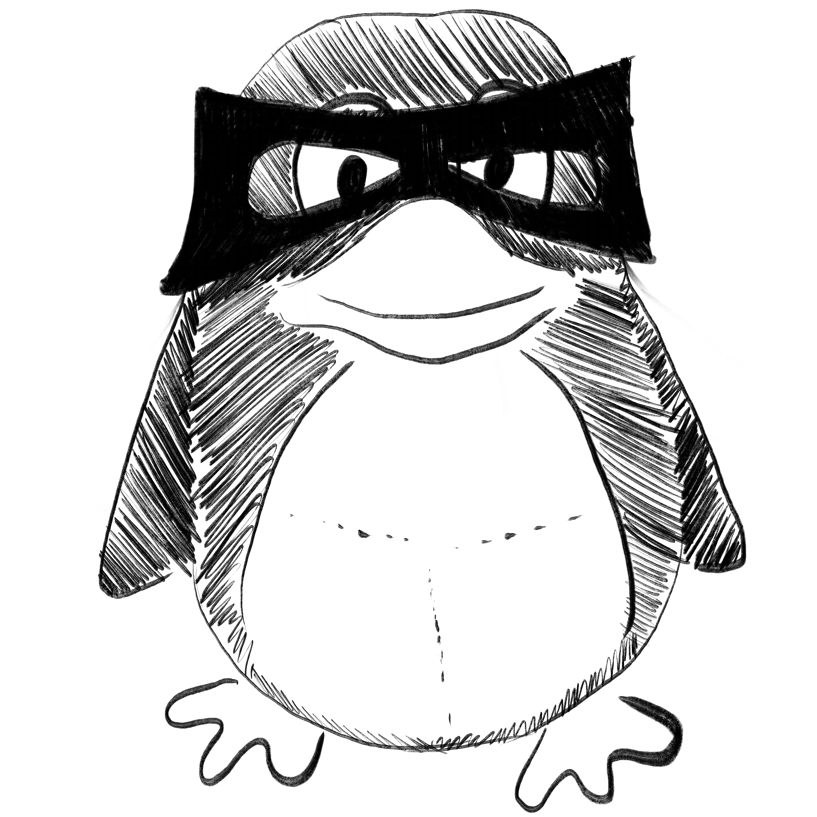
Artificial Neural Networks for the Prediction of Monkeypox Outbreak.
In Tropical medicine and infectious disease
Manohar Balakrishnama, Das Raja
2022-Dec-08
COVID-19, Hessian matrix, K-fold cross-validation, Levenberg–Marquardt model, machine learning, regression analysis, sigmoid function
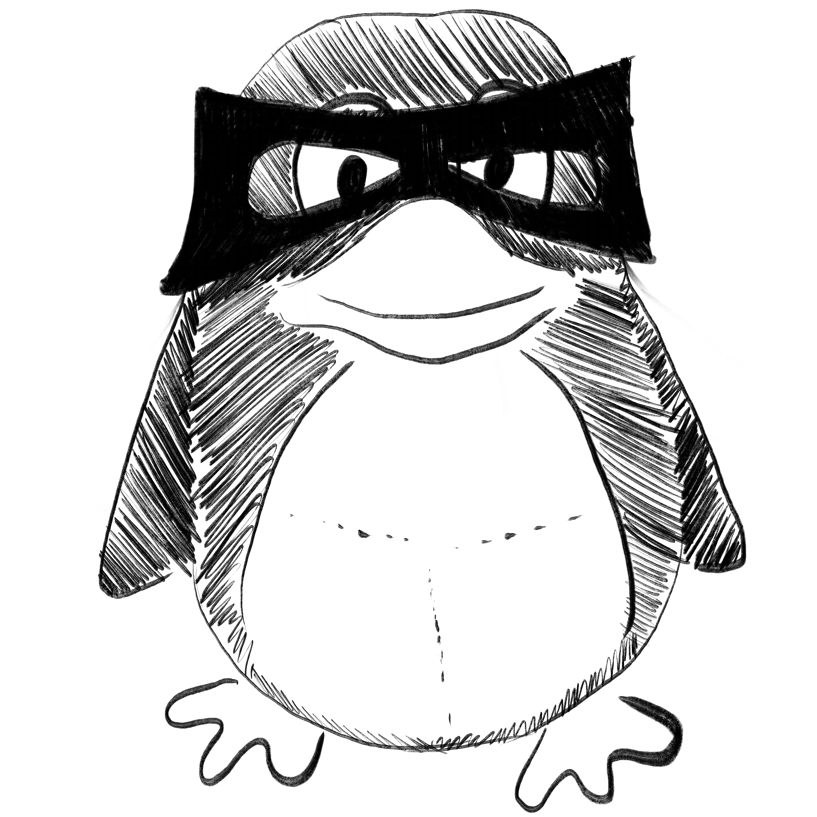
Prediction and assessment of the impact of COVID-19 lockdown on air quality over Kolkata: a deep transfer learning approach.
In Environmental monitoring and assessment
Dutta Debashree, Pal Sankar K
2022-Dec-22
Air quality, Bidirectional LSTM, COVID-19, Deep learning, Lockdown, PM10, PM2.5, Random forest, Transfer learning
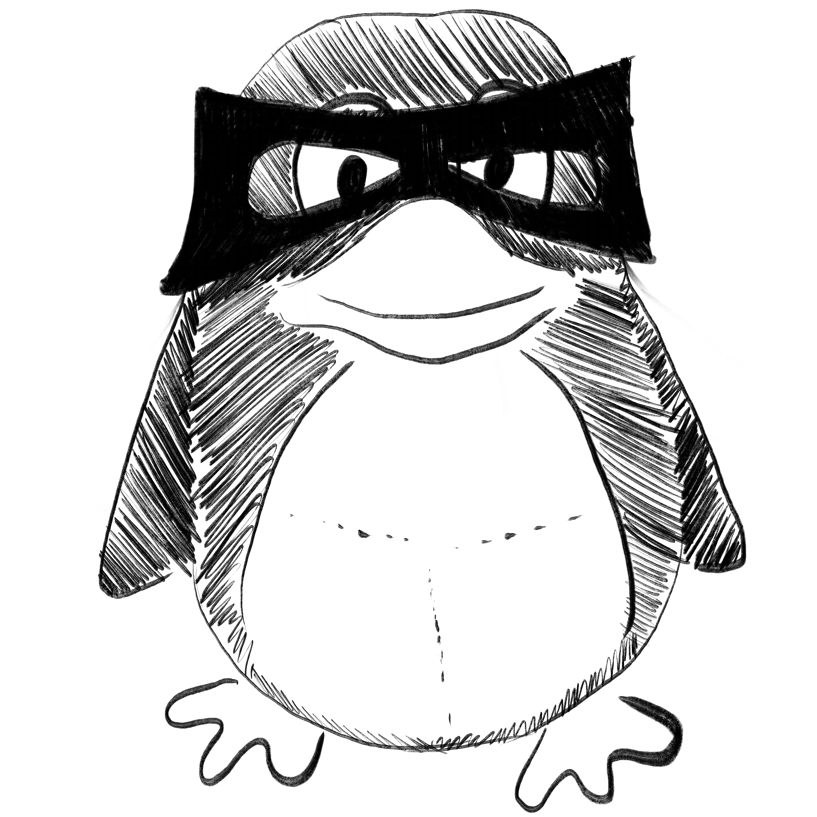
Maimuna (Maia) Majumder.
In Cell reports. Medicine
**
2022-Dec-20
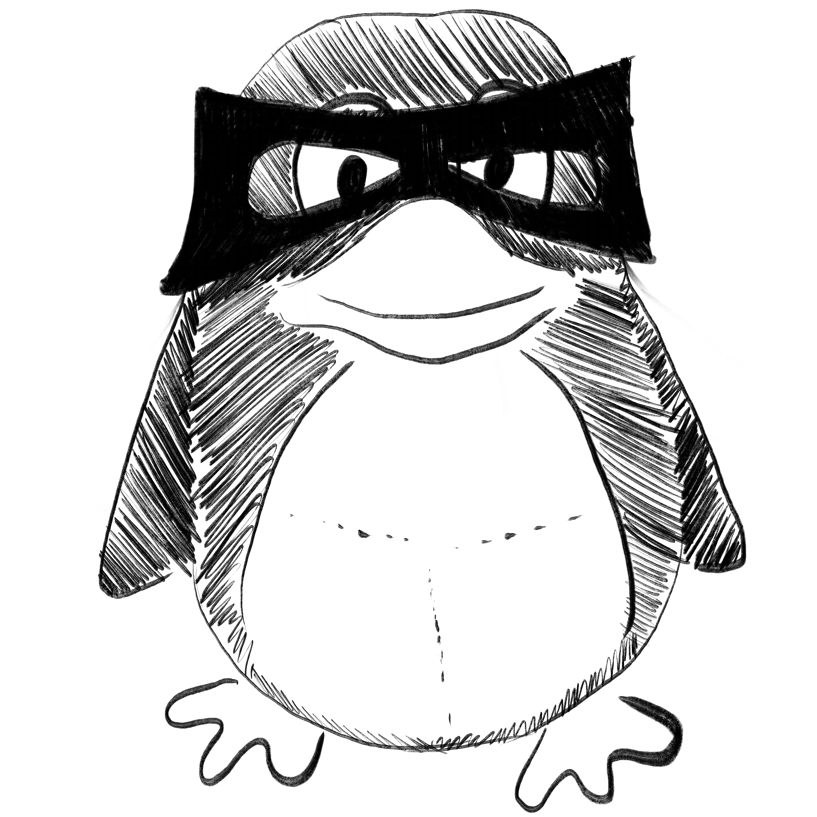
Improving COVID-19 CT classification of CNNs by learning parameter-efficient representation.
In Computers in biology and medicine
Xu Yujia, Lam Hak-Keung, Jia Guangyu, Jiang Jian, Liao Junkai, Bao Xinqi
2022-Dec-15
CNNs, COVID-19, Computed tomography, Deep learning, Similarity regularization
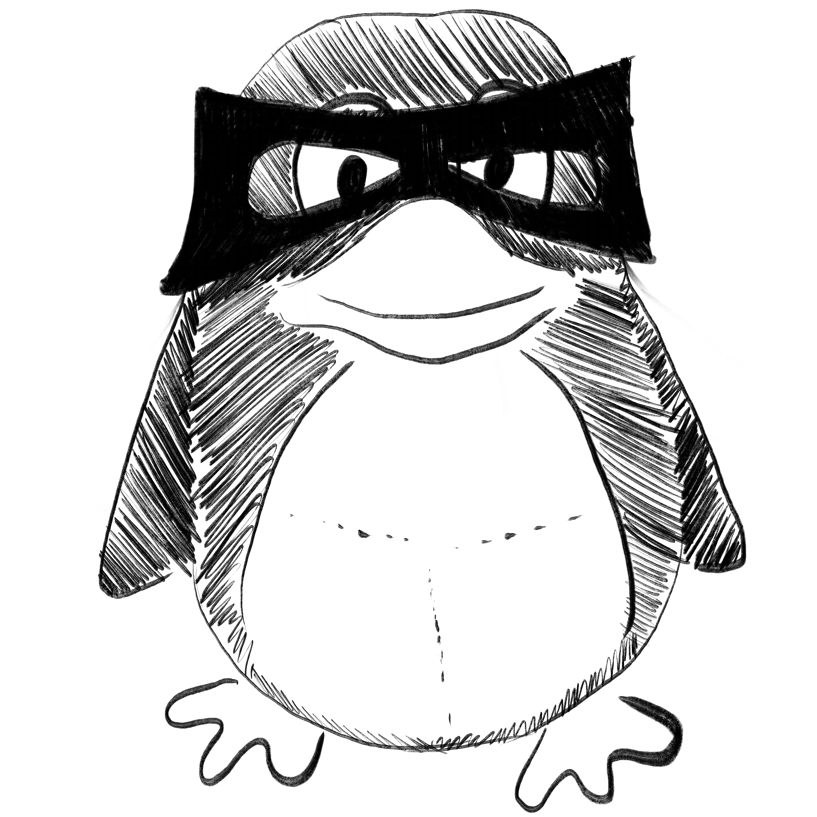
Evaluation of shelter dog activity levels before and during COVID-19 using automated analysis.
In Applied animal behaviour science ; h5-index 32.0
Byosiere Sarah-Elizabeth, Feighelstein Marcelo, Wilson Kristiina, Abrams Jennifer, Elad Guy, Farhat Nareed, van der Linden Dirk, Kaplun Dmitrii, Sinitca Aleksandr, Zamansky Anna
2022-May
Applied behavior, COVID-19, Computer vision, Dog behavior, Machine learning, Shelter research
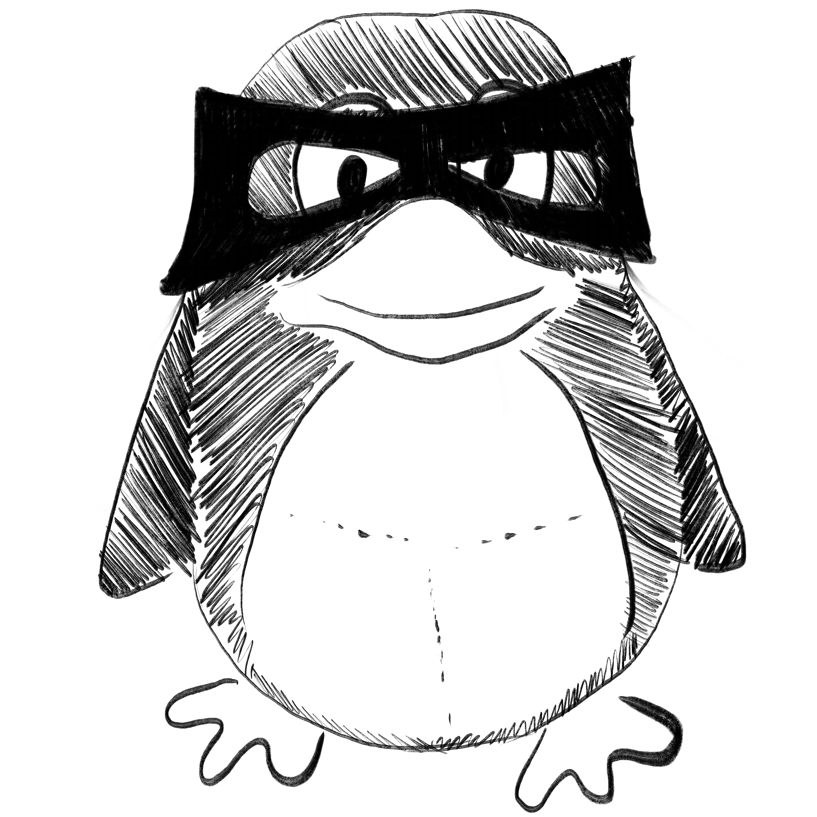
Reliability of crowdsourced data and patient-reported outcome measures in cough-based COVID-19 screening.
In Scientific reports ; h5-index 158.0
Xiong Hao, Berkovsky Shlomo, Kâafar Mohamed Ali, Jaffe Adam, Coiera Enrico, Sharan Roneel V
2022-Dec-20
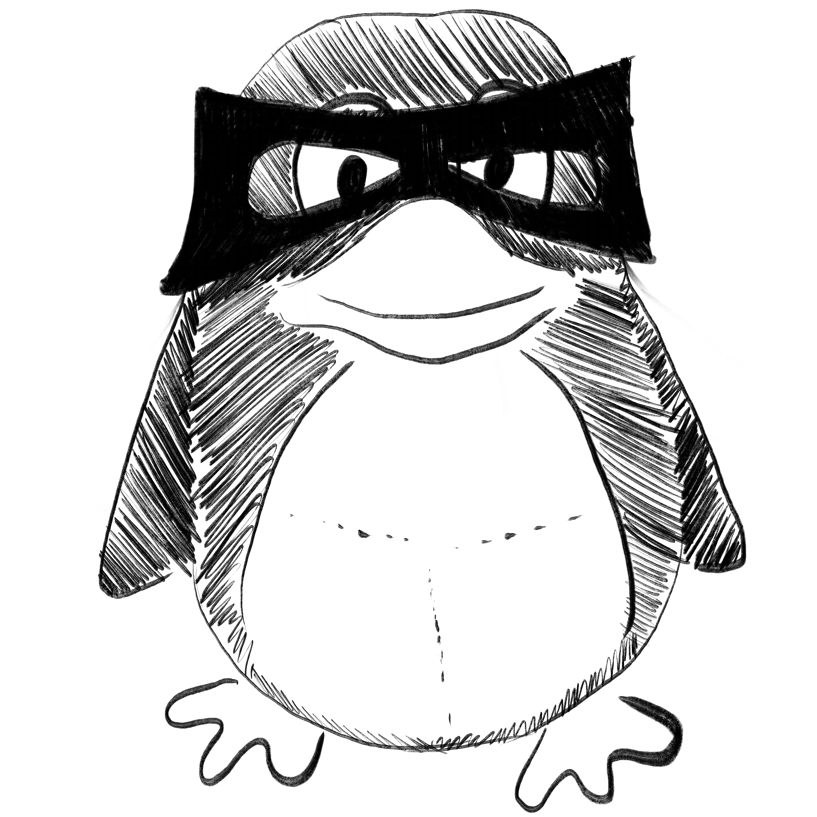
Dementia Analytics Research User Group (DARUG) - A Model for meaningful stakeholder engagement in dementia research.
In Alzheimer's & dementia : the journal of the Alzheimer's Association
BACKGROUND :
METHOD :
RESULTS :
CONCLUSION :
Carlin Paul, Wallace Jonathan, Moore Adrian, Hughes Catherine, Black Michaela, Rankin Deborah, Hoey Leane, McNulty Helene
2022-Dec
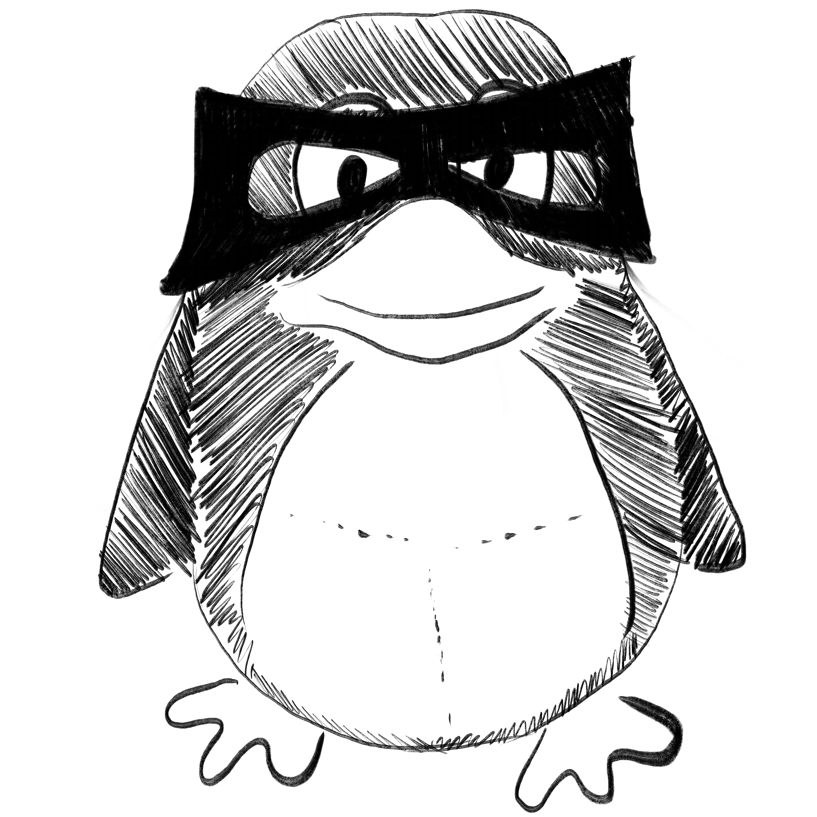
Drug development process and COVID-19 pandemic: Flourishing era of outsourcing.
In Indian journal of pharmacology
Wasan Himika, Singh Devendra, Reeta K H, Gupta Pooja, Gupta Yogendra Kumar
2022
Artificial intelligence, COVID-19, contract research organizations, drug development, outsourcing
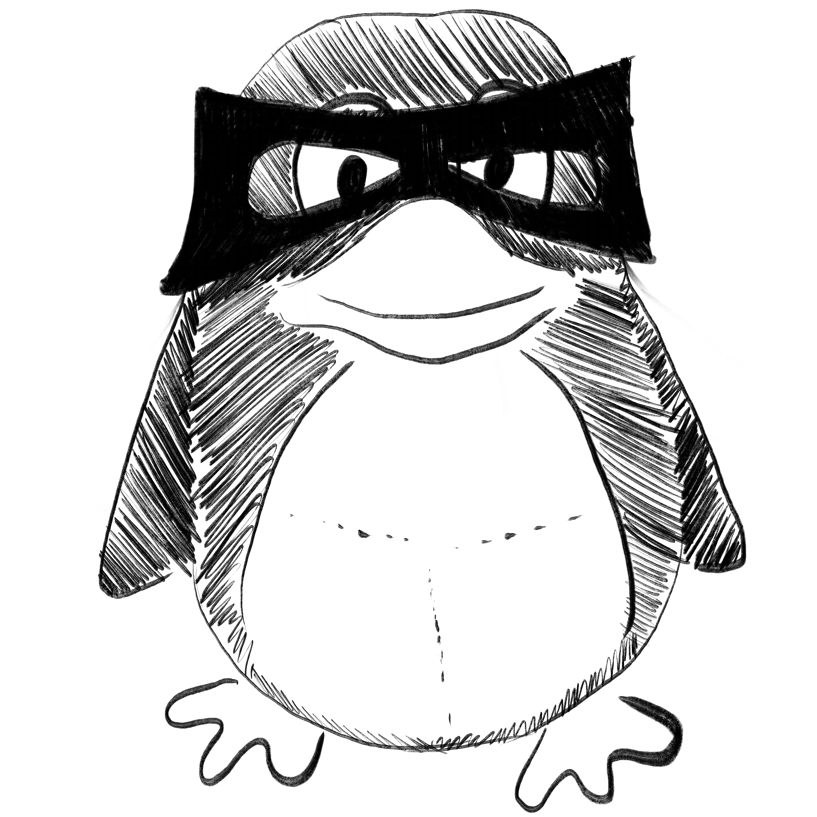
D-Cov19Net: A DNN based COVID-19 detection system using lung sound.
In Journal of computational science
Chatterjee Sukanya, Roychowdhury Jishnu, Dey Anilesh
2022-Dec-15
Auto-diagnosis system, COVID-19 Detection, Convolution Neural Network (CNN), Deep Learning, Lung/Respiratory sound
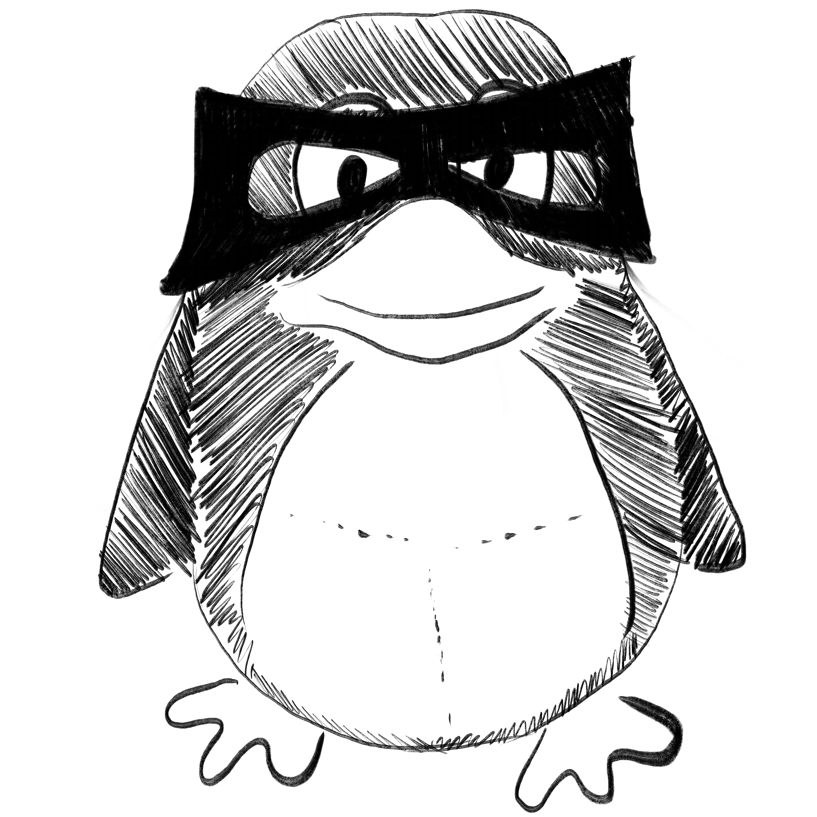
A residual network-based framework for COVID-19 detection from CXR images.
In Neural computing & applications
Kibriya Hareem, Amin Rashid
2022-Dec-15
COVID-19, CXR images, Machine learning, ResNet50, SVM
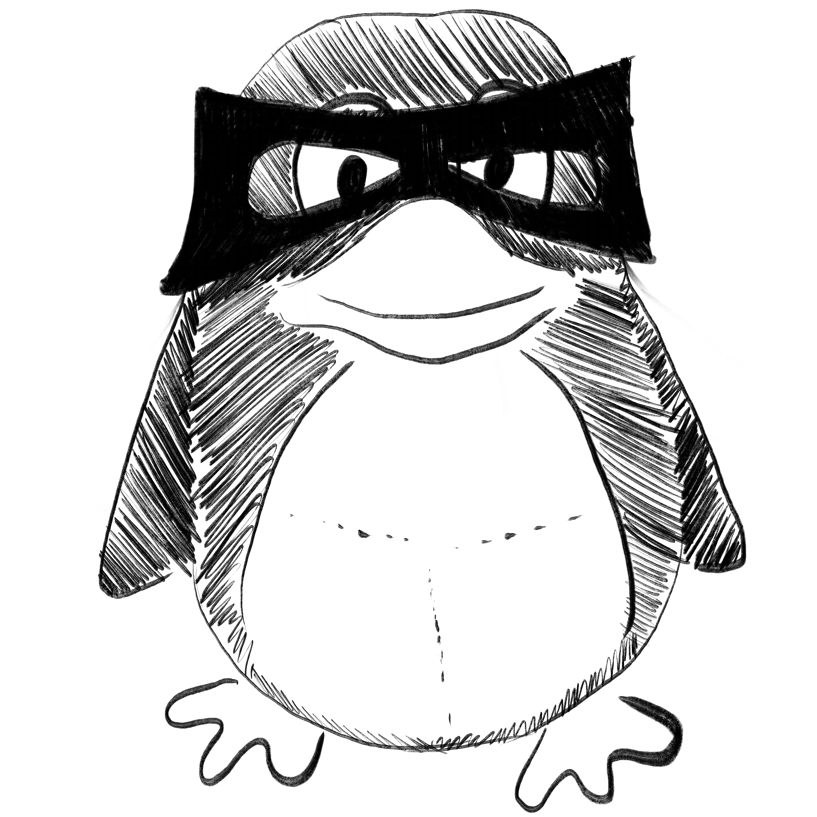
TransMUSE: Transferable Traffic Prediction in MUlti-Service Edge Networks.
In Computer networks
Xu Luyang, Liu Haoyu, Song Junping, Li Rui, Hu Yahui, Zhou Xu, Patras Paul
2023-Feb
Edge model transfer, Multi-service traffic prediction, Service clustering
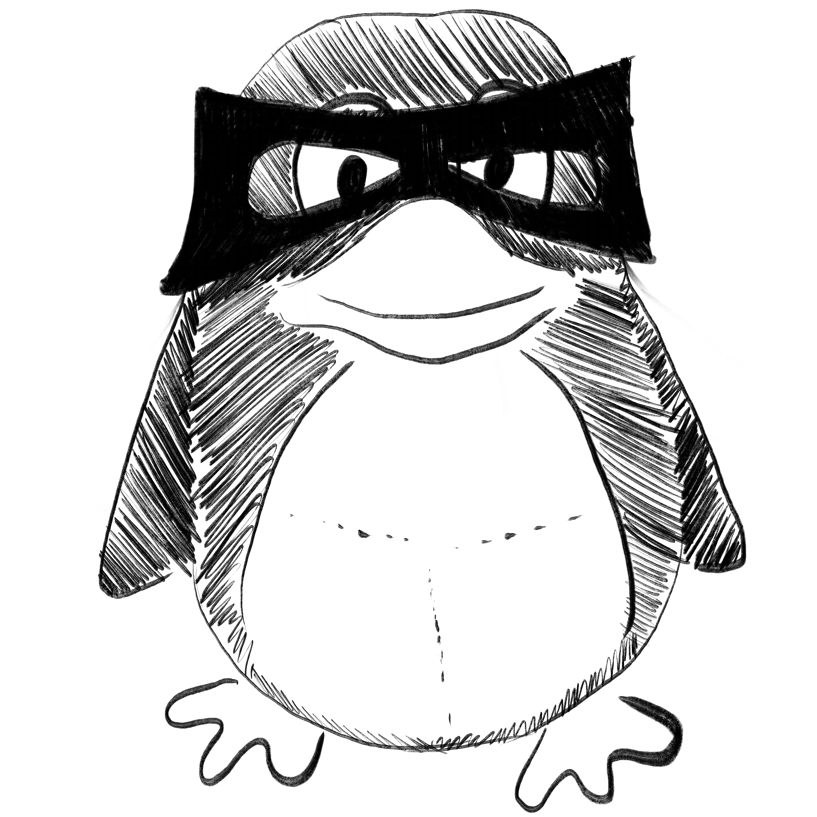
A multi-task fully deep convolutional neural network for contactless fingerprint minutiae extraction.
In Pattern recognition
Zhang Zhao, Liu Shuxin, Liu Manhua
2021-Dec
00-01, 99-00, Contactless fingerprint, Deep convolutional neural network, Minutiae extraction, Multi-task learning
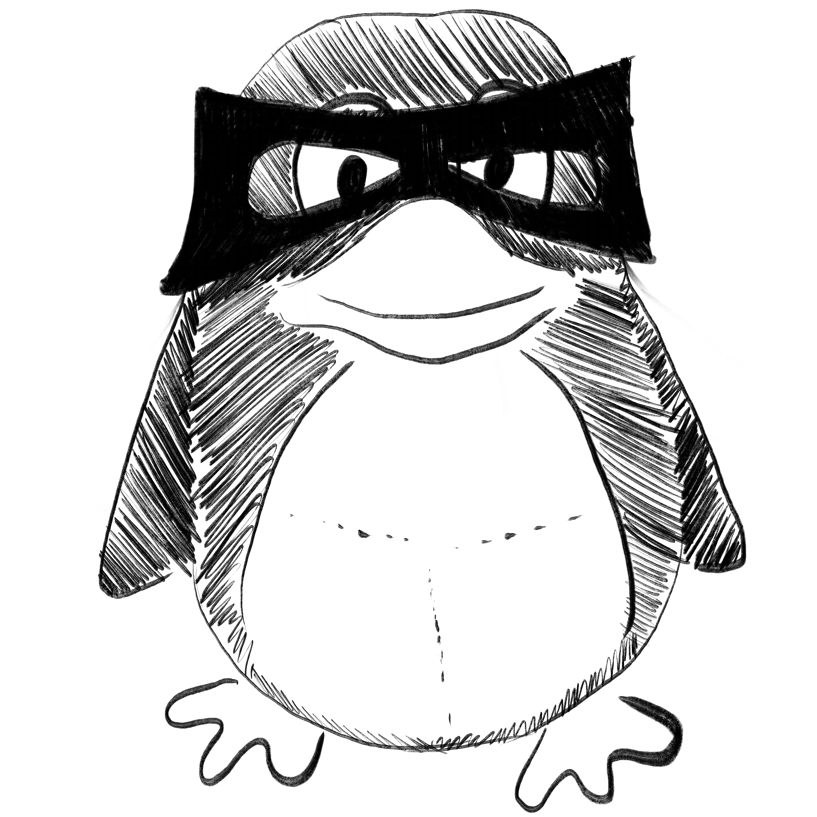
Altered somatic hypermutation patterns in COVID-19 patients classifies disease severity
bioRxiv Preprint
Safra, M.; Tamari, Z.; Polak, P.; Shiber, S.; Matan, M.; Karameh, H.; Helviz, Y.; Levy-Barda, A.; Yahalom, V.; Peretz, A.; Ben-Chetrit, E.; Brenner, B.; Tuller, T.; Gal-Tanamy, M.; Yaari, G.
2022-12-21
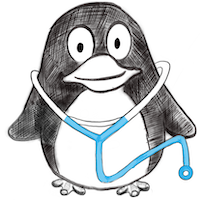
Predicting oxygen requirements in patients with coronavirus disease 2019 using an artificial intelligence-clinician model based on local non-image data.
In Frontiers in medicine
BACKGROUND :
MATERIALS AND METHODS :
RESULTS :
CONCLUSION :
Muto Reiko, Fukuta Shigeki, Watanabe Tetsuo, Shindo Yuichiro, Kanemitsu Yoshihiro, Kajikawa Shigehisa, Yonezawa Toshiyuki, Inoue Takahiro, Ichihashi Takuji, Shiratori Yoshimune, Maruyama Shoichi
2022
COVID-19, artificial intelligence-human collaboration, clinical practice, oxygen needs, sodium chloride difference
An NLP tool for data extraction from electronic health records: COVID-19 mortalities and comorbidities.
In Frontiers in public health
BACKGROUND :
METHOD :
RESULTS :
CONCLUSION :
BuHamra Sana S, Almutairi Abdullah N, Buhamrah Abdullah K, Almadani Sabah H, Alibrahim Yusuf A
2022
SARS-CoV-2, decision tree, information extraction, mortality, natural language processing, prediction, text mining
Can a chatbot enhance hazard awareness in the construction industry?
In Frontiers in public health
Zhu Xiaoe, Li Rita Yi Man, Crabbe M James C, Sukpascharoen Khunanan
2022
chatbot safety training, construction hazard awareness, construction practitioners, design of experiment, eye-tracking
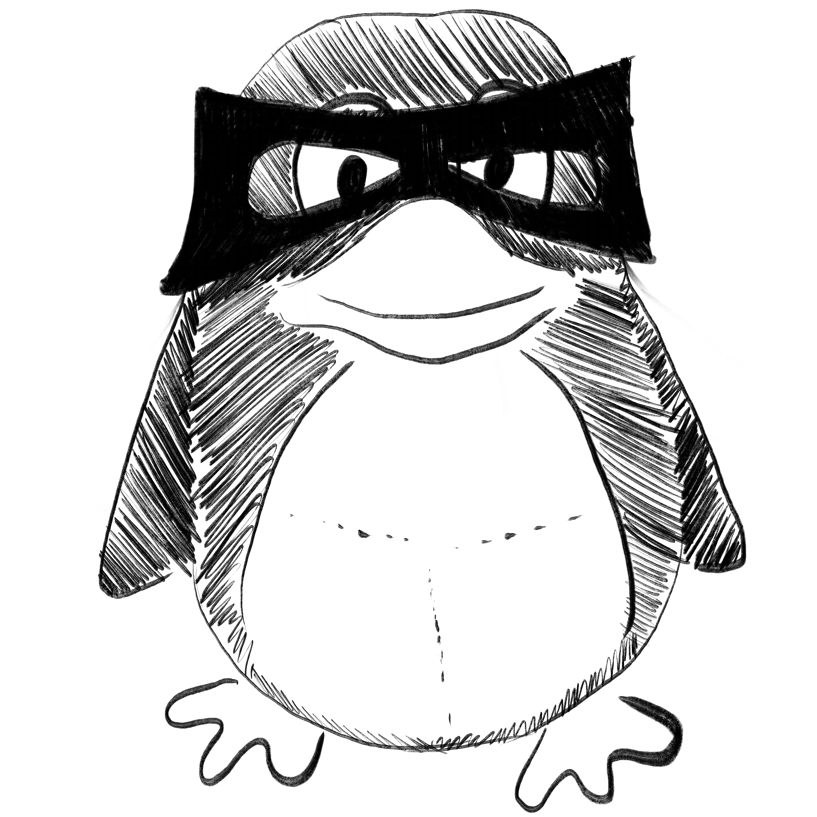
An artificial neural network classification method employing longitudinally monitored immune biomarkers to predict the clinical outcome of critically ill COVID-19 patients.
In PeerJ
BACKGROUND :
METHODS :
RESULTS :
CONCLUSIONS :
Martinez Gustavo, Garduno Alexis, Mahmud-Al-Rafat Abdullah, Ostadgavahi Ali Toloue, Avery Ann, de Avila E Silva Scheila, Cusack Rachael, Cameron Cheryl, Cameron Mark, Martin-Loeches Ignacio, Kelvin David
2022
Artificial Neural Networks, Biomarkers, Classification, Deep learning, Immunology
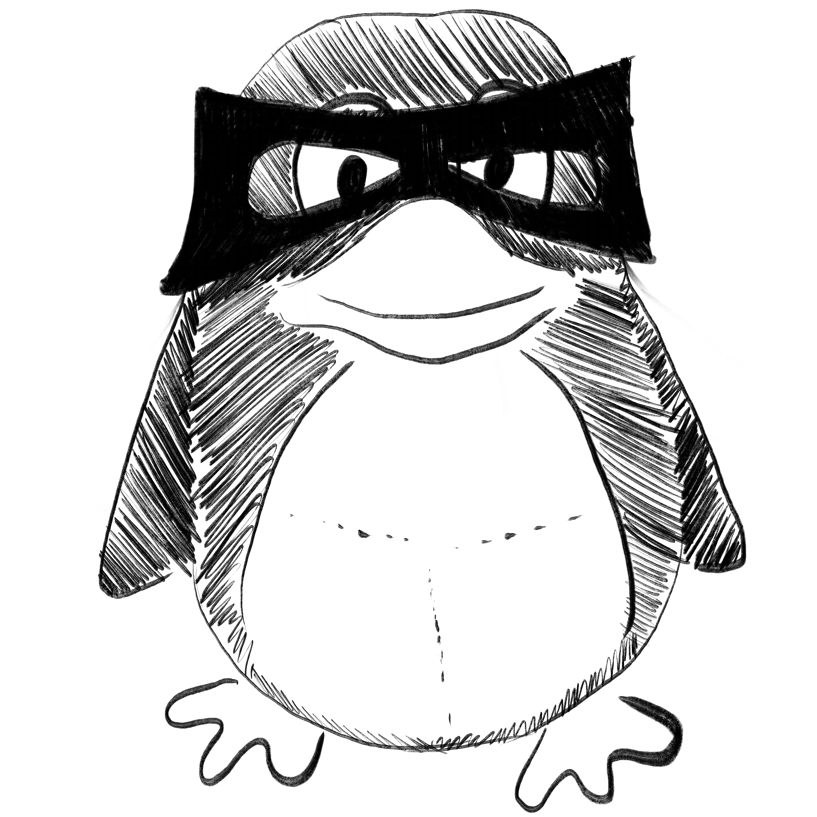
COVID-19 spread control policies based early dynamics forecasting using deep learning algorithm.
In Chaos, solitons, and fractals
Ali Furqan, Ullah Farman, Khan Junaid Iqbal, Khan Jebran, Sardar Abdul Wasay, Lee Sungchang
2022-Dec-13
COVID-19, Deep Learning, Forecasting, Long short-term memory, Pandemic, Stacked Bi-LSTM, Time series
Use of AI to assess COVID-19 variant impacts on hospitalization, ICU, and death.
In Frontiers in artificial intelligence
Hilal Waleed, Chislett Michael G, Snider Brett, McBean Edward A, Yawney John, Gadsden S Andrew
2022
COVID-19, Delta variant, Omicron variant, XGBoost, healthcare, hospitalization, medical risk factors
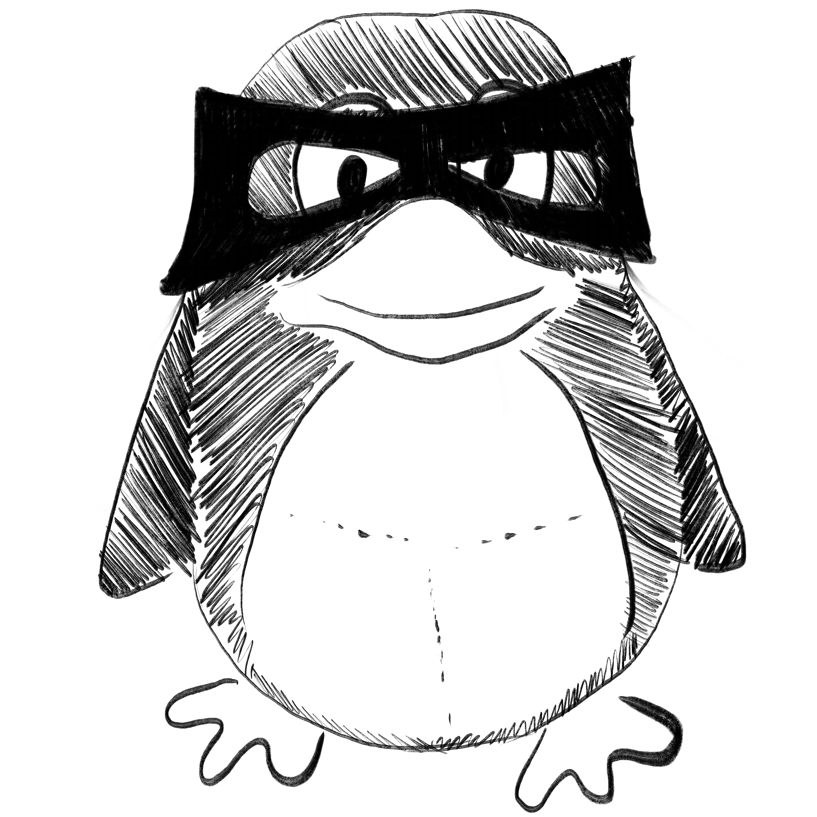
A survey of COVID-19 detection and prediction approaches using mobile devices, AI, and telemedicine.
In Frontiers in artificial intelligence
Shen John, Ghatti Siddharth, Levkov Nate Ryan, Shen Haiying, Sen Tanmoy, Rheuban Karen, Enfield Kyle, Facteau Nikki Reyer, Engel Gina, Dowdell Kim
2022
AI, COVID-19 detection, IoT, mobile devices, telemedicine
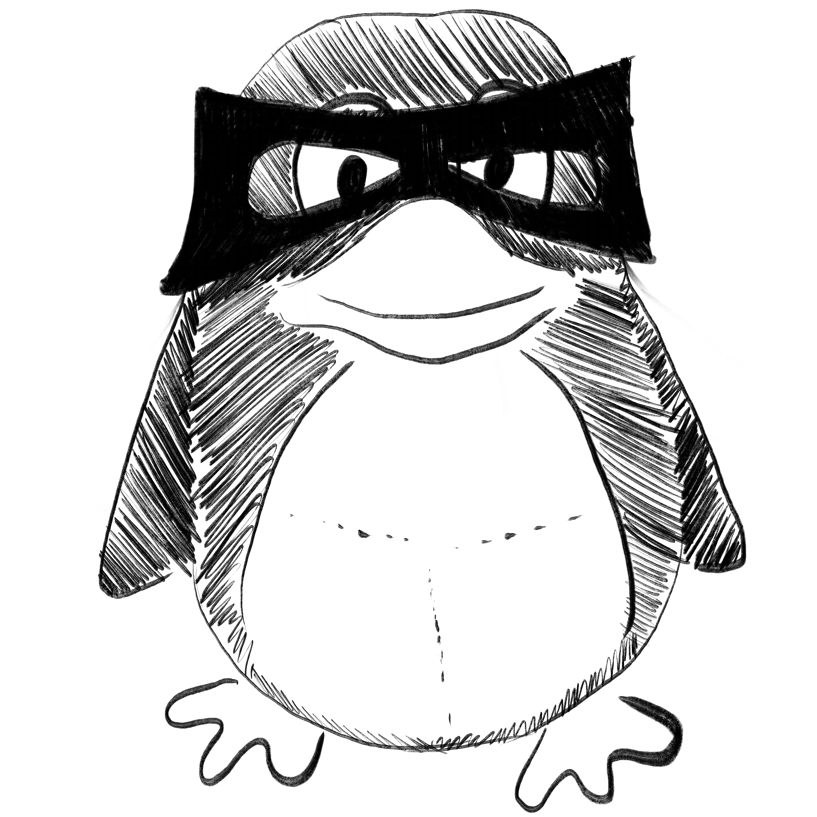
COVID-19 and human development: An approach for classification of HDI with deep CNN.
In Biomedical signal processing and control
Kavuran Gürkan, Gökhan Şeyma, Yeroğlu Celaleddin
2023-Mar
Artificial intelligence, COVID-19, Classification, Continuous wavelet transform, Deep learning, Human Development Index
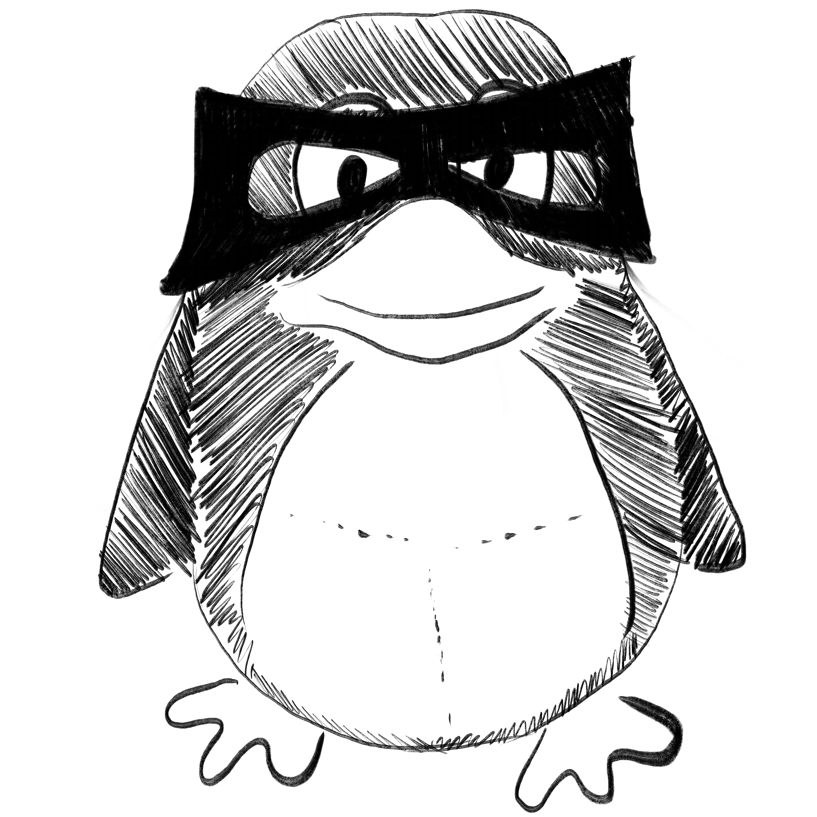
The design of compounds with desirable properties - The anti-HIV case study.
In Journal of computational chemistry
Novak Jurica, Pathak Prateek, Grishina Maria A, Potemkin Vladimir A
2022-Dec-19
3CLpro, HIV-1 protease, QSAR, cytotoxicity, drug repurposing
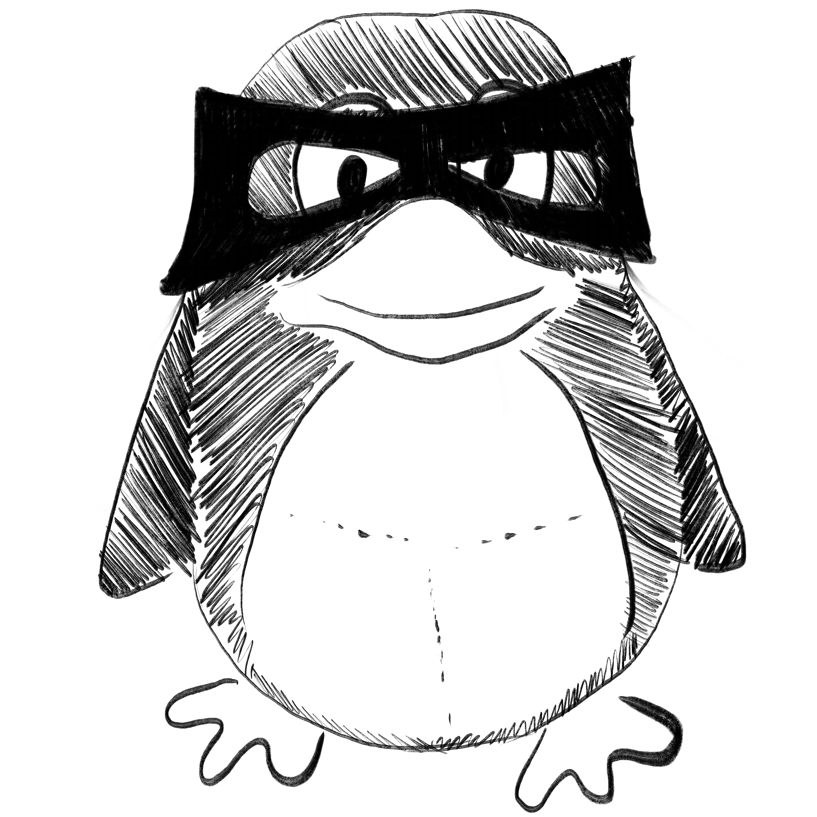
Does media sentiment affect stock prices? Evidence from China's STAR market.
In Frontiers in psychology ; h5-index 92.0
OBJECTIVE :
METHODS :
RESULTS :
DISCUSSION :
Dong Xiuliang, Xu Shiying, Liu Jianing, Tsai Fu-Sheng
2022
IPO first-day stock performance, IPO pricing efficiency, machine learning, media sentiment, stock price, the STAR market
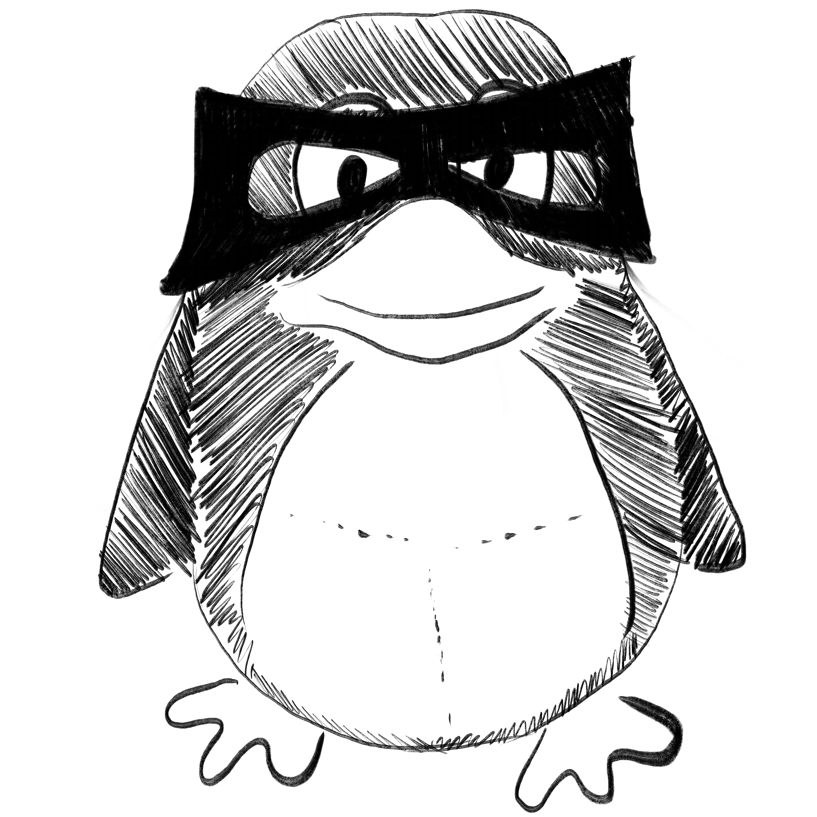
Research on the state of blended learning among college students - A mixed-method approach.
In Frontiers in psychology ; h5-index 92.0
Deng Chao, Peng Jiao, Li ShuFei
2022
TAM 3, blended learning, learner engagement theory, student engagement, technology acceptance
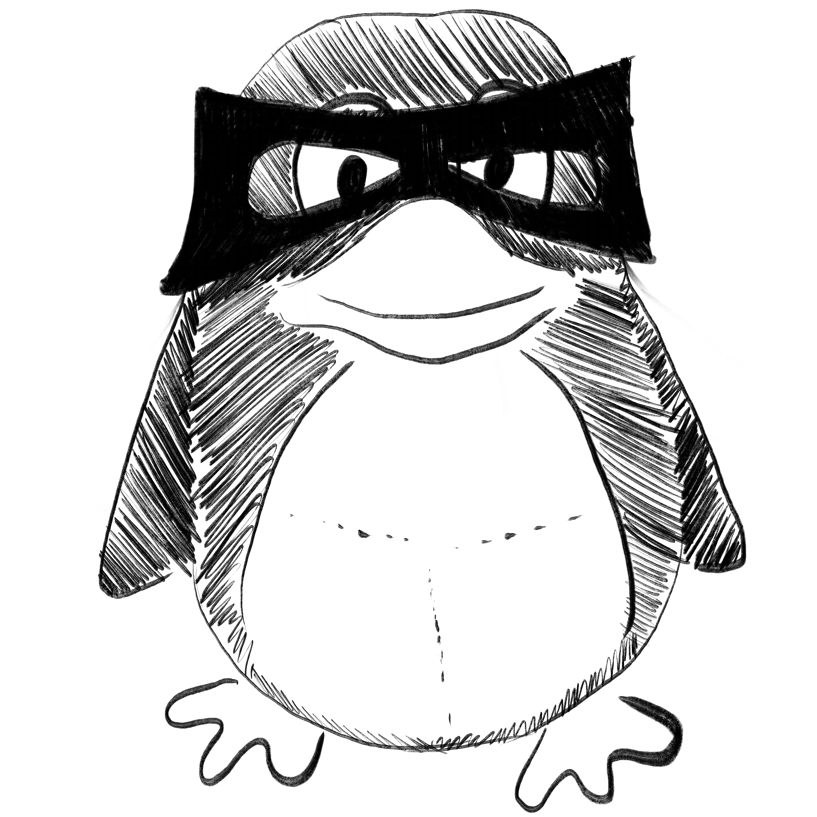
Intelligent lead-based bidirectional long short term memory for COVID-19 sentiment analysis.
In Social network analysis and mining
Kumari Santoshi, Pushphavathi T P
2023
COVID-19, Deep learning, LSTM, Optimization, Sentiment analysis
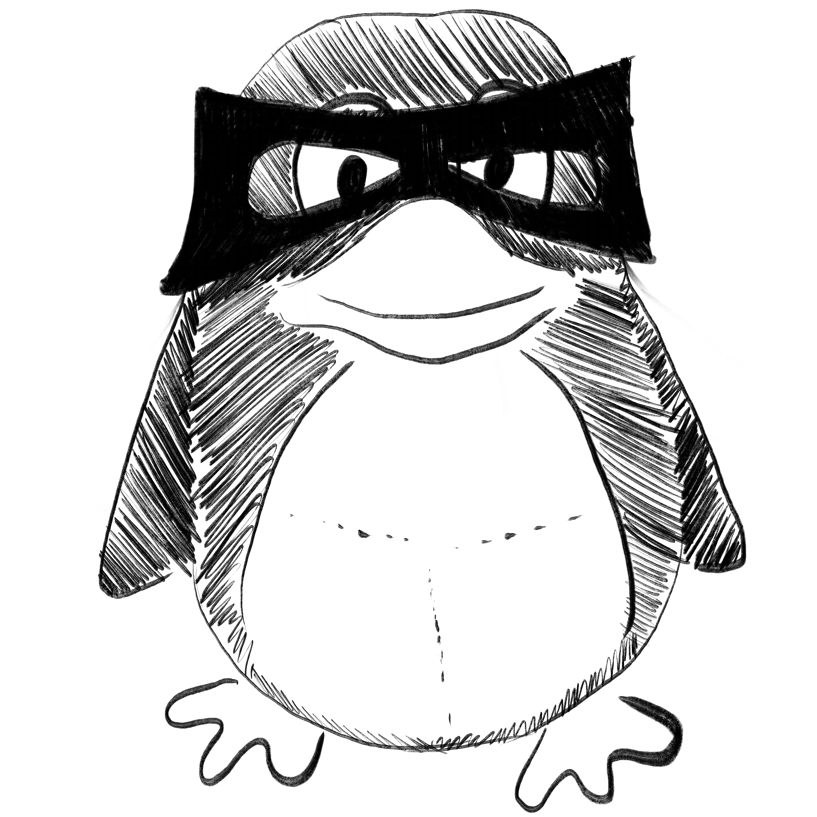
Deep features to detect pulmonary abnormalities in chest X-rays due to infectious diseaseX: Covid-19, pneumonia, and tuberculosis.
In Information sciences
Mahbub Md Kawsher, Biswas Milon, Gaur Loveleen, Alenezi Fayadh, Santosh K C
2022-May
ACC, Accuracy, AI, Artificial Intelligence, AUC, Area Under the Curve, CADx, Computer-Aided Diagnosis, CNN, Convolutional Neural Network, CT, Computed Tomography, CXR, Chest X-ray, Chest X-ray, Covid-19, DL, Deep Learning, DNN, DNN, Deep Neural Network, Infectious DiseaseX, ML, Machine Learning, MTB, Mycobacterium Tuberculosis, Medical imaging, NN, Neural Network, Pneumonia, SEN, Sensitivity, SPEC, Specificity, TB, Tuberculosis, Tuberculosis, WHO, World Health Organization
An efficient approach to identifying anti-government sentiment on Twitter during Michigan protests.
In PeerJ. Computer science
Nguyen Hieu, Gokhale Swapna
2022
Anti-government, COVID-19, Lockdown protests, Machine Learning, Social media
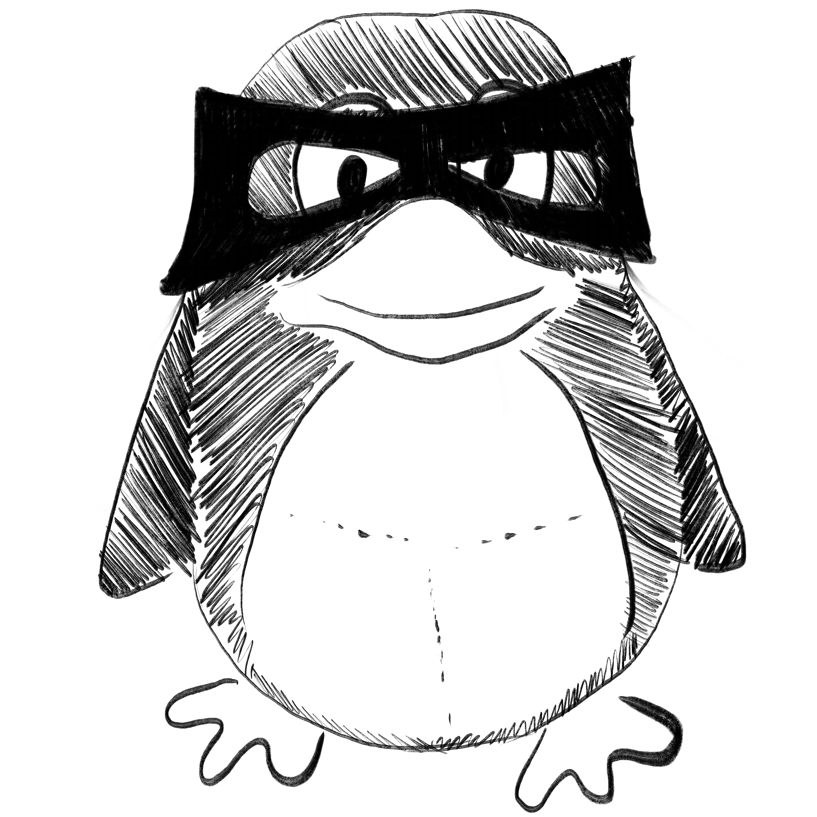
Multi-label multi-class COVID-19 Arabic Twitter dataset with fine-grained misinformation and situational information annotations.
In PeerJ. Computer science
Obeidat Rasha, Gharaibeh Maram, Abdullah Malak, Alharahsheh Yara
2022
BERT, COVID-19, Data annotation, Data collection, Deep learning, Fake news, Machine learning, Misinformation detection, Situational information, Transformers
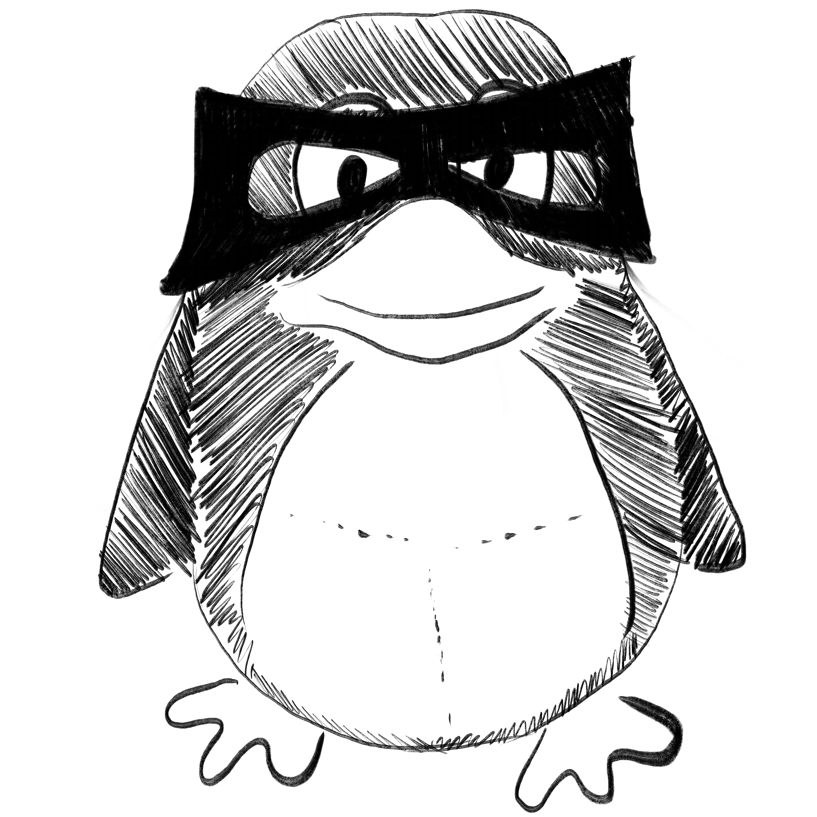
Machine Learning and Deep Learning Based Time Series Prediction and Forecasting of Ten Nations' COVID-19 Pandemic.
In SN computer science
Kumar Yogesh, Koul Apeksha, Kaur Sukhpreet, Hu Yu-Chen
2023
COVID-19, Facebook Prophet, Holt model, Prediction, RANSAC regressor, Random forest regressor, Stacked gated recurrent units, Stacked long short-term memory, XG Boost
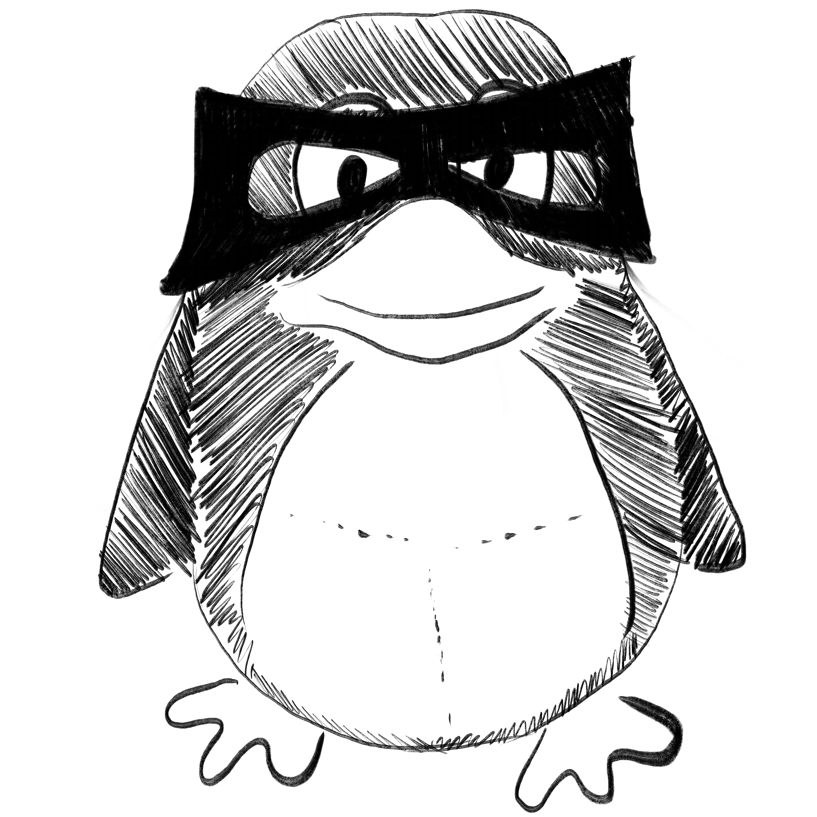
A Precise Method to Detect Post-COVID-19 Pulmonary Fibrosis Through Extreme Gradient Boosting.
In SN computer science
Jha Manika, Gupta Richa, Saxena Rajiv
2023
COVID-19, Clinical decision support, Extreme gradient boosting, Machine learning, Medical diagnosis, Pulmonary fibrosis, Tree boosting
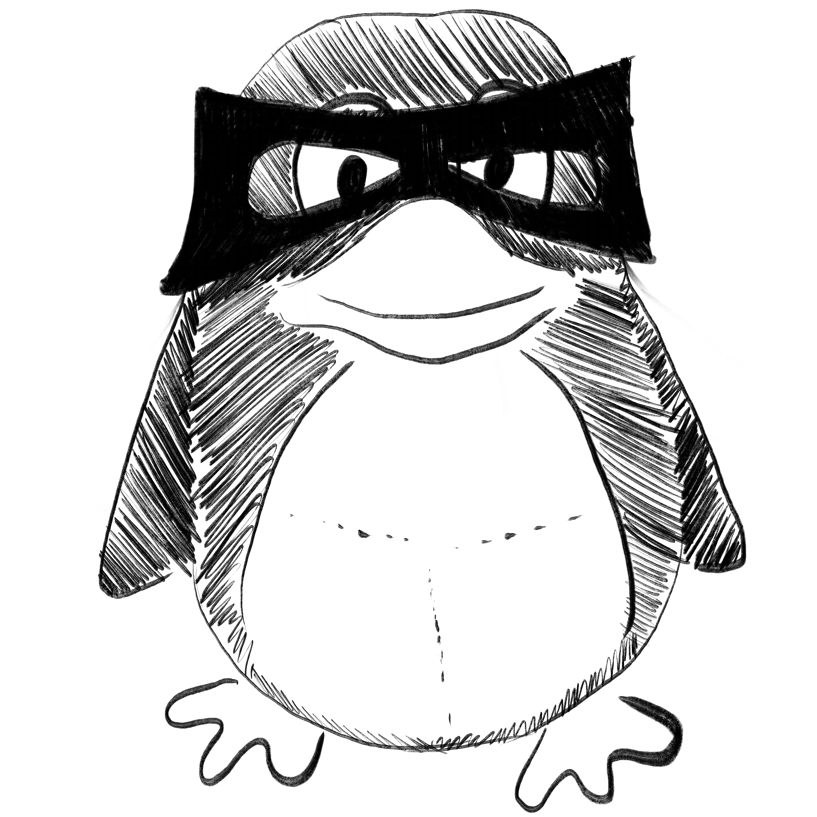
LWSNet - a novel deep-learning architecture to segregate Covid-19 and pneumonia from x-ray imagery.
In Multimedia tools and applications
Lasker Asifuzzaman, Ghosh Mridul, Obaidullah Sk Md, Chakraborty Chandan, Roy Kaushik
2022-Dec-03
Chest radiography, Covid-19, Deep neural network, Lung diseases, Pneumonia, Stack ensemble technique
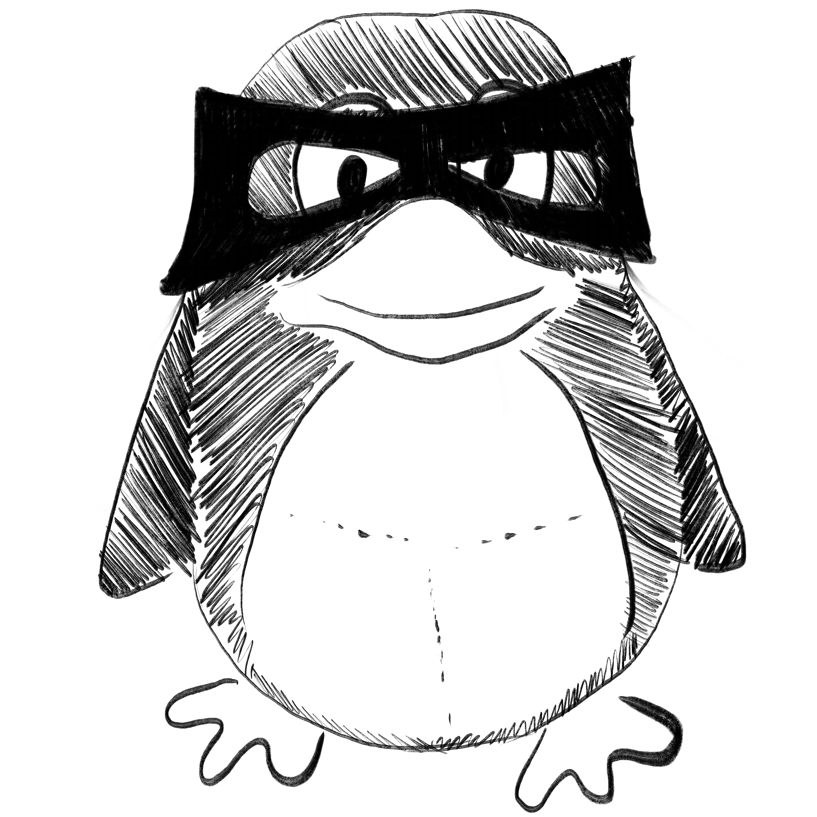
Contactless blood oxygen estimation from face videos: A multi-model fusion method based on deep learning.
In Biomedical signal processing and control
Hu Min, Wu Xia, Wang Xiaohua, Xing Yan, An Ning, Shi Piao
2023-Mar
Coordinate attention, Deep learning, Estimation, Multi-model fusion, Remote photo-plethysmography, Residual network
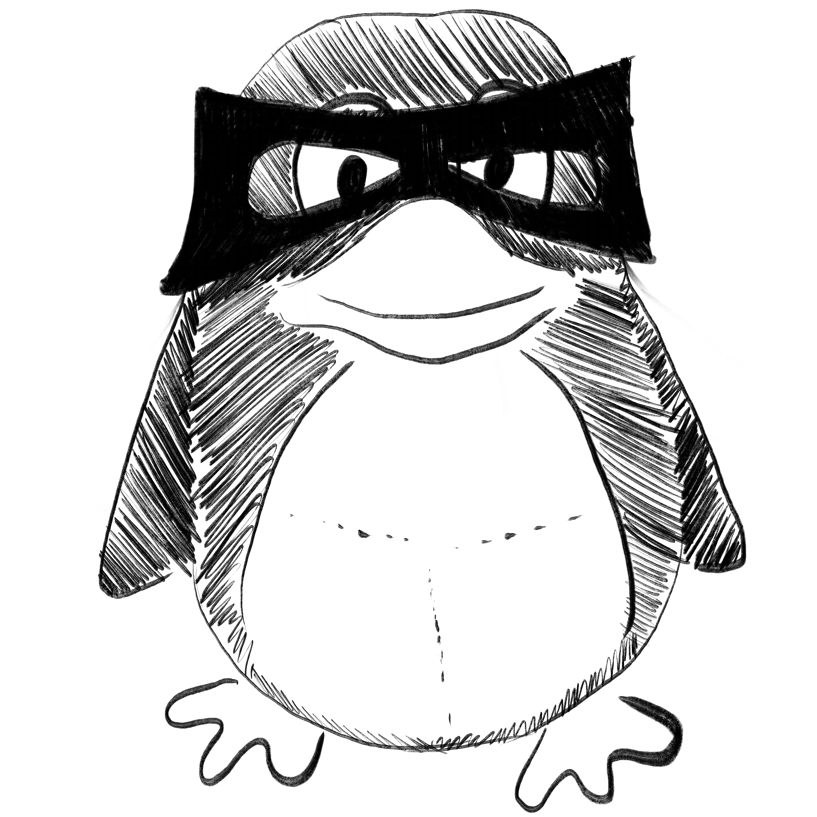
MIC-Net: A deep network for cross-site segmentation of COVID-19 infection in the fog-assisted IoMT.
In Information sciences
Ding Weiping, Abdel-Basset Mohamed, Hawash Hossam, Pedrycz Witold
2023-Apr
COVID-19, Data Heterogeneity, Deep Learning, Fog Computing, Internet of Medical Things
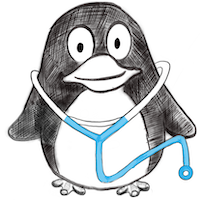
Multi-objective automatic analysis of lung ultrasound data from COVID-19 patients by means of deep learning and decision trees.
In Applied soft computing
Custode Leonardo Lucio, Mento Federico, Tursi Francesco, Smargiassi Andrea, Inchingolo Riccardo, Perrone Tiziano, Demi Libertario, Iacca Giovanni
2023-Jan
COVID-19, Decision trees, Evolutionary algorithms, Grammatical evolution, Lung ultrasound, Neuro-symbolic artificial intelligence
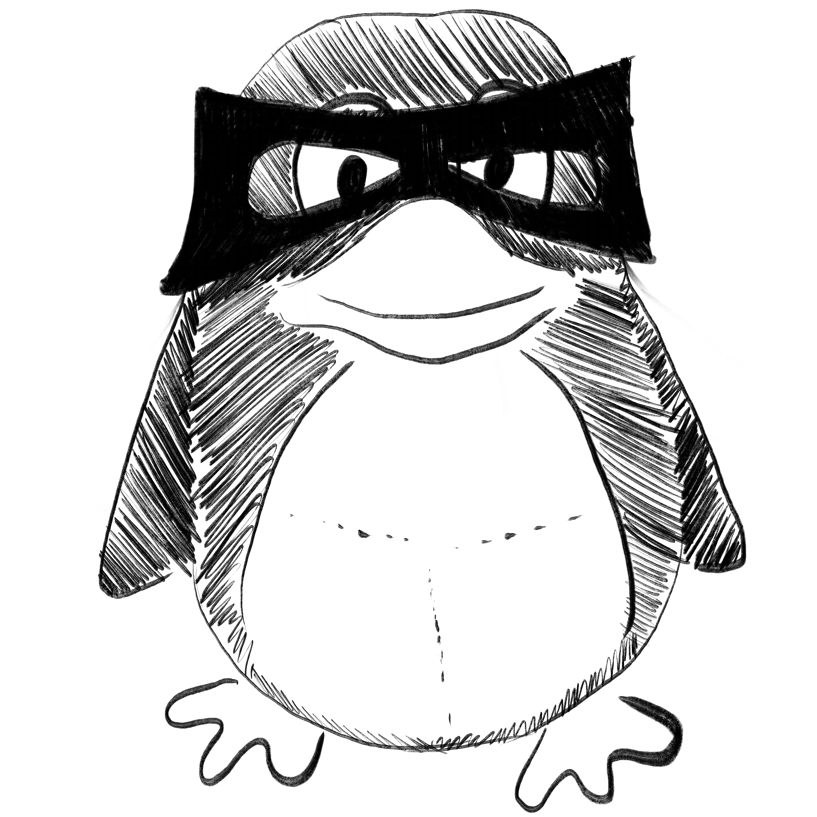
Single-cell multiomics revealed the dynamics of antigen presentation, immune response and T cell activation in the COVID-19 positive and recovered individuals.
In Frontiers in immunology ; h5-index 100.0
INTRODUCTION :
METHODS :
RESULTS :
DISCUSSION :
Chattopadhyay Partha, Khare Kriti, Kumar Manish, Mishra Pallavi, Anand Alok, Maurya Ranjeet, Gupta Rohit, Sahni Shweta, Gupta Ayushi, Wadhwa Saruchi, Yadav Aanchal, Devi Priti, Tardalkar Kishore, Joshi Meghnad, Sethi Tavpritesh, Pandey Rajesh
2022
COVID-19, T-cell activation, bayesian network model, immune response, recovered COVID-19 individuals, single cell multi-omics
SARS-CoV-2 induces "cytokine storm" hyperinflammatory responses in RA patients through pyroptosis.
In Frontiers in immunology ; h5-index 100.0
BACKGROUND :
METHODS :
RESULTS :
CONCLUSIONS :
Zheng Qingcong, Lin Rongjie, Chen Yuchao, Lv Qi, Zhang Jin, Zhai Jingbo, Xu Weihong, Wang Wanming
2022
COVID-19, SARS-CoV-2, caspase-1, minocycline, pyroptosis, rheumatoid arthritis
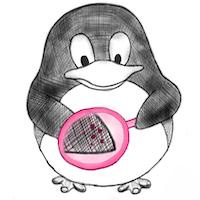
Integrated bioinformatics to identify potential key biomarkers for COVID-19-related chronic urticaria.
In Frontiers in immunology ; h5-index 100.0
BACKGROUND :
METHODS :
RESULTS :
CONCLUSIONS :
Zhang Teng, Feng Hao, Zou Xiaoyan, Peng Shixiong
2022
COVID-19, bioinformatics, biomarker, chronic Urticaria (CU), immunology
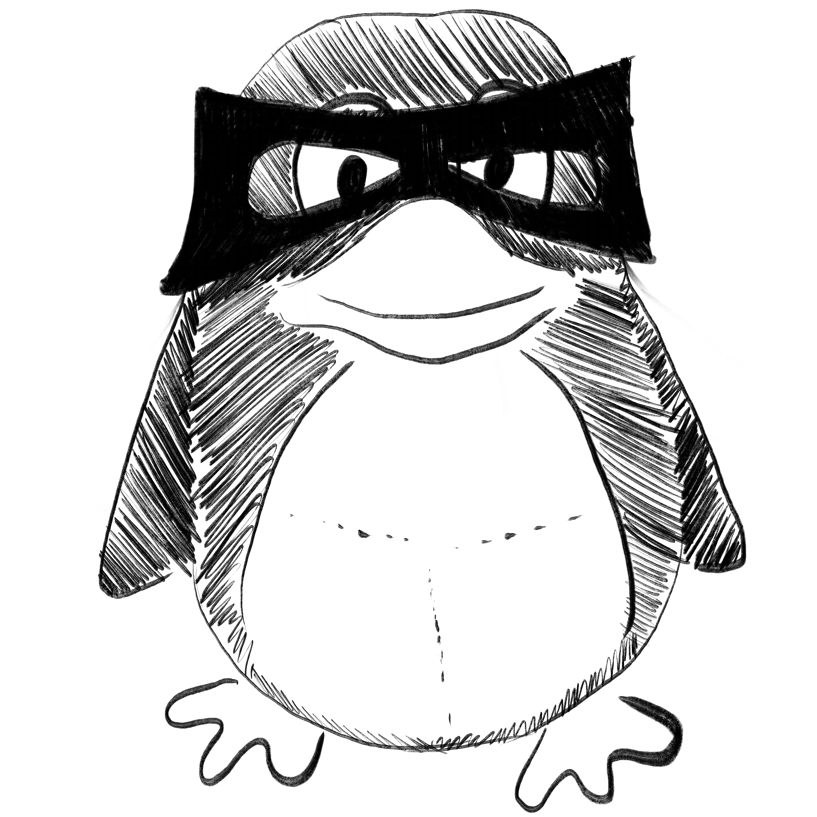
COVID vaccine stigma: detecting stigma across social media platforms with computational model based on deep learning.
In Applied intelligence (Dordrecht, Netherlands)
Straton Nadiya
2022-Dec-07
COVID-19, Deep learning, Social media, Stigma, Vaccine
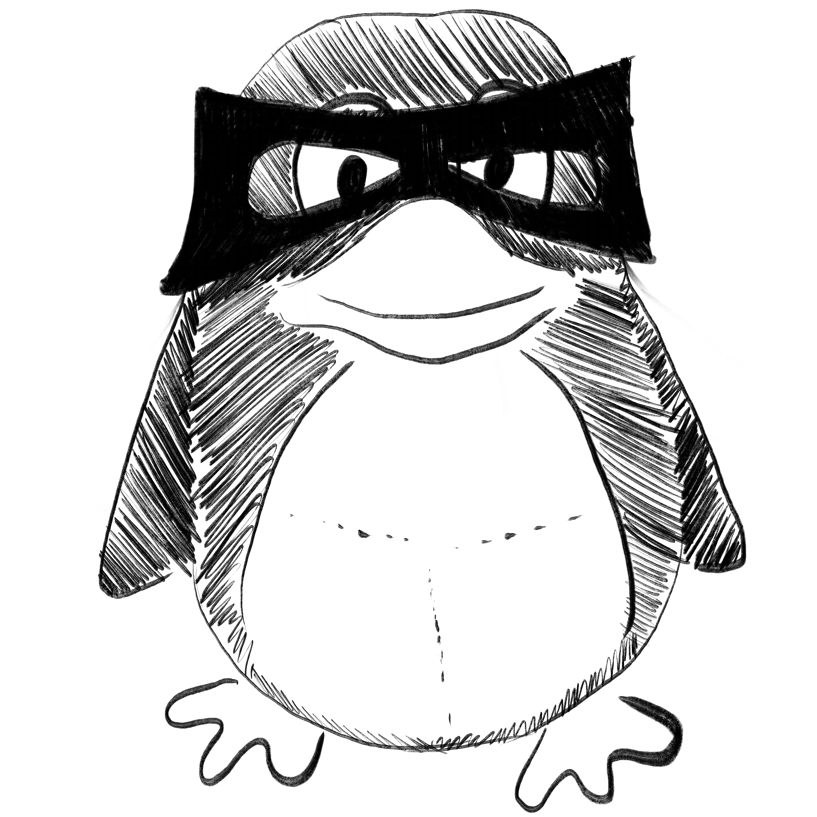
3D face recognition algorithm based on deep Laplacian pyramid under the normalization of epidemic control.
In Computer communications
Kong Weiyi, You Zhisheng, Lv Xuebin
2022-Dec-13
3D face recognition, Deep learning, Epidemic control, Face reconstruction, Multimodal fusion
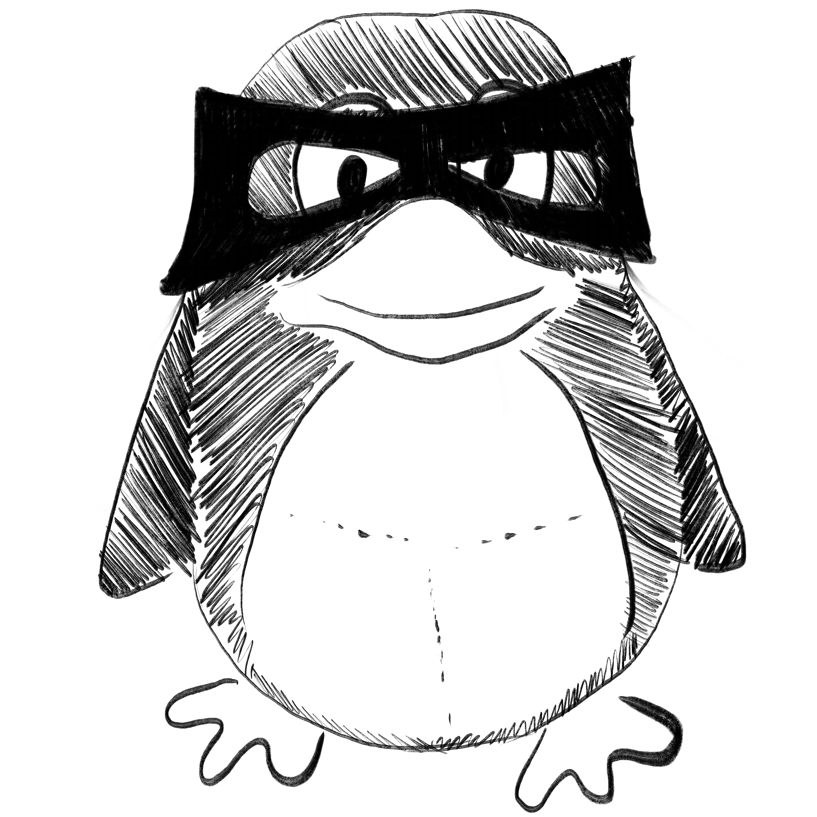
COVID-19 health data analysis and personal data preserving: A homomorphic privacy enforcement approach.
In Computer communications
Dhasarathan Chandramohan, Hasan Mohammad Kamrul, Islam Shayla, Abdullah Salwani, Mokhtar Umi Asma, Javed Abdul Rehman, Goundar Sam
2022-Dec-14
Deep learning system, Healthcare, Homomorphic, Privacy metrics, Privacy preserving, Security
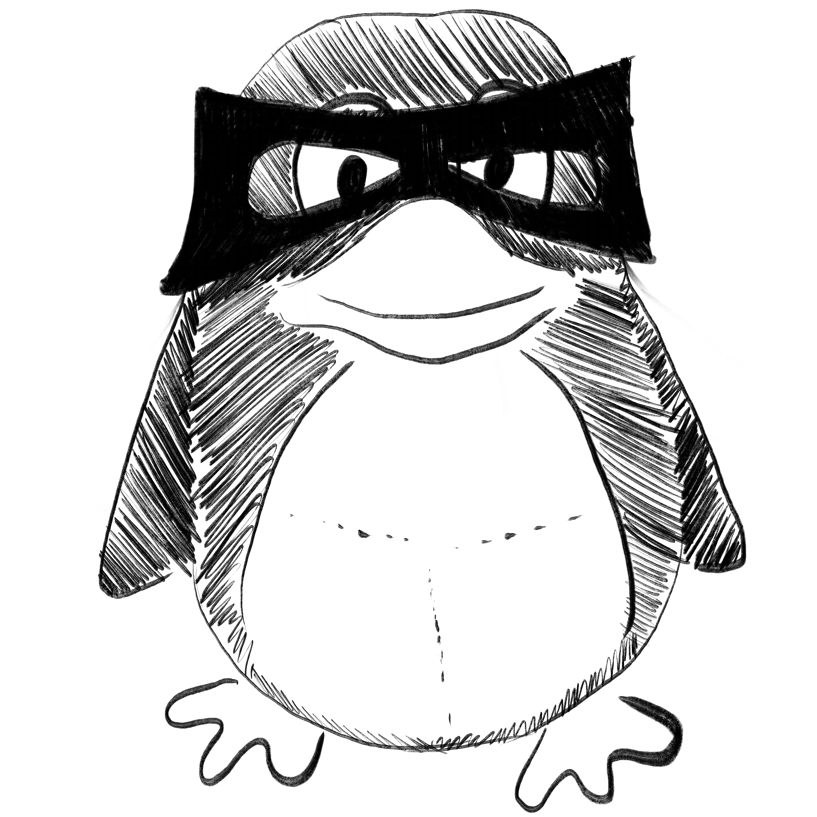
SARS-CoV-2 virus classification based on stacked sparse autoencoder.
In Computational and structural biotechnology journal
Coutinho Maria G F, Câmara Gabriel B M, Barbosa Raquel de M, Fernandes Marcelo A C
2023
COVID-19, Deep learning, SARS-CoV-2, Sparse autoencoder, Viral classification
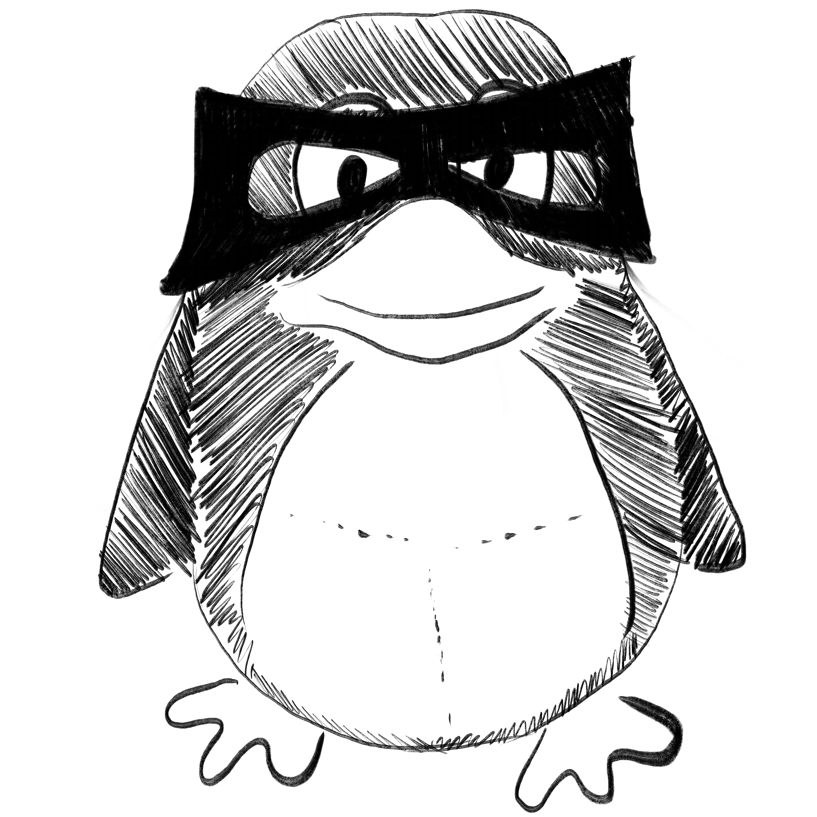
Combating Covid-19 using machine learning and deep learning: Applications, challenges, and future perspectives.
In Array (New York, N.Y.)
Paul Showmick Guha, Saha Arpa, Biswas Al Amin, Zulfiker Md Sabab, Arefin Mohammad Shamsul, Rahman Md Mahfujur, Reza Ahmed Wasif
2022-Dec-10
Artificial intelligence, COVID-19, Deep learning, Machine learning, Pandemic
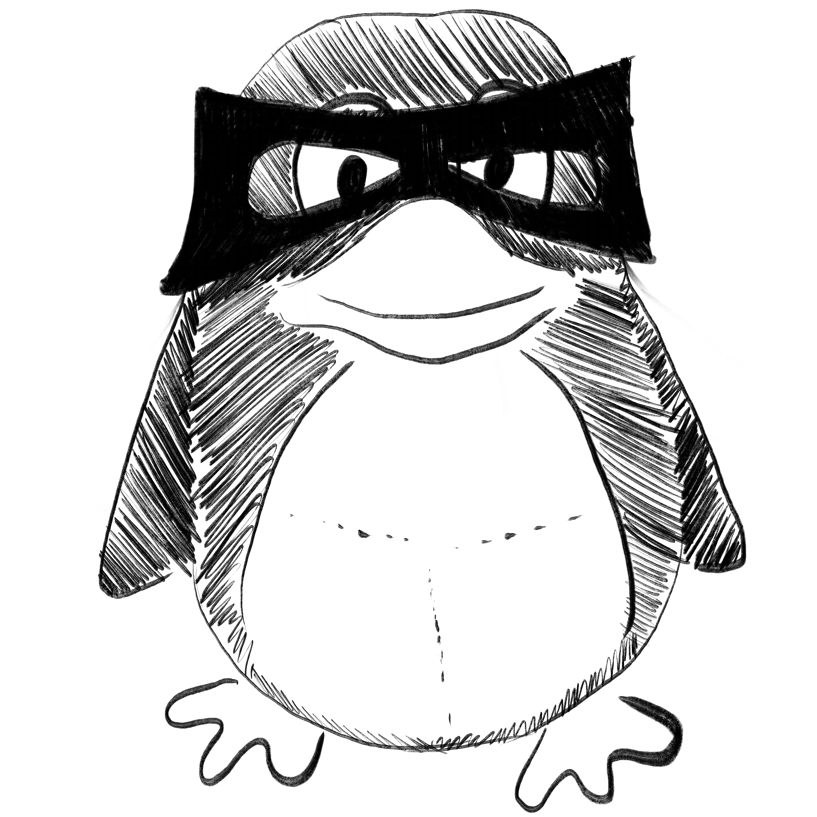
FreeEnricher: Enriching Face Landmarks without Additional Cost
ArXiv Preprint
Yangyu Huang, Xi Chen, Jongyoo Kim, Hao Yang, Chong Li, Jiaolong Yang, Dong Chen
2022-12-19
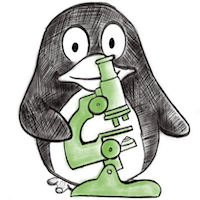
Optimal Transport for Unsupervised Hallucination Detection in Neural Machine Translation
ArXiv Preprint
Nuno M. Guerreiro, Pierre Colombo, Pablo Piantanida, André F. T. Martins
2022-12-19
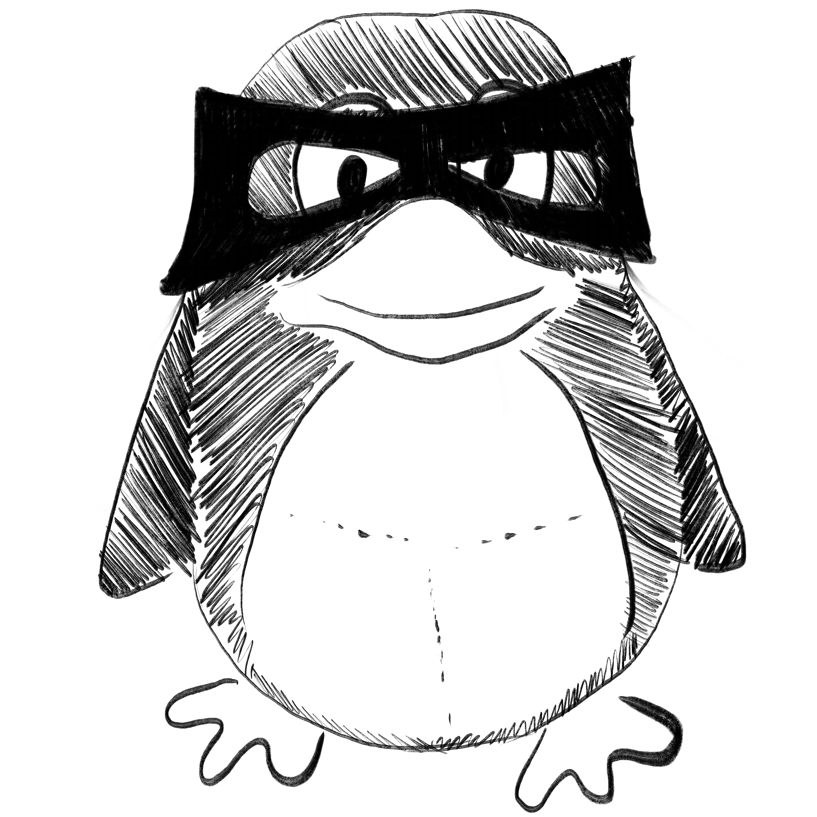
Predicting Ejection Fraction from Chest X-rays Using Computer Vision for Diagnosing Heart Failure
ArXiv Preprint
Walt Williams, Rohan Doshi, Yanran Li, Kexuan Liang
2022-12-19
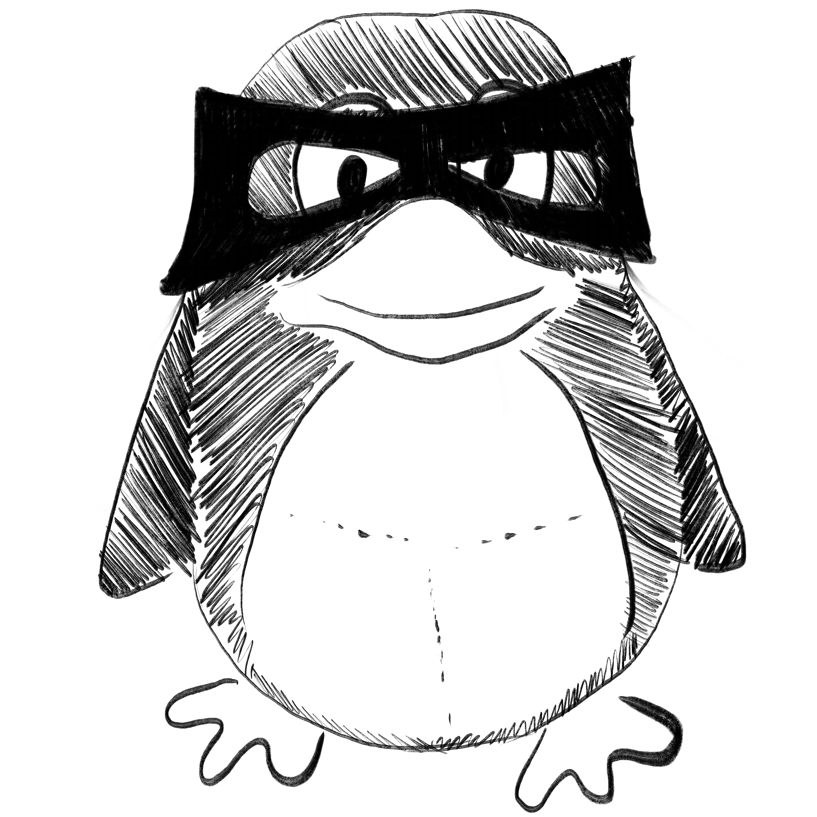
Exploring Hybrid and Ensemble Models for Multiclass Prediction of Mental Health Status on Social Media
ArXiv Preprint
Sourabh Zanwar, Daniel Wiechmann, Yu Qiao, Elma Kerz
2022-12-19
Boosting Automatic COVID-19 Detection Performance with Self-Supervised Learning and Batch Knowledge Ensembling
ArXiv Preprint
Guang Li, Ren Togo, Takahiro Ogawa, Miki Haseyama
2022-12-19
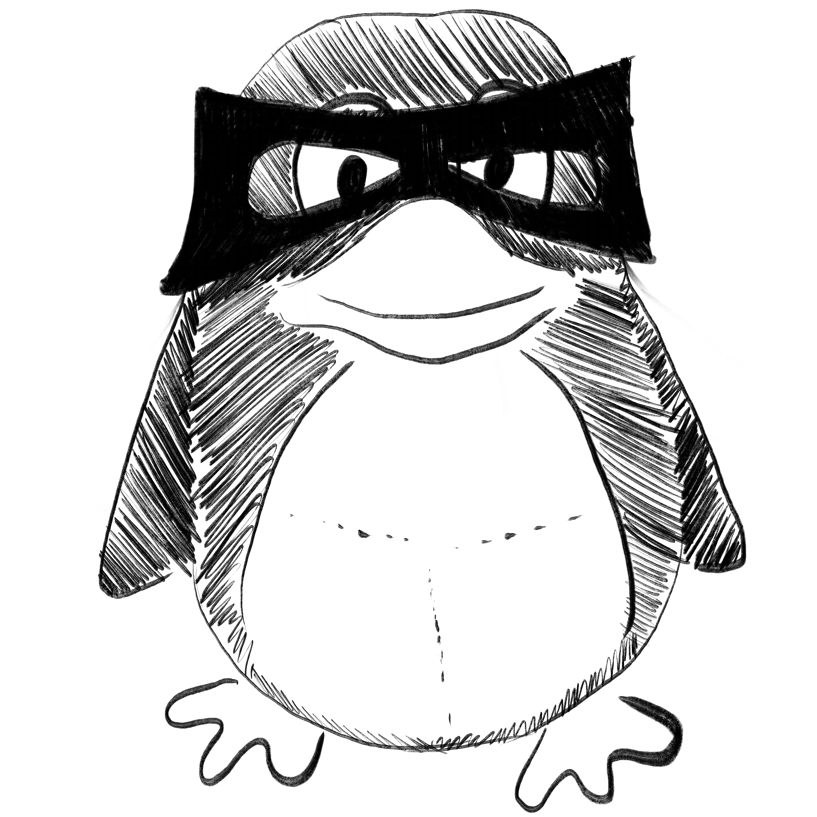
PAL: Persona-Augmented Emotional Support Conversation Generation
ArXiv Preprint
Jiale Cheng, Sahand Sabour, Hao Sun, Zhuang Chen, Minlie Huang
2022-12-19
COVID-19 Detection Based on Self-Supervised Transfer Learning Using Chest X-Ray Images
ArXiv Preprint
Guang Li, Ren Togo, Takahiro Ogawa, Miki Haseyama
2022-12-19
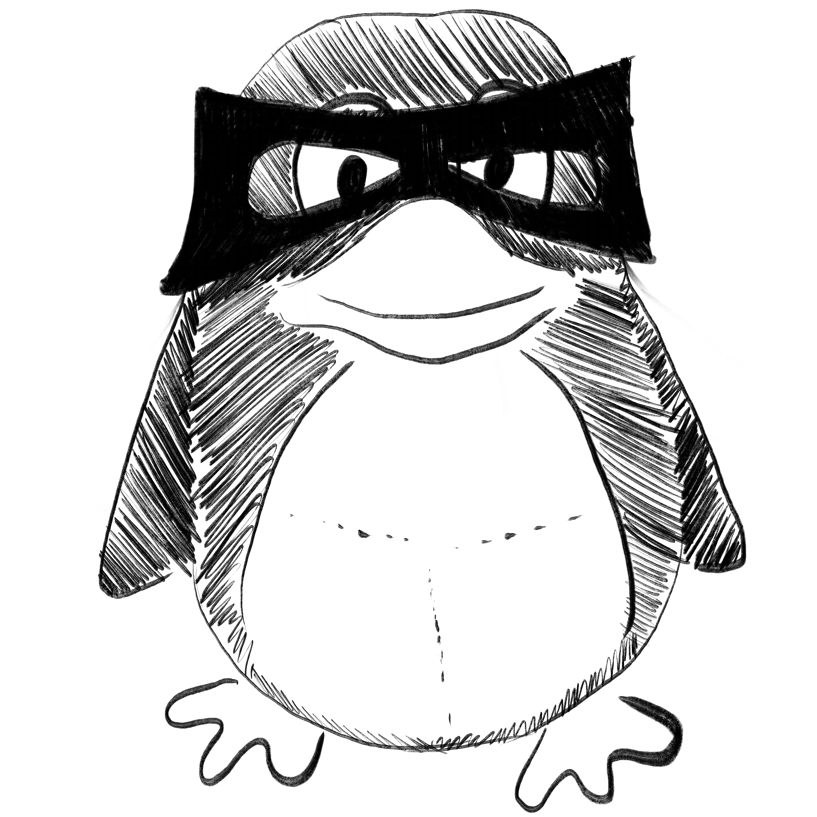
Feature fusion based VGGFusionNet model to detect COVID-19 patients utilizing computed tomography scan images.
In Scientific reports ; h5-index 158.0
Uddin Khandaker Mohammad Mohi, Dey Samrat Kumar, Babu Hafiz Md Hasan, Mostafiz Rafid, Uddin Shahadat, Shoombuatong Watshara, Moni Mohammad Ali
2022-Dec-16
Machine-learning approaches to identify determining factors of happiness during the COVID-19 pandemic: retrospective cohort study.
In BMJ open
OBJECTIVE :
DESIGN :
SETTING :
PARTICIPANTS :
MAIN OUTCOME MEASURE :
RESULTS :
CONCLUSION :
Osawa Itsuki, Goto Tadahiro, Tabuchi Takahiro, Koga Hayami K, Tsugawa Yusuke
2022-Dec-16
COVID-19, MENTAL HEALTH, PUBLIC HEALTH
AI support for accurate and fast radiological diagnosis of COVID-19: an international multicenter, multivendor CT study.
In European radiology ; h5-index 62.0
OBJECTIVES :
METHODS :
RESULTS :
CONCLUSION :
KEY POINTS :
Meng Fanyang, Kottlors Jonathan, Shahzad Rahil, Liu Haifeng, Fervers Philipp, Jin Yinhua, Rinneburger Miriam, Le Dou, Weisthoff Mathilda, Liu Wenyun, Ni Mengzhe, Sun Ye, An Liying, Huai Xiaochen, Móré Dorottya, Giannakis Athanasios, Kaltenborn Isabel, Bucher Andreas, Maintz David, Zhang Lei, Thiele Frank, Li Mingyang, Perkuhn Michael, Zhang Huimao, Persigehl Thorsten
2022-Dec-16
Artificial intelligence, COVID-19, Computed tomography, Radiologists
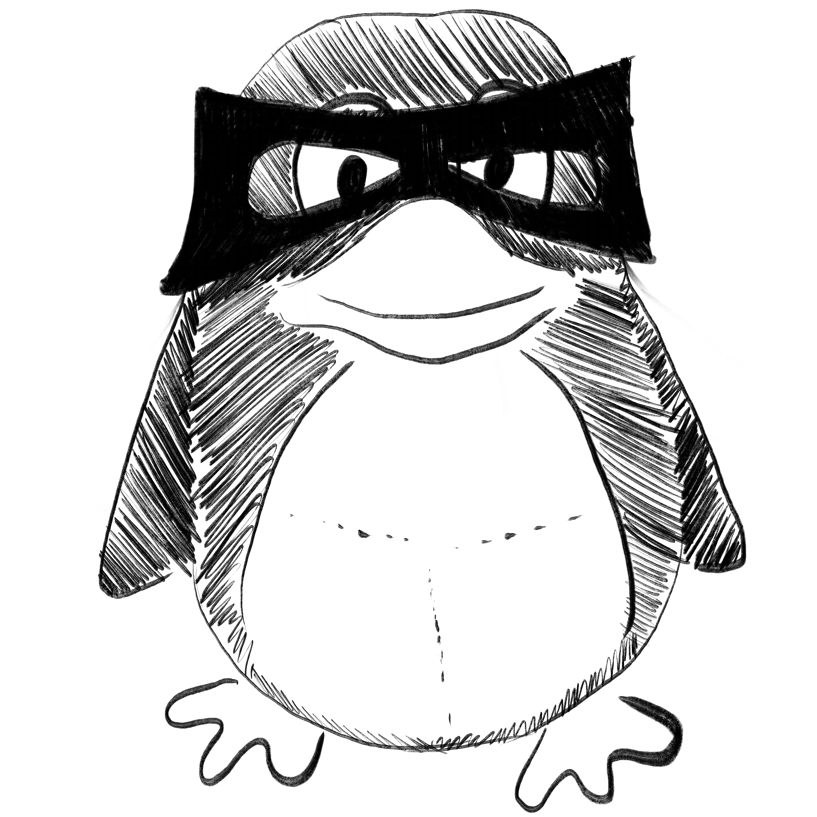
Cardiopulmonary examinations of athletes returning to high-intensity sport activity following SARS-CoV-2 infection.
In Scientific reports ; h5-index 158.0
Babity Mate, Zamodics Mark, Konig Albert, Kiss Anna Reka, Horvath Marton, Gregor Zsofia, Rakoczi Reka, Kovacs Eva, Fabian Alexandra, Tokodi Marton, Sydo Nora, Csulak Emese, Juhasz Vencel, Lakatos Balint Karoly, Vago Hajnalka, Kovacs Attila, Merkely Bela, Kiss Orsolya
2022-Dec-15
Development of criteria for cognitive dysfunction in post-COVID syndrome: the IC-CoDi-COVID approach.
In Psychiatry research ; h5-index 64.0
BACKGROUND :
METHODS :
RESULTS :
CONCLUSIONS :
Matias-Guiu Jordi A, Herrera Elena, González-Nosti María, Krishnan Kamini, Delgado-Alonso Cristina, Díez-Cirarda María, Yus Miguel, Martínez-Petit Álvaro, Pagán Josué, Matías-Guiu Jorge, Ayala José Luis, Busch Robyn, Hermann Bruce P
2022-Dec-10
COVID-19, Cognitive, IC-CoDi-COVID, Machine learning, Post-COVID syndrome., neuropsychological
Machine learning models to predict the maximum severity of COVID-19 based on initial hospitalization record.
In Frontiers in public health
BACKGROUND :
OBJECTIVE :
METHODS :
RESULTS :
CONCLUSIONS :
Hwangbo Suhyun, Kim Yoonjung, Lee Chanhee, Lee Seungyeoun, Oh Bumjo, Moon Min Kyong, Kim Shin-Woo, Park Taesung
2022
COVID-19, artificial intelligence, machine learning, nomogram, severity
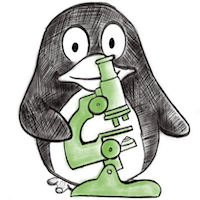
PredictION: a predictive model to establish the performance of Oxford sequencing reads of SARS-CoV-2.
In PeerJ
Valencia-Valencia David E, Lopez-Alvarez Diana, Rivera-Franco Nelson, Castillo Andres, Piña Johan S, Pardo Carlos A, Parra Beatriz
2022
Genomes, Linear models, Machine learning, Oxford nanopore technologies, Sequences
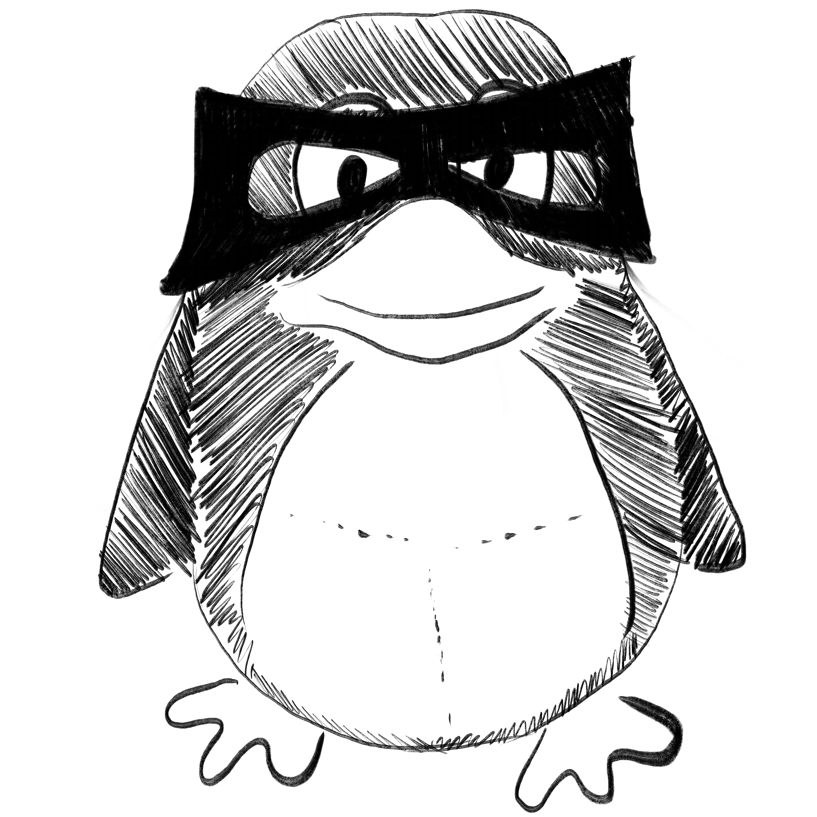
A signal recognition particle-related joint model of LASSO regression, SVM-RFE and artificial neural network for the diagnosis of systemic sclerosis-associated pulmonary hypertension.
In Frontiers in genetics ; h5-index 62.0
Xu Jingxi, Liang Chaoyang, Li Jiangtao
2022
artificial neural network, diagnostic model, machine learning, signal recognition particle, systemic sclerosis-associated pulmonary hypertension
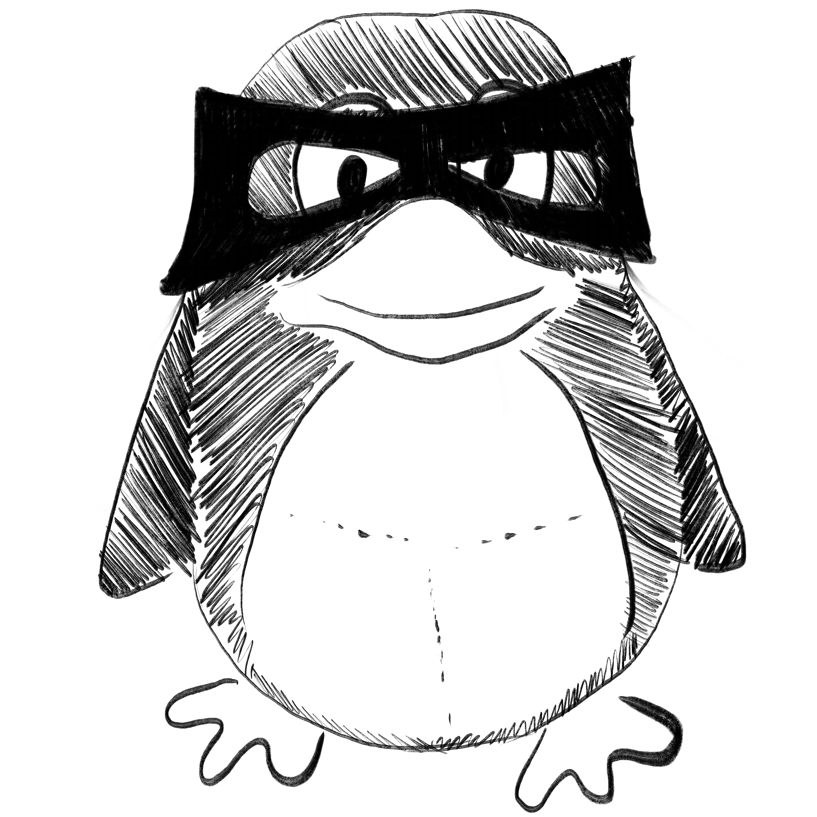
Digital Omicron Detection using Unscripted Voice Samples from Social Media.
In medRxiv : the preprint server for health sciences
Anibal James T, Landa Adam J, Nguyen Hang T, Peltekian Alec K, Shin Andrew D, Song Miranda J, Christou Anna S, Hazen Lindsey A, Rivera Jocelyne, Morhard Robert A, Bagci Ulas, Li Ming, Clifton David A, Wood Bradford J
2022-Oct-06
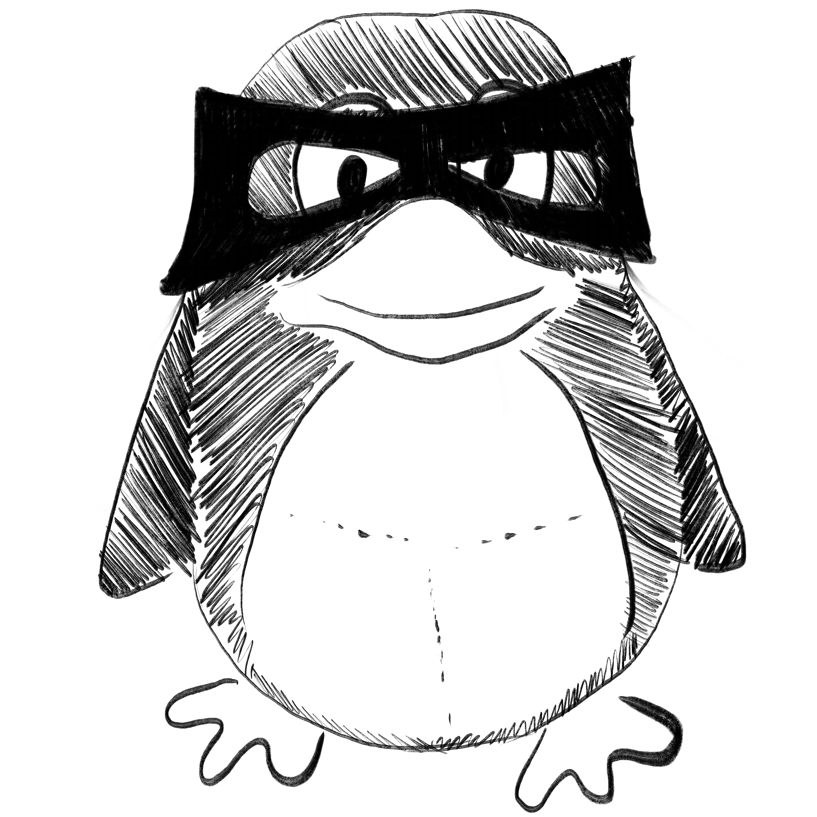
Investigating pregnant women's health information needs during pregnancy on internet platforms.
In Frontiers in physiology
Hou Keke, Hou Tingting
2022
health information, pregnancy, sentiment analysis, text analysis, topic analysis
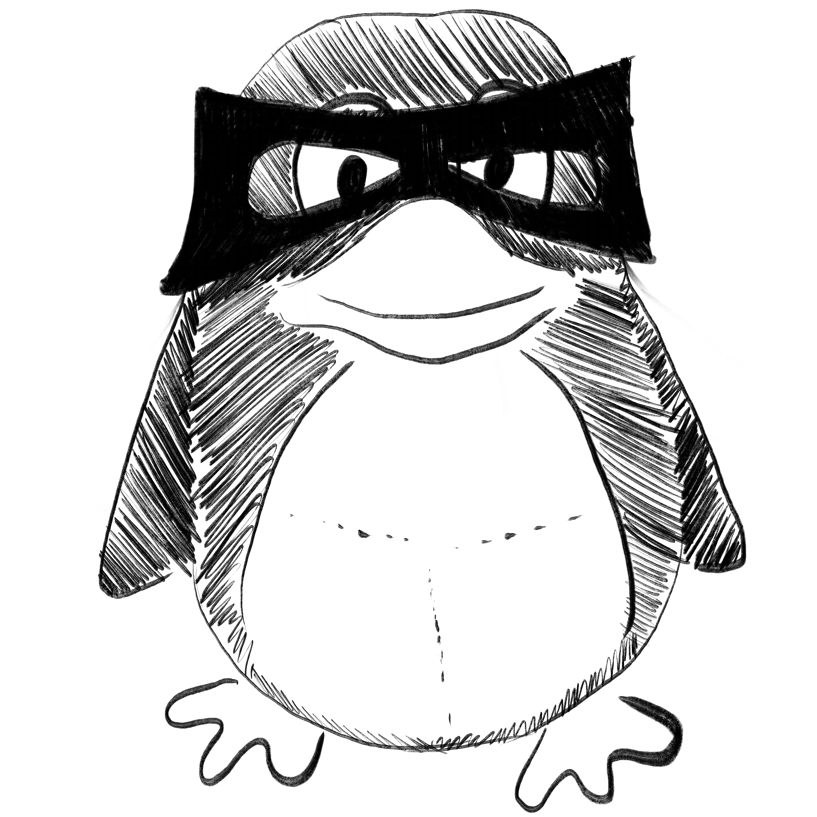
Molecular modeling of C1-inhibitor as SARS-CoV-2 target identified from the immune signatures of multiple tissues: An integrated bioinformatics study.
In Cell biochemistry and function
Sekaran Karthik, Polachirakkal Varghese Rinku, Gnanasambandan R, Karthik G, Ramya I, George Priya Doss C
2022-Dec-14
C1-inhibitor, COVID-19, Shapley additive explanations, druggability assessment, explainable artificial intelligence, gene expression, immune-related genes, machine learning
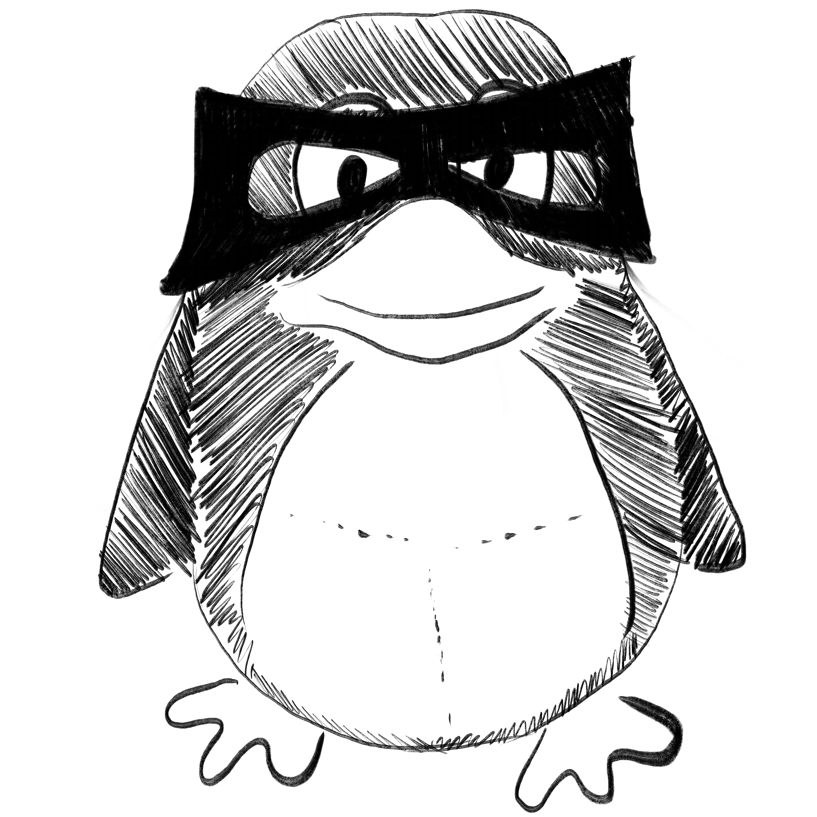
Audio-based AI classifiers show no evidence of improved COVID-19 screening over simple symptoms checkers
ArXiv Preprint
Harry Coppock, George Nicholson, Ivan Kiskin, Vasiliki Koutra, Kieran Baker, Jobie Budd, Richard Payne, Emma Karoune, David Hurley, Alexander Titcomb, Sabrina Egglestone, Ana Tendero Cañadas, Lorraine Butler, Radka Jersakova, Jonathon Mellor, Selina Patel, Tracey Thornley, Peter Diggle, Sylvia Richardson, Josef Packham, Björn W. Schuller, Davide Pigoli, Steven Gilmour, Stephen Roberts, Chris Holmes
2022-12-15
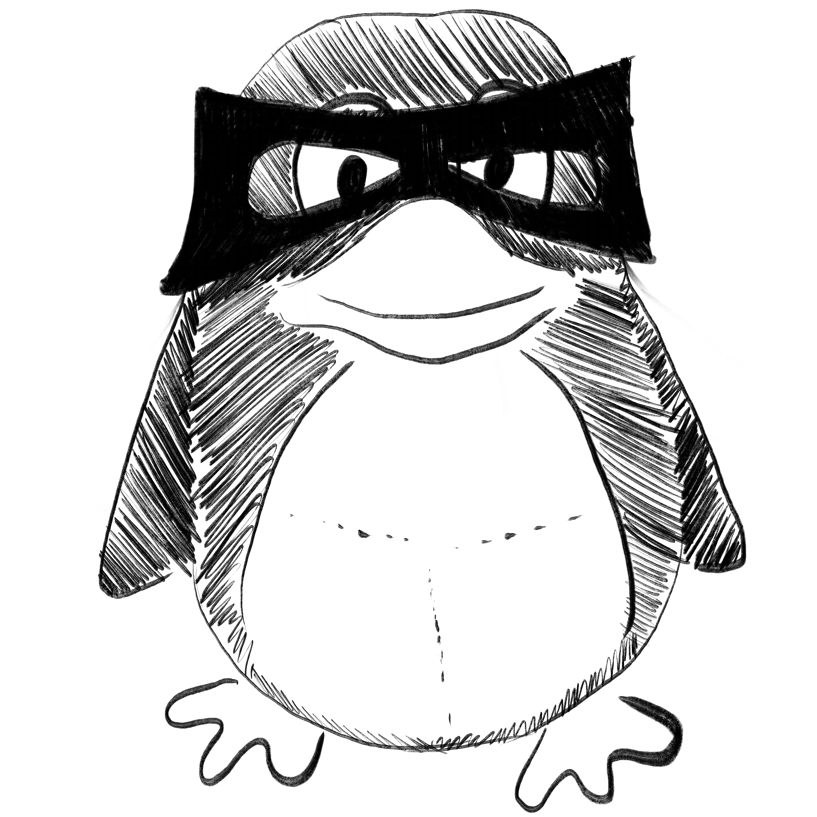
The effects of gender bias in word embeddings on depression prediction
ArXiv Preprint
Gizem Sogancioglu, Heysem Kaya
2022-12-15
Physician preference for receiving machine learning predictive results: A cross-sectional multicentric study.
In PloS one ; h5-index 176.0
Wichmann Roberta Moreira, Fagundes Thales Pardini, de Oliveira Tiago Almeida, Batista André Filipe de Moraes, Chiavegatto Filho Alexandre Dias Porto
2022
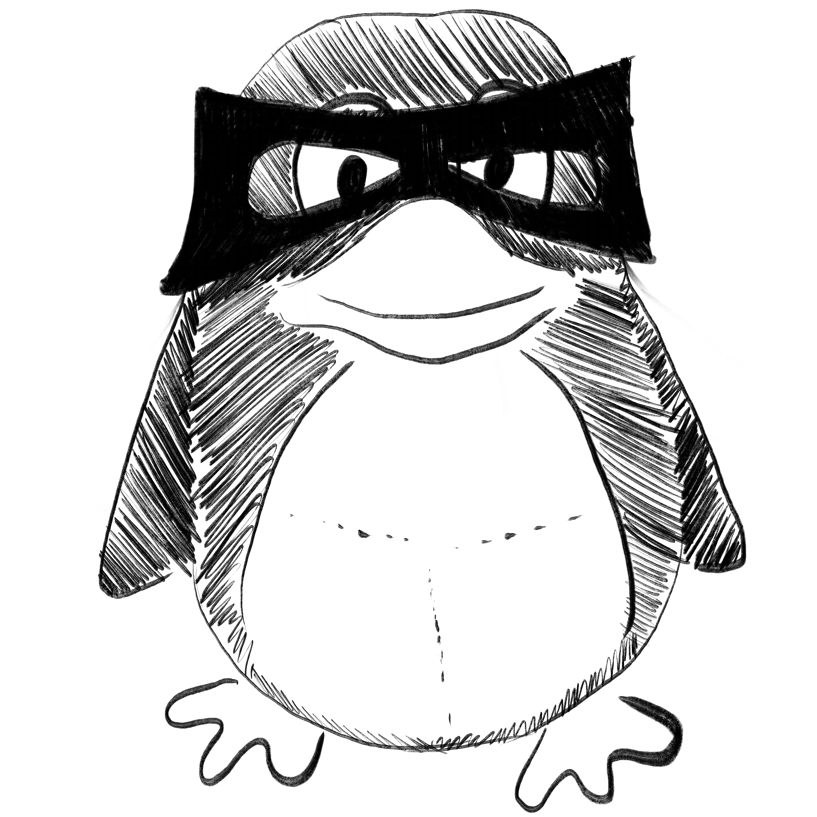
Statistical Design and Analysis for Robust Machine Learning: A Case Study from COVID-19
ArXiv Preprint
Davide Pigoli, Kieran Baker, Jobie Budd, Lorraine Butler, Harry Coppock, Sabrina Egglestone, Steven G. Gilmour, Chris Holmes, David Hurley, Radka Jersakova, Ivan Kiskin, Vasiliki Koutra, Jonathon Mellor, George Nicholson, Joe Packham, Selina Patel, Richard Payne, Stephen J. Roberts, Björn W. Schuller, Ana Tendero-Cañadas, Tracey Thornley, Alexander Titcomb
2022-12-15
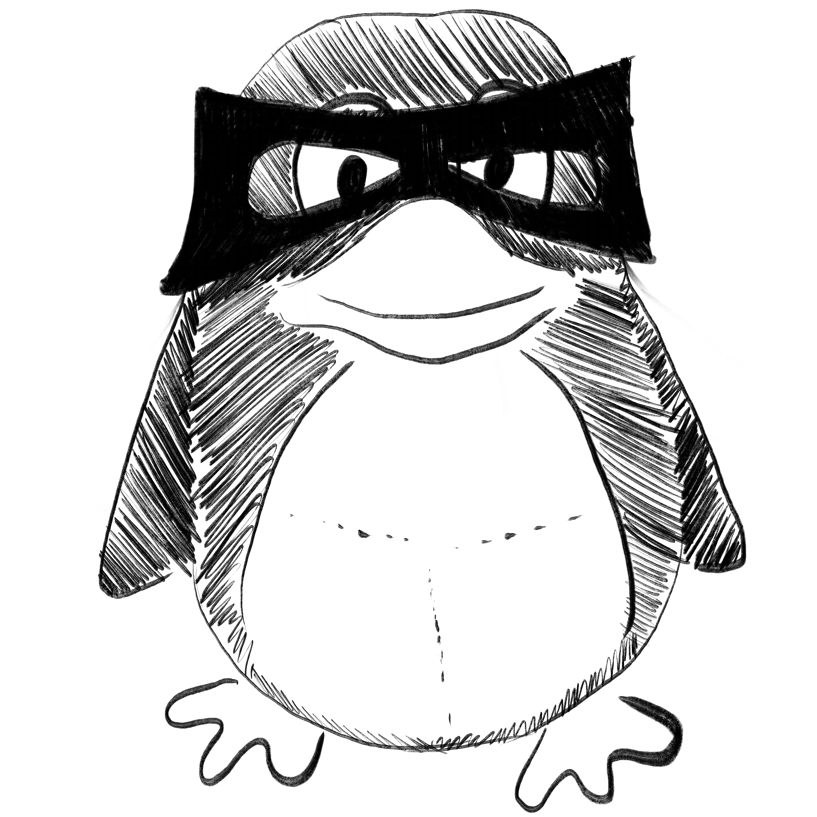
Doctor/Data Scientist/Artificial Intelligence Communication Model. Case Study.
In Procedia computer science
Belciug Smaranda, Ivanescu Renato Constantin, Popa Sebastian-Doru, Iliescu Dominic Gabriel
2022
computer aided medical diagnosis, congenital anomalies, deep learning, second trimester morphology, statistical learning, statistics
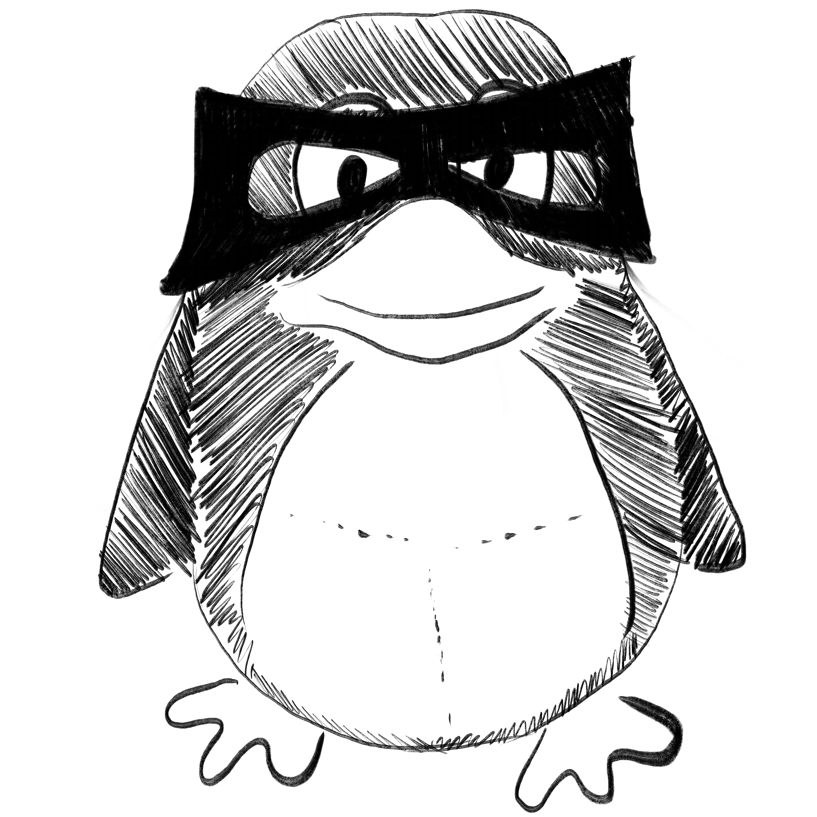
Chaotic Variational Auto Encoder based One Class Classifier for Insurance Fraud Detection
ArXiv Preprint
K. S. N. V. K. Gangadhar, B. Akhil Kumar, Yelleti Vivek, Vadlamani Ravi
2022-12-15
Mitogen Activated Protein Kinase (MAPK) Activation, p53, and Autophagy Inhibition Characterize the Severe Acute Respiratory Syndrome Coronavirus 2 (SARS-CoV-2) Spike Protein Induced Neurotoxicity.
In Cureus
Kyriakopoulos Anthony M, Nigh Greg, McCullough Peter A, Seneff Stephanie
2022-Dec
aging, autoimmunity, autophagy, covid-19, mrna vaccines, p53, prion and prion-like diseases, sars-cov-2 spike protein, senescence, wip1
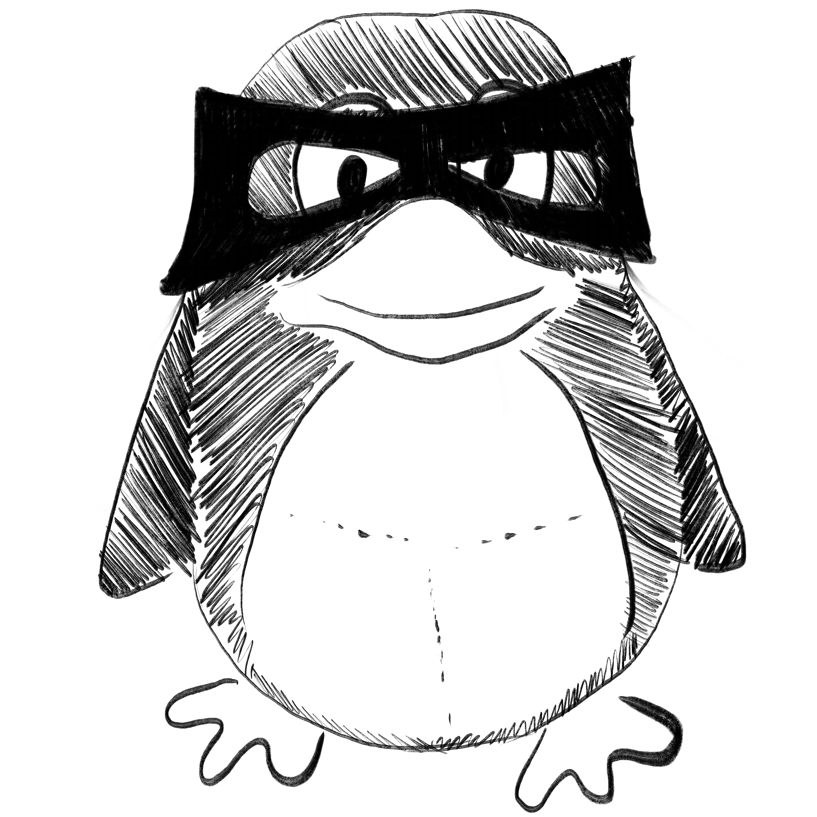
Crowdsourcing Temporal Transcriptomic Coronavirus Host Infection Data: resources, guide, and novel insights.
bioRxiv Preprint
Flynn, J.; Ahmadi, M.; McFarland, C.; Kubal, M.; Taylor, M.; Cheng, Z.; Torchia, E. C.; Edwards, M.
2022-12-15
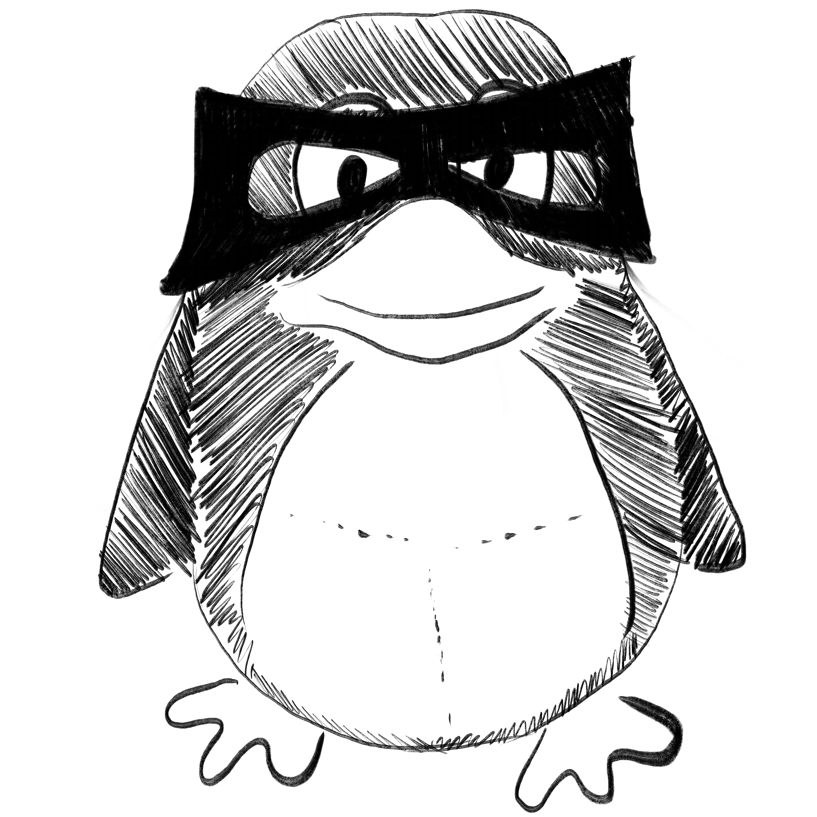
A large-scale and PCR-referenced vocal audio dataset for COVID-19
ArXiv Preprint
Jobie Budd, Kieran Baker, Emma Karoune, Harry Coppock, Selina Patel, Ana Tendero Cañadas, Alexander Titcomb, Richard Payne, David Hurley, Sabrina Egglestone, Lorraine Butler, Jonathon Mellor, George Nicholson, Ivan Kiskin, Vasiliki Koutra, Radka Jersakova, Rachel A. McKendry, Peter Diggle, Sylvia Richardson, Björn W. Schuller, Steven Gilmour, Davide Pigoli, Stephen Roberts, Josef Packham, Tracey Thornley, Chris Holmes
2022-12-15
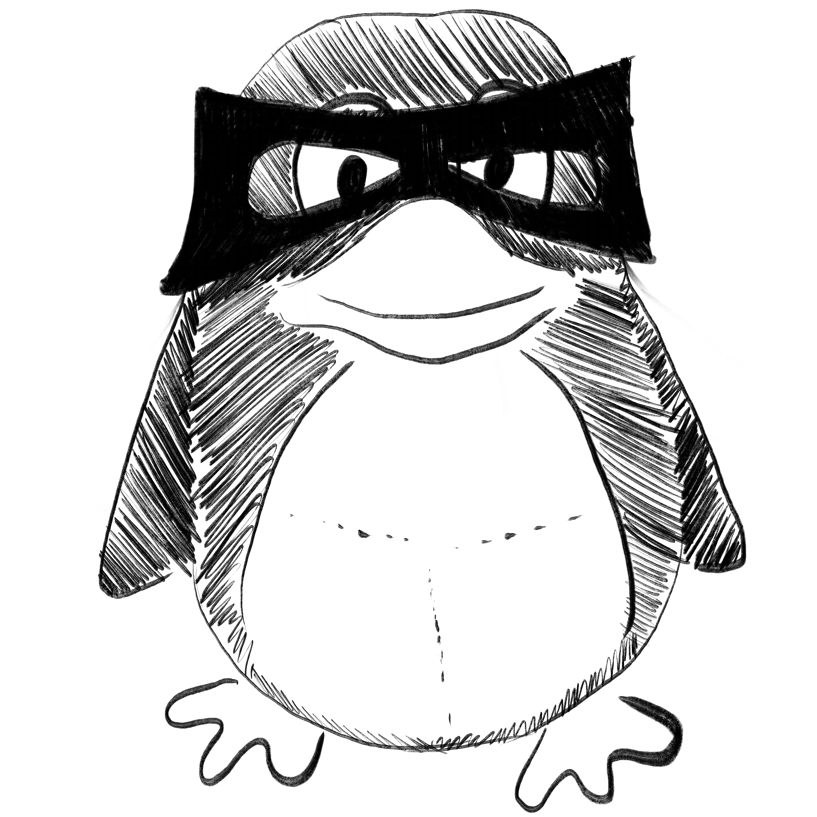
Vaccine supply chain management: An intelligent system utilizing blockchain, IoT and machine learning.
In Journal of business research
Hu Hui, Xu Jiajun, Liu Mengqi, Lim Ming K
2023-Feb
BILSTM, Bidirectional Long-Short Term Memory, Blockchain, CNN, Convolutional Neural Network, COVID-19 pandemic, DTs, Digital Technologies, GRU, Gate Recurrent Unit, IPFS, Interplanetary File System, Intelligent system, Internet of things, IoT, Internet of Things, LSTM, Long-Short Term Memory, Machine learning, RFID, Radio Frequency Identification, RNN, Recurrent Neural Network, VSC, Vaccine Supply Chain, Vaccine supply chain, dApp, Decentralized Application
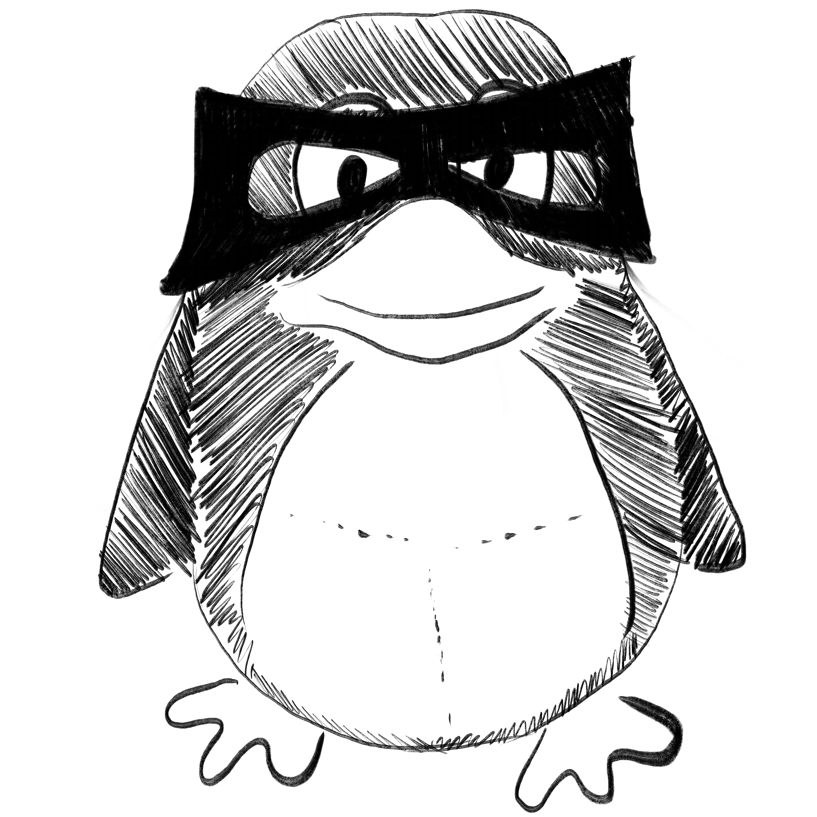
Finite-Sample Two-Group Composite Hypothesis Testing via Machine Learning.
In Journal of computational and graphical statistics : a joint publication of American Statistical Association, Institute of Mathematical Statistics, Interface Foundation of North America
Zhan Tianyu, Kang Jian
2022
Confirmatory adaptive clinical trials, Deep neural networks, Efficient inference methods, Neyman-Pearson Lemma, Research assistant tools
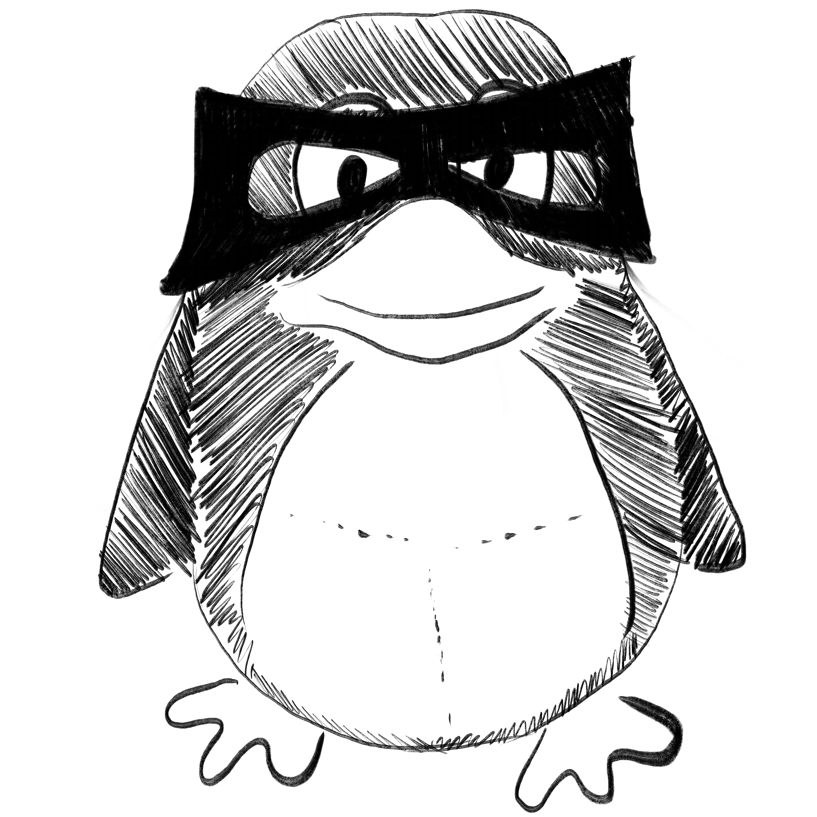
Structural analysis of SARS-CoV-2 Spike protein variants through graph embedding.
In Network modeling and analysis in health informatics and bioinformatics
Guzzi Pietro Hiram, Lomoio Ugo, Puccio Barbara, Veltri Pierangelo
2023
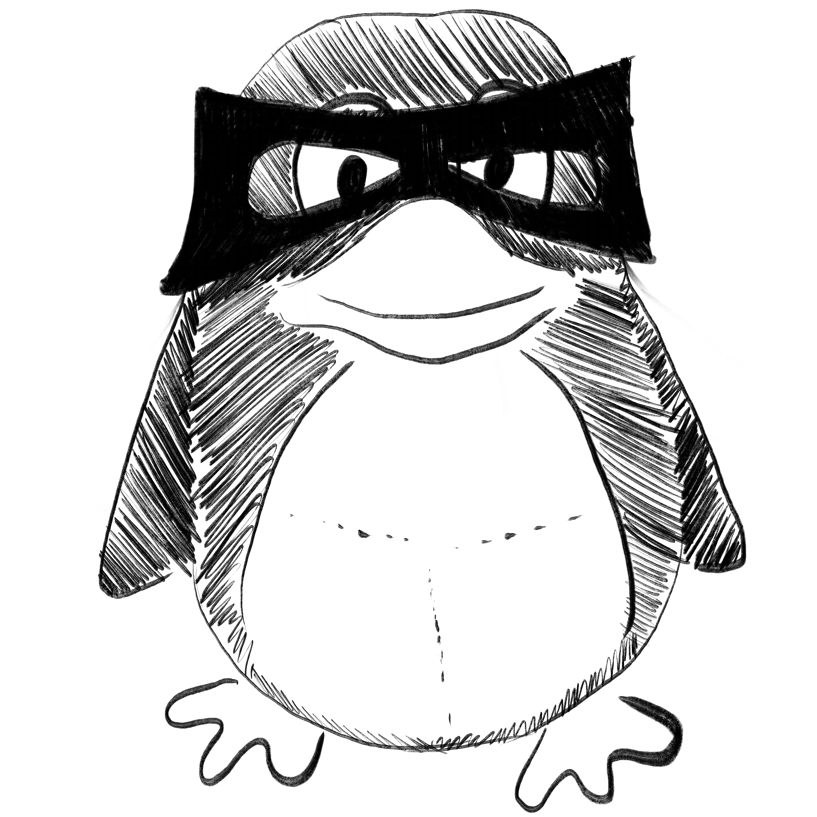
Profiling the B cell immune response elicited by vaccination against the respiratory virus SARS-CoV-2.
In Frontiers in immunology ; h5-index 100.0
Pettini Elena, Medaglini Donata, Ciabattini Annalisa
2022
B cell ELISpot, BCR repertoire, COVID-19 vaccines, SARS-CoV-2, computational flow cytometry, memory B cells, respiratory virus
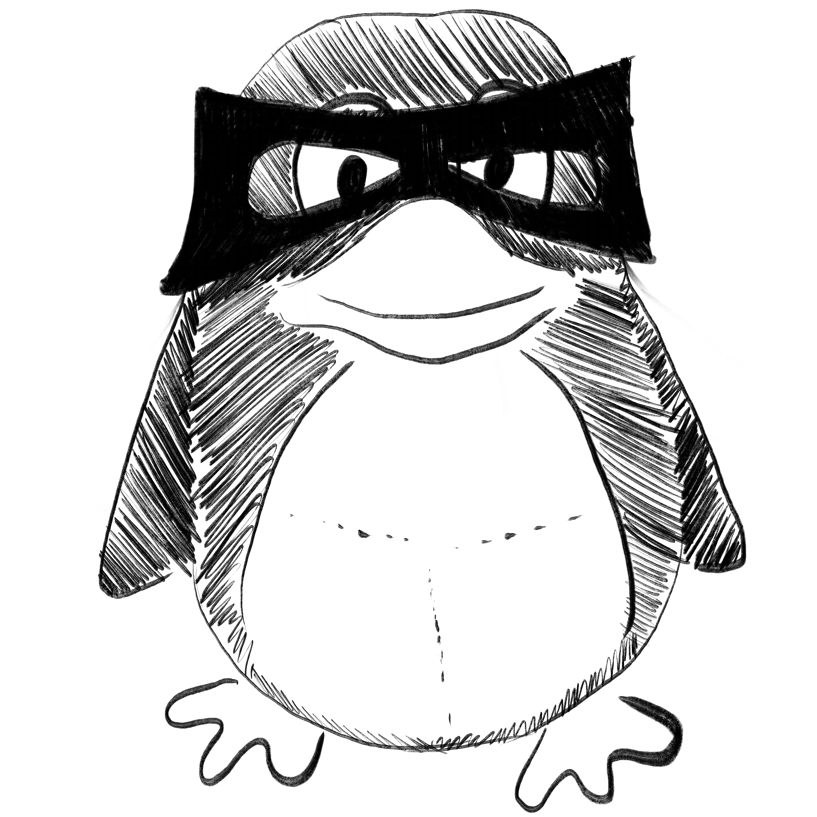
Vaccine supply chain coordination using blockchain and artificial intelligence technologies.
In Computers & industrial engineering
Gao Ye, Gao Hongwei, Xiao Han, Yao Fanjun
2022-Dec-05
AI technology, Blockchain technology, Differential game, Supply chain management, Vaccine supply chain
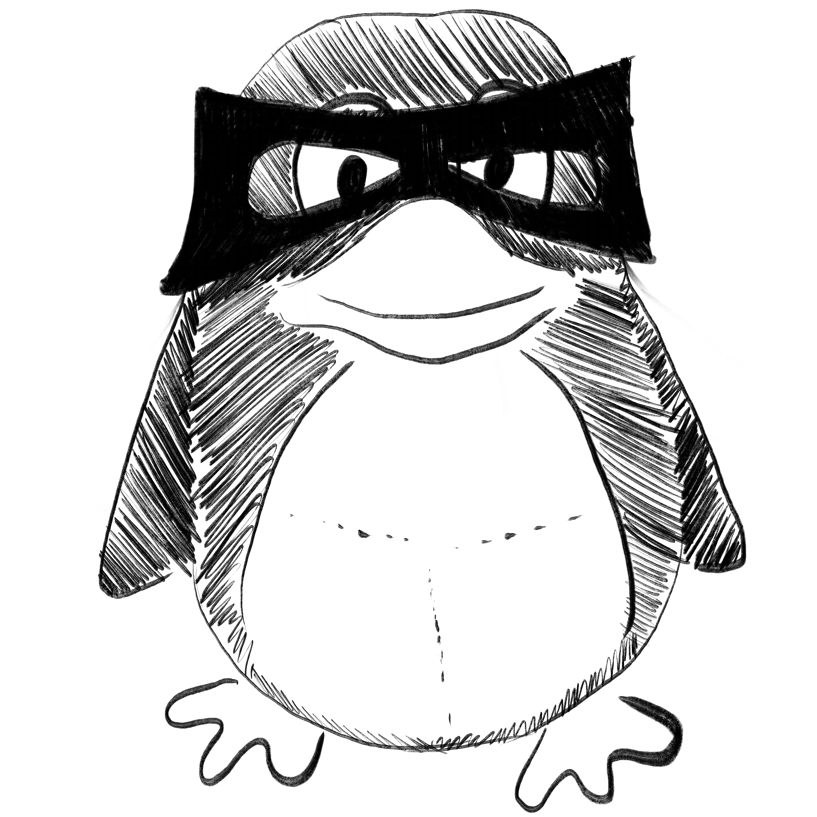
COVID-19 CT ground-glass opacity segmentation based on attention mechanism threshold.
In Biomedical signal processing and control
Rao Yunbo, Lv Qingsong, Zeng Shaoning, Yi Yuling, Huang Cheng, Gao Yun, Cheng Zhanglin, Sun Jihong
2023-Mar
Adaptive threshold, Attention mechanism, COVID-19, CT image, GGO segmentation, Pneumonia
How public health agencies communicate with the public on TikTok under the normalization of COVID-19: A case of 2022 Shanghai's outbreak.
In Frontiers in public health
OBJECTIVE :
METHODS :
RESULTS :
CONCLUSIONS :
Che ShaoPeng, Zhang Shunan, Kim Jang Hyun
2022
COVID-19, TikTok, communication strategy, crisis and emergency risk communication, negative binomial regression, public health agency, public health emergency, social media
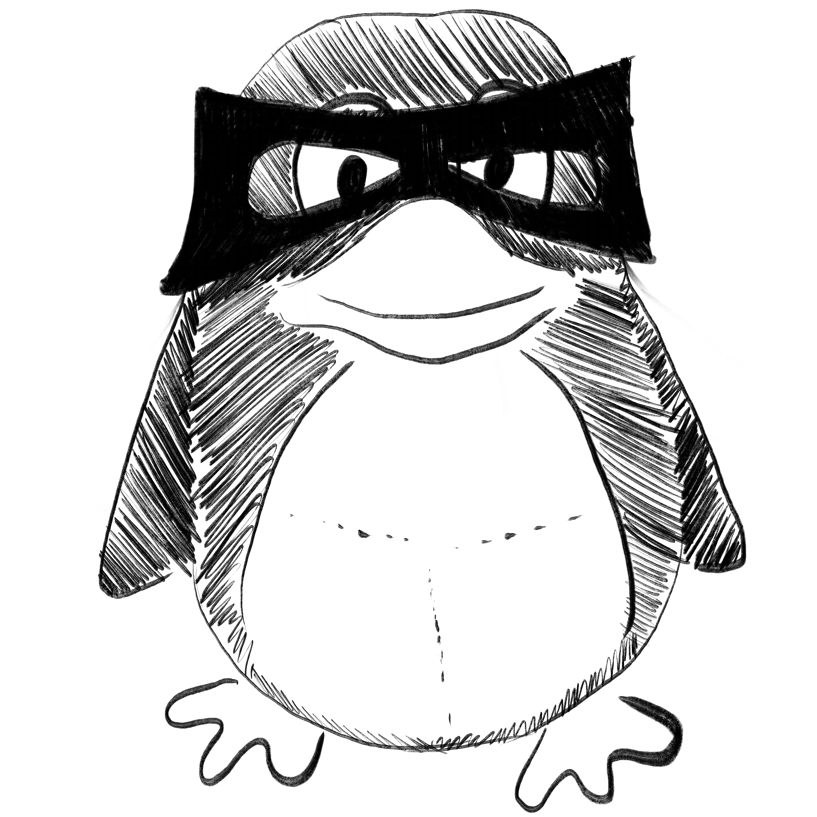
Detection of Covid-19 and other pneumonia cases from CT and X-ray chest images using deep learning based on feature reuse residual block and depthwise dilated convolutions neural network.
In Applied soft computing
Celik Gaffari
2022-Dec-07
Covid-19 diagnosis, Deep learning, Depthwise dilated convolutions, Feature reuse residual block, Gradient boosting
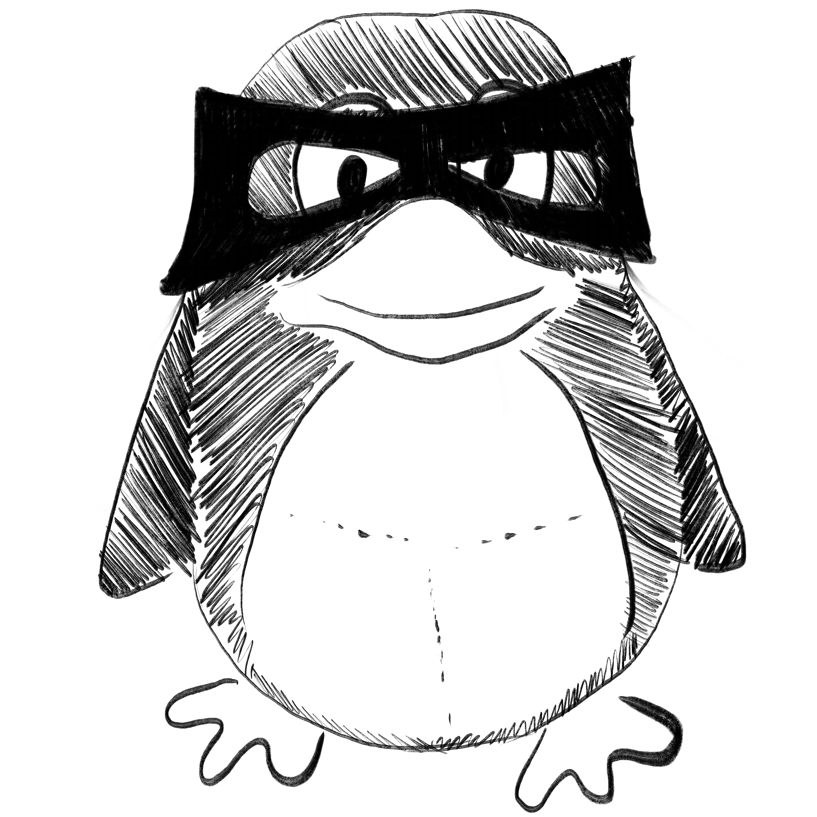
Automatic diagnosis of COVID-19 with MCA-inspired TQWT-based classification of chest X-ray images.
In Computers in biology and medicine
Jyoti Kumari, Sushma Sai, Yadav Saurabh, Kumar Pawan, Pachori Ram Bilas, Mukherjee Shaibal
2022-Nov-23
COVID-19, Chest X-ray images, Image decomposition and classification, Memristive crossbar array (MCA) based model, TQWT method
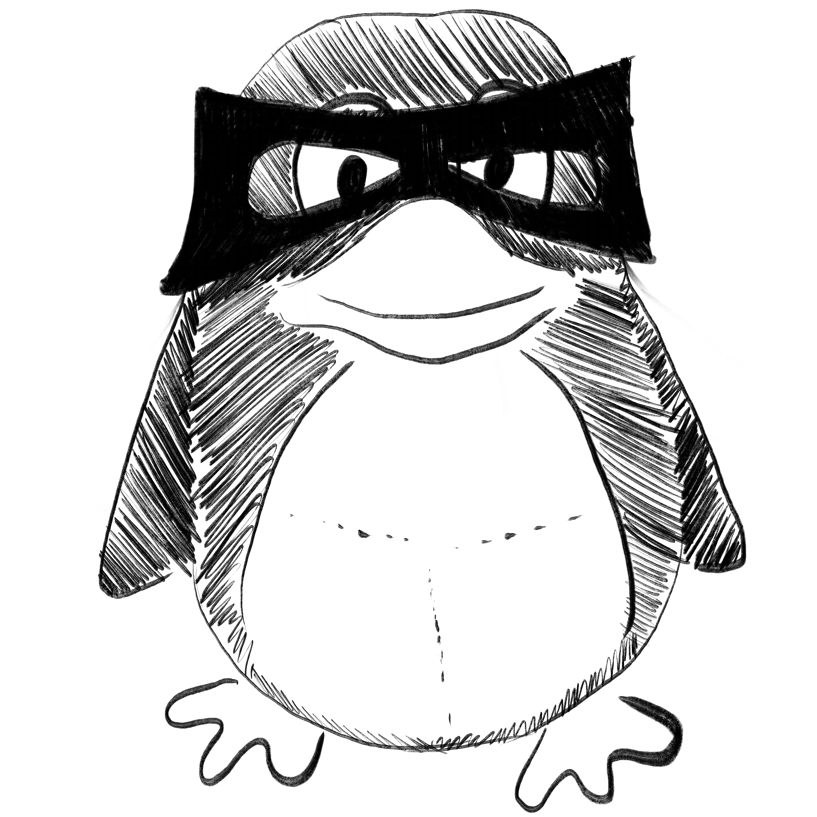
Diagnosis of COVID-19 Disease in Chest CT-Scan Images Based on Combination of Low-Level Texture Analysis and MobileNetV2 Features.
In Computational intelligence and neuroscience
Yazdani Azita, Fekri-Ershad Shervan, Jelvay Saeed
2022
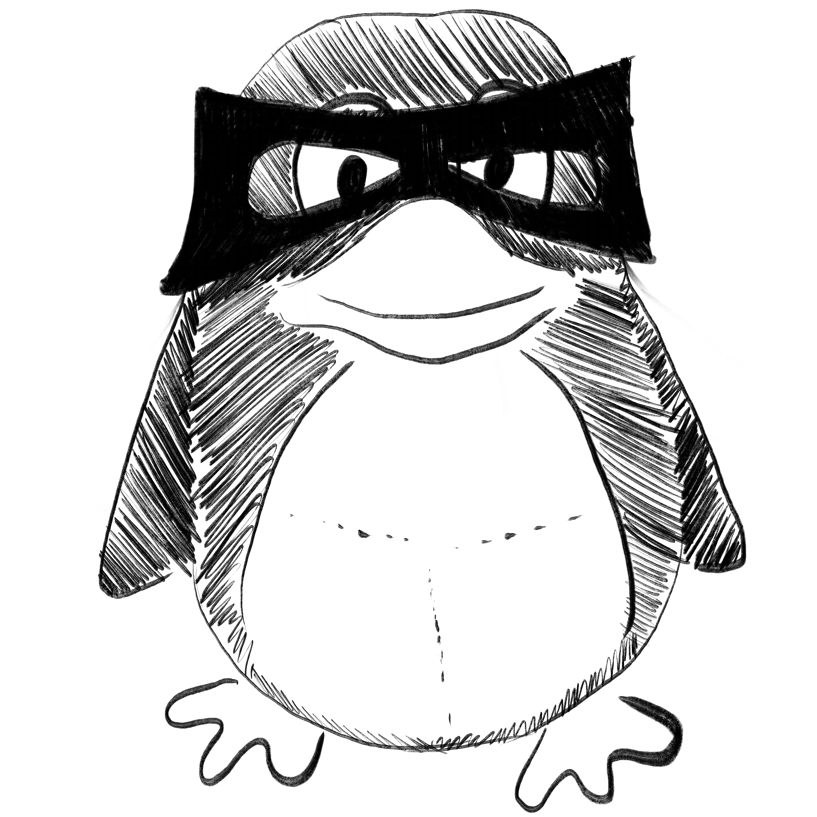
The influence of the Fourth Industrial Revolution on organisational culture: An empirical investigation.
In Frontiers in psychology ; h5-index 92.0
Singaram Shwetha, Mayer Claude-Hélène
2022
COVID-19, Fourth Industrial Revolution, South Africa, higher education, organisational culture
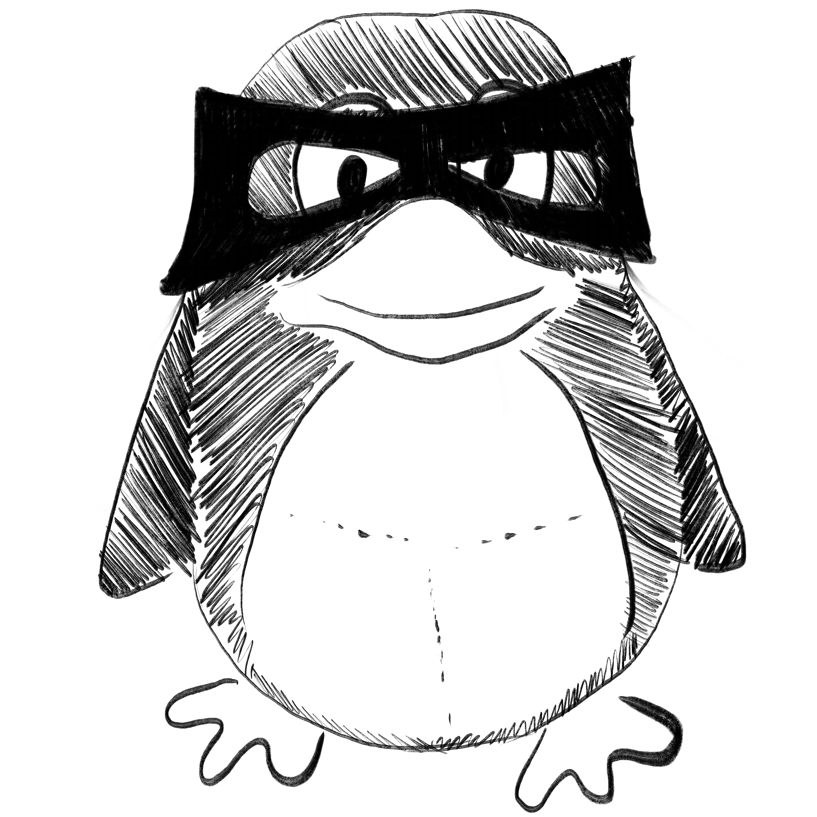
COVID-19 related TV news and stock returns: Evidence from major US TV stations.
In The Quarterly review of economics and finance : journal of the Midwest Economics Association
Möller Rouven, Reichmann Doron
2023-Feb
COVID-19 TV news, Natural language processing, Stock returns, Topic modeling
Toward the determination of sensitive and reliable whole-lung computed tomography features for robust standard radiomics and delta-radiomics analysis in a nonhuman primate model of coronavirus disease 2019.
In Journal of medical imaging (Bellingham, Wash.)
PURPOSE :
APPROACH :
RESULTS :
CONCLUSIONS :
Castro Marcelo A, Reza Syed, Chu Winston T, Bradley Dara, Lee Ji Hyun, Crozier Ian, Sayre Philip J, Lee Byeong Y, Mani Venkatesh, Friedrich Thomas C, O’Connor David H, Finch Courtney L, Worwa Gabriella, Feuerstein Irwin M, Kuhn Jens H, Solomon Jeffrey
2022-Nov
COVID-19, animal models, computed tomography, radiomics, reliability, sensitivity
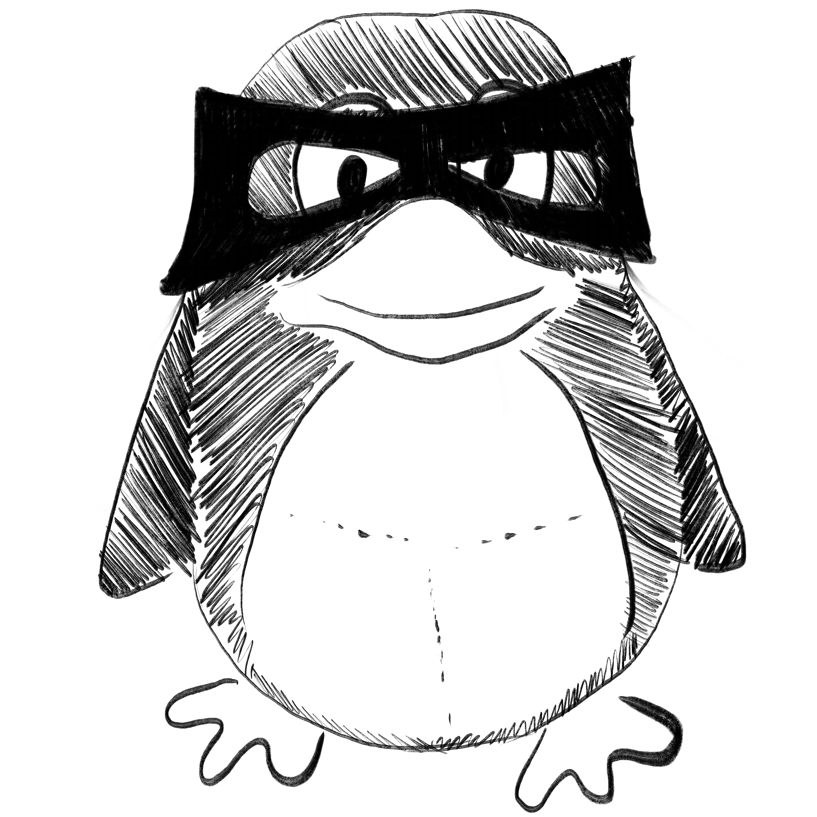
Analyzing the Prospects of Blockchain in Healthcare Industry.
In Computational and mathematical methods in medicine
Srivastava Shilpa, Pant Millie, Jauhar Sunil Kumar, Nagar Atulya K
2022
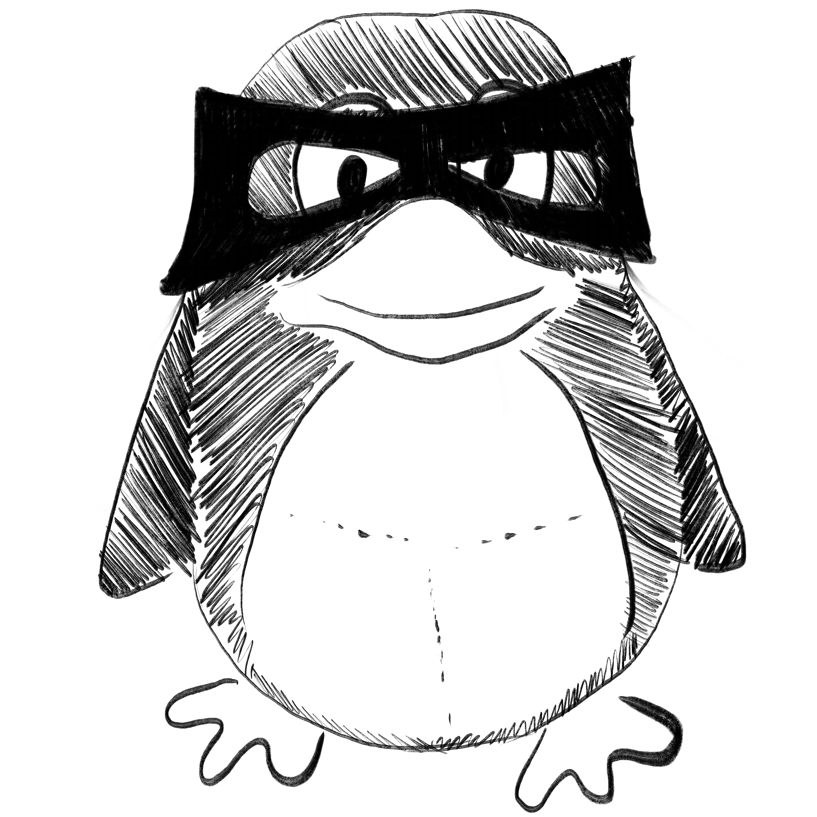
SARS-CoV-2 primary and breakthrough infections in patients with cancer: Implications for patient care.
In Best practice & research. Clinical haematology
Wang Lindsey, Wang William, Xu Rong, Berger Nathan A
2022-Sep
COVID-19, Cancer, Hematologic malignancies, SARS-CoV-2
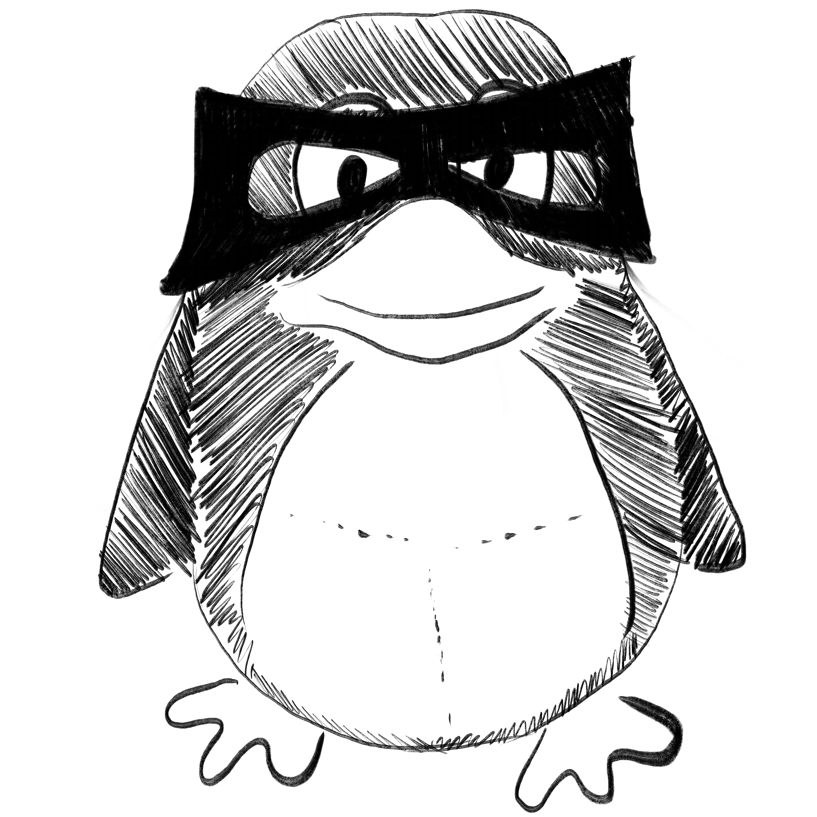
US-Net: A lightweight network for simultaneous speckle suppression and texture enhancement in ultrasound images.
In Computers in biology and medicine
BACKGROUND :
METHODS :
RESULTS :
CONCLUSIONS :
Monkam Patrice, Lu Wenkai, Jin Songbai, Shan Wenjun, Wu Jing, Zhou Xiang, Tang Bo, Zhao Hua, Zhang Hongmin, Ding Xin, Chen Huan, Su Longxiang
2022-Nov-30
Deep learning, Improved diagnostic accuracy, Speckle suppression, Texture enhancement, Ultrasound image
A multistage multimodal deep learning model for disease severity assessment and early warnings of high-risk patients of COVID-19.
In Frontiers in public health
Li Zhuo, Xu Ruiqing, Shen Yifei, Cao Jiannong, Wang Ben, Zhang Ying, Li Shikang
2022
COVID-19, disease progression prediction, disease severity assessment, multimodal feature fusion, sequential stage-wise learning
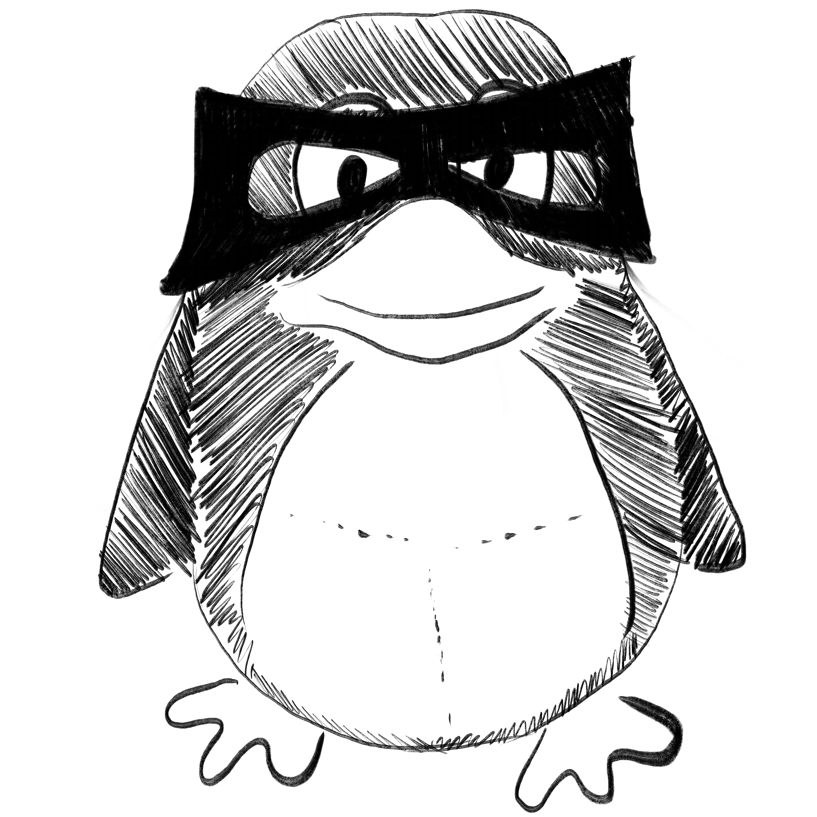
Deep Evolutionary Forecasting identifies highly-mutated SARS-CoV-2 variants via functional sequence-landscape enumeration.
In Research square
Colom Mireia Solà, Vucinic Jelena, Adolf-Bryfogle Jared, Bowman James W, Verel Sébastien, Moczygemba Isabelle, Schiex Thomas, Simoncini David, Bahl Christopher D
2022-Dec-02
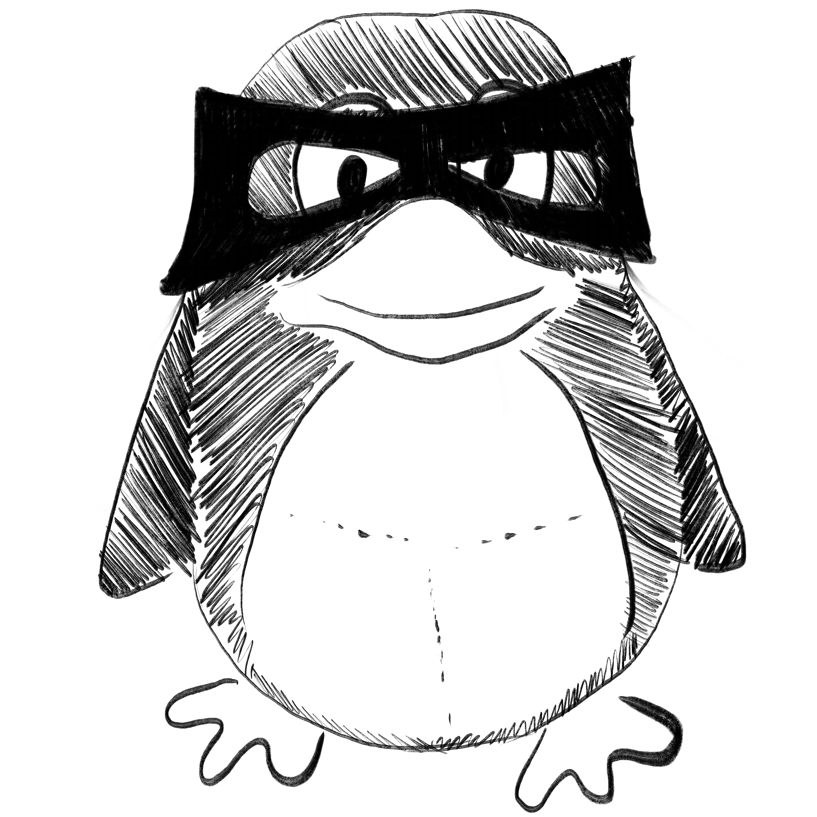
[Chest imaging-based artificial intelligence in the diagnosis of coronavirus disease 2019 and prospects for future research].
In Zhonghua jie he he hu xi za zhi = Zhonghua jiehe he huxi zazhi = Chinese journal of tuberculosis and respiratory diseases
Li Y, Liu S Y, Zheng J P
2022-Dec-12
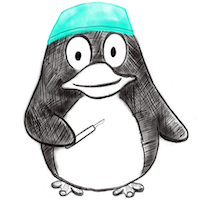
Preparing for the next pandemic: Simulation-based deep reinforcement learning to discover and test multimodal control of systemic inflammation using repurposed immunomodulatory agents.
In Frontiers in immunology ; h5-index 100.0
BACKGROUND :
METHODS :
RESULTS :
DISCUSSION :
Cockrell Chase, Larie Dale, An Gary
2022
COVID - 19, agent - based modeling, cytokine storm, deep reinforcement learning, drug repurposing, machine learning and AI, multiscale modeling and simulation, sepsis
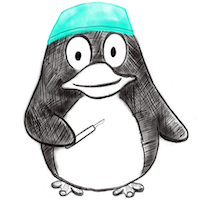
Military Medical Role in Civilian Disaster.
In AACN advanced critical care
Flarity Kathleen, DeDecker Lisa D, Averett-Brauer Tamara A, Duquette-Frame Teresa, Rougeau Tami R, Aycock Andrew, Urban Shane, McKay Jerome T, Cox Daniel B
2022-Dec-15
civil defense, disaster medicine, disasters, military, military medicine
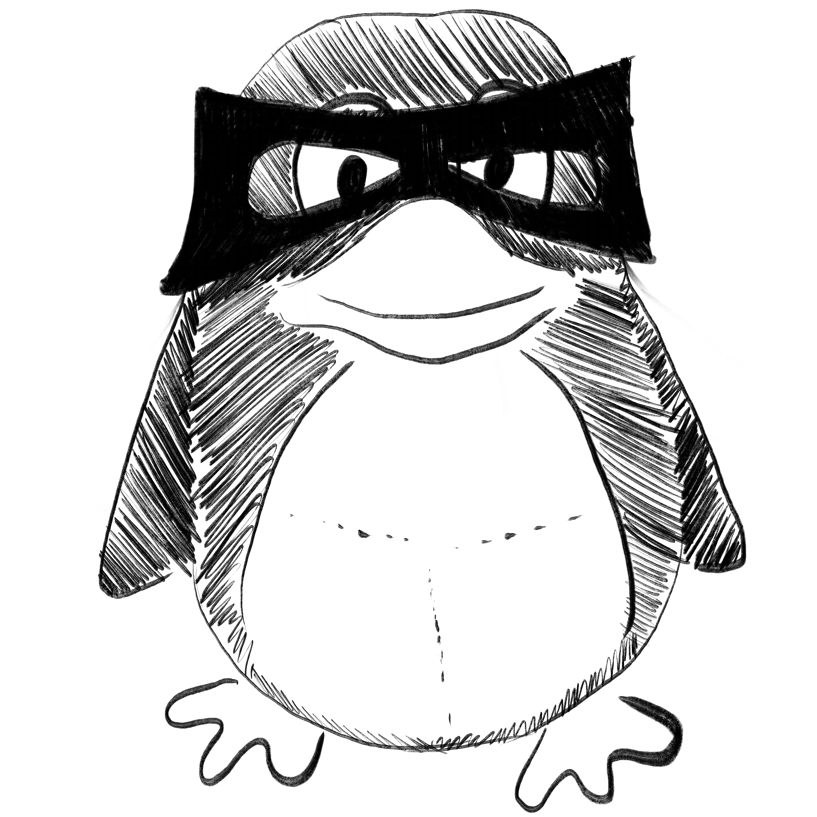
Insights from Incorporating Quantum Computing into Drug Design Workflows.
In Bioinformatics (Oxford, England)
MOTIVATION :
RESULTS :
AVAILABILITY :
SUPPLEMENTARY INFORMATION :
Lau Bayo, Emani Prashant S, Chapman Jackson, Yao Lijing, Lam Tarsus, Merrill Paul, Warrell Jonathan, Gerstein Mark B, Lam Hugo Y K
2022-Dec-07
Prediction of oxygen requirement in patients with COVID-19 using a pre-trained chest radiograph xAI model: efficient development of auditable risk prediction models via a fine-tuning approach.
In Scientific reports ; h5-index 158.0
Chung Joowon, Kim Doyun, Choi Jongmun, Yune Sehyo, Song Kyungdoo, Kim Seonkyoung, Chua Michelle, Succi Marc D, Conklin John, Longo Maria G Figueiro, Ackman Jeanne B, Petranovic Milena, Lev Michael H, Do Synho
2022-Dec-07
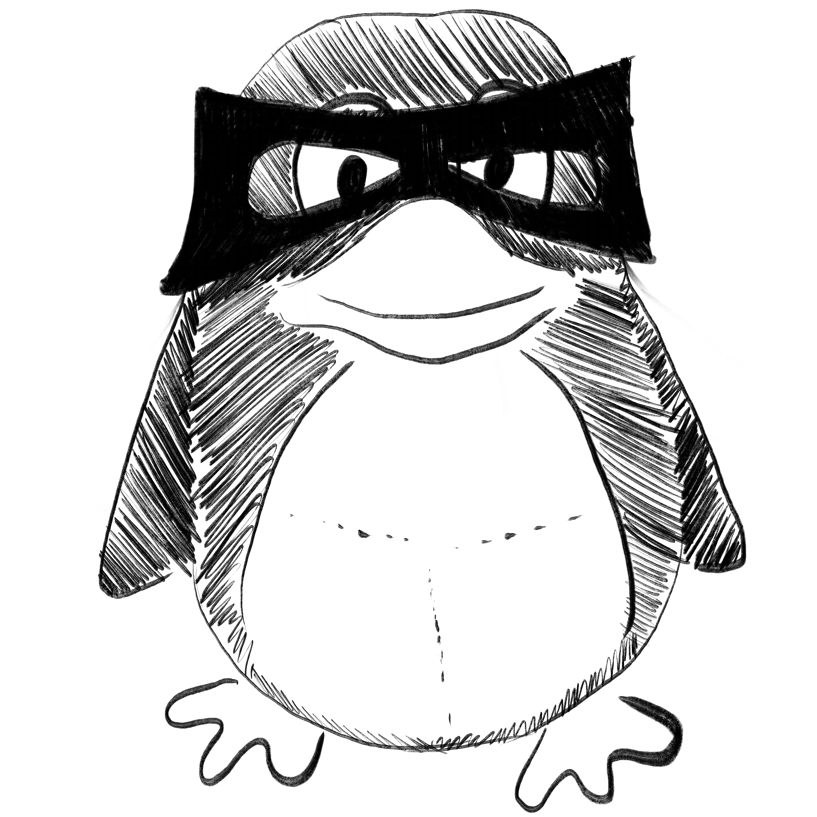
Cov-caldas: A new COVID-19 chest X-Ray dataset from state of Caldas-Colombia.
In Scientific data
Alzate-Grisales Jesús Alejandro, Mora-Rubio Alejandro, Arteaga-Arteaga Harold Brayan, Bravo-Ortiz Mario Alejandro, Arias-Garzón Daniel, López-Murillo Luis Humberto, Mercado-Ruiz Esteban, Villa-Pulgarin Juan Pablo, Cardona-Morales Oscar, Orozco-Arias Simon, Buitrago-Carmona Felipe, Palancares-Sosa Maria Jose, Martínez-Rodríguez Fernanda, Contreras-Ortiz Sonia H, Saborit-Torres Jose Manuel, Montell Serrano Joaquim Ángel, Ramirez-Sánchez María Mónica, Sierra-Gaber Mario Alfonso, Jaramillo-Robledo Oscar, de la Iglesia-Vayá Maria, Tabares-Soto Reinel
2022-Dec-07
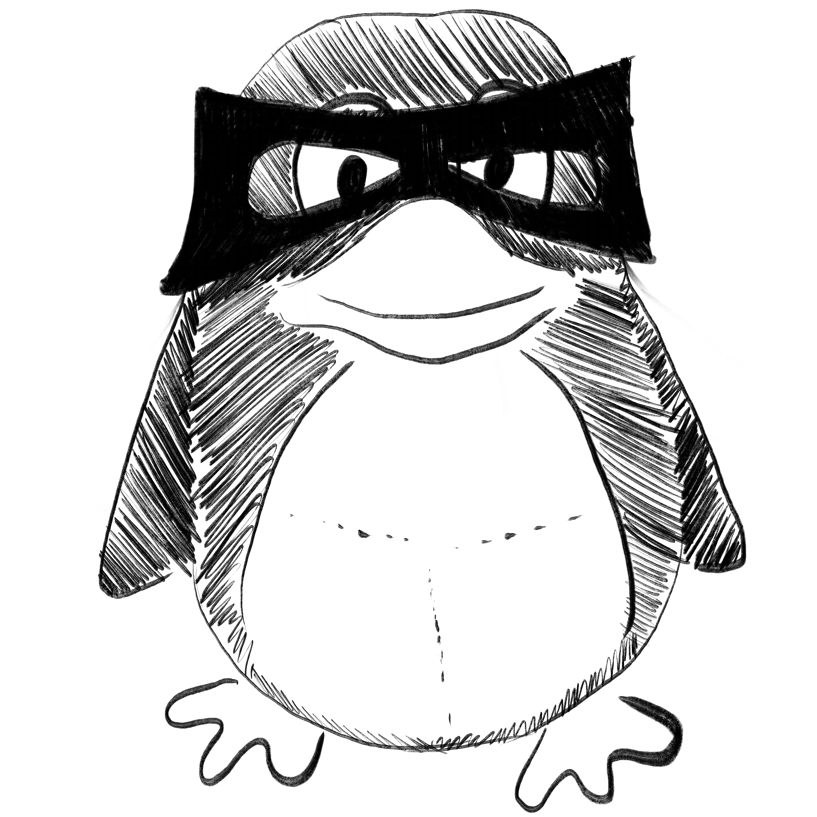
The First Case Series From Japan of Primary Headache Patients Treated by Completely Online Telemedicine.
In Cureus
Katsuki Masahito
2022-Nov
artificial intelligence, coronavirus disease 2019 (covid-19), information technology, medication-overuse headache (moh), migraine, online telemedicine, tension-type headache
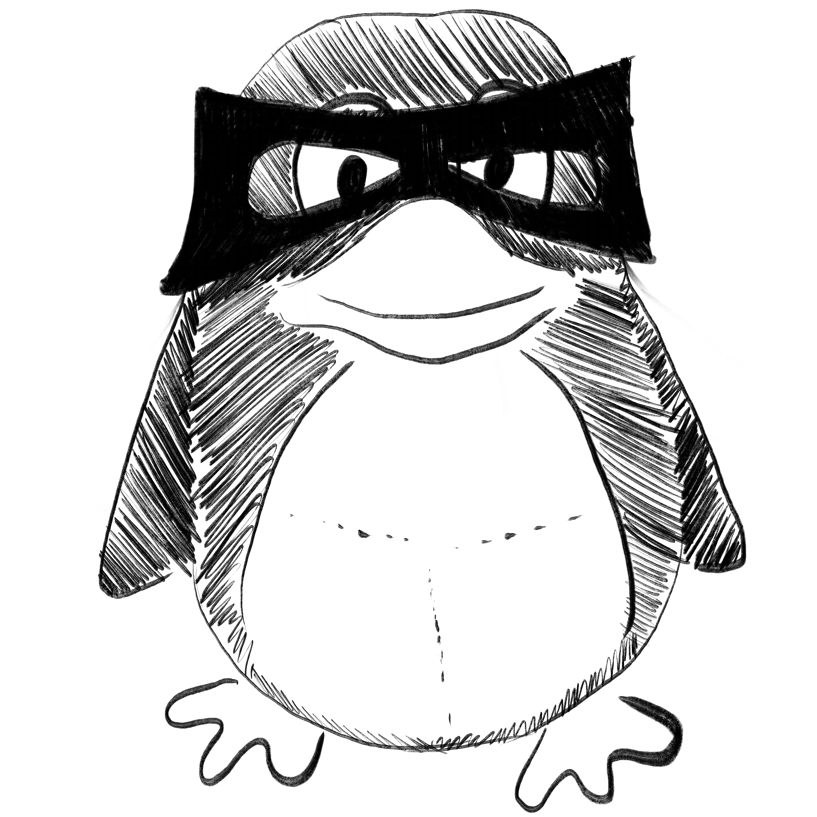
Exploring the factors that affect user experience in mobile-health applications: A text-mining and machine-learning approach.
In Journal of business research
Pal Shounak, Biswas Baidyanath, Gupta Rohit, Kumar Ajay, Gupta Shivam
2023-Feb
Machine learning, Mobile health, Proportional-odds logit, Service-dominant logic, Text analytics
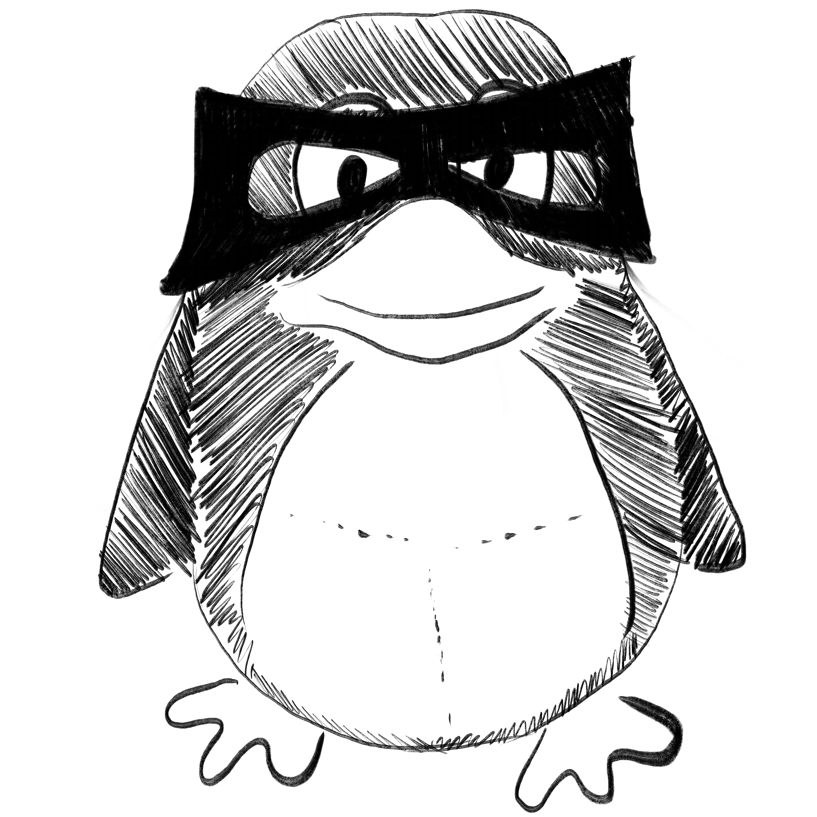
Dynamics of the COVID-19 pandemic: nonlinear approaches on the modelling, prediction and control.
In The European physical journal. Special topics
Banerjee Santo
2022-Dec-02
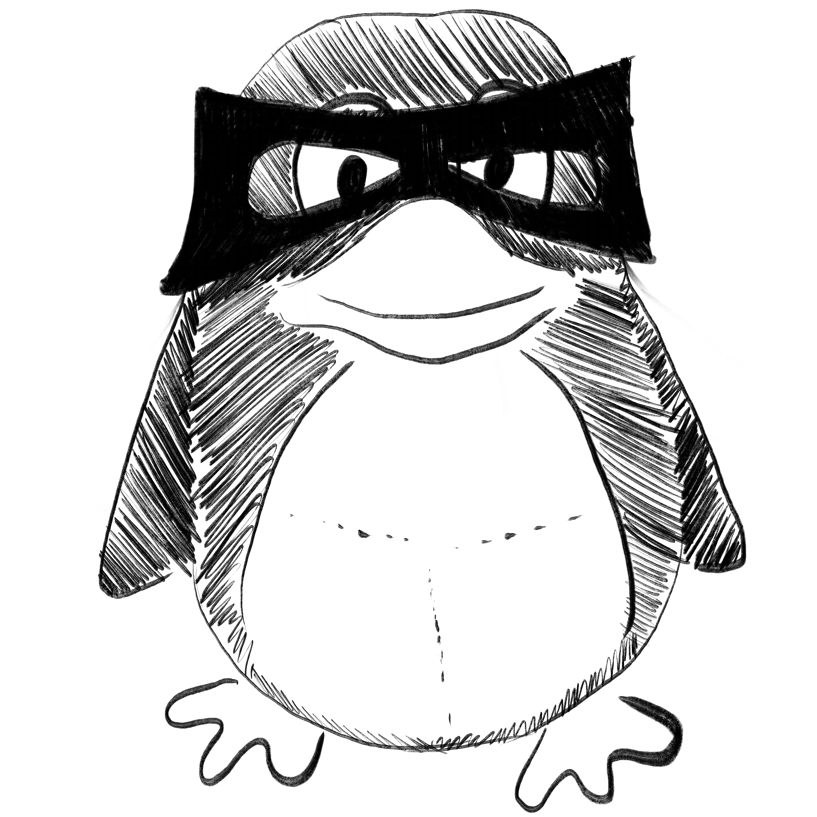
Supply chain risk management with machine learning technology: A literature review and future research directions.
In Computers & industrial engineering
Yang Mei, Lim Ming K, Qu Yingchi, Ni Du, Xiao Zhi
2023-Jan
Algorithm, COVID-19, Machine learning, Research status, Supply chain risk management
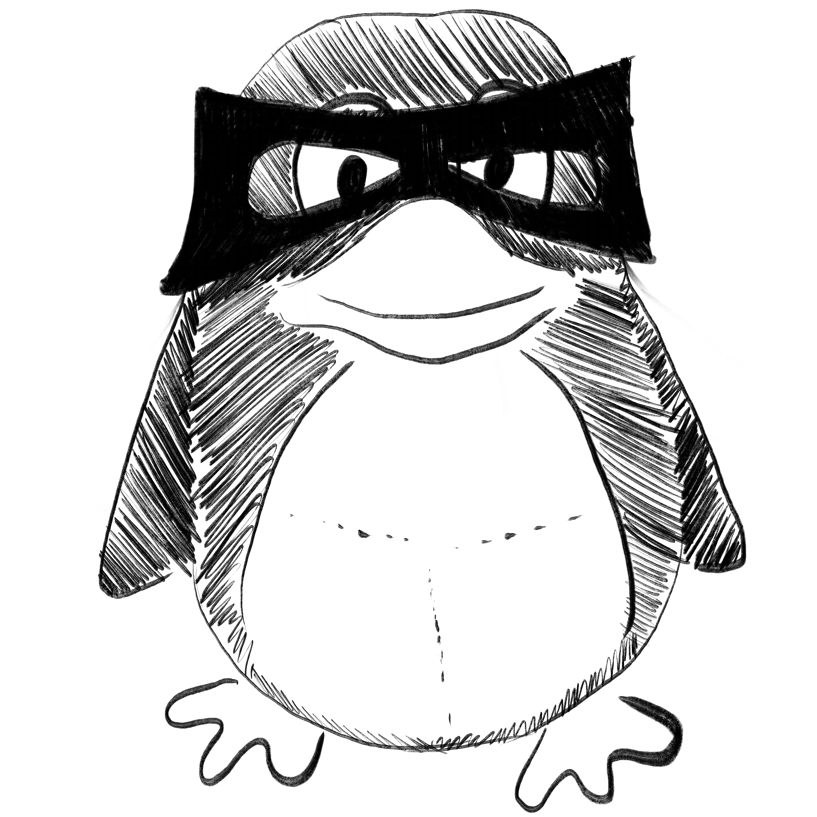
Automatic approach for mask detection: effective for COVID-19.
In Soft computing
Banik Debajyoty, Rawat Saksham, Thakur Aayush, Parwekar Pritee, Satapathy Suresh Chandra
2022-Dec-02
Boundary-layer meteorology, CNN (Convolutional neural network), COVID-19, Grad CAM, MobileNetV2
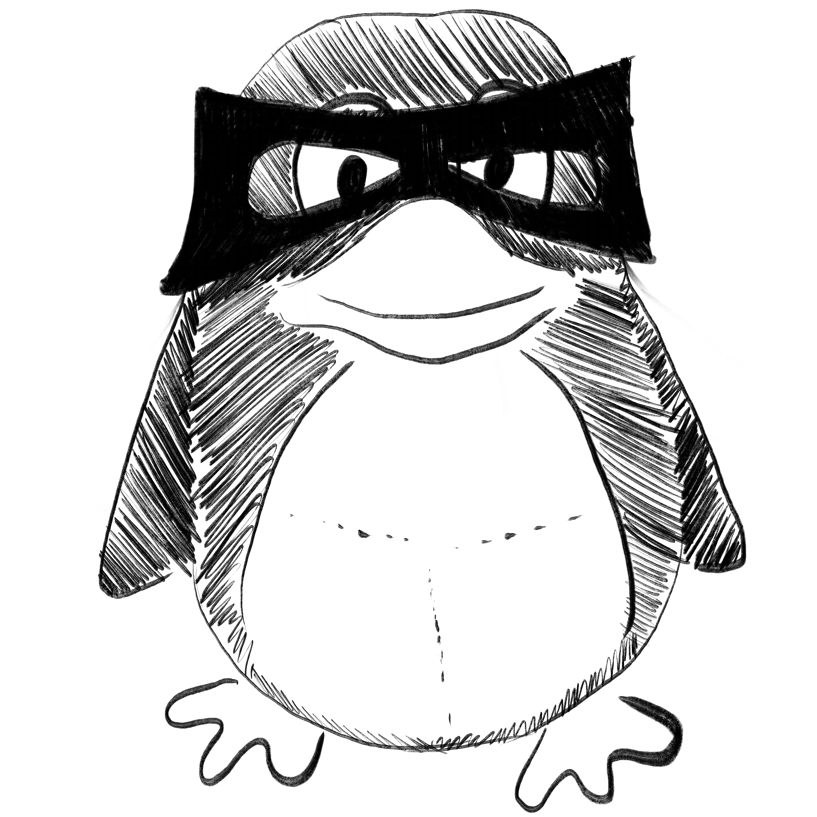
Immunophenotypes of anti-SARS-CoV-2 responses associated with fatal COVID-19.
In ERJ open research
BACKGROUND :
METHODS :
RESULTS :
CONCLUSIONS :
Šelb Julij, Bitežnik Barbara, Bidovec Stojković Urška, Rituper Boštjan, Osolnik Katarina, Kopač Peter, Svetina Petra, Cerk Porenta Kristina, Šifrer Franc, Lorber Petra, Trinkaus Leiler Darinka, Hafner Tomaž, Jerič Tina, Marčun Robert, Lalek Nika, Frelih Nina, Bizjak Mojca, Lombar Rok, Nikolić Vesna, Adamič Katja, Mohorčič Katja, Grm Zupan Sanja, Šarc Irena, Debeljak Jerneja, Koren Ana, Luzar Ajda Demšar, Rijavec Matija, Kern Izidor, Fležar Matjaž, Rozman Aleš, Korošec Peter
2022-Oct
MRI Assessment of Cerebral Blood Flow in Nonhospitalized Adults Who Self-Isolated Due to COVID-19.
In Journal of magnetic resonance imaging : JMRI
BACKGROUND :
PURPOSE :
STUDY TYPE :
POPULATION :
FIELD STRENGTH AND SEQUENCES :
ASSESSMENT :
STATISTICAL TESTS :
RESULTS :
DATA CONCLUSION :
EVIDENCE LEVEL :
TECHNICAL EFFICACY :
Kim William S H, Ji Xiang, Roudaia Eugenie, Chen J Jean, Gilboa Asaf, Sekuler Allison, Gao Fuqiang, Lin Zhongmin, Jegatheesan Aravinthan, Masellis Mario, Goubran Maged, Rabin Jennifer S, Lam Benjamin, Cheng Ivy, Fowler Robert, Heyn Chris, Black Sandra E, Graham Simon J, MacIntosh Bradley J
2022-Dec-06
COVID-19, SARS-CoV-2, cerebral blood flow, fatigue, post-COVID-19
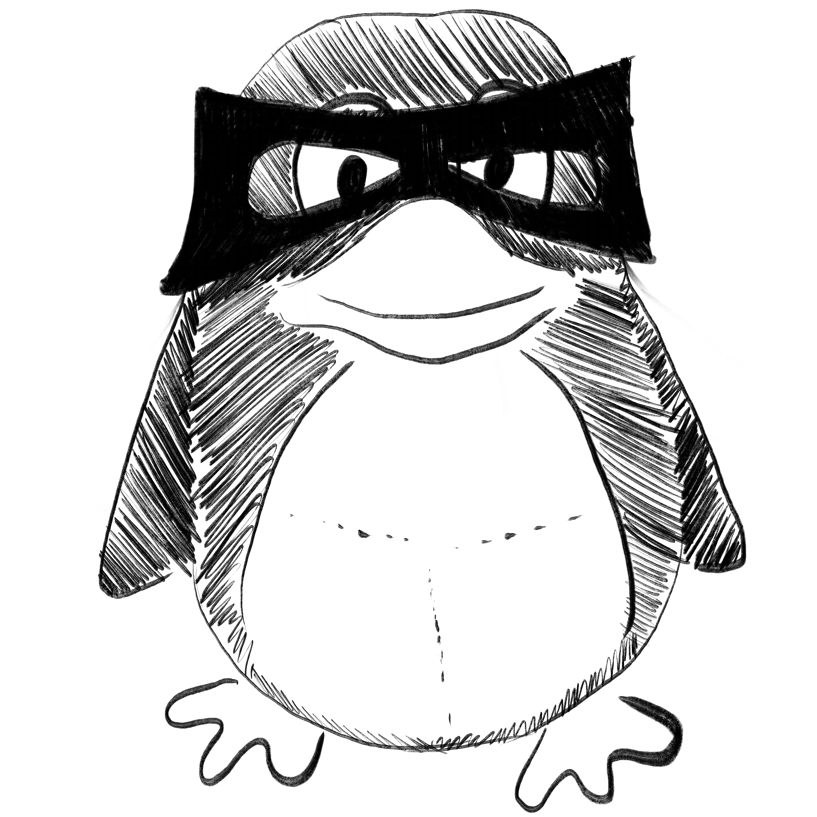
Harris hawks optimization for COVID-19 diagnosis based on multi-threshold image segmentation.
In Neural computing & applications
Ryalat Mohammad Hashem, Dorgham Osama, Tedmori Sara, Al-Rahamneh Zainab, Al-Najdawi Nijad, Mirjalili Seyedali
2022-Dec-01
CT images, Covid-19, Harris hawks optimization, Image segmentation, Multilevel thresholding, Otsu method
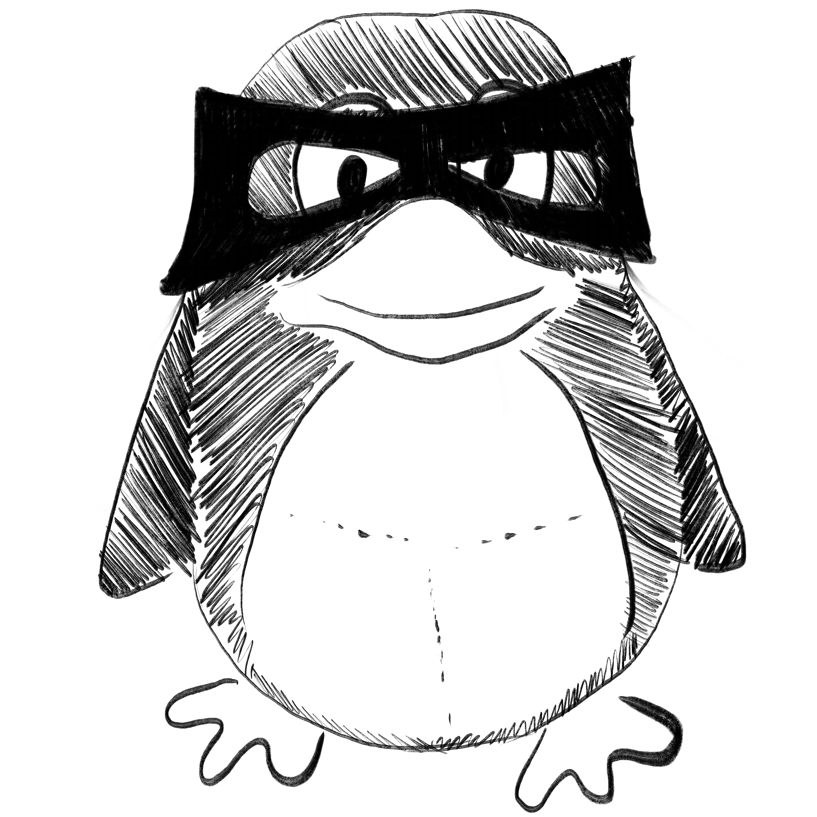
Comprehensively identifying Long Covid articles with human-in-the-loop machine learning.
In Patterns (New York, N.Y.)
Leaman Robert, Islamaj Rezarta, Allot Alexis, Chen Qingyu, Wilbur W John, Lu Zhiyong
2022-Dec-01
COVID-19, Long Covid, active learning, data programming, machine learning, natural language processing, post-acute sequelae of SARS-CoV-2 infection, text classification, weak supervision
A four-generation family transmission chain of COVID-19 along the China-Myanmar border in October to November 2021.
In Frontiers in public health
BACKGROUND :
METHODS :
RESULTS :
CONCLUSION :
Yan Xiangyu, Xiao Wei, Zhou Saipeng, Wang Xuechun, Wang ZeKun, Zhao Mingchen, Li Tao, Jia Zhongwei, Zhang Bo, Shui Tiejun
2022
COVID-19, China-Myanmar border, outbreak, refugees, transmission chain
The machine learning model based on trajectory analysis of ribonucleic acid test results predicts the necessity of quarantine in recurrently positive patients with SARS-CoV-2 infection.
In Frontiers in public health
BACKGROUND :
METHODS :
RESULTS :
CONCLUSION :
Song Qi-Xiang, Jin Zhichao, Fang Weilin, Zhang Chenxu, Peng Chi, Chen Min, Zhuang Xu, Zhai Wei, Wang Jun, Cao Min, Wei Shun, Cai Xia, Pan Lei, Xu Qingrong, Zheng Junhua
2022
SARS-CoV-2, infectivity, nucleic acid test, recurrently positive, viral load, virus isolation
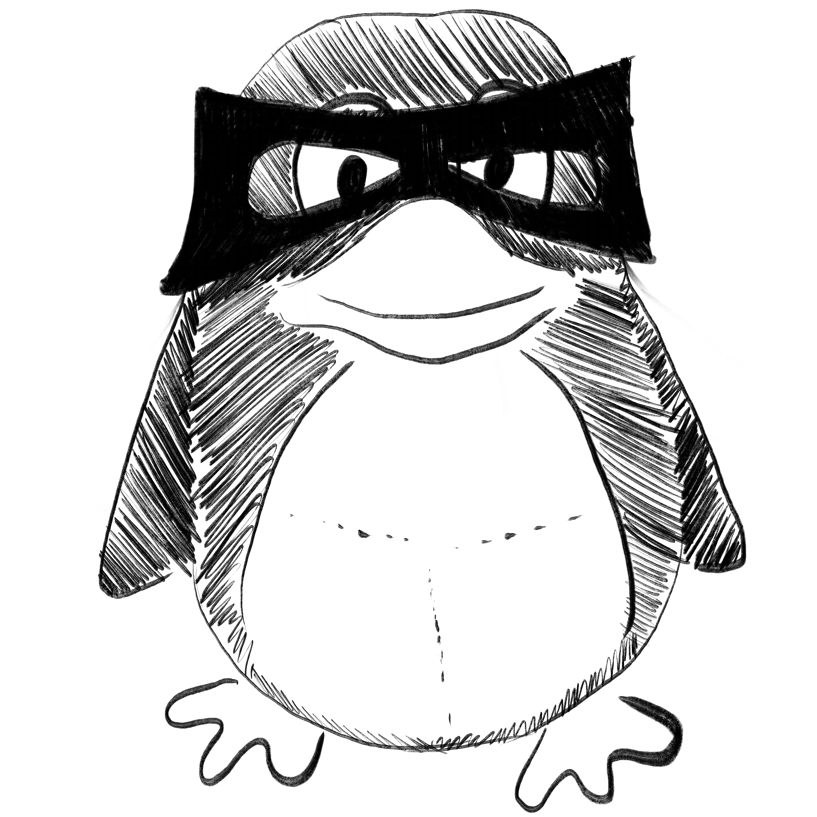
Repurposing of drugs for combined treatment of COVID-19 cytokine storm using machine learning.
In Medicine in drug discovery
Gantla Maanaskumar R, Tsigelny Igor F, Kouznetsova Valentina L
2022-Nov-29
1D 2D 3D, one- two- three-dimensional, ADAM17, A disintegrin and metalloprotease 17, ARDS, acute respiratory distress syndrome, AT1R, Angiotensin II receptor type 1, AUROC, area under receiver operator characteristic curve, COVID–19, coronavirus disease 2019, COVID–19, CRS, cytokine release syndrome, CXCL10, CXC–chemokine ligand 10, FDA, Food and Drug Administration, G–CSF, granulocyte colony stimulating factor, IC50, half maximal inhibitory concentration, ICU, intensive care unit, IL, interleukin, JAK1, Janus kinase 1, MCP1, monocyte chemoattractant protein–1, MIP1α, macrophage inflammatory protein 1, ML, machine learning, NF–κB, Nuclear Factor–Kappa B, PDB, Protein Data Bank, PaDEL, Pharmaeutical data exploration laboratory, ROC, receiver operator characteristic curve, SARS–CoV–2, SMILES, Simplified Molecular-Input Line-Entry System, STAT3, signal transducer and activator of transcription 3, TNFα, tumor necrosis factor α, WEKA, Waikato Environment for Knowledge Analysis, docking, machine learning, multi-targeted drug discovery, screening of FDA-approved drugs
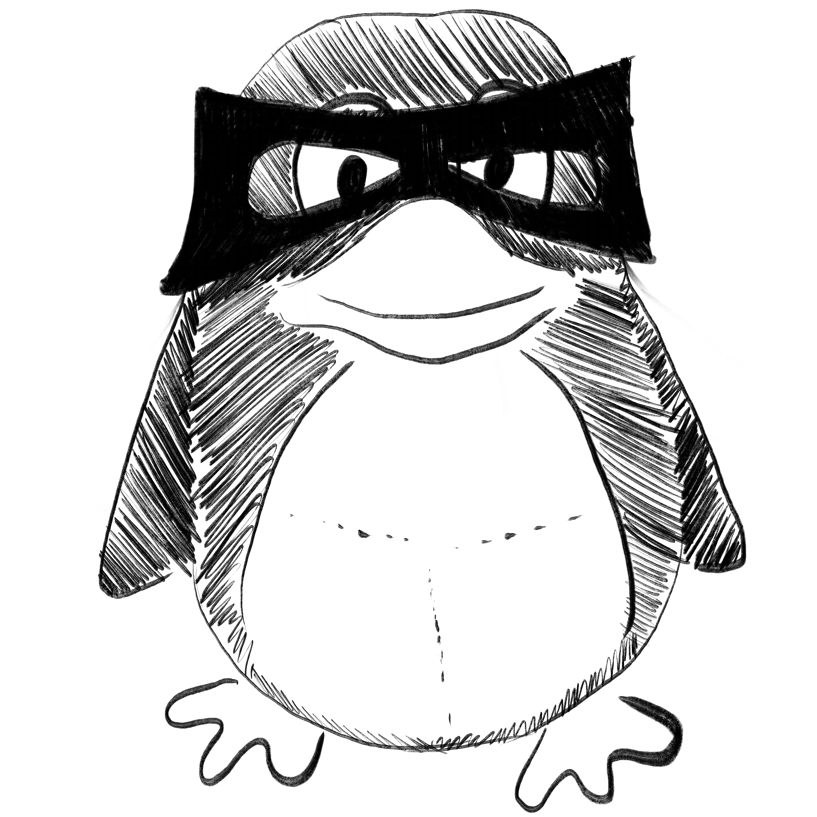
On the accuracy of Covid-19 forecasting methods in Russia for two years.
In Procedia computer science
Moloshnikov I A, Sboev A G, Naumov A V, Zavertyaev S V, Rybka R B
2022
SIR, covid-19 forecasting, machine learning, time series analysis, total cases prediction
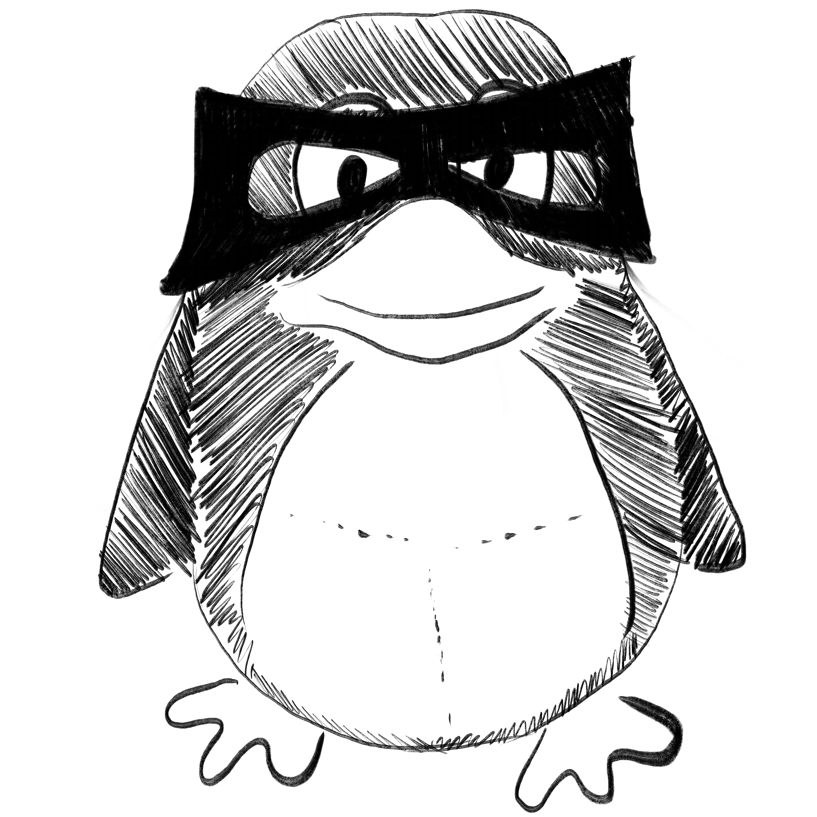
COVID-19 detection and classification for machine learning methods using human genomic data.
In Measurement. Sensors
Ahemad Mohd Thousif, Hameed Mohd Abdul, Vankdothu Ramdas
2022-Dec
Classification, Corona virus, Covid -19, Human Genomic data, Machine learning, Pneumonia, X-rays
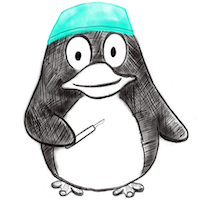
An artificial intelligence system to predict the optimal timing for mechanical ventilation weaning for intensive care unit patients: A two-stage prediction approach.
In Frontiers in medicine
BACKGROUND :
PURPOSE :
METHODS :
RESULTS :
CONCLUSION :
Liu Chung-Feng, Hung Chao-Ming, Ko Shian-Chin, Cheng Kuo-Chen, Chao Chien-Ming, Sung Mei-I, Hsing Shu-Chen, Wang Jhi-Joung, Chen Chia-Jung, Lai Chih-Cheng, Chen Chin-Ming, Chiu Chong-Chi
2022
artificial intelligence, intensive care unit, machine learning, optimal weaning timing, weaning mechanical ventilation
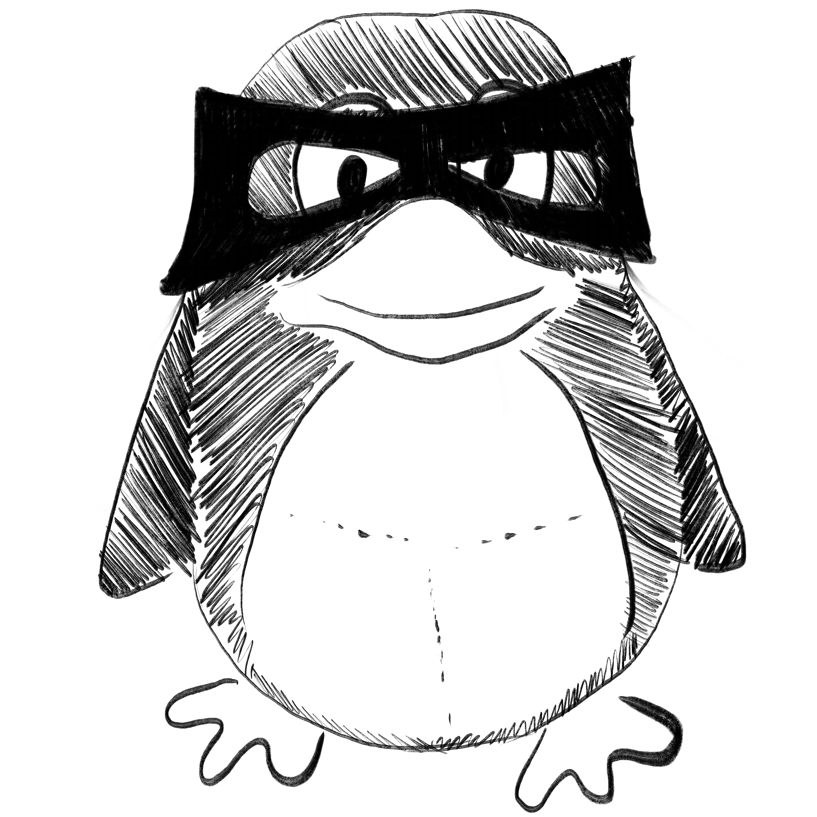
Are Twitter Sentiments During COVID-19 Pandemic a Critical Determinant to Predict Stock Market Movements? A Machine Learning Approach.
In Scientific African
Jena Pradyot Ranjan, Majhi Ritanjali
2022-Nov-29
COVID-19, LSTM, Sentiment Analysis, Stock Market, Twitter
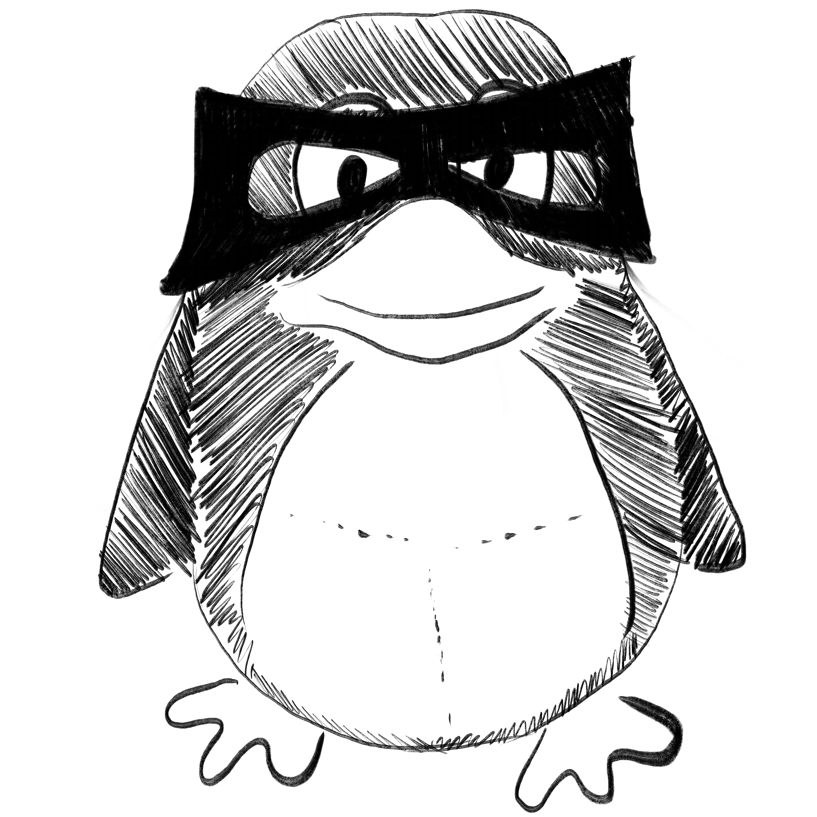
SODA: A Natural Language Processing Package to Extract Social Determinants of Health for Cancer Studies
ArXiv Preprint
Zehao Yu, Xi Yang, Chong Dang, Prakash Adekkanattu, Braja Gopal Patra, Yifan Peng, Jyotishman Pathak, Debbie L. Wilson, Ching-Yuan Chang, Wei-Hsuan Lo-Ciganic, Thomas J. George, William R. Hogan, Yi Guo, Jiang Bian, Yonghui Wu
2022-12-06
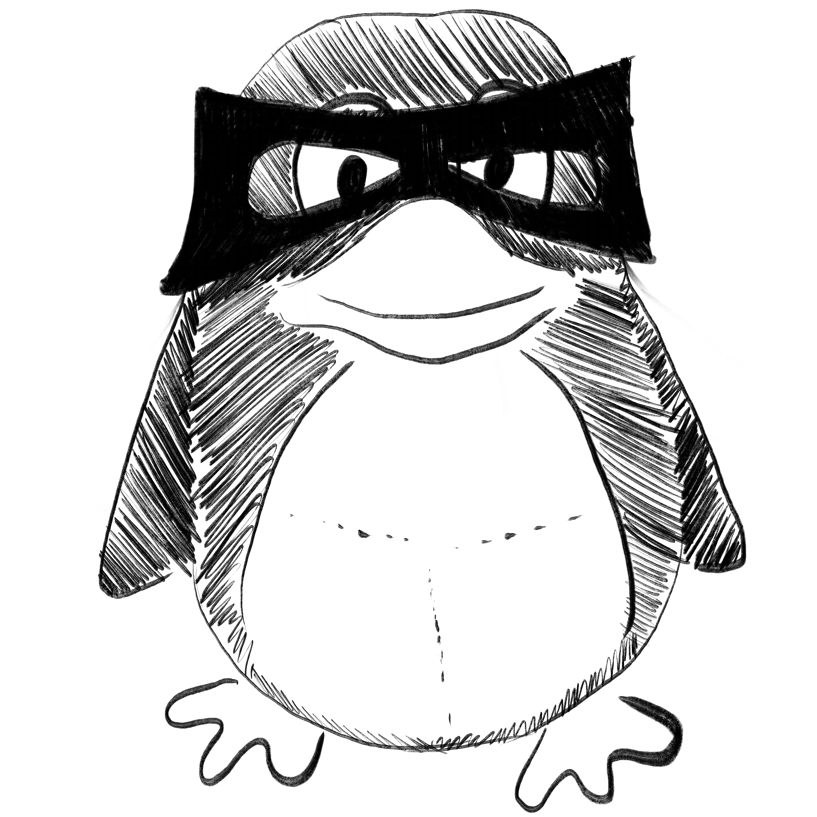
Predictive modeling and analysis of air quality - Visualizing before and during COVID-19 scenarios.
In Journal of environmental management
Persis Jinil, Ben Amar Amine
2022-Dec-01
Air pollution, Air quality index, COVID-19, Machine learning
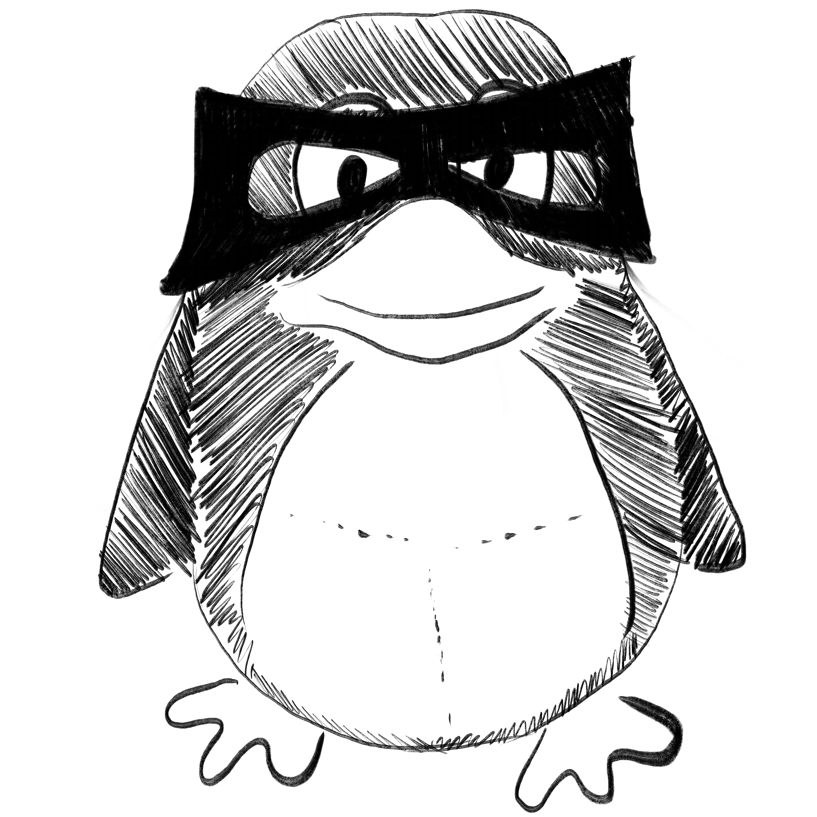
A novel intelligent radiomic analysis of perfusion SPECT/CT images to optimize pulmonary embolism diagnosis in COVID-19 patients.
In EJNMMI physics
BACKGROUND :
METHODS :
RESULTS :
CONCLUSION :
HIGHLIGHTS :
Baeza Sonia, Gil Debora, Garcia-Olivé Ignasi, Salcedo-Pujantell Maite, Deportós Jordi, Sanchez Carles, Torres Guillermo, Moragas Gloria, Rosell Antoni
2022-Dec-05
COVID-19, CT, Neural networks, Pulmonary embolism, Radiomics, SPECT
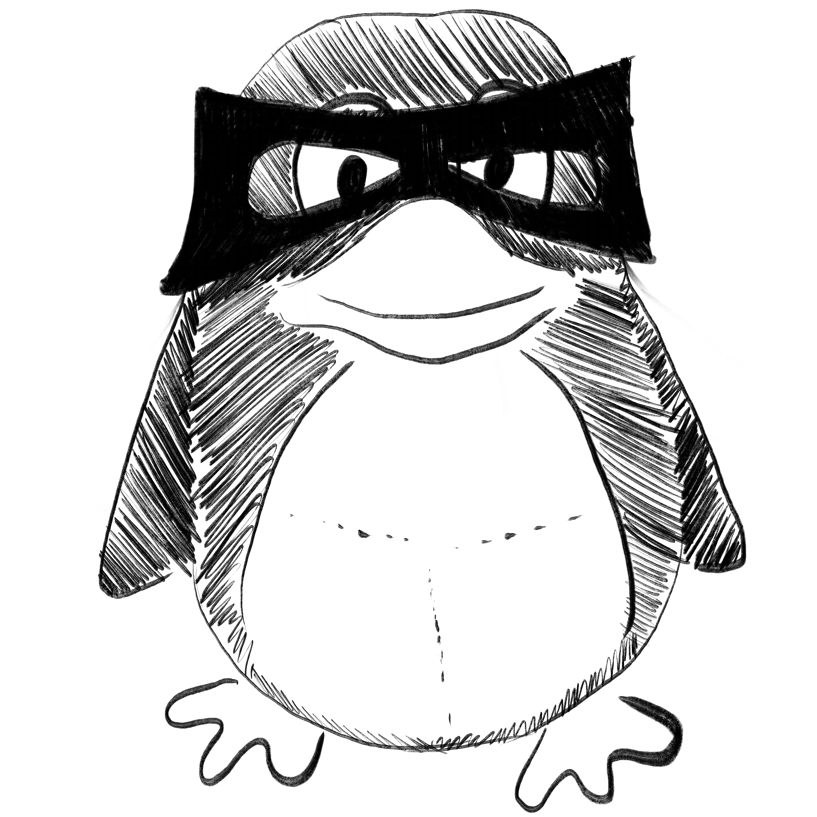
A Bayesian analysis based on multivariate stochastic volatility model: evidence from green stocks.
In Journal of combinatorial optimization
Ma Ming, Zhang Jing
2023
Bayesian analysis, Green stock, Machine learning, Markov chain Monte Carlo, Spillover Effect
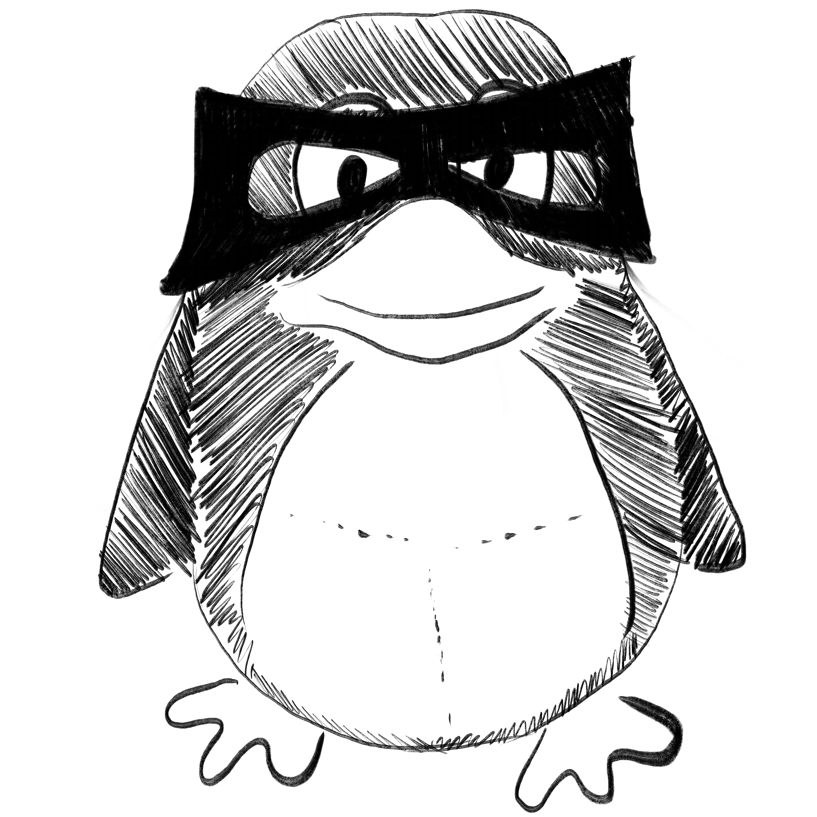
Automated Physical Distance Estimation and Crowd Monitoring Through Surveillance Video.
In SN computer science
Junayed Masum Shah, Islam Md Baharul
2023
COVID-19 social distancing, Crowd monitoring, Distance measurement, Human detection and tracking, Video surveillance
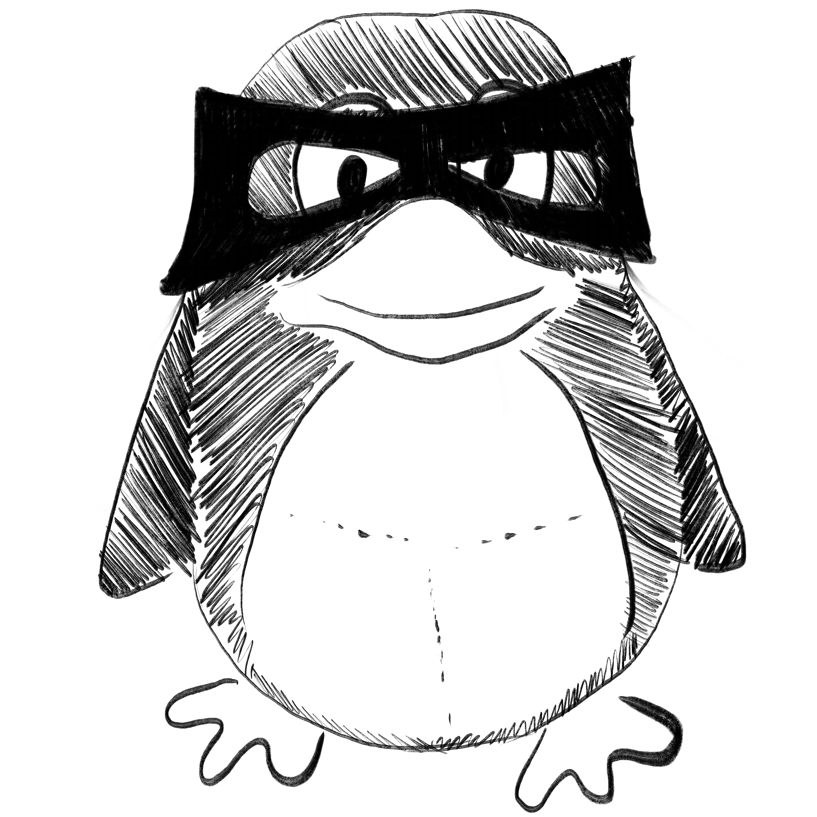
Application of Machine Learning and Deep Learning Techniques for COVID-19 Screening Using Radiological Imaging: A Comprehensive Review.
In SN computer science
Lasker Asifuzzaman, Obaidullah Sk Md, Chakraborty Chandan, Roy Kaushik
2023
COVID-19, CT, Deep learning, Machine learning, Radiological imaging, X-ray
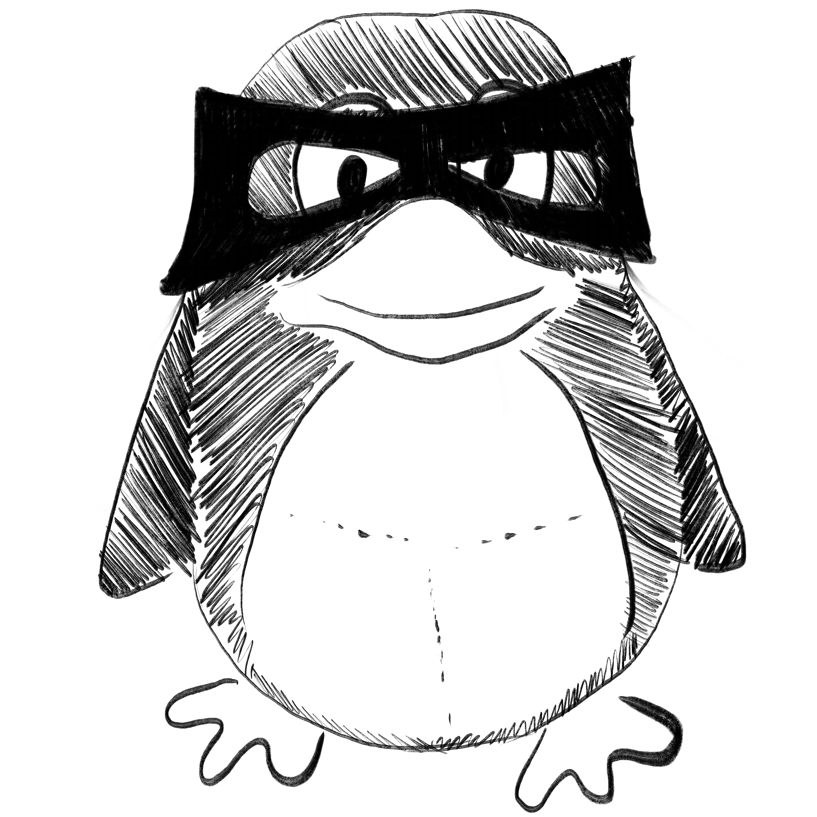
Human migration-based graph convolutional network for PM2.5 forecasting in post-COVID-19 pandemic age.
In Neural computing & applications
Zhan Choujun, Jiang Wei, Min Hu, Gao Ying, Tse C K
2022-Nov-22
Air pollution, COVID-19, Deep learning, Graph neural network
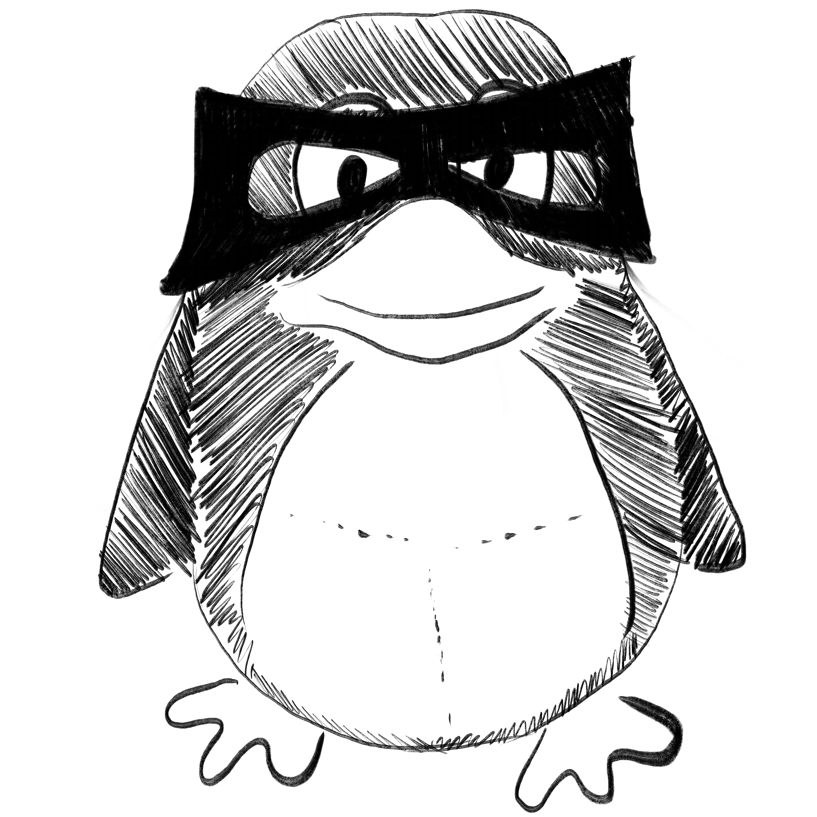
Multi-class classification of COVID-19 documents using machine learning algorithms.
In Journal of intelligent information systems
Rabby Gollam, Berka Petr
2022-Nov-29
COVID-19, Machine learning algorithms, Multi-class classification, Text mining
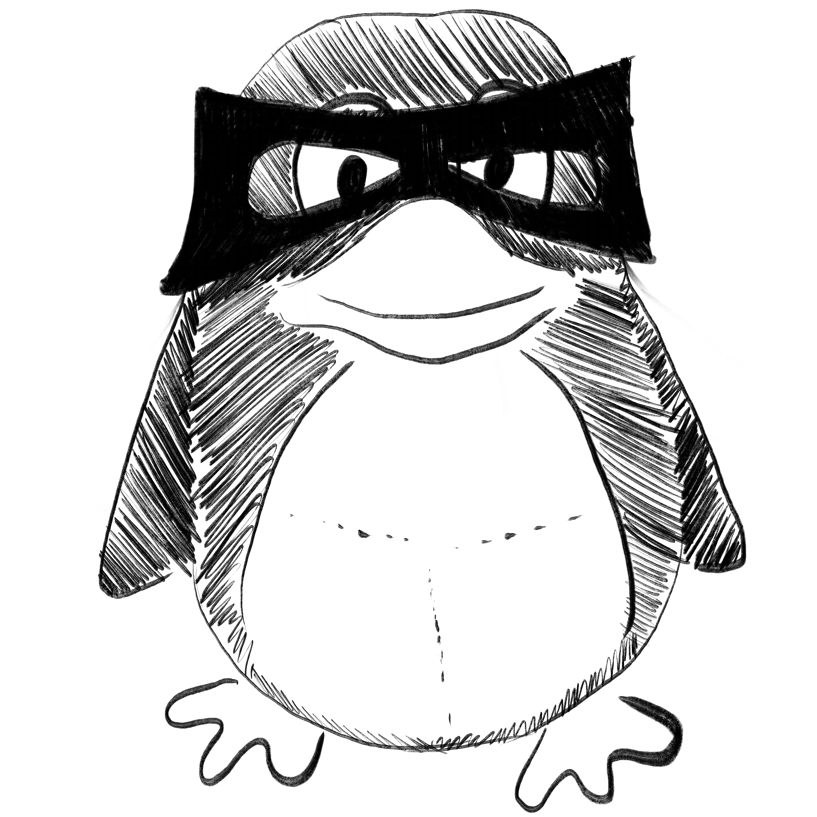
A Trustworthy Framework for Medical Image Analysis with Deep Learning
ArXiv Preprint
Kai Ma, Siyuan He, Pengcheng Xi, Ashkan Ebadi, Stéphane Tremblay, Alexander Wong
2022-12-06
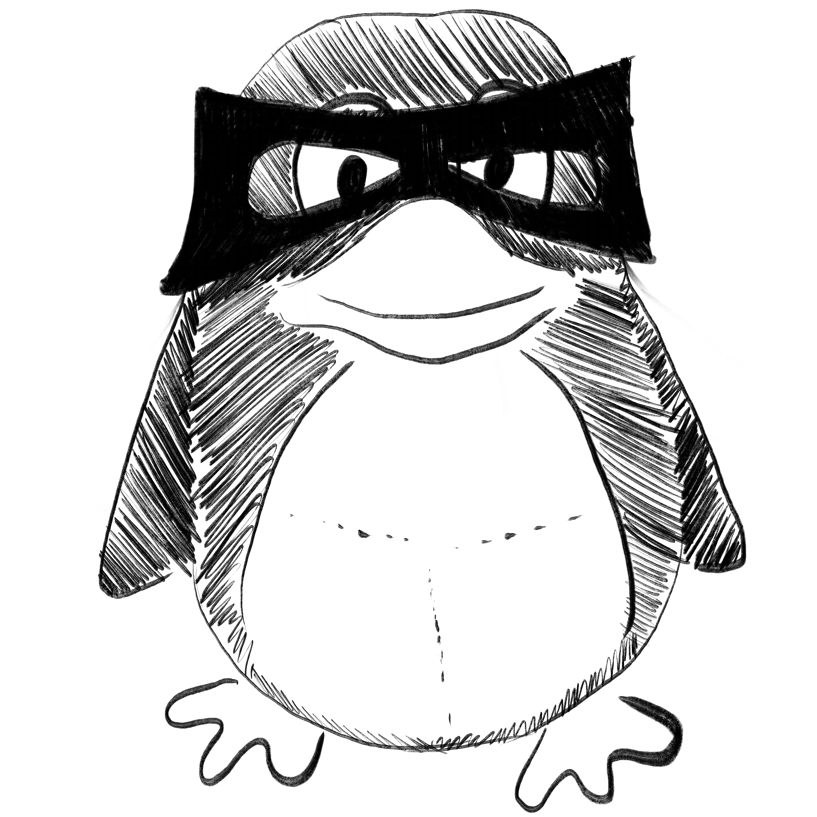
Differences between remote and analog design thinking through the lens of distributed cognition.
In Frontiers in artificial intelligence
Wolferts Daniel, Stein Elisabeth, Bernards Ann-Kathrin, Reiners René
2022
Design Thinking (DT), artificial intelligence (AI), distributed cognition for teamwork, human-computer interaction (HCI), remote work
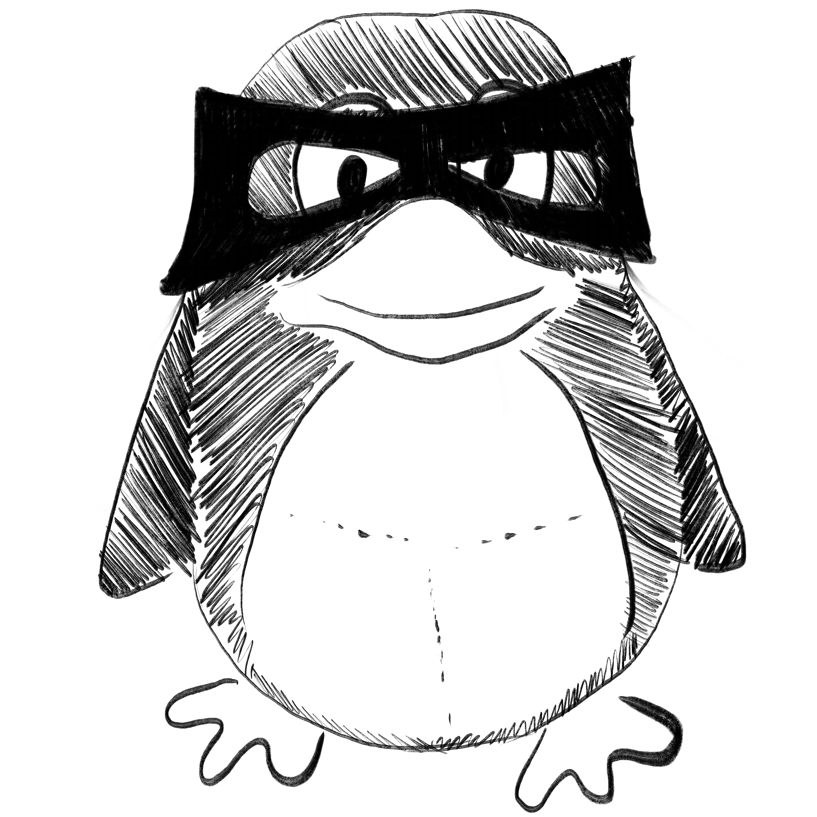
An efficient hybrid stock trend prediction system during COVID-19 pandemic based on stacked-LSTM and news sentiment analysis.
In Multimedia tools and applications
Sharaf Marwa, Hemdan Ezz El-Din, El-Sayed Ayman, El-Bahnasawy Nirmeen A
2022-Nov-28
COVID-19 pandemic, Machine learning, Prediction, Sentimental analysis, Stacked-LSTM, Stock market
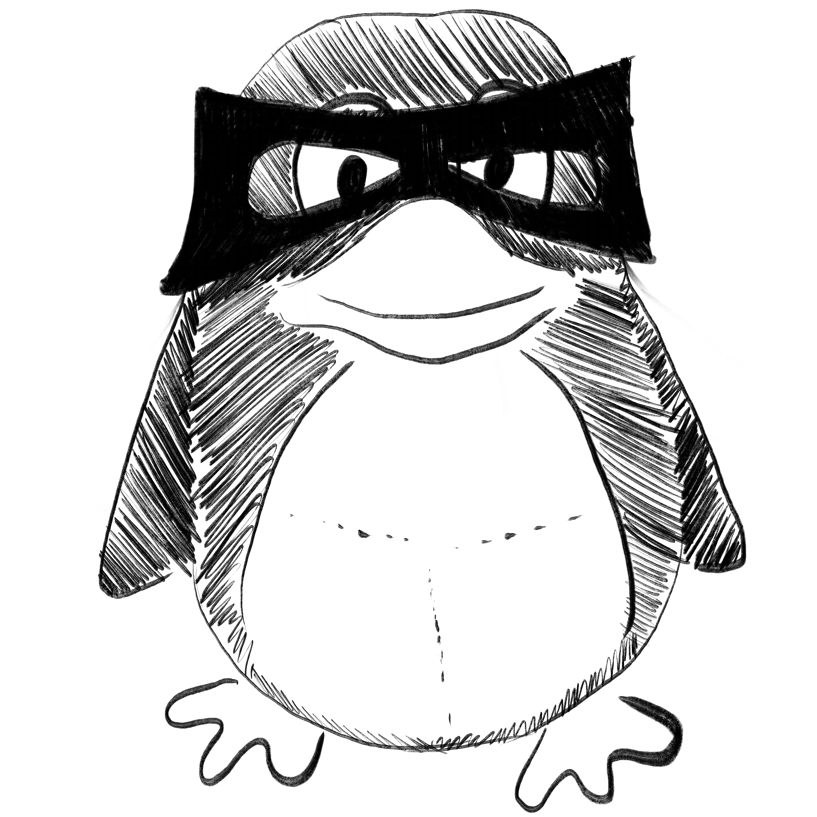
COVID-19 risk reduce based YOLOv4-P6-FaceMask detector and DeepSORT tracker.
In Multimedia tools and applications
Mokeddem Mohammed Lakhdar, Belahcene Mebarka, Bourennane Salah
2022-Nov-25
Deep learning, Detection, Localization, Scaled-YOLOv4, Tracking
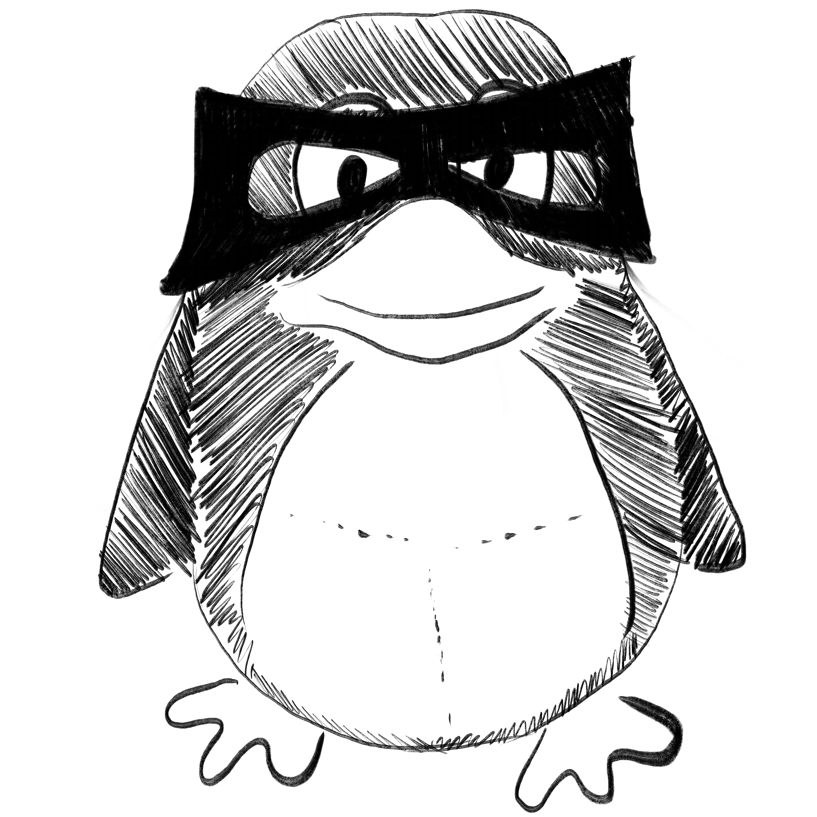
A data-driven spatially-specific vaccine allocation framework for COVID-19.
In Annals of operations research
Hong Zhaofu, Li Yingjie, Gong Yeming, Chen Wanying
2022-Nov-22
COVID-19, Data-driven decision making, Deep learning, Spatially-specific SEIR model, Vaccine allocation
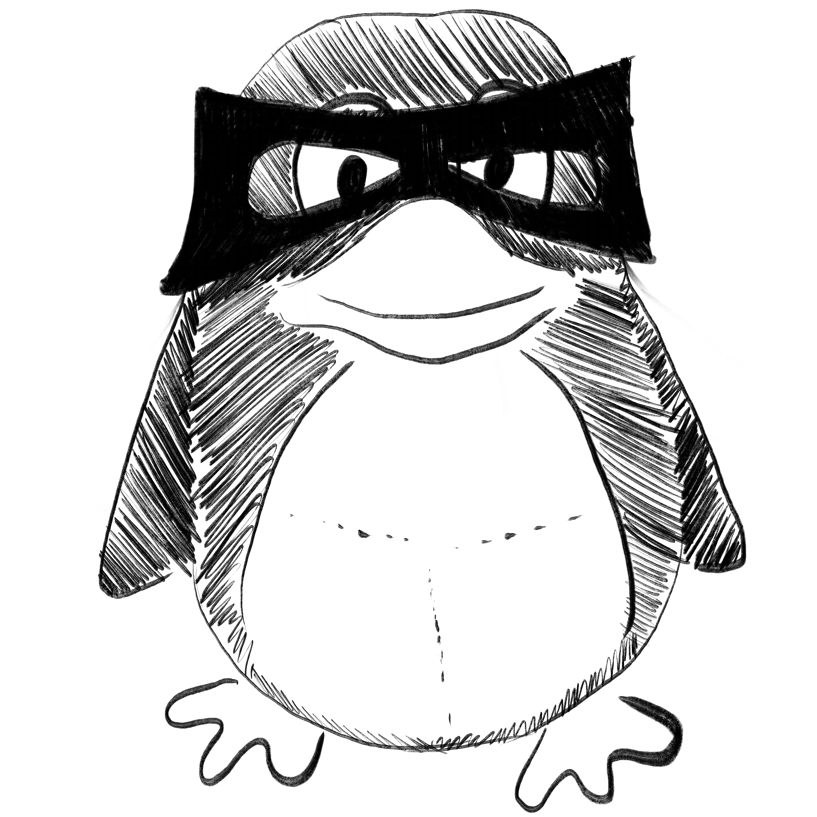
Correction to: The role of cryptocurrencies in predicting oil prices pre and during COVID-19 pandemic using machine learning.
In Annals of operations research
Elamer Ahmed A, Abdou Hussein A, Ibrahim Bassam A
2022-Nov-21
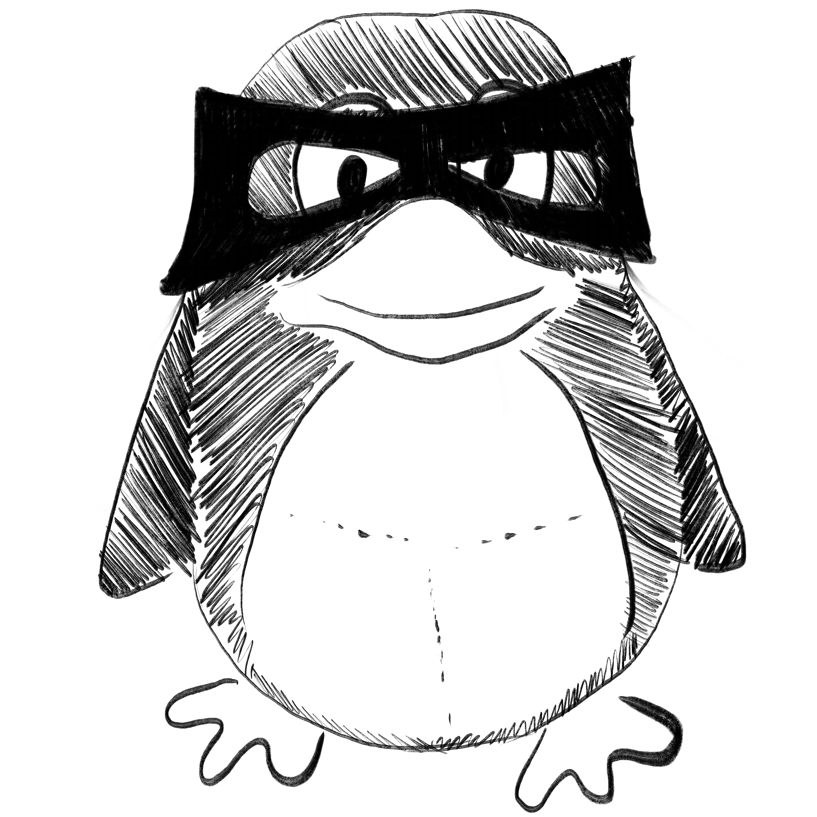
The labor market in the digital era: What matters for the Gulf Cooperation Council countries?
In Frontiers in sociology
Bousrih Jihen, Elhaj Manal, Hassan Fatma
2022
GCC countries, advanced countries, digitalization, employment rate, information and communication technologies
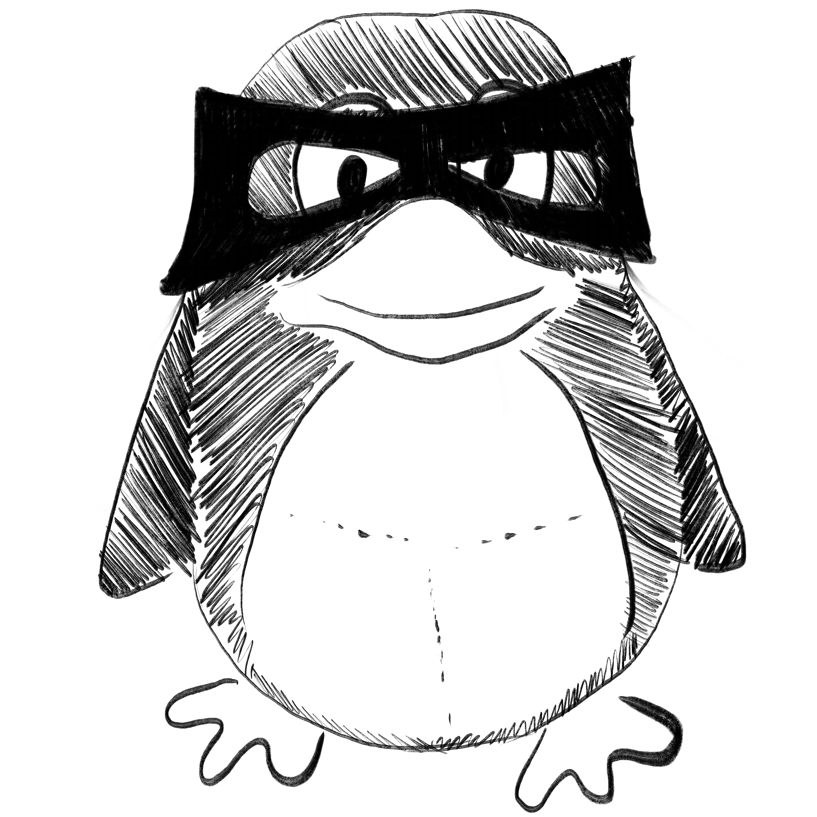
Retraction Note to: Accurate computation: COVID-19 rRT-PCR positive test dataset using stages classification through textual big data mining with machine learning.
In The Journal of supercomputing
Ramanathan Shalini, Ramasundaram Mohan
2022-Nov-21
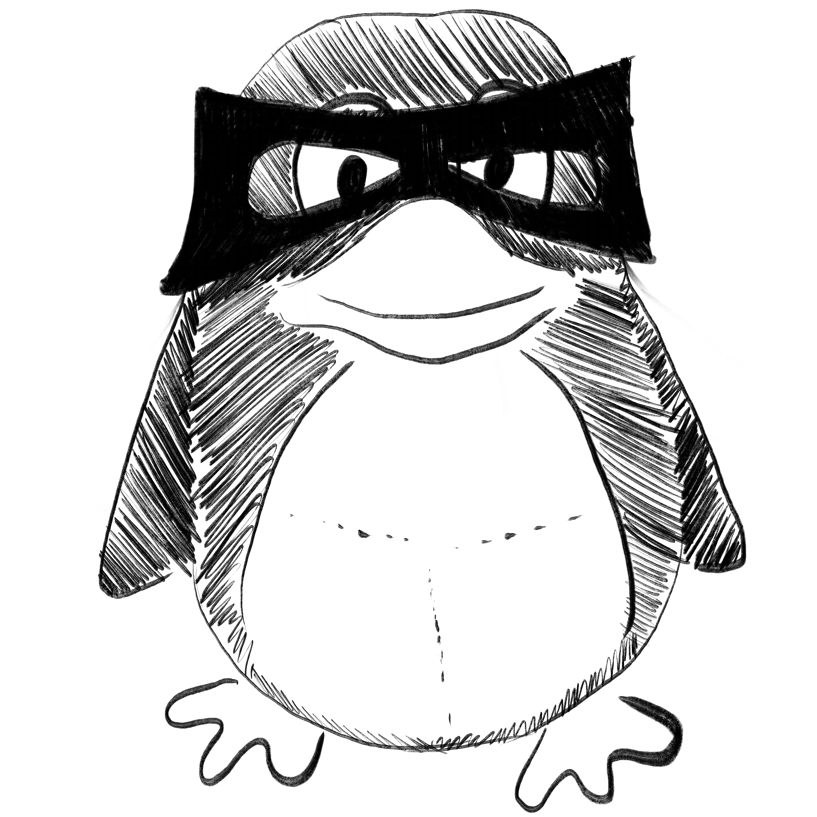
PulDi-COVID: Chronic Obstructive Pulmonary (Lung) Diseases With COVID-19 Classification Using Ensemble Deep Convolutional Neural Network From Chest X-Ray Images To Minimize Severity And Mortality Rates.
In Biomedical signal processing and control
BACKGROUND :
METHODS :
RESULTS :
CONCLUSION :
Bhosale Yogesh H, Sridhar Patnaik K
2022-Nov-30
Biomedical engineering, COVID-19, Chronic Pulmonary disease, Convolution neural networks (CNN), Diagnosis & Classification, Ensemble deep learning, Transfer learning
Identification of hospitalized mortality of patients with COVID-19 by machine learning models based on blood inflammatory cytokines.
In Frontiers in public health
Yu Zhixiang, Li Xiayin, Zhao Jin, Sun Shiren
2022
COVID-19, inflammatory cytokines, machine learning, outcome, prognostic models
COVID-19 outbreaks analysis in the Valencian Region of Spain in the prelude of the third wave.
In Frontiers in public health
INTRODUCTION :
METHODS :
RESULTS :
DISCUSSION :
Fuente David, Hervás David, Rebollo Miguel, Conejero J Alberto, Oliver Nuria
2022
Bayesian statistical model, COVID-19, SARS-CoV-2, biomedical data science, cluster, epidemiological analysis, outbreak modeling
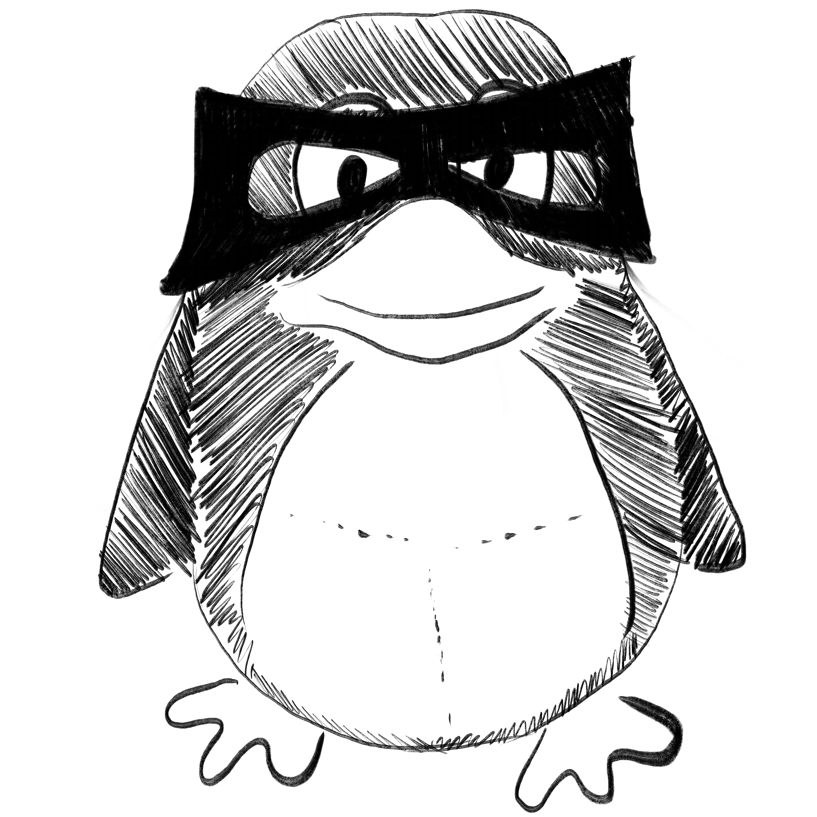
Bootstrapping random forest and CHAID for prediction of white spot disease among shrimp farmers.
In Scientific reports ; h5-index 158.0
Edeh Michael Onyema, Dalal Surjeet, Obagbuwa Ibidun Christiana, Prasad B V V Siva, Ninoria Shalini Zanzote, Wajid Mohd Anas, Adesina Ademola Olusola
2022-Dec-03
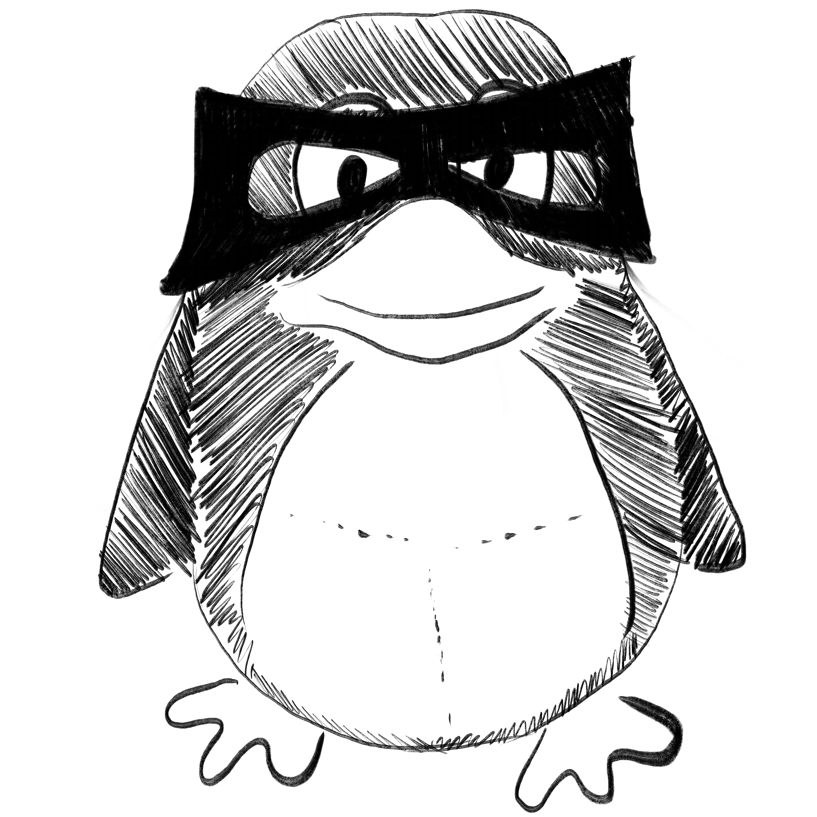
COVID-DSNet: A novel deep convolutional neural network for detection of coronavirus (SARS-CoV-2) cases from CT and Chest X-Ray images.
In Artificial intelligence in medicine ; h5-index 34.0
Reis Hatice Catal, Turk Veysel
2022-Dec
COVID-DSNet, Chest CT-scan images, Chest X-ray images, Depthwise separable convolution, SARS-CoV-2
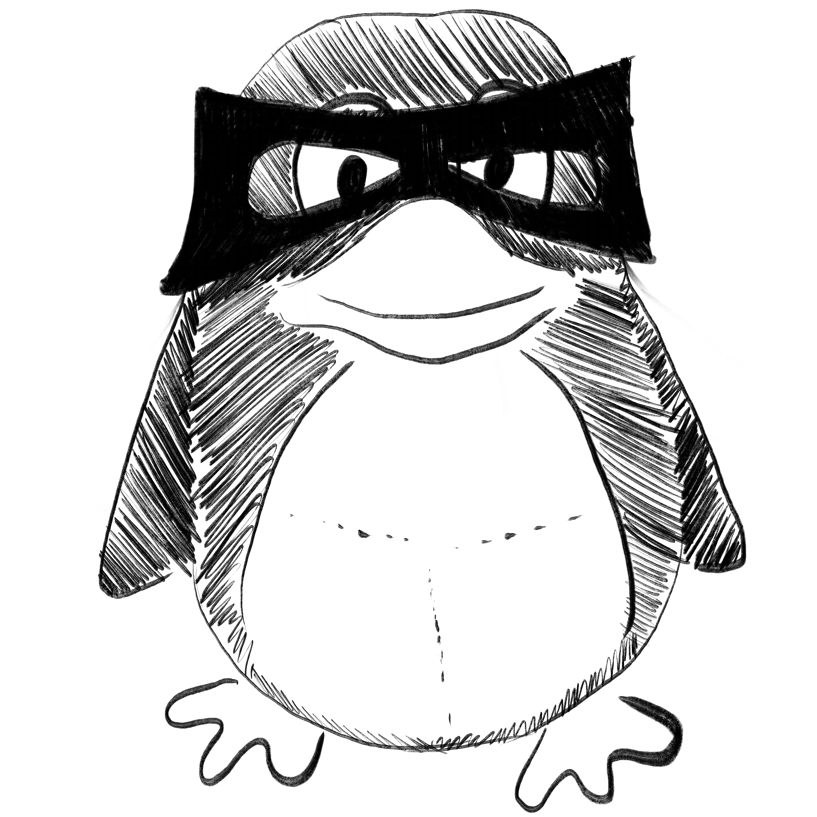
Deep variational graph autoencoders for novel host-directed therapy options against COVID-19.
In Artificial intelligence in medicine ; h5-index 34.0
Ray Sumanta, Lall Snehalika, Mukhopadhyay Anirban, Bandyopadhyay Sanghamitra, Schönhuth Alexander
2022-Dec
COVID-19, Host directed therapy, Molecular interaction network, Node2Vec, Variational graph autoEncoder
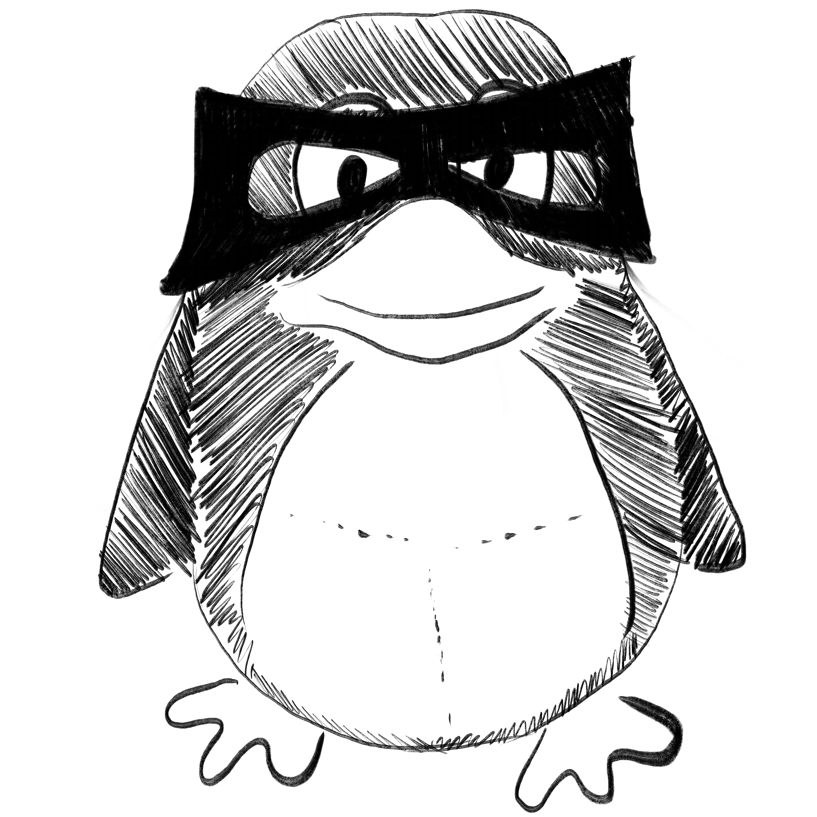
Ubiquitous and smart healthcare monitoring frameworks based on machine learning: A comprehensive review.
In Artificial intelligence in medicine ; h5-index 34.0
Motwani Anand, Shukla Piyush Kumar, Pawar Mahesh
2022-Dec
Big data, Chronic diseases, Cloud computing, Cognitive computing, Data analytics, Edge computing, Internet-of-things, Machine learning, Remote patient monitoring, Smart healthcare monitoring, Ubiquitous computing
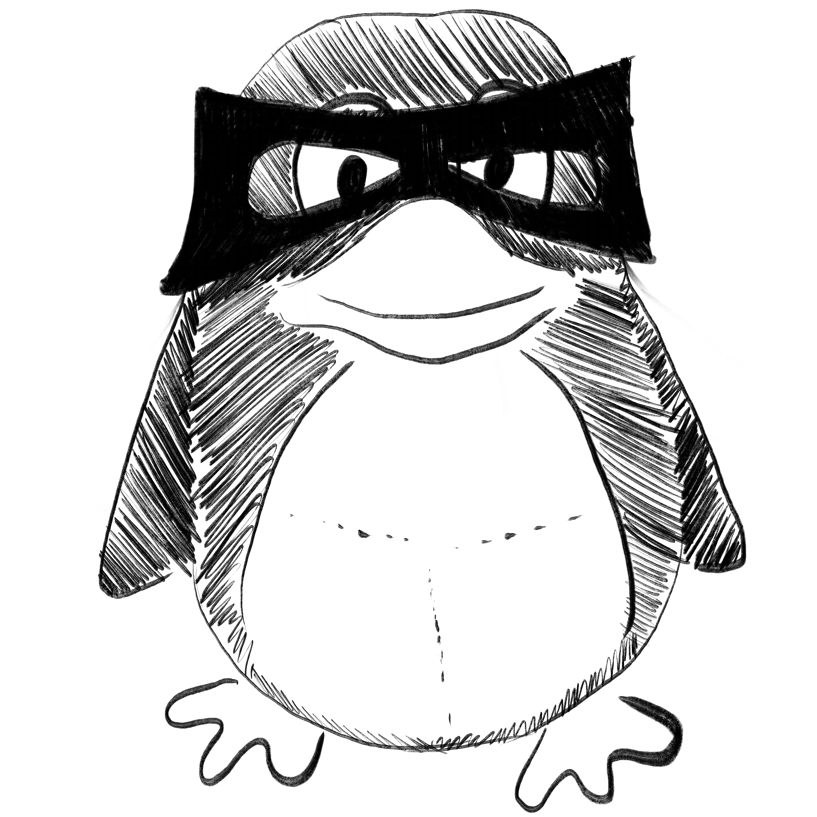
Predicting depression and anxiety of Chinese population during COVID-19 in psychological evaluation data by XGBoost.
In Journal of affective disorders ; h5-index 79.0
BACKGROUND :
METHODS :
RESULTS :
CONCLUSIONS :
Tian Zhanxiao, Qu Wei, Zhao Yanli, Zhu Xiaolin, Wang Zhiren, Tan Yunlong, Jiang Ronghuan, Tan Shuping
2022-Nov-30
Anxiety, COVID-19 pandemic, Depression, Machine learning, Resilience, Social support
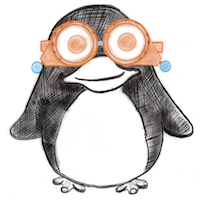
Transforming ophthalmology in the digital century-new care models with added value for patients.
In Eye (London, England) ; h5-index 41.0
Faes Livia, Maloca Peter M, Hatz Katja, Wolfensberger Thomas J, Munk Marion R, Sim Dawn A, Bachmann Lucas M, Schmid Martin K
2022-Dec-03
Classification and visual explanation for COVID-19 pneumonia from CT images using triple learning.
In Scientific reports ; h5-index 158.0
Kato Sota, Oda Masahiro, Mori Kensaku, Shimizu Akinobu, Otake Yoshito, Hashimoto Masahiro, Akashi Toshiaki, Hotta Kazuhiro
2022-Dec-02
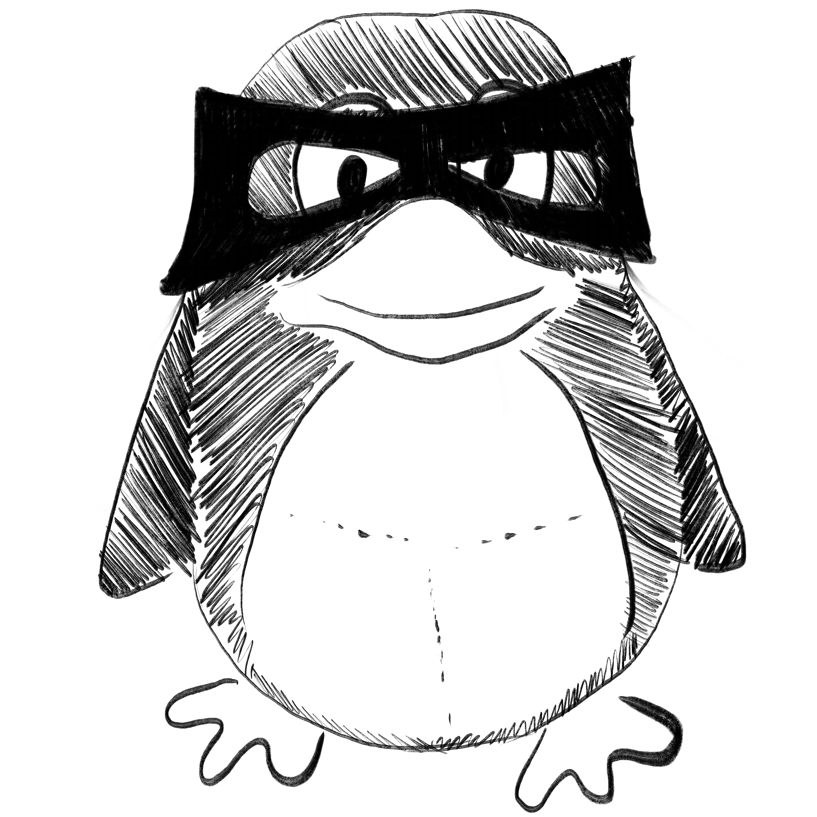
A lightweight network for COVID-19 detection in X-ray images.
In Methods (San Diego, Calif.)
Shi Yong, Tang Anda, Xiao Yang, Niu Lingfeng
2022-Nov-29
COVID-19 detection, network pruning, neural network
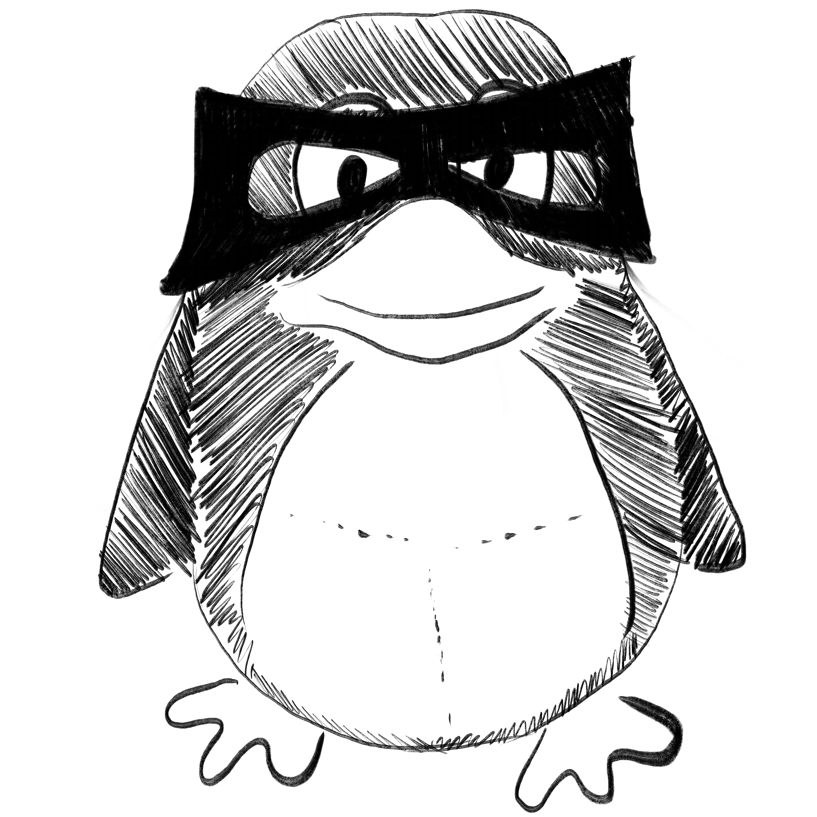
Res-SE-ConvNet: A Deep Neural Network for Hypoxemia Severity Prediction for Hospital In-Patients Using Photoplethysmograph Signal.
In IEEE journal of translational engineering in health and medicine
Mahmud Talha Ibn, Imran Sheikh Asif, Shahnaz Celia
2022
Saturated oxygen, attention, deep learning, excitation, feature map
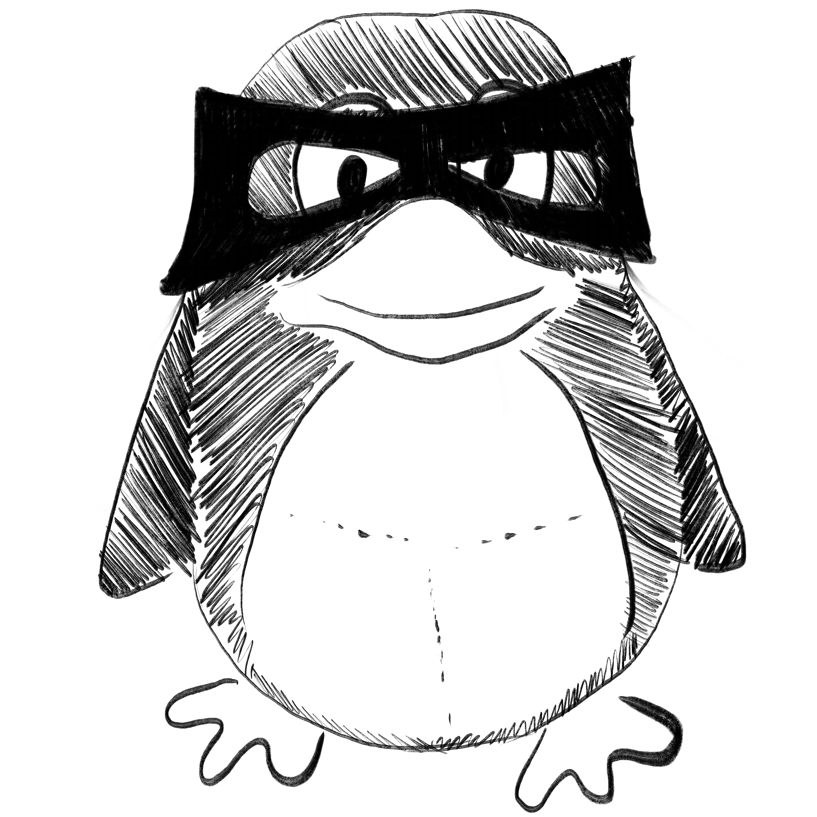
Analysis of the effect of an artificial intelligence chatbot educational program on non-face-to-face classes: a quasi-experimental study.
In BMC medical education
BACKGROUND :
OBJECTIVES :
DESIGN :
METHODS :
RESULTS :
CONCLUSION :
Han Jeong-Won, Park Junhee, Lee Hanna
2022-Dec-01
Artificial intelligence, Chatbot program, Clinical reasoning, Data processing, Education, Nursing
Data-driven identification of post-acute SARS-CoV-2 infection subphenotypes.
In Nature medicine ; h5-index 170.0
Zhang Hao, Zang Chengxi, Xu Zhenxing, Zhang Yongkang, Xu Jie, Bian Jiang, Morozyuk Dmitry, Khullar Dhruv, Zhang Yiye, Nordvig Anna S, Schenck Edward J, Shenkman Elizabeth A, Rothman Russell L, Block Jason P, Lyman Kristin, Weiner Mark G, Carton Thomas W, Wang Fei, Kaushal Rainu
2022-Dec-01
Modeling approaches for early warning and monitoring of pandemic situations as well as decision support.
In Frontiers in public health
Botz Jonas, Wang Danqi, Lambert Nicolas, Wagner Nicolas, Génin Marie, Thommes Edward, Madan Sumit, Coudeville Laurent, Fröhlich Holger
2022
agent-based-modeling, artificial intelligence, compartmental models, machine learning, pandemic
Symptom diaries as a digital tool to detect SARS-CoV-2 infections and differentiate between prevalent variants.
In Frontiers in public health
Grüne Barbara, Kugler Sabine, Ginzel Sebastian, Wolff Anna, Buess Michael, Kossow Annelene, Küfer-Weiß Annika, Rüping Stefan, Neuhann Florian
2022
SARS-CoV-2, classification, digital symptom diaries, health department, machine learning, prevalent virus variants, symptom combinations
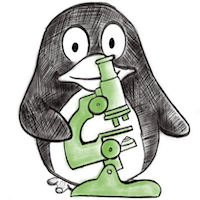
Computational approaches for network-based integrative multi-omics analysis.
In Frontiers in molecular biosciences
Agamah Francis E, Bayjanov Jumamurat R, Niehues Anna, Njoku Kelechi F, Skelton Michelle, Mazandu Gaston K, Ederveen Thomas H A, Mulder Nicola, Chimusa Emile R, ‘t Hoen Peter A C
2022
data integration, machine learning, multi-modal network, multi-omics, network causal inference, network diffusion/propagation
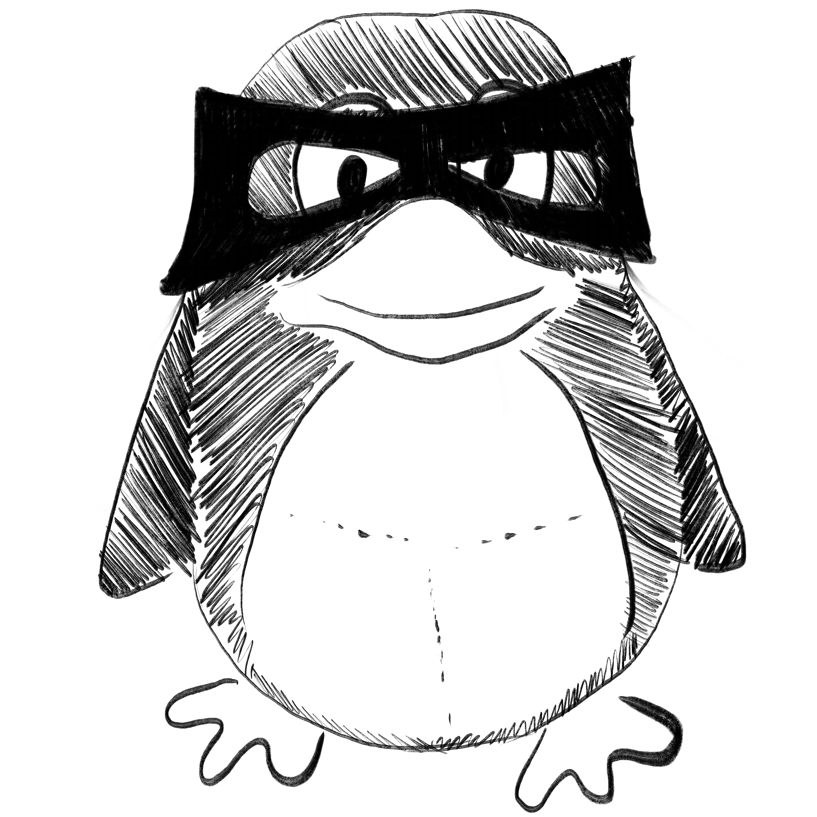
Radiomorphological signs and clinical severity of SARS-CoV-2 lineage B.1.1.7.
In BJR open
OBJECTIVE :
METHODS :
RESULTS :
CONCLUSION :
ADVANCES IN KNOWLEDGE :
Simon Judit, Grodecki Kajetan, Cadet Sebastian, Killekar Aditya, Slomka Piotr, Zara Samuel James, Zsarnóczay Emese, Nardocci Chiara, Nagy Norbert, Kristóf Katalin, Vásárhelyi Barna, Müller Veronika, Merkely Béla, Dey Damini, Maurovich-Horvat Pál
2022
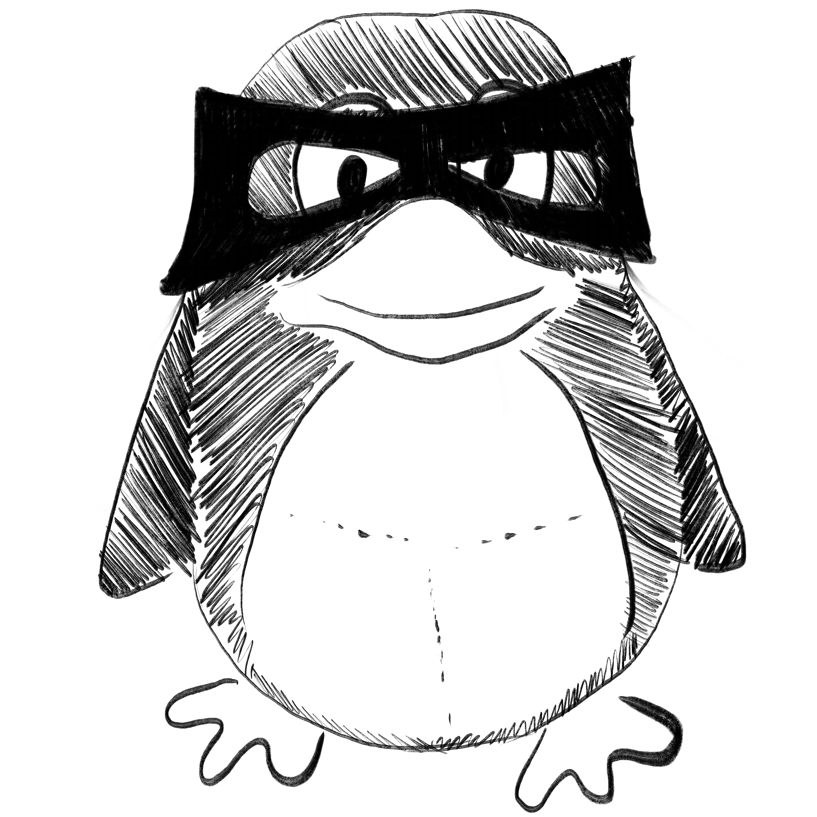
Next-generation proteomics of serum extracellular vesicles combined with single-cell RNA sequencing identifies MACROH2A1 associated with refractory COVID-19.
In Inflammation and regeneration
BACKGROUND :
METHODS :
RESULTS :
CONCLUSIONS :
Kawasaki Takahiro, Takeda Yoshito, Edahiro Ryuya, Shirai Yuya, Nogami-Itoh Mari, Matsuki Takanori, Kida Hiroshi, Enomoto Takatoshi, Hara Reina, Noda Yoshimi, Adachi Yuichi, Niitsu Takayuki, Amiya Saori, Yamaguchi Yuta, Murakami Teruaki, Kato Yasuhiro, Morita Takayoshi, Yoshimura Hanako, Yamamoto Makoto, Nakatsubo Daisuke, Miyake Kotaro, Shiroyama Takayuki, Hirata Haruhiko, Adachi Jun, Okada Yukinori, Kumanogoh Atsushi
2022-Nov-30
COVID-19, Exosome, Liquid biopsy, MACROH2A1, Multi-omics, SARS-CoV-2
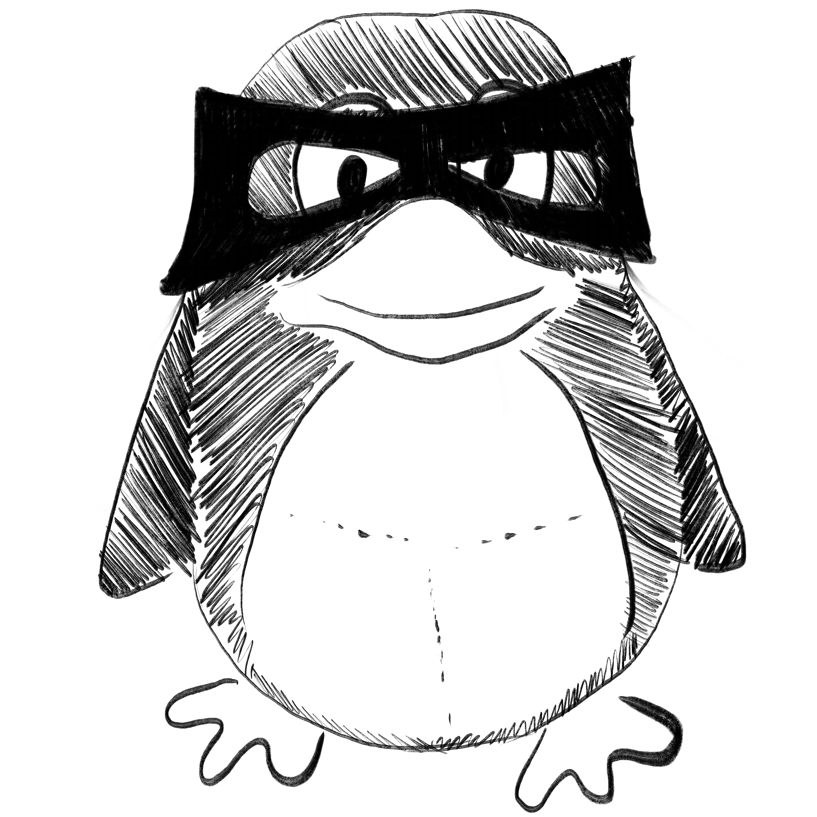
Predicting Immune Escape with Pretrained Protein Language Model Embeddings
bioRxiv Preprint
Swanson, K.; Chang, H.; Zou, J.
2022-12-02
Application of deep learning-based diagnostic systems in screening asymptomatic COVID-19 patients among oversea returnees.
In Journal of infection in developing countries
INTRODUCTION :
METHODOLOGY :
RESULTS :
CONCLUSIONS :
ADVANCES IN KNOWLEDGE :
Dong Dawei, Luo Zujin, Zheng Yue, Liang Ying, Zhao Pengfei, Feng Linlin, Wang Dawei, Cao Ying, Zhao Zhenhao, Ma Yingmin
2022-Nov-29
COVID-19, Deep learning, asymptomatic cases, diagnostic systems, performance evaluation
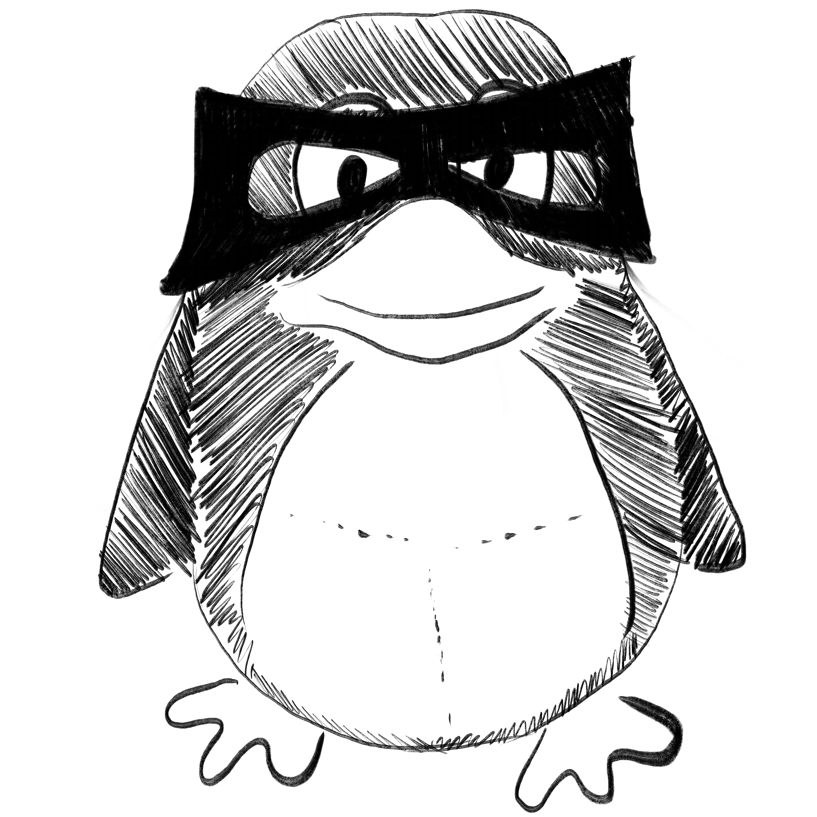
Multigroup prediction in lung cancer patients and comparative controls using signature of volatile organic compounds in breath samples.
In PloS one ; h5-index 176.0
Rai Shesh N, Das Samarendra, Pan Jianmin, Mishra Dwijesh C, Fu Xiao-An
2022
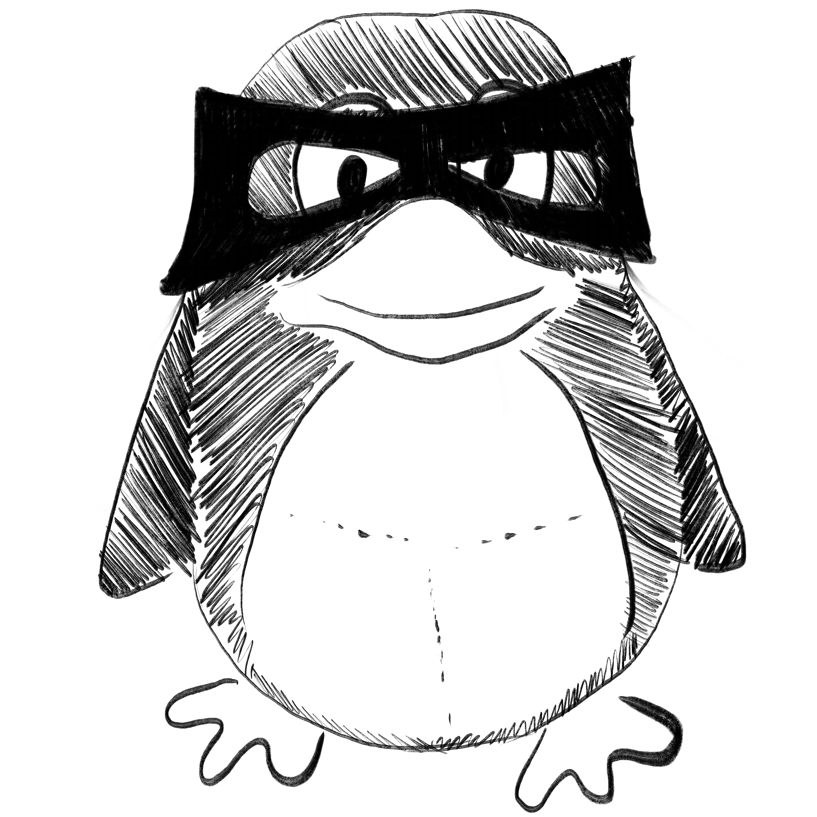
Highly Adsorptive Au-TiO2 Nanocomposites for the SERS Face Mask Allow the Machine-Learning-Based Quantitative Assay of SARS-CoV-2 in Artificial Breath Aerosols.
In ACS applied materials & interfaces ; h5-index 147.0
Hwang Charles S H, Lee Sangyeon, Lee Sejin, Kim Hanjin, Kang Taejoon, Lee Doheon, Jeong Ki-Hun
2022-Nov-30
SARS-CoV-2, breath biopsy, machine-learning, nanocomposite, plasmonics, surface-enhanced Raman spectroscopy
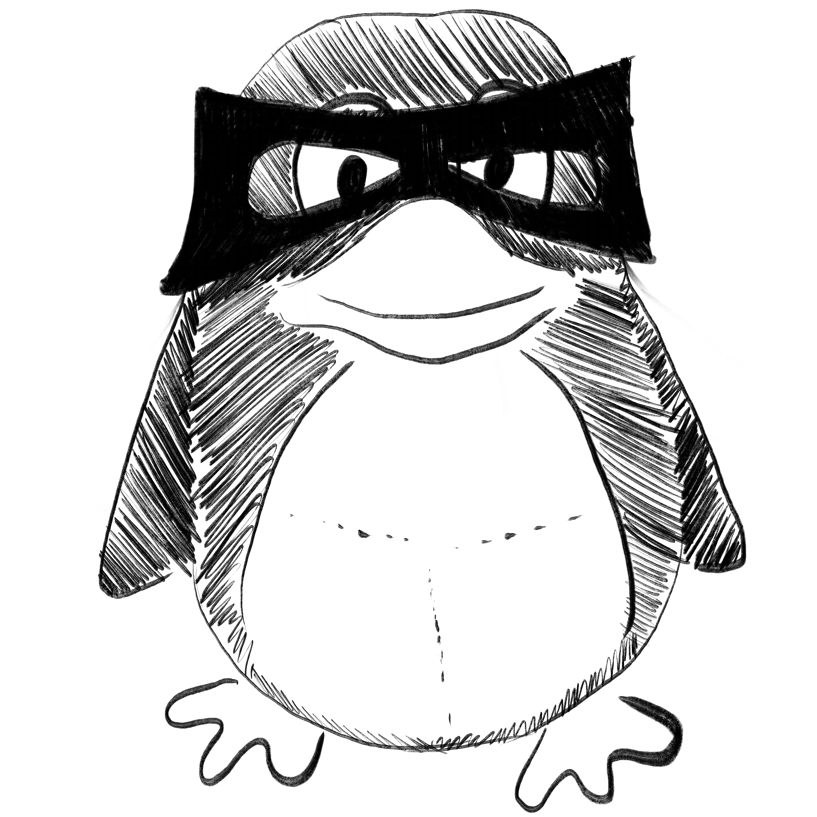
Emerging 0D, 1D, 2D, and 3D nanostructures for efficient point-of-care biosensing.
In Biosensors & bioelectronics: X
Byakodi Manisha, Shrikrishna Narlawar Sagar, Sharma Riya, Bhansali Shekhar, Mishra Yogendra, Kaushik Ajeet, Gandhi Sonu
2022-Nov-25
0D to 3D nanomaterials, Biosensors, Efficient diagnostics, Personalized health management, Point-of-care testing, Wearable
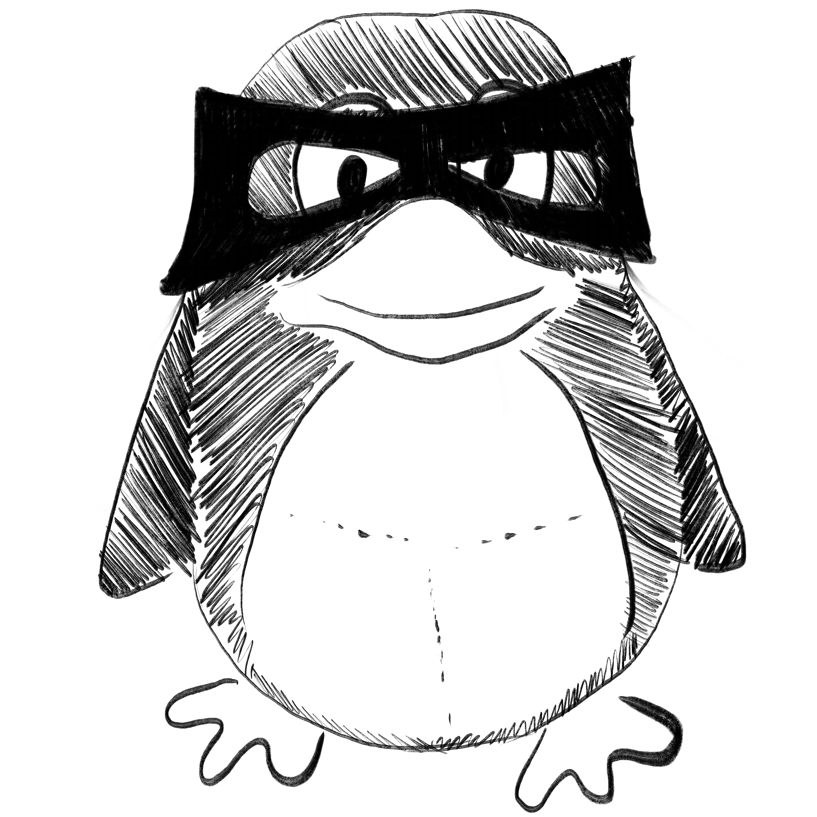
Automatic detection of Covid-19 from chest X-ray and lung computed tomography images using deep neural networks and transfer learning.
In Applied soft computing
Duong Linh T, Nguyen Phuong T, Iovino Ludovico, Flammini Michele
2022-Nov-24
AI Diagnosis systems, COVID-19, Chest X-ray image, Expert systems, Lung CT images
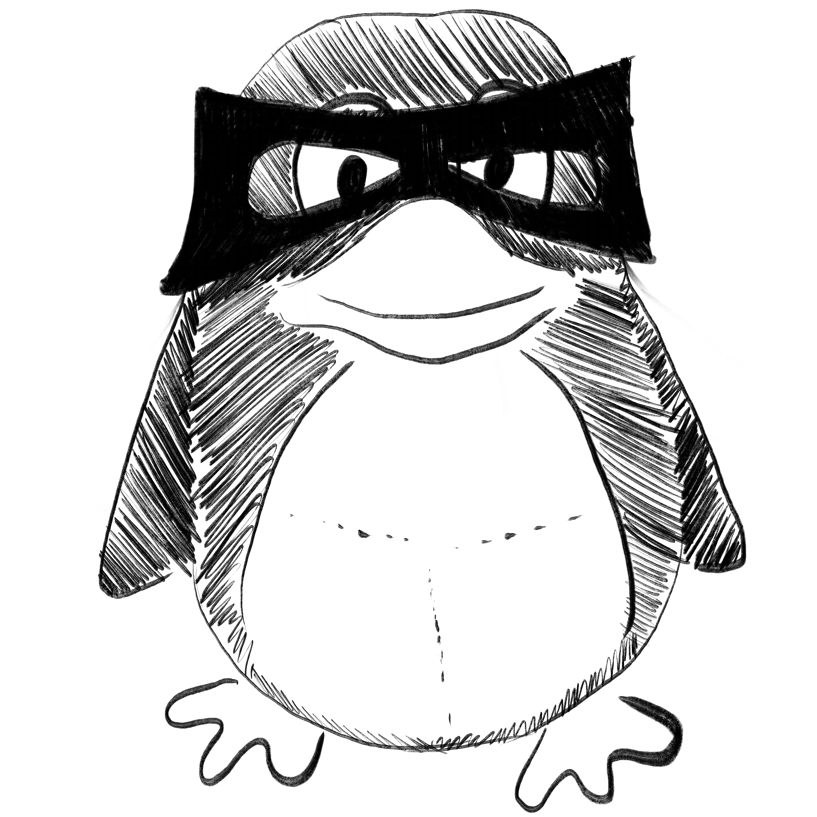
Contextual counters and multimodal Deep Learning for activity-level traffic classification of mobile communication apps during COVID-19 pandemic.
In Computer networks
Guarino Idio, Aceto Giuseppe, Ciuonzo Domenico, Montieri Antonio, Persico Valerio, Pescapè Antonio
2022-Dec-24
COVID-19, Collaboration apps, Communication apps, Contextual counters, Deep Learning, Encrypted traffic, Multimodal techniques, Traffic classification
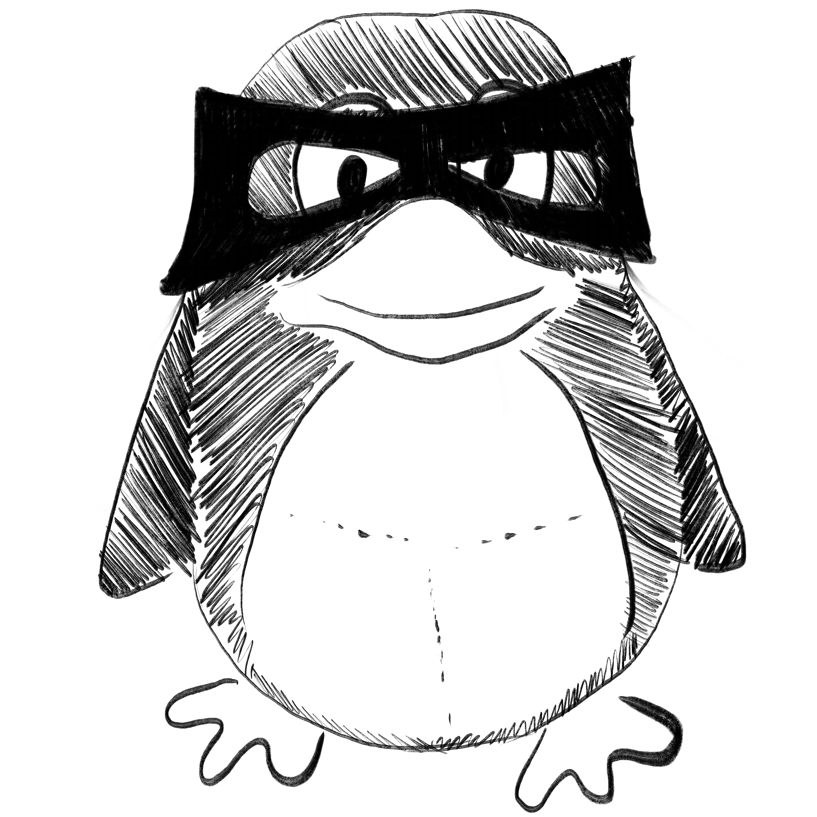
Supervised Machine Learning Approach to COVID-19 Detection Based on Clinical Data.
In Medical journal of the Islamic Republic of Iran
Yazdani Azita, Zahmatkeshan Maryam, Ravangard Ramin, Sharifian Roxana, Shirdeli Mohammad
2022
Artificial Intelligence, COVID-19, Classification, Data mining, Machine Learning
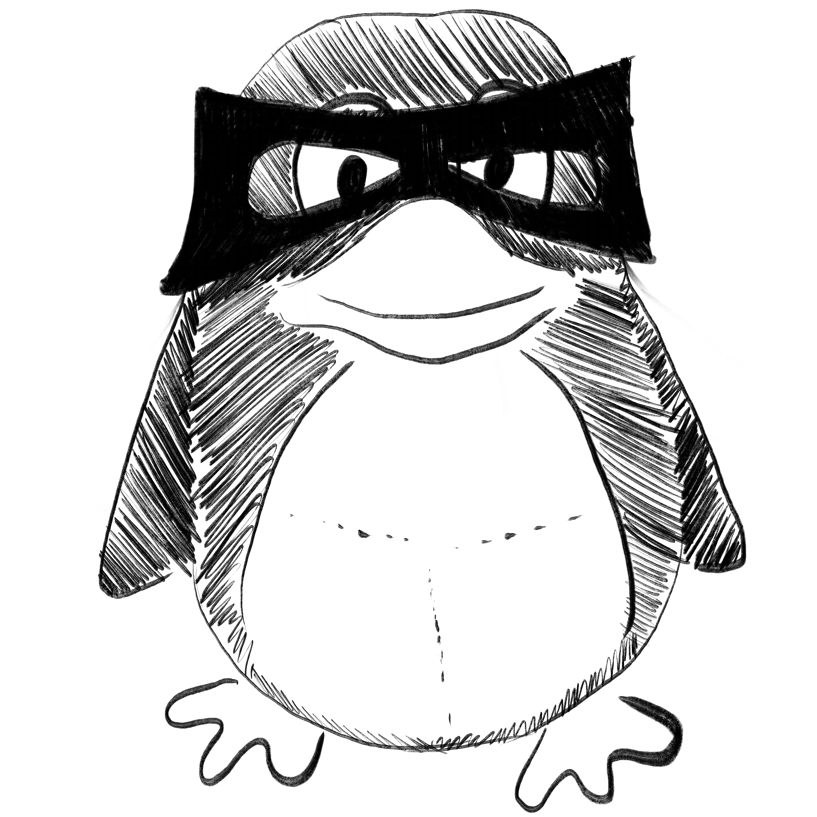
Disease-related compound identification based on deeping learning method.
In Scientific reports ; h5-index 158.0
Yang Bin, Bao Wenzheng, Wang Jinglong, Chen Baitong, Iwamori Naoki, Chen Jiazi, Chen Yuehui
2022-Nov-29
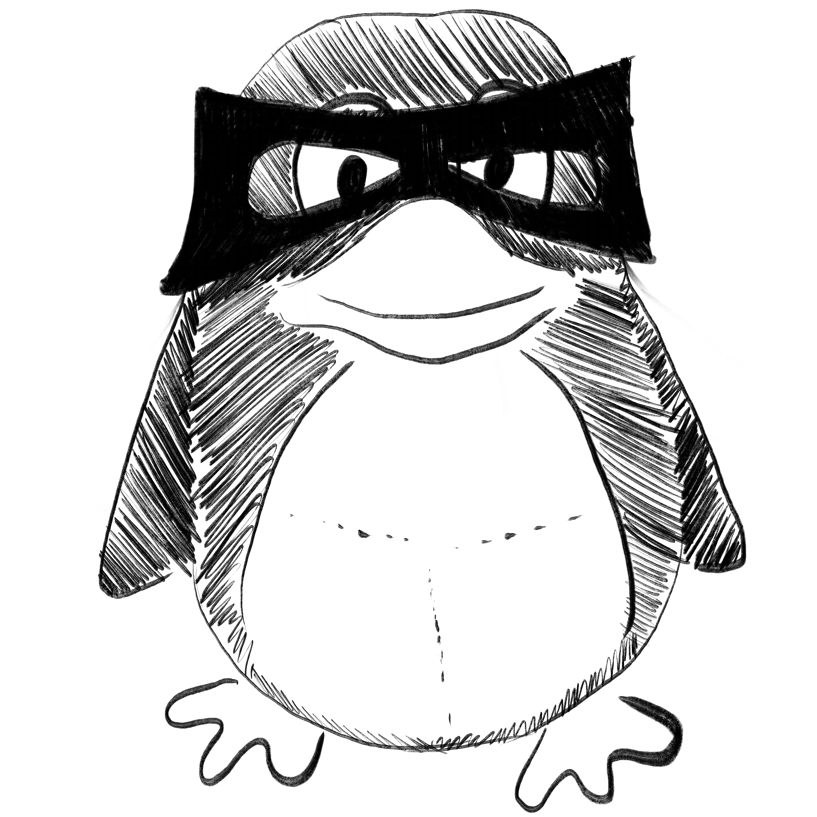
Random Copolymer inverse design system orienting on Accurate discovering of Antimicrobial peptide-mimetic copolymers
ArXiv Preprint
Tianyu Wu, Yang Tang
2022-11-30
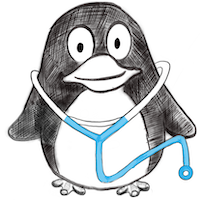
The prognostic utility of serum thyrotropin in hospitalized Covid-19 patients: statistical and machine learning approaches.
In Endocrine
PURPOSE :
METHODS :
RESULTS :
CONCLUSION :
Pappa E, Gourna P, Galatas G, Manti M, Romiou A, Panagiotou L, Chatzikyriakou R, Trakas N, Feretzakis G, Christopoulos C
2022-Nov-29
Artificial intelligence, Bayes classifier, COVID-19, Machine learning, Non-thyroidal illness syndrome, Thyroid stimulating hormone
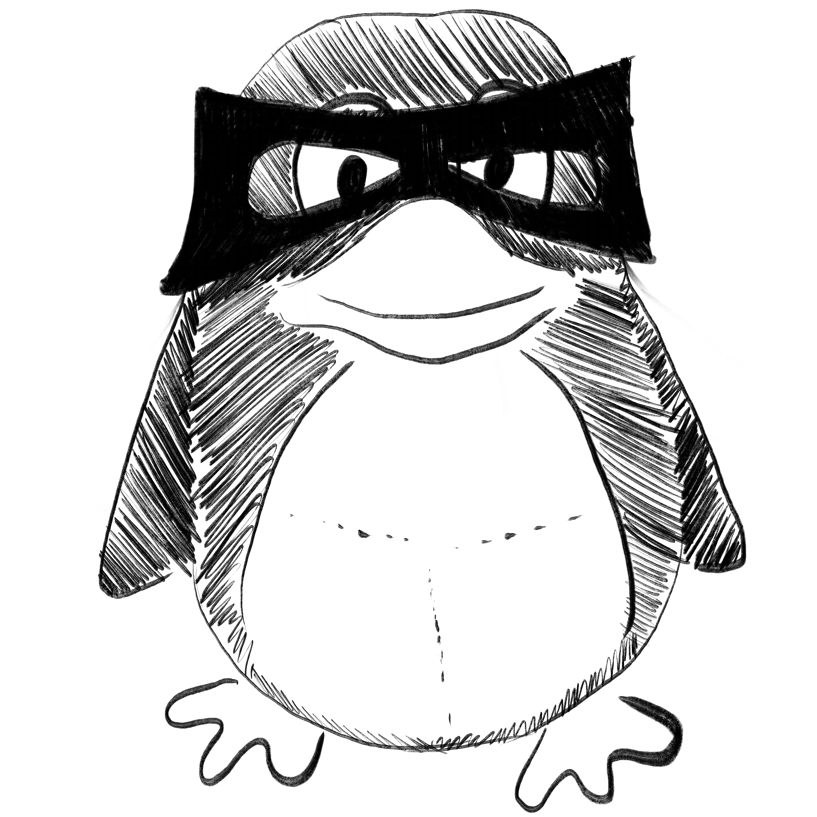
On the Design of Communication-Efficient Federated Learning for Health Monitoring
ArXiv Preprint
Dong Chu, Wael Jaafar, Halim Yanikomeroglu
2022-11-30
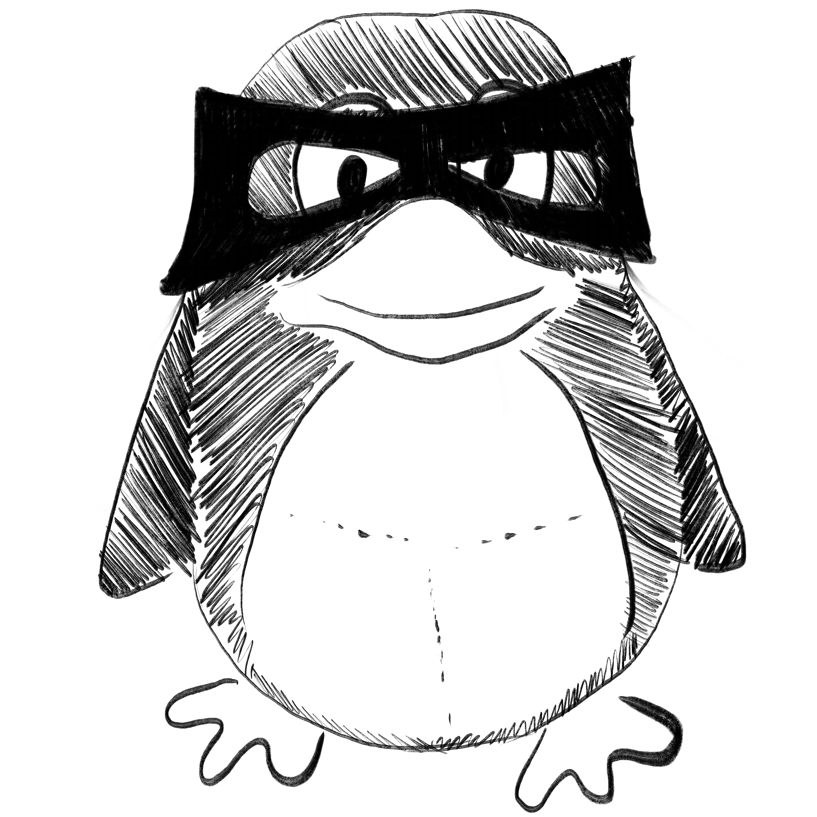
Automatic Detection of Twitter Users Who Express Chronic Stress Experiences via Supervised Machine Learning and Natural Language Processing.
In Computers, informatics, nursing : CIN
Yang Yuan-Chi, Xie Angel, Kim Sangmi, Hair Jessica, Al-Garadi Mohammed, Sarker Abeed
2022-Nov-28
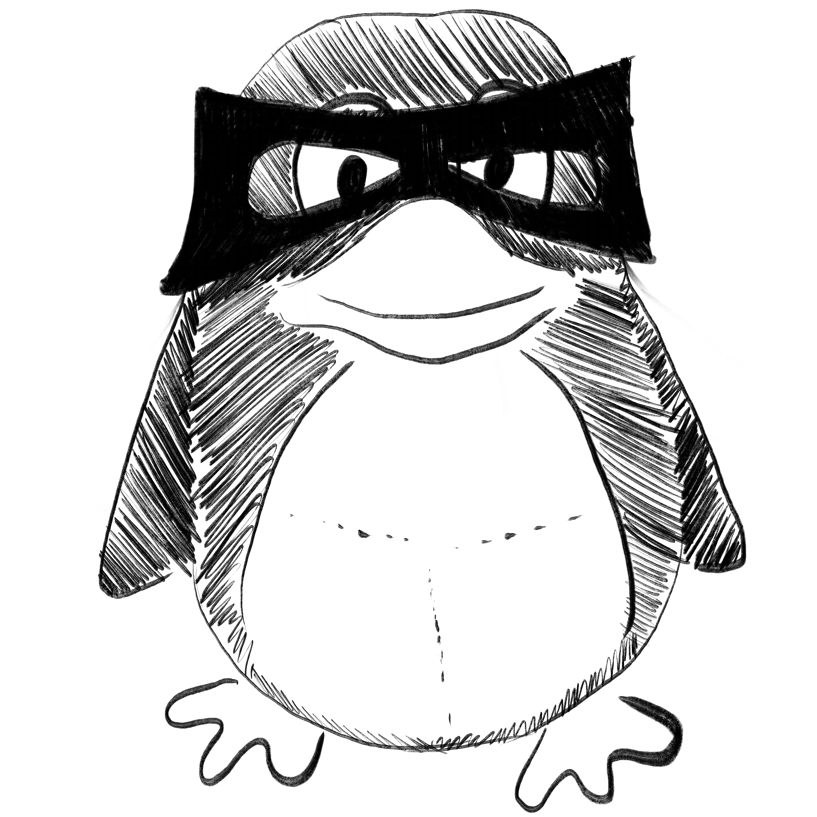
Learning Motion-Robust Remote Photoplethysmography through Arbitrary Resolution Videos
ArXiv Preprint
Jianwei Li, Zitong Yu, Jingang Shi
2022-11-30
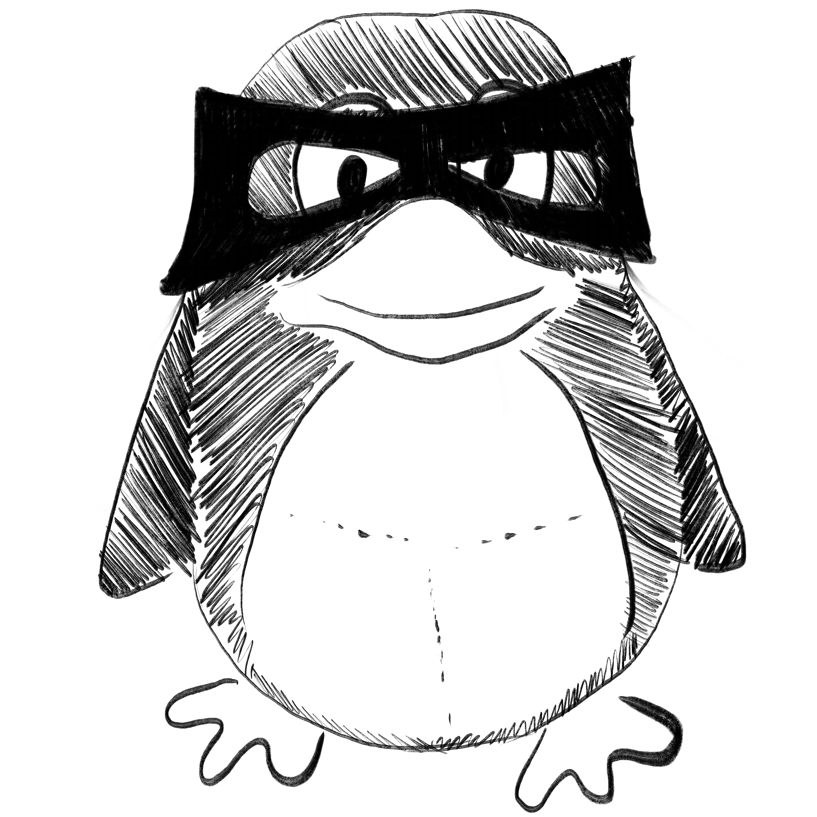
Reactive-diffusion epidemic model on human mobility networks: Analysis and applications to COVID-19 in China.
In Physica A
Li Ruqi, Song Yurong, Wang Haiyan, Jiang Guo-Ping, Xiao Min
2022-Nov-21
City clusters, Human mobility networks, Intervention, Reactive-diffusion epidemic model, Threshold behavior
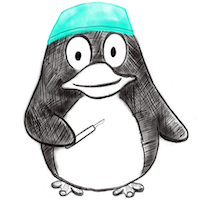
The optimal use of colon capsule endoscopes in clinical practice.
In Therapeutic advances in chronic disease
Bjørsum-Meyer Thomas, Koulaouzidis Anastasios, Baatrup Gunnar
2022
artificial intelligence, capsule endoscopy, colonic disease, endoscopy, routine diagnostic test, wireless capsule endoscopy
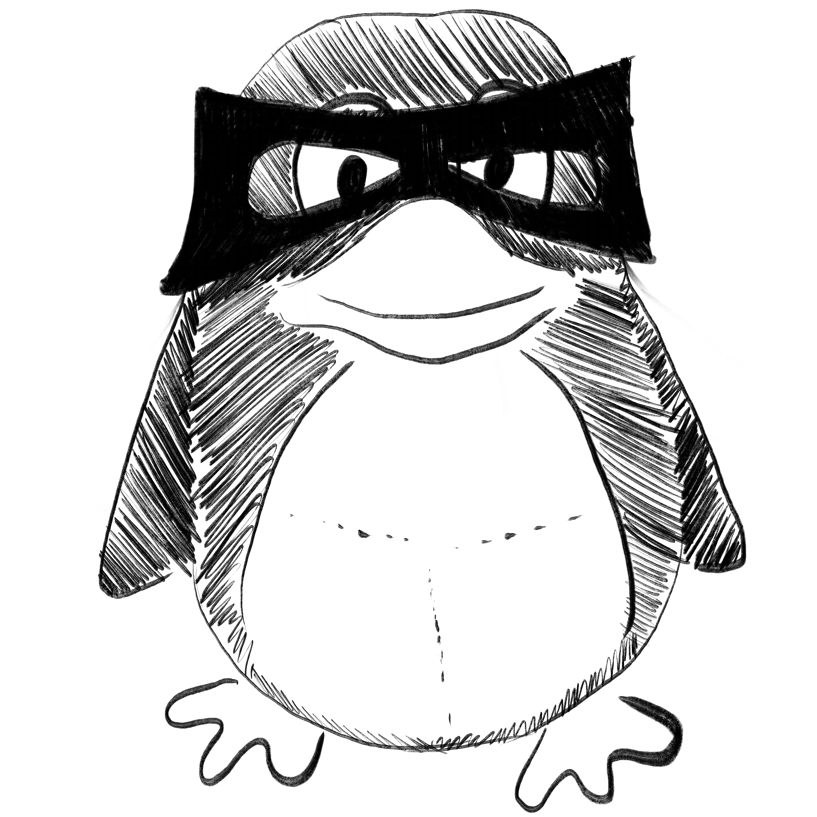
Food waste reduction and economic savings in times of crisis: The potential of machine learning methods to plan guest attendance in Swedish public catering during the Covid-19 pandemic.
In Socio-economic planning sciences
Malefors Christopher, Secondi Luca, Marchetti Stefano, Eriksson Mattias
2022-Aug
Food waste school kitchens forecasting random-forest system optimization
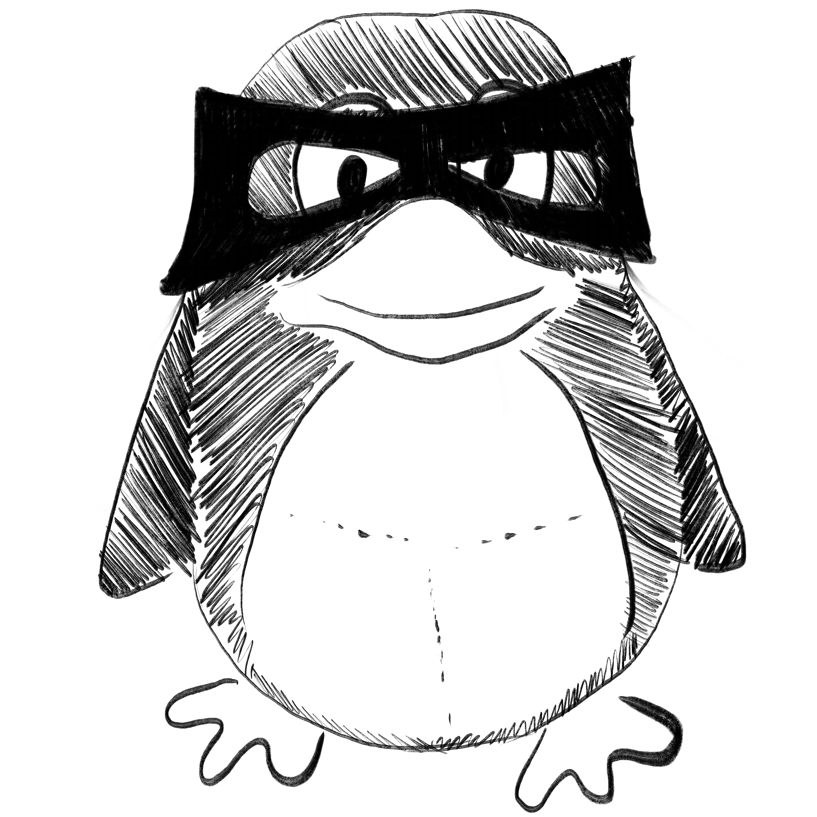
Natural language processing analysis applied to COVID-19 open-text opinions using a distilBERT model for sentiment categorization.
In AI & society
Jojoa Mario, Eftekhar Parvin, Nowrouzi-Kia Behdin, Garcia-Zapirain Begonya
2022-Nov-21
Deep learning, DistilBERT, Natural language processing, Sentiment analysis, Transformer
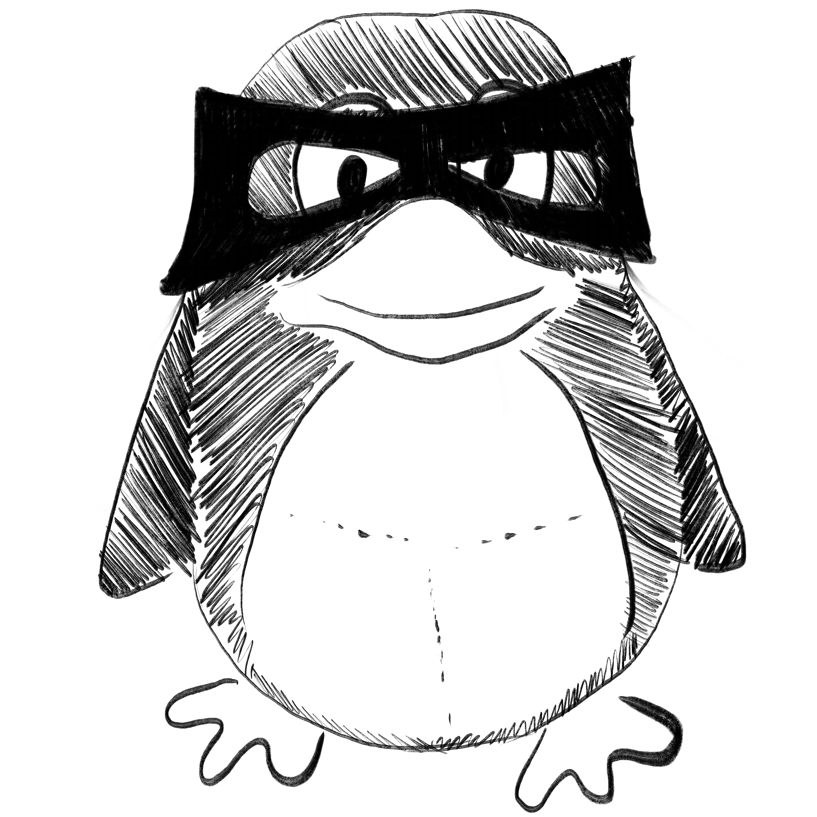
Variational Autoencoder Based Imbalanced COVID-19 Detection Using Chest X-Ray Images.
In New generation computing
Chatterjee Sankhadeep, Maity Soumyajit, Bhattacharjee Mayukh, Banerjee Soumen, Das Asit Kumar, Ding Weiping
2022-Nov-19
COVID-19, Class imbalance, Oversampling, Undersampling, Variational autoencoder
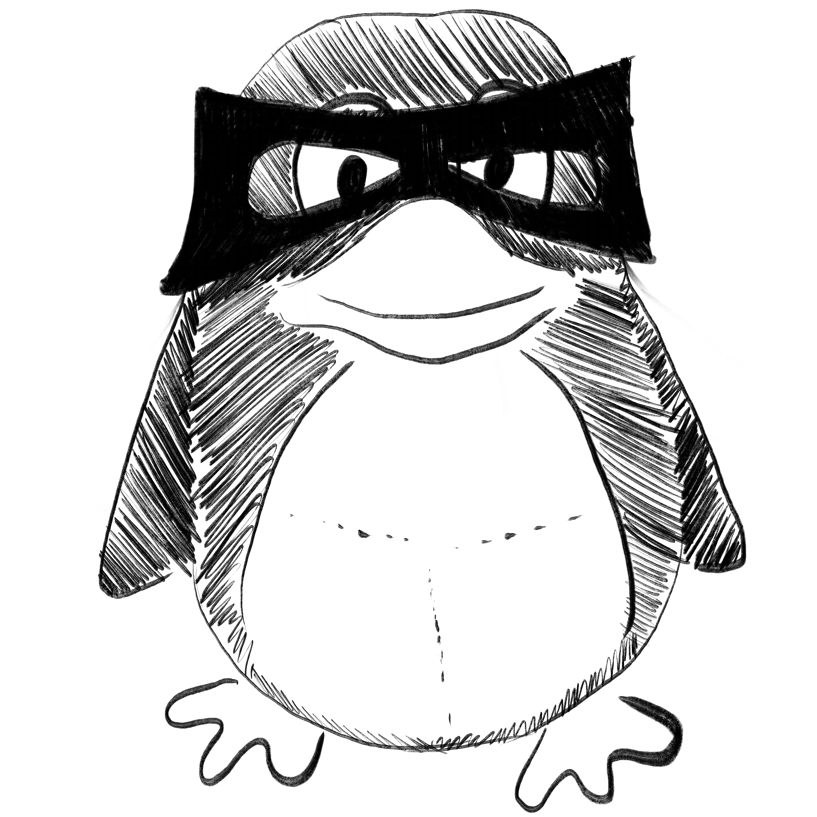
Combined Cloud-Based Inference System for the Classification of COVID-19 in CT-Scan and X-Ray Images.
In New generation computing
Dubey Ankit Kumar, Mohbey Krishna Kumar
2022-Nov-20
Area under curve, Artificial intelligence, COVID 19, Computed tomography, Inception, Transfer learning
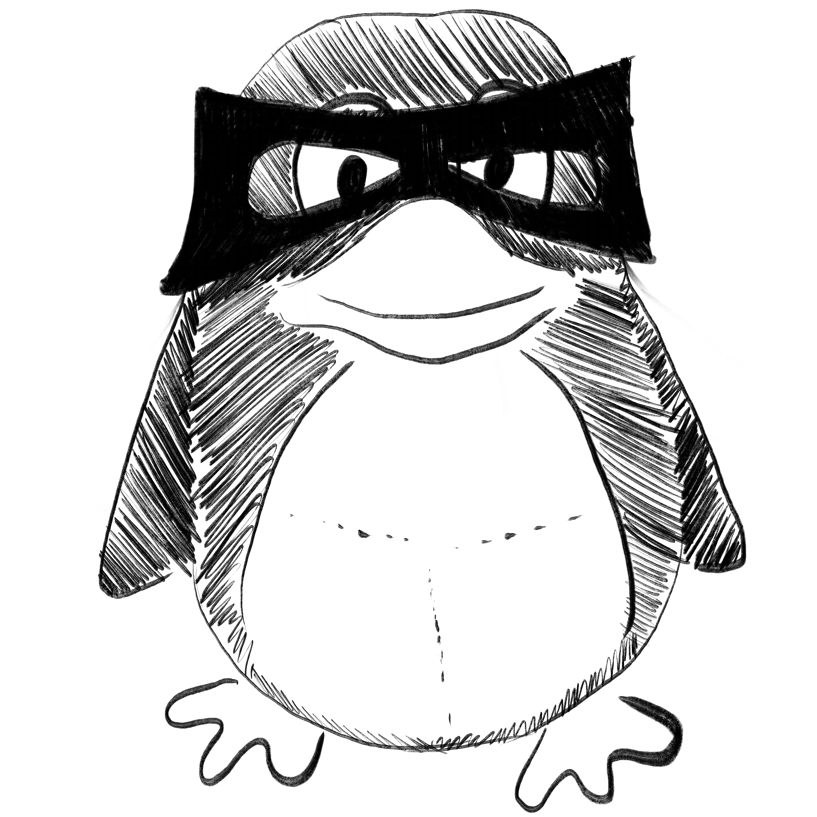
Artificial intelligence-based internet hospital pharmacy services in China: Perspective based on a case study.
In Frontiers in pharmacology
Bu Fengjiao, Sun Hong, Li Ling, Tang Fengmin, Zhang Xiuwen, Yan Jingchao, Ye Zhengqiang, Huang Taomin
2022
artificial intelligence, internet hospital, medication pick-up code, online medication consultation, prescription preview
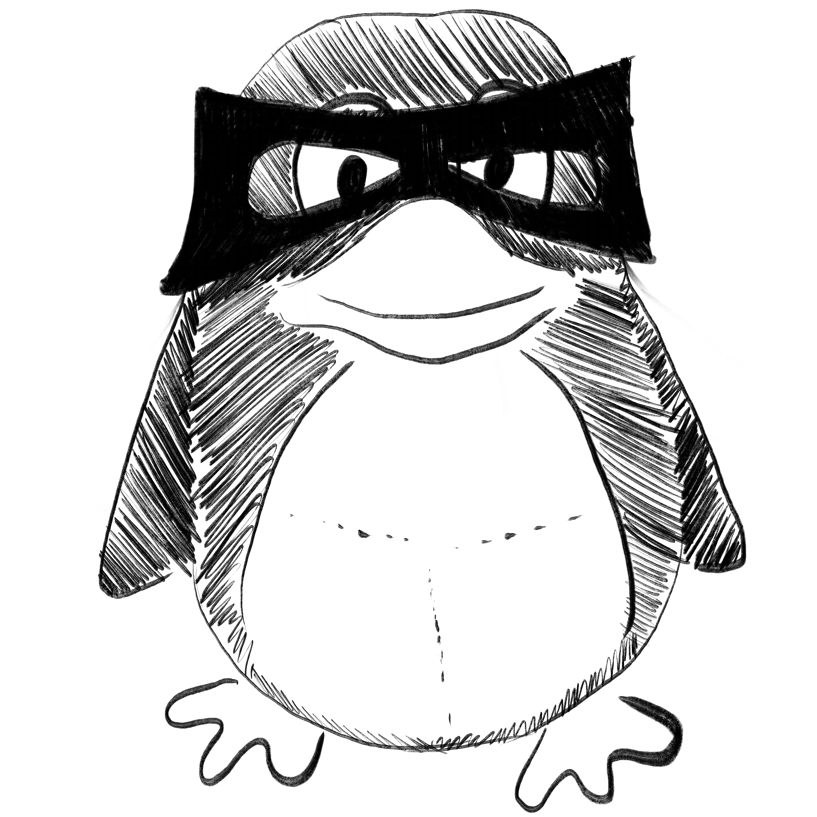
Space-Distributed Traffic-Enhanced LSTM-Based Machine Learning Model for COVID-19 Incidence Forecasting.
In Computational intelligence and neuroscience
Muñoz-Organero Mario
2022
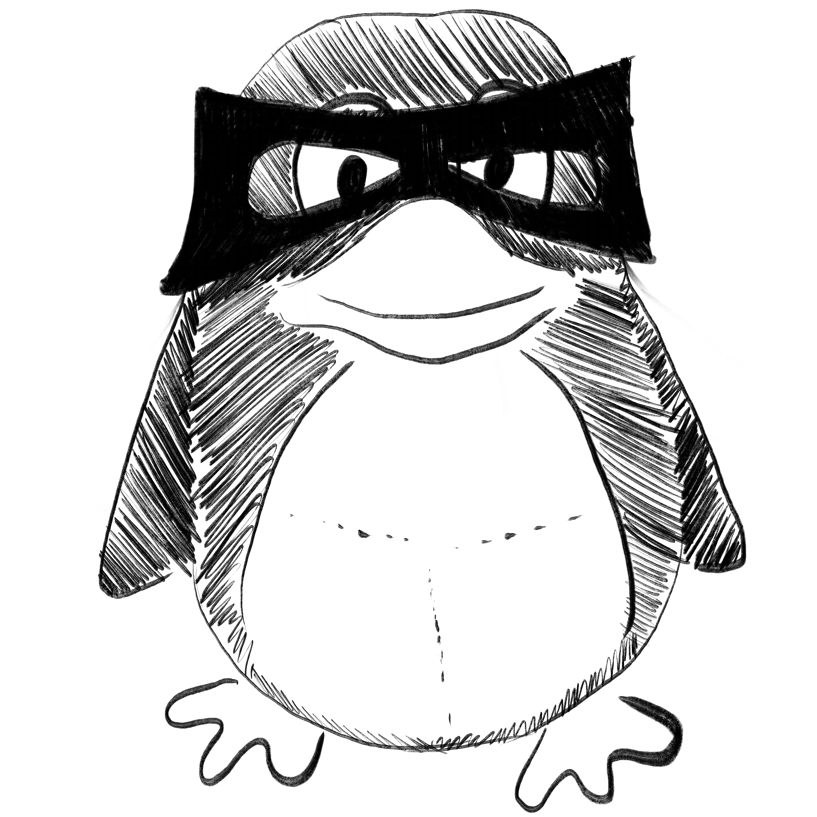
An ensemble prediction model for COVID-19 mortality risk.
In Biology methods & protocols
BACKGROUND :
METHODS :
RESULTS :
CONCLUSIONS :
Li Jie, Li Xin, Hutchinson John, Asad Mohammad, Liu Yinghui, Wang Yadong, Wang Edwin
2022
COVID-19, SARS-CoV-2, cohort studies, mortality prediction, prognosis
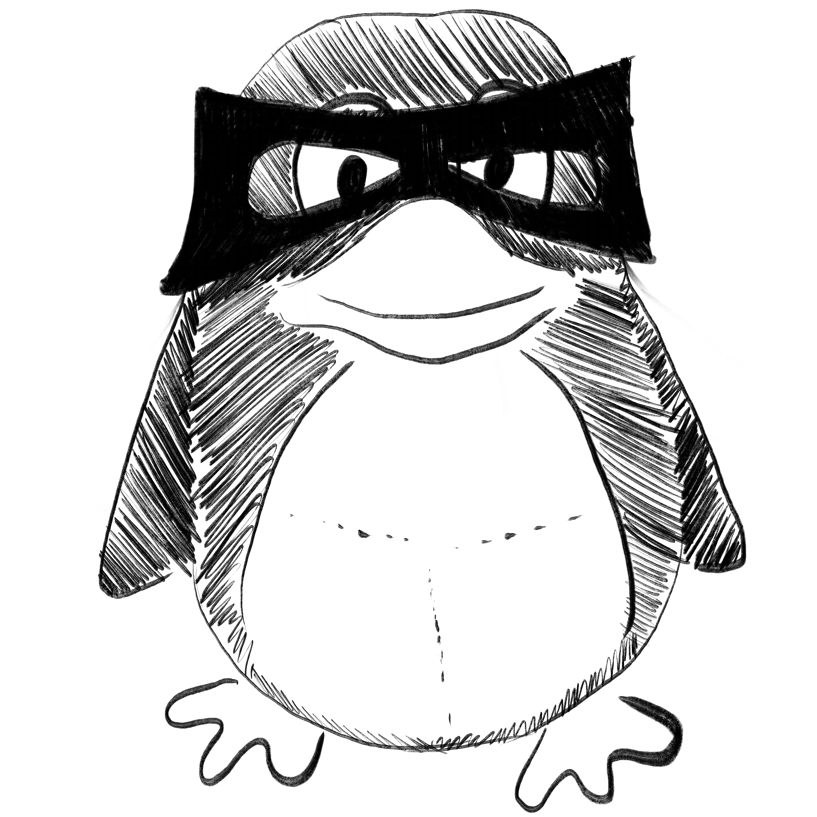
A semi-supervised Bayesian mixture modelling approach for joint batch correction and classification
bioRxiv Preprint
Coleman, S.; Nicholls, K. C.; Castro Dopico, X.; Karlsson Hedestam, G. B.; Kirk, P. D.; Wallace, C.
2022-11-29
Machine learning based regional epidemic transmission risks precaution in digital society.
In Scientific reports ; h5-index 158.0
Shi Zhengyu, Qian Haoqi, Li Yao, Wu Fan, Wu Libo
2022-Nov-28
Contextual factors predicting compliance behavior during the COVID-19 pandemic: A machine learning analysis on survey data from 16 countries.
In PloS one ; h5-index 176.0
Hajdu Nandor, Schmidt Kathleen, Acs Gergely, Röer Jan P, Mirisola Alberto, Giammusso Isabella, Arriaga Patrícia, Ribeiro Rafael, Dubrov Dmitrii, Grigoryev Dmitry, Arinze Nwadiogo C, Voracek Martin, Stieger Stefan, Adamkovic Matus, Elsherif Mahmoud, Kern Bettina M J, Barzykowski Krystian, Ilczuk Ewa, Martončik Marcel, Ropovik Ivan, Ruiz-Fernandez Susana, Baník Gabriel, Ulloa José Luis, Aczel Balazs, Szaszi Barnabas
2022
Advances in Cardiac Electrophysiology.
In Circulation. Arrhythmia and electrophysiology
Piccini Jonathan P, Russo Andrea M, Sharma Parikshit S, Kron Jordana, Tzou Wendy, Sauer William, Park David S, Birgersdotter-Green Ulrika, Frankel David S, Healey Jeff S, Hummel John, Koruth Jacob, Linz Dominik, Mittal Suneet, Nair Devi G, Nattel Stanley, Noseworthy Peter A, Steinberg Benjamin A, Trayanova Natalia A, Wan Elaine Y, Wissner Erik, Zeitler Emily P, Wang Paul J
2022-Nov-28
arrhythmias, atrial fibrillation, cardiac electrophysiology, implantable defibrillators, ventricular tachycardia
Comparison of machine learning methods with logistic regression analysis in creating predictive models for risk of critical in-hospital events in COVID-19 patients on hospital admission.
In BMC medical informatics and decision making ; h5-index 38.0
BACKGROUND :
METHODS :
RESULTS :
CONCLUSIONS :
TRIAL REGISTRATION NUMBER :
Sievering Aaron W, Wohlmuth Peter, Geßler Nele, Gunawardene Melanie A, Herrlinger Klaus, Bein Berthold, Arnold Dirk, Bergmann Martin, Nowak Lorenz, Gloeckner Christian, Koch Ina, Bachmann Martin, Herborn Christoph U, Stang Axel
2022-Nov-28
COVID-19, Clinical decision-making, Critical event prediction, Machine learning, Predictive models
Applied artificial intelligence in healthcare: Listening to the winds of change in a post-COVID-19 world.
In Experimental biology and medicine (Maywood, N.J.)
Shaban-Nejad Arash, Michalowski Martin, Bianco Simone, Brownstein John S, Buckeridge David L, Davis Robert L
2022-Nov-25
AI governance, COVID-19, Health AI, artificial intelligence, ethical AI, human-centered AI, machine learning, multimodal AI
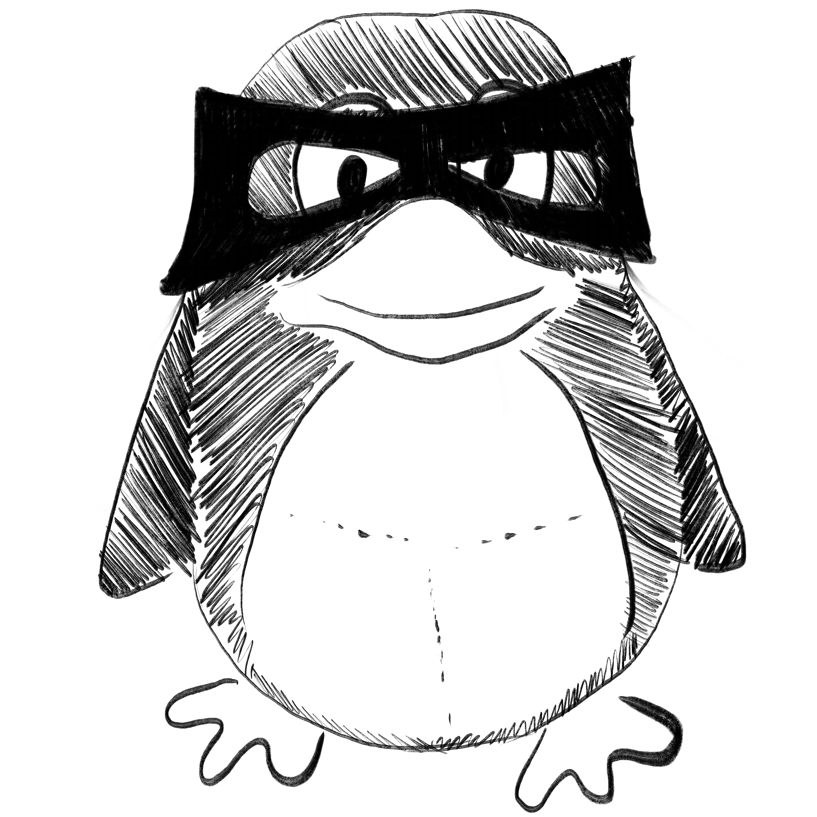
Epidemiology and clinical features of SARS-CoV-2 infection in hospitalized children across four waves in Hungary: A retrospective, comparative study from March 2020 to December 2021.
In Health science reports
BACKGROUND AND AIMS :
METHODS :
RESULTS :
CONCLUSIONS :
Takács Andrea T, Bukva Mátyás, Gavallér Gabriella, Kapus Katalin, Rózsa Mária, Bán-Gagyi Boglárka, Sinkó Mária, Szűcs Dániel, Terhes Gabriella, Bereczki Csaba
2022-Nov
COVID‐19, SARS‐CoV‐2 infection, children, hospitalization wave
Prediction of Global Psychological Stress and Coping Induced by the COVID-19 Outbreak: A Machine Learning Study.
In Alpha psychiatry
BACKGROUND :
METHODS :
RESULTS :
CONCLUSIONS :
Prerna Tigga Neha, Garg Shruti
2022-Jul
COVID-19, coping, machine learning, public health, stress
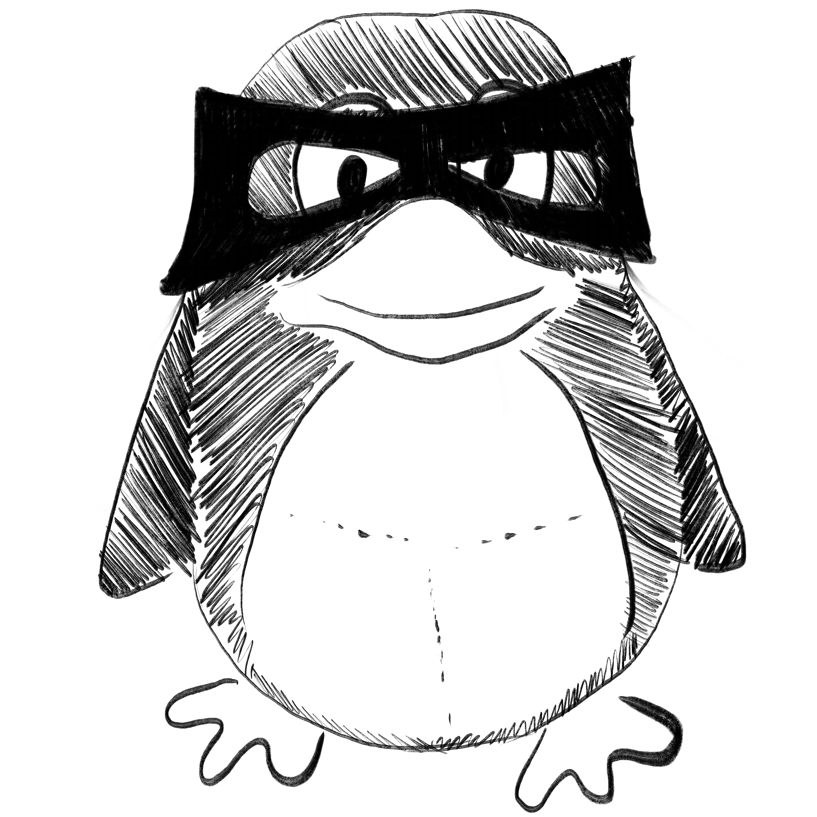
COVID-19 screening with digital holographic microscopy using intra-patient probability functions of spatio-temporal bio-optical attributes.
In Biomedical optics express
O’Connor Timothy, Javidi Bahram
2022-Oct-01
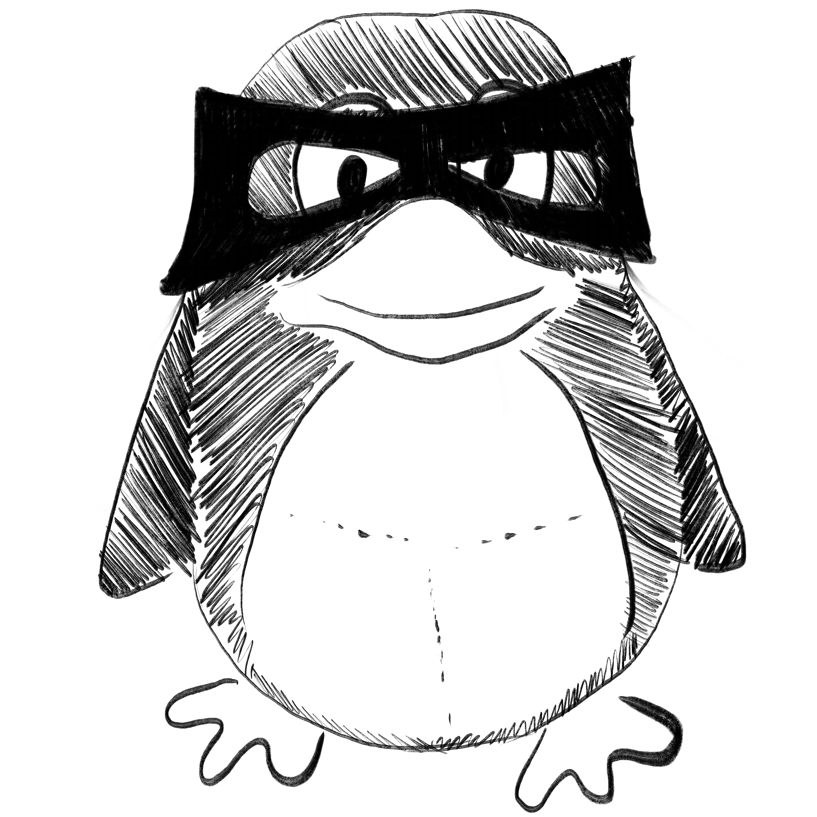
Dual_Pachi: Attention-based dual path framework with intermediate second order-pooling for Covid-19 detection from chest X-ray images.
In Computers in biology and medicine
Ukwuoma Chiagoziem C, Qin Zhiguang, Agbesi Victor K, Cobbinah Bernard M, Yussif Sophyani B, Abubakar Hassan S, Lemessa Bona D
2022-Nov-18
Attention mechanism, COVID-19 detection, Chest X-rays images, Deep learning, Feature extraction, Global second-order pooling
The Role of Natural Language Processing during the COVID-19 Pandemic: Health Applications, Opportunities, and Challenges.
In Healthcare (Basel, Switzerland)
Al-Garadi Mohammed Ali, Yang Yuan-Chi, Sarker Abeed
2022-Nov-12
COVID-19, deep learning, health applications, machine learning, natural language processing
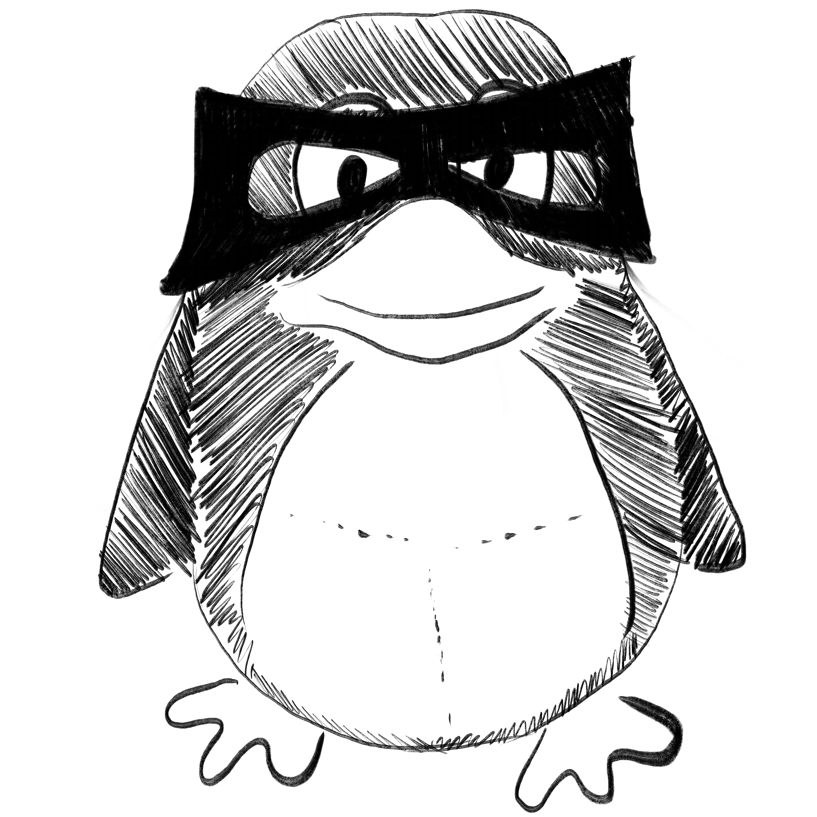
Automated Lung-Related Pneumonia and COVID-19 Detection Based on Novel Feature Extraction Framework and Vision Transformer Approaches Using Chest X-ray Images.
In Bioengineering (Basel, Switzerland)
Ukwuoma Chiagoziem C, Qin Zhiguang, Heyat Md Belal Bin, Akhtar Faijan, Smahi Abla, Jackson Jehoiada K, Furqan Qadri Syed, Muaad Abdullah Y, Monday Happy N, Nneji Grace U
2022-Nov-18
COVID-19, artificial intelligence, automatic detection, chest X-rays images, epidemic, feature extraction, lung disease, pneumonia
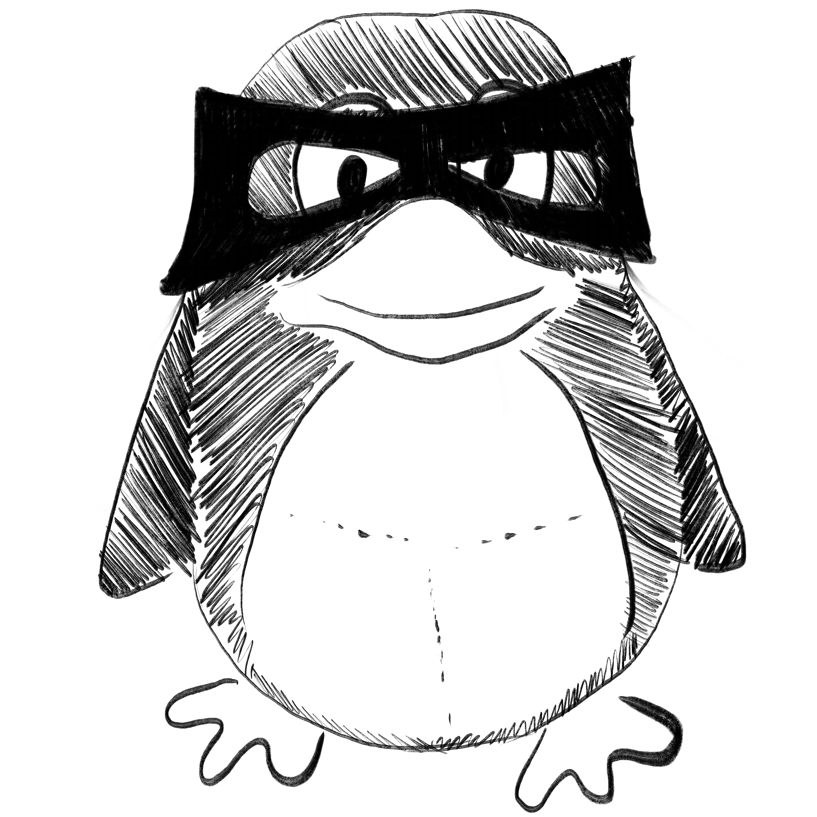
Diverse COVID-19 CT Image-to-Image Translation with Stacked Residual Dropout.
In Bioengineering (Basel, Switzerland)
Lee Kin Wai, Chin Renee Ka Yin
2022-Nov-16
COVID-19, chest computed tomography, generative adversarial networks, image synthesis
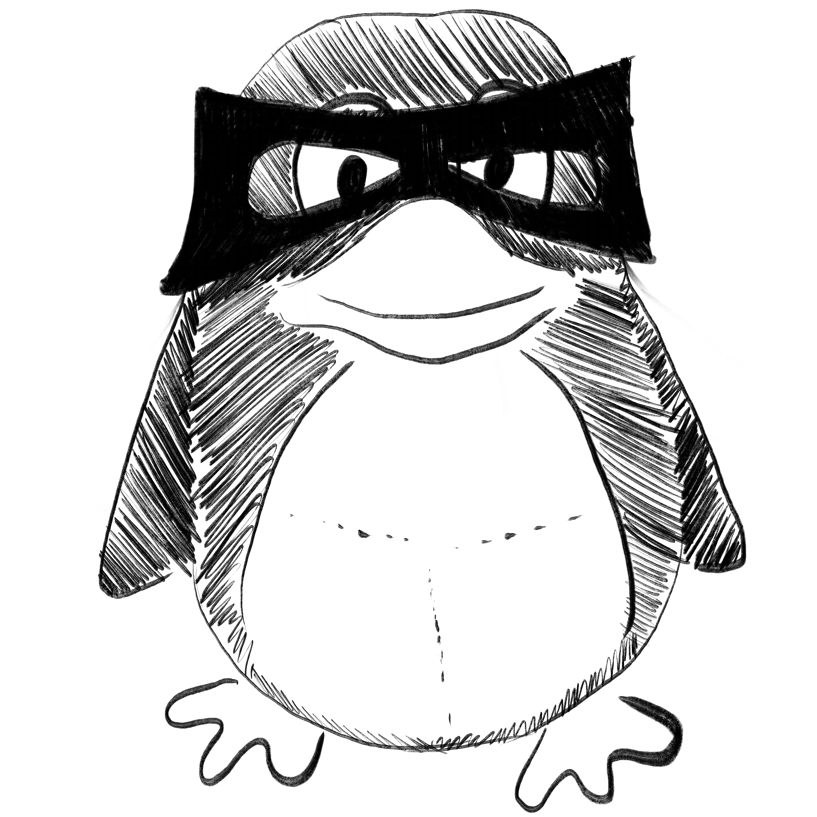
Portable, Automated and Deep-Learning-Enabled Microscopy for Smartphone-Tethered Optical Platform Towards Remote Homecare Diagnostics: A Review.
In Small methods
Kim Kisoo, Lee Won Gu
2022-Nov-24
Smartphone-Tethered Optical Platform, deep learning-enhanced microscopic imaging, lens-free holographic imaging, personalized diagnostics, portable COVID screening system, smartphone-based microscopy
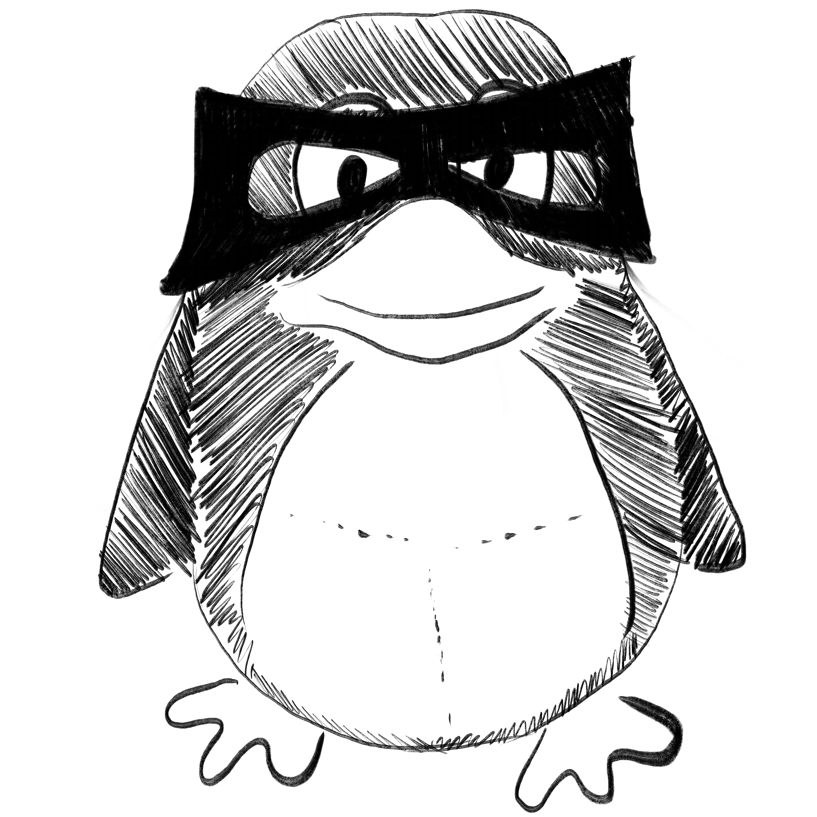
A systematic review: Chest radiography images (X-ray images) analysis and COVID-19 categorization diagnosis using artificial intelligence techniques.
In Network (Bristol, England)
Suba Saravanan, Muthulakshmi M
2022-Nov-24
AI tools deep learning approaches, COVID-19, chest radiography images, machine learning approaches
COVID-19 smart surveillance: Examination of Knowledge of Apps and mobile thermometer detectors (MTDs) in a high-risk society.
In Digital health
BACKGROUND :
MATERIALS AND METHODS :
RESULTS :
CONCLUSION :
Sayibu Muhideen, Chu Jianxun, Tosin Yinka Akintunde, Rufai Olayemi Hafeez, Shahani Riffat, Jin M A
2022
COVID-19 surveillance, knowledge of app, mobile intelligence, mobile thermometer detectors (MTD), risk mitigation
Deep-Data-Driven Neural Networks for COVID-19 Vaccine Efficacy.
In Epidemiolgia (Basel, Switzerland)
Torku Thomas K, Khaliq Abdul Q M, Furati Khaled M
2021-Nov-30
COVID-19, RNN, ResNet, data-driven, deep learning, k-fold cross validation, vaccination strategy
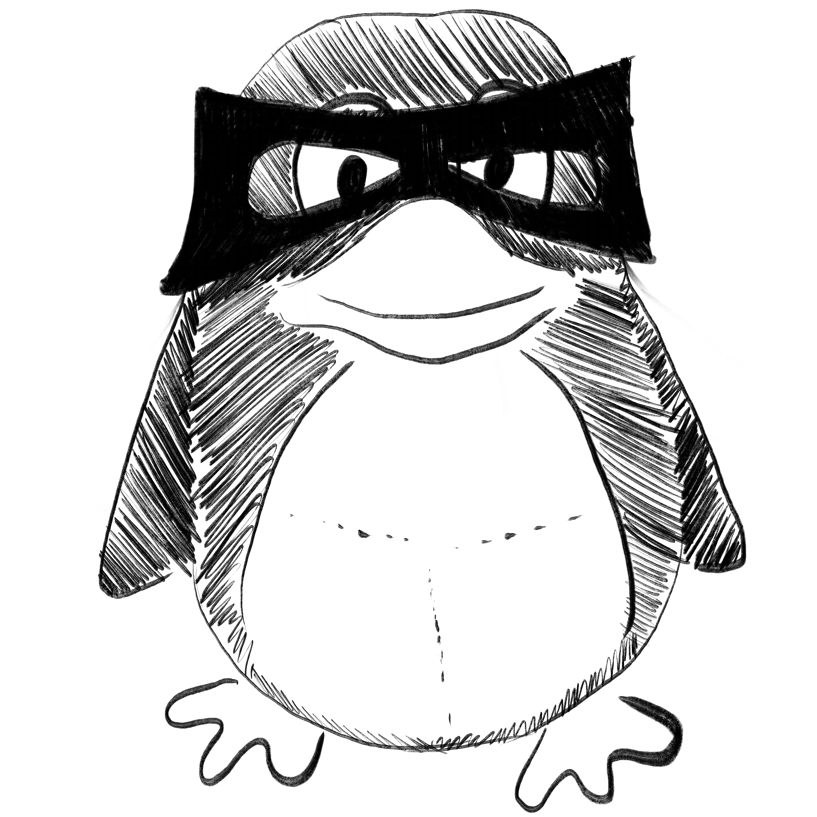
Variation in the ACE2 receptor has limited utility for SARS-CoV-2 host prediction.
In eLife
Mollentze Nardus, Keen Deborah, Munkhbayar Uuriintuya, Biek Roman, Streicker Daniel G
2022-Nov-23
ACE2, SARS-CoV-2, ecology, host range, infectious disease, microbiology, viruses
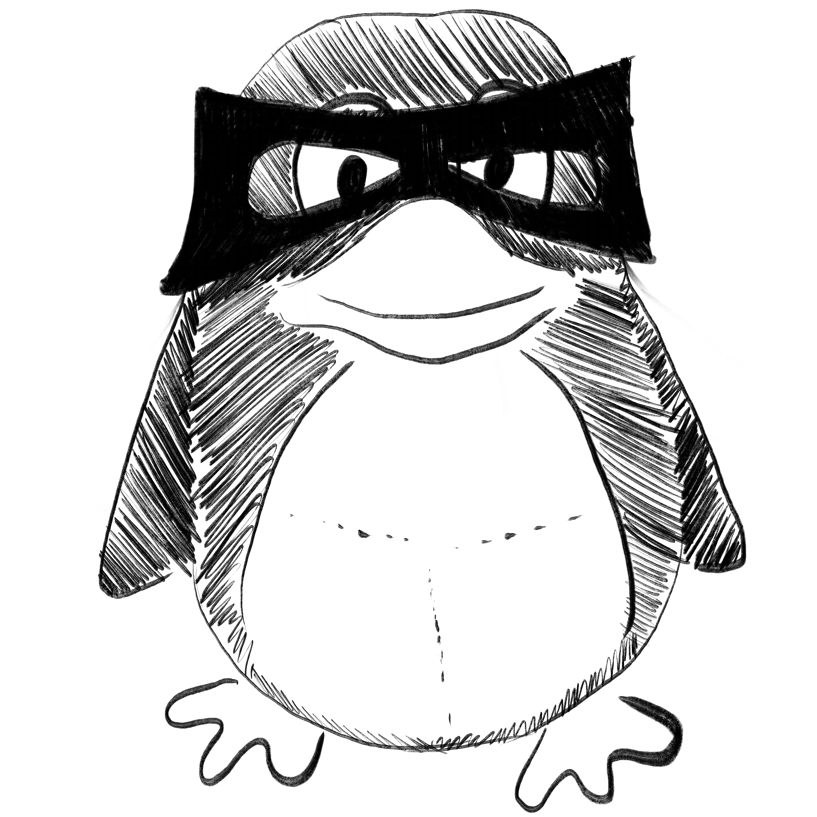
Cov-TransNet: Dual branch fusion network with transformer for COVID-19 infection segmentation.
In Biomedical signal processing and control
Peng Yanjun, Zhang Tong, Guo Yanfei
2023-Feb
Attention mechanism, COVID-19, CT images, Deep learning, Semantic segmentation, Transformer
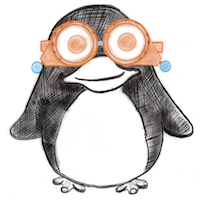
Ischemic stroke of unclear aetiology: a case-by-case analysis and call for a multi-professional predictive, preventive and personalised approach.
In The EPMA journal
Golubnitschaja Olga, Potuznik Pavel, Polivka Jiri, Pesta Martin, Kaverina Olga, Pieper Claus C, Kropp Martina, Thumann Gabriele, Erb Carl, Karabatsiakis Alexander, Stetkarova Ivana, Polivka Jiri, Costigliola Vincenzo
2022-Nov-17
Blood pressure, Blood–brain barrier breakdown, Body mass index, COVID-19, Cancer, Coagulation, Connective tissue impairments, Diabetes comorbidities, Endothelial dysfunction, Endothelin-1, Flammer Syndrome phenotype, Health policy, Health risk assessment, Health-to-disease transition, Hypoxia-reperfusion, Individualised protection, Ischemic stroke, Lacunar stroke, Mental health, Metastasis, Normal-tension glaucoma, Optic nerve degeneration, Paradigm change, Pre-pregnancy check-up, Predictive preventive personalised medicine (PPPM / 3PM), Primary care, Pro-inflammation, Retinal microvascular abnormalities, Screening, Secondary care, Silent brain infarct, Small vessel disease, Stress, Sub-optimal health, Systemic effects, Thromboembolism, Vascular stiffness, Vasospasm, Young populations
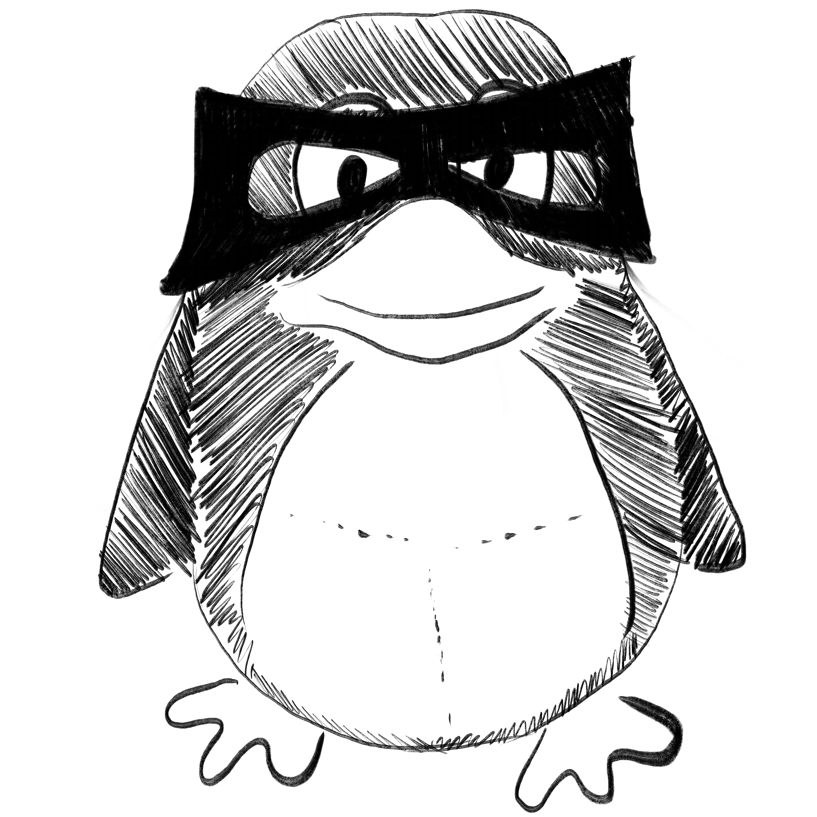
Integration of machine learning prediction and heuristic optimization for mask delivery in COVID-19.
In Swarm and evolutionary computation
Chen Xin, Yan Hong-Fang, Zheng Yu-Jun, Karatas Mumtaz
2022-Nov-16
Heuristic optimization, Machine learning, Pre-distribution, Tabu search, Variable neighborhood, Vehicle routing
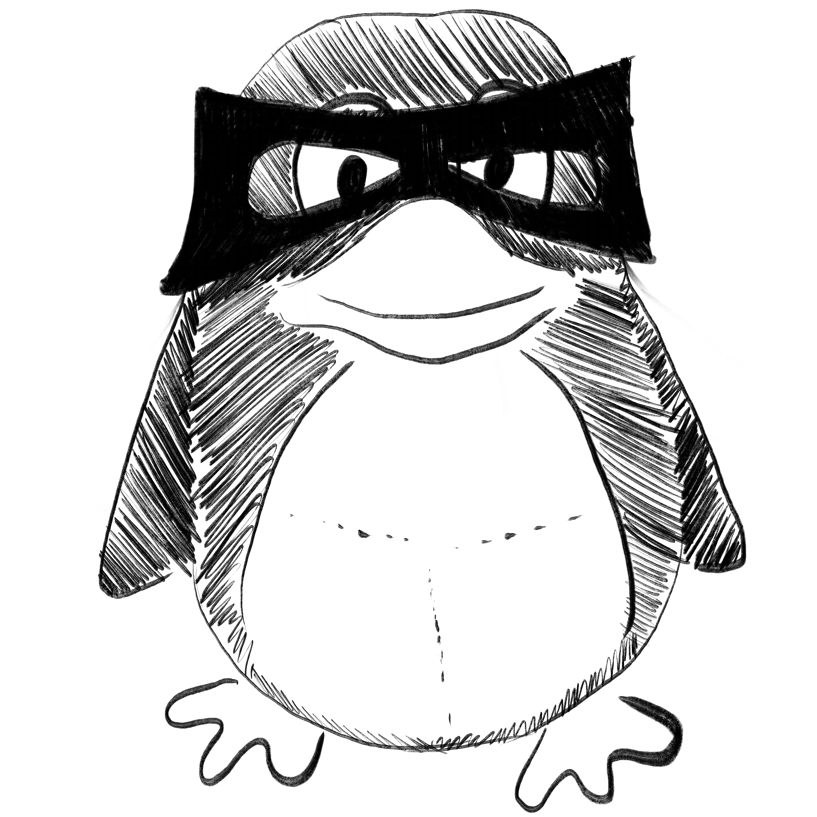
Future forecasting prediction of Covid-19 using hybrid deep learning algorithm.
In Multimedia tools and applications
Yenurkar Ganesh, Mal Sandip
2022-Nov-18
As well as mayfly optimization (MO) algorithm, Corona disease, Feature extraction, Feature selection, GoogleNet, Hybrid deep learning model, ResNet
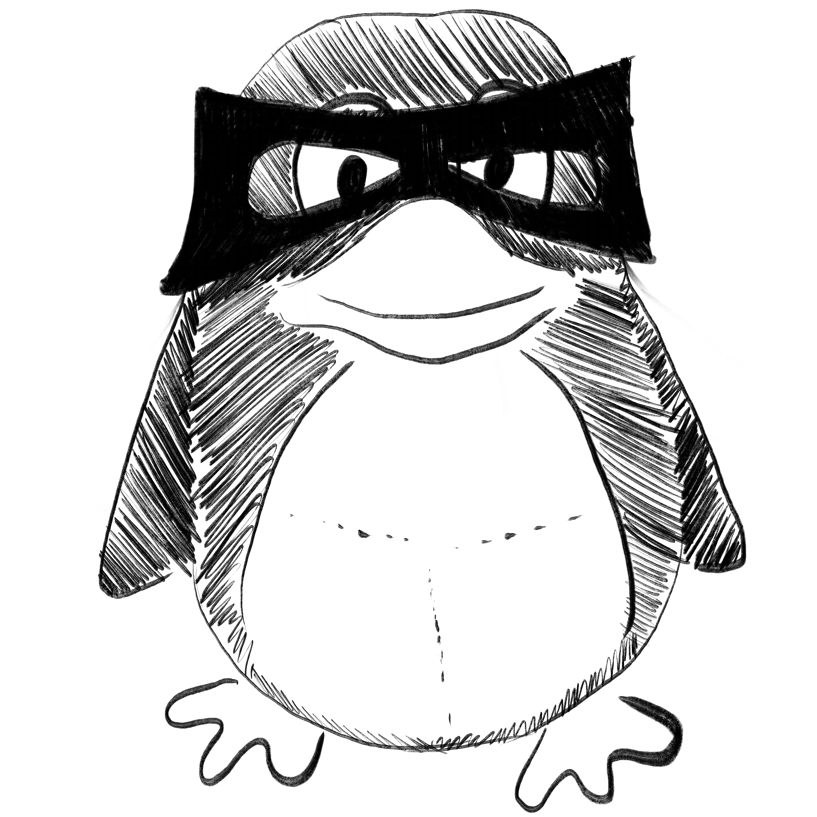
ECG-COVID: An end-to-end deep model based on electrocardiogram for COVID-19 detection.
In Information sciences
Sakr Ahmed S, Pławiak Paweł, Tadeusiewicz Ryszard, Pławiak Joanna, Sakr Mohamed, Hammad Mohamed
2023-Jan
CNN, COVID-19, Deep learning, ECG, End-to-end
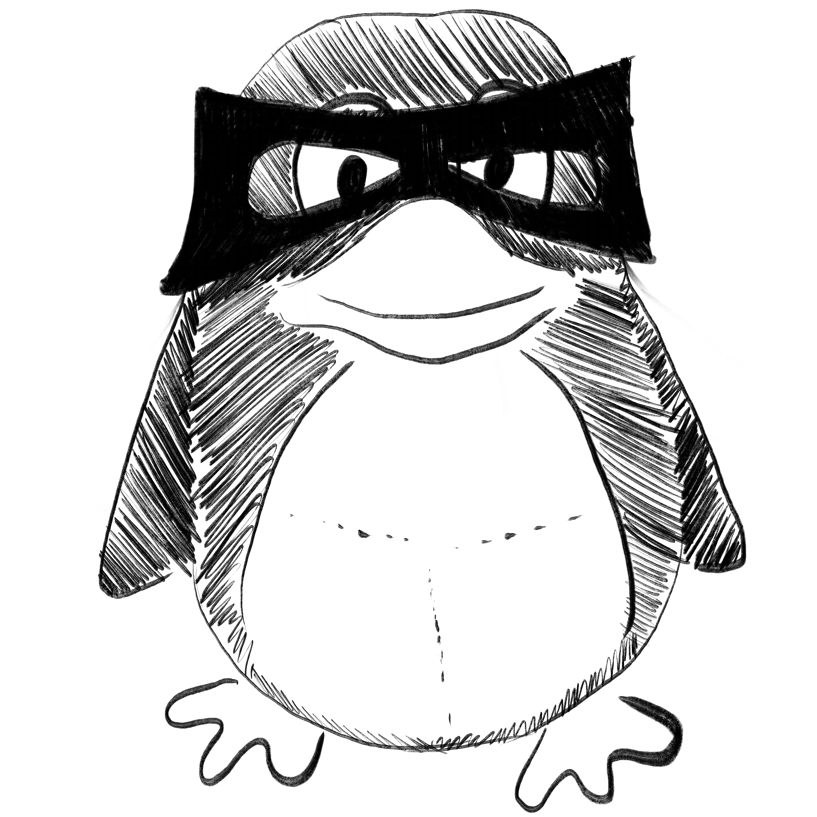
Explaining COVID-19 diagnosis with Taylor decompositions.
In Neural computing & applications
Hassan Mohammad Mehedi, AlQahtani Salman A, Alelaiwi Abdulhameed, Papa João P
2022-Nov-17
COVID-19, Deep Taylor expansion, Explainable artificial intelligence, Machine learning
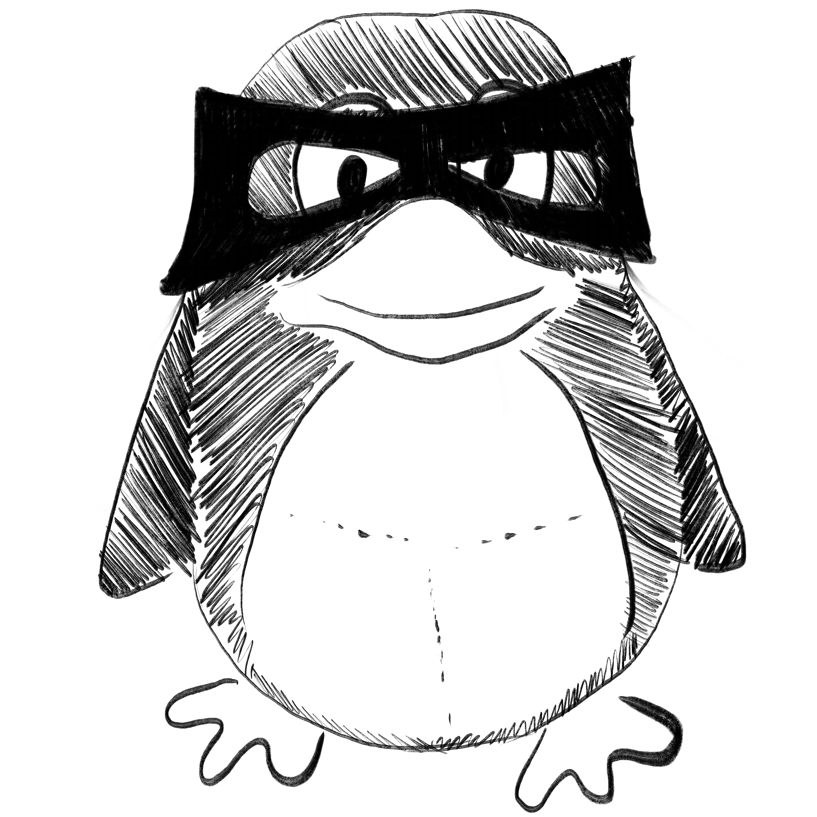
Fragment-Based Hit Discovery via Unsupervised Learning of Fragment-Protein Complexes
bioRxiv Preprint
McCorkindale, W. J.; Ahel, I.; Barr, H.; Correy, G. J.; Fraser, J. S.; London, N.; Schuller, M.; Shurrush, K.; Lee, A. A.
2022-11-24
Vocal biomarker predicts fatigue in people with COVID-19: results from the prospective Predi-COVID cohort study.
In BMJ open
OBJECTIVE :
DESIGN :
SETTING :
PARTICIPANTS :
PRIMARY AND SECONDARY OUTCOME MEASURES :
RESULTS :
CONCLUSIONS :
TRIAL REGISTRATION NUMBER :
Elbéji Abir, Zhang Lu, Higa Eduardo, Fischer Aurélie, Despotovic Vladimir, Nazarov Petr V, Aguayo Gloria, Fagherazzi Guy
2022-Nov-22
COVID-19, Health informatics, Public health
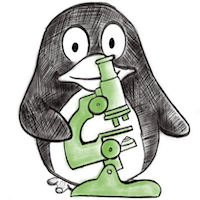
Risk Stratification of COVID-19 Using Routine Laboratory Tests: A Machine Learning Approach.
In Infectious disease reports
Mlambo Farai, Chironda Cyril, George Jaya
2022-Nov-21
COVID-19, laboratory tests, machine learning, risk stratification
MonkeyPox2022Tweets: A Large-Scale Twitter Dataset on the 2022 Monkeypox Outbreak, Findings from Analysis of Tweets, and Open Research Questions.
In Infectious disease reports
Thakur Nirmalya
2022-Nov-14
big data, data analysis, data mining, dataset, machine learning, monkeypox, natural language processing, social media, tweets, twitter
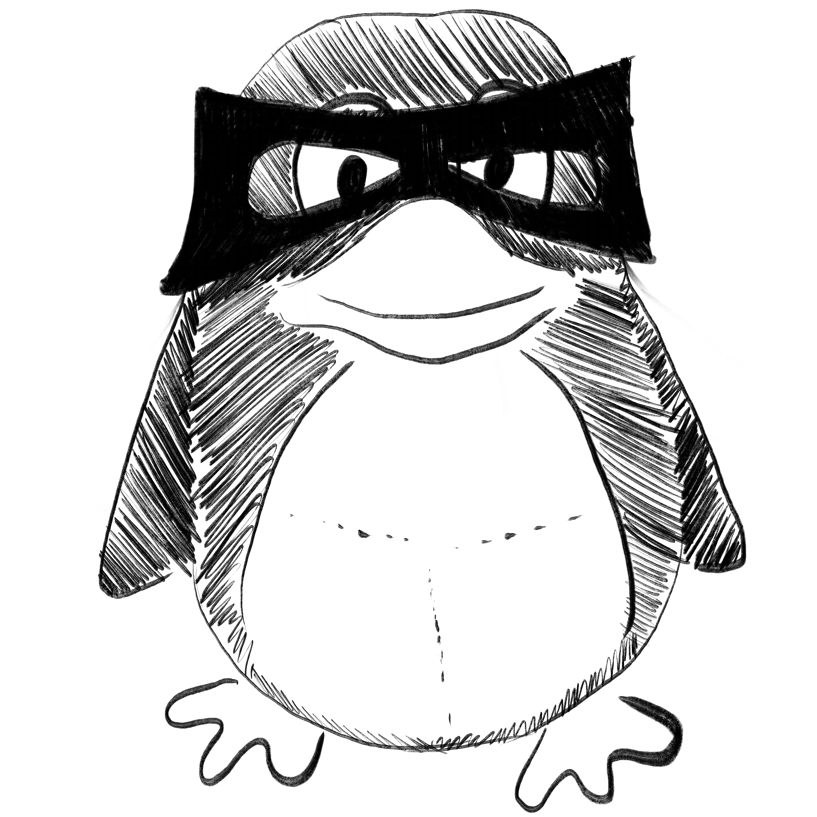
Online Dynamic Reliability Evaluation of Wind Turbines based on Drone-assisted Monitoring
ArXiv Preprint
Sohag Kabir, Koorosh Aslansefat, Prosanta Gope, Felician Campean, Yiannis Papadopoulos
2022-11-23
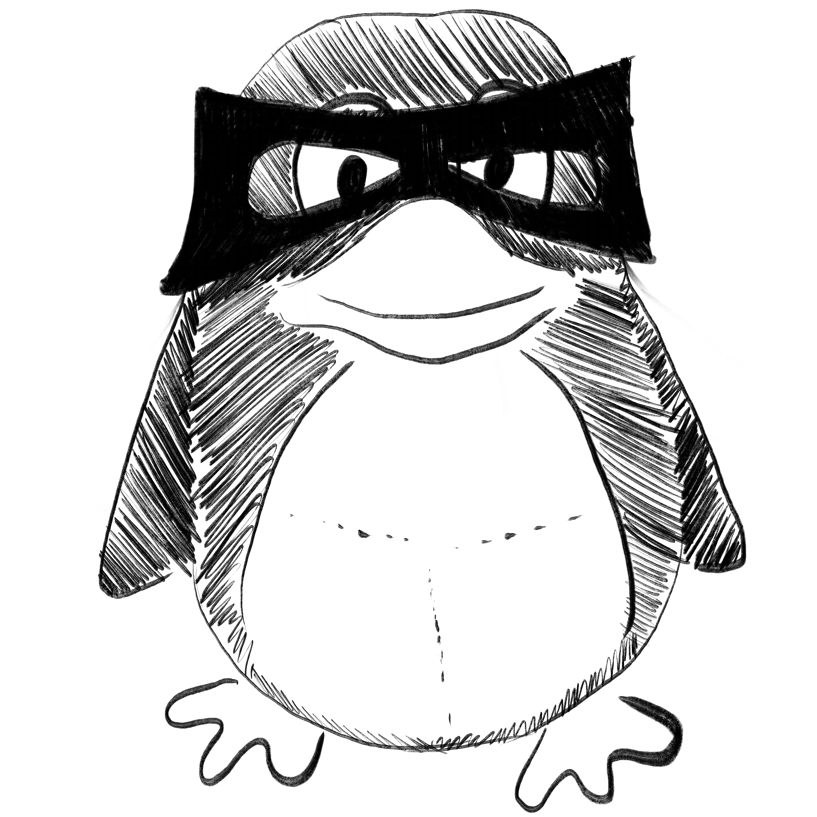
Predicting drug-target binding affinity through molecule representation block based on multi-head attention and skip connection.
In Briefings in bioinformatics
Zhang Li, Wang Chun-Chun, Chen Xing
2022-Nov-19
SARS-CoV-2, computational model, drug–target binding affinity, molecule representation block, multi-head attention, skip connection
RoentGen: Vision-Language Foundation Model for Chest X-ray Generation
ArXiv Preprint
Pierre Chambon, Christian Bluethgen, Jean-Benoit Delbrouck, Rogier Van der Sluijs, Małgorzata Połacin, Juan Manuel Zambrano Chaves, Tanishq Mathew Abraham, Shivanshu Purohit, Curtis P. Langlotz, Akshay Chaudhari
2022-11-23
CNN-LSTM deep learning based forecasting model for COVID-19 infection cases in Nigeria, South Africa and Botswana.
In Health and technology
Background :
Methods :
Results :
Conclusions :
Muhammad L J, Haruna Ahmed Abba, Sharif Usman Sani, Mohammed Mohammed Bappah
2022
COVID-19, Deep Learning, Forecasting Model, Infection, Omicron Variant of Concern
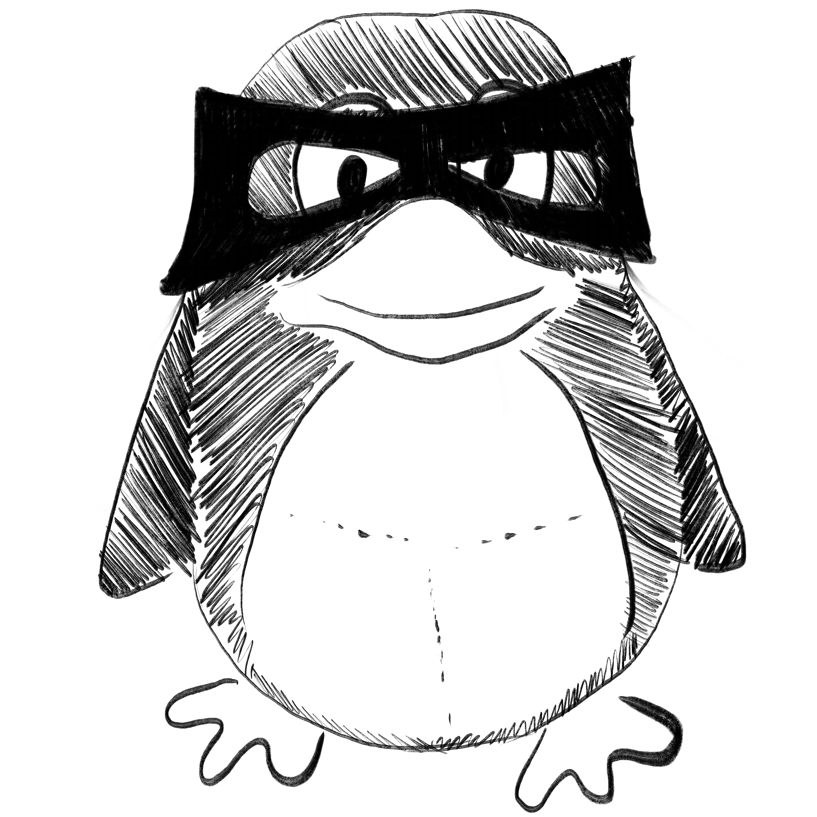
Machine learning for optimizing daily COVID-19 vaccine dissemination to combat the pandemic.
In Health and technology
Introduction :
Materials and methods :
Results :
Conclusion :
Oyewola David Opeoluwa, Dada Emmanuel Gbenga, Misra Sanjay
2022
COVID-19, Elastic net (ENET), Gaussian process (GAUSS), Machine learning, Spikes and slab (SPIKES), Vaccination
Using Natural Language Processing to Explore Mental Health Insights From UK Tweets During the COVID-19 Pandemic: Infodemiology Study.
In JMIR infodemiology
Background :
Objective :
Methods :
Results :
Conclusions :
Marshall Christopher, Lanyi Kate, Green Rhiannon, Wilkins Georgina C, Pearson Fiona, Craig Dawn
COVID-19, Twitter, artificial intelligence, lockdown, machine learning, mental health, natural language processing, sentiment, soft intelligence
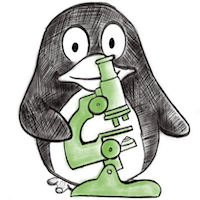
The need for measurement science in digital pathology.
In Journal of pathology informatics ; h5-index 23.0
Background :
Methods :
Findings :
Conclusions :
Romanchikova Marina, Thomas Spencer, Dexter Alex, Shaw Mike, Partarrieau Ignacio, Smith Nadia, Venton Jenny, Adeogun Michael, Brettle David, Turpin Robert James
2022-Nov-10
Artificial intelligence, Calibration, DICOM, Digital pathology, FAIR principles, Machine learning, Metadata, Metrology, Standards, Whole slide imaging
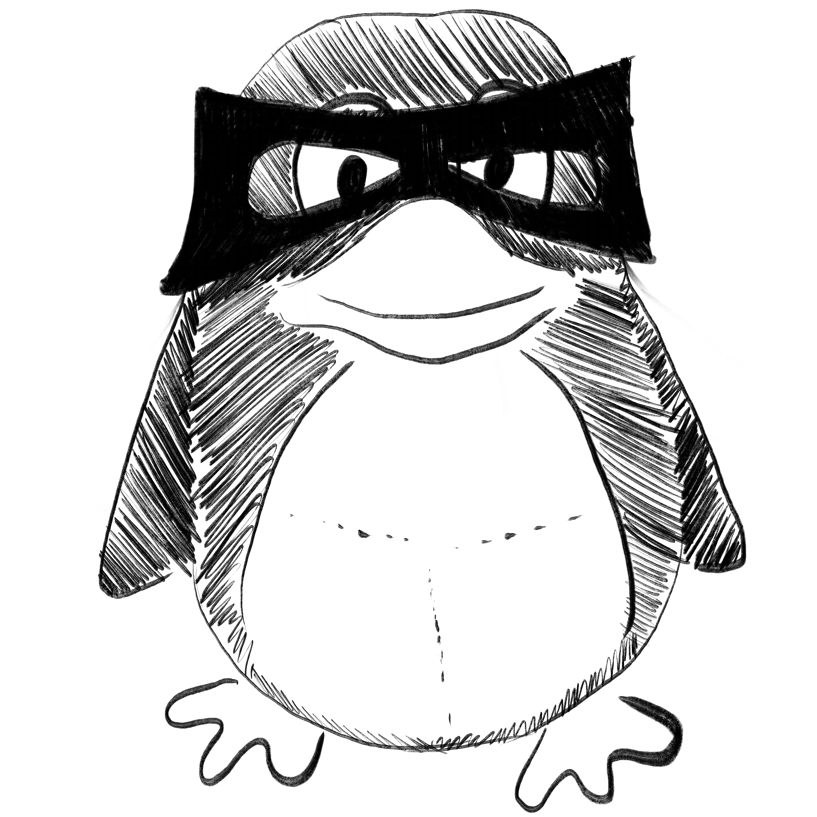
Using machine learning models to predict the duration of the recovery of COVID-19 patients hospitalized in Fangcang shelter hospital during the Omicron BA. 2.2 pandemic.
In Frontiers in medicine
Background :
Methods :
Results :
Conclusion :
Xu Yu, Ye Wei, Song Qiuyue, Shen Linlin, Liu Yu, Guo Yuhang, Liu Gang, Wu Hongmei, Wang Xia, Sun Xiaorong, Bai Li, Luo Chunmei, Liao Tongquan, Chen Hao, Song Caiping, Huang Chunji, Wu Yazhou, Xu Zhi
2022
COVID-19, Fangcang shelter, machine learning model, omicron, vaccination
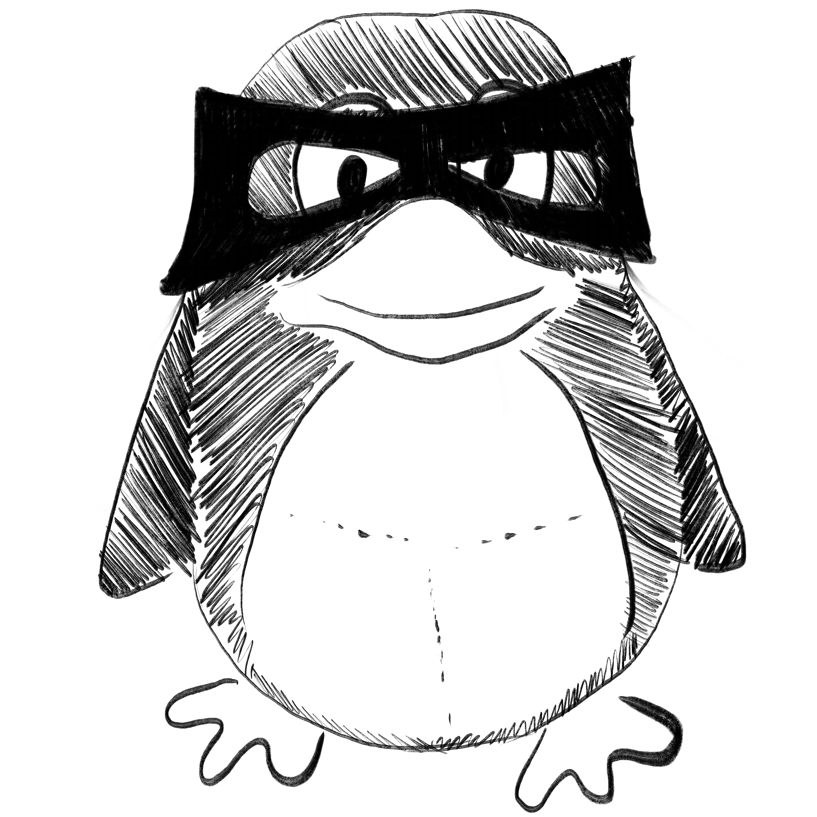
Efficient-ECGNet framework for COVID-19 classification and correlation prediction with the cardio disease through electrocardiogram medical imaging.
In Frontiers in medicine
Nawaz Marriam, Nazir Tahira, Javed Ali, Malik Khalid Mahmood, Saudagar Abdul Khader Jilani, Khan Muhammad Badruddin, Abul Hasanat Mozaherul Hoque, AlTameem Abdullah, AlKhathami Mohammed
2022
COVID-19, ECG, Efficient-ECGNet, computer vision, deep learning, medical imaging
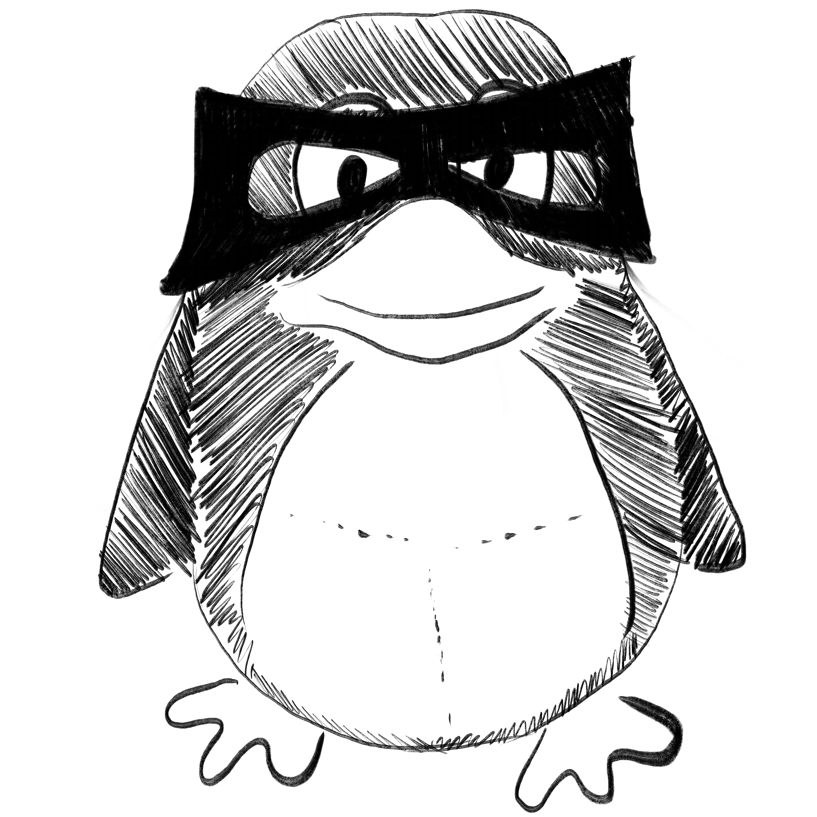
Monitoring the security of audio biomedical signals communications in wearable IoT healthcare.
In Digital communications and networks
Yazdanpanah Saeid, Chaeikar Saman Shojae, Jolfaei Alireza
2022-Nov-14
Audio security, Audio signal processing, Data hiding, Healthcare data, IoT security
Managing healthcare supply chain through Artificial Intelligence (AI): A study of critical success factors.
In Computers & industrial engineering
Kumar Ashwani, Mani Venkatesh, Jain Vranda, Gupta Himanshu, Venkatesh V G
2022-Nov-15
Artificial Intelligence, Healthcare, SWARA, supply chain
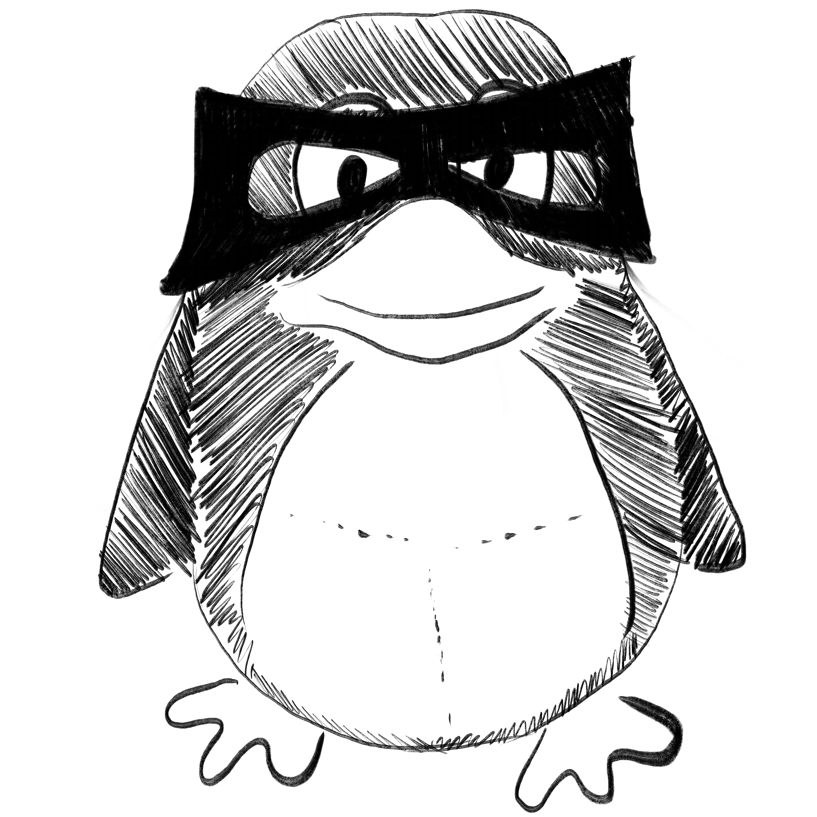
On the Frontlines: The Impact of the COVID-19 Pandemic on Social Workers' Well-Being.
In Social work
Schwartz Tayri Talia Meital
2022-Nov-21
COVID-19 pandemic, machine learning emotion detection, moral distress, moral injury, social work services
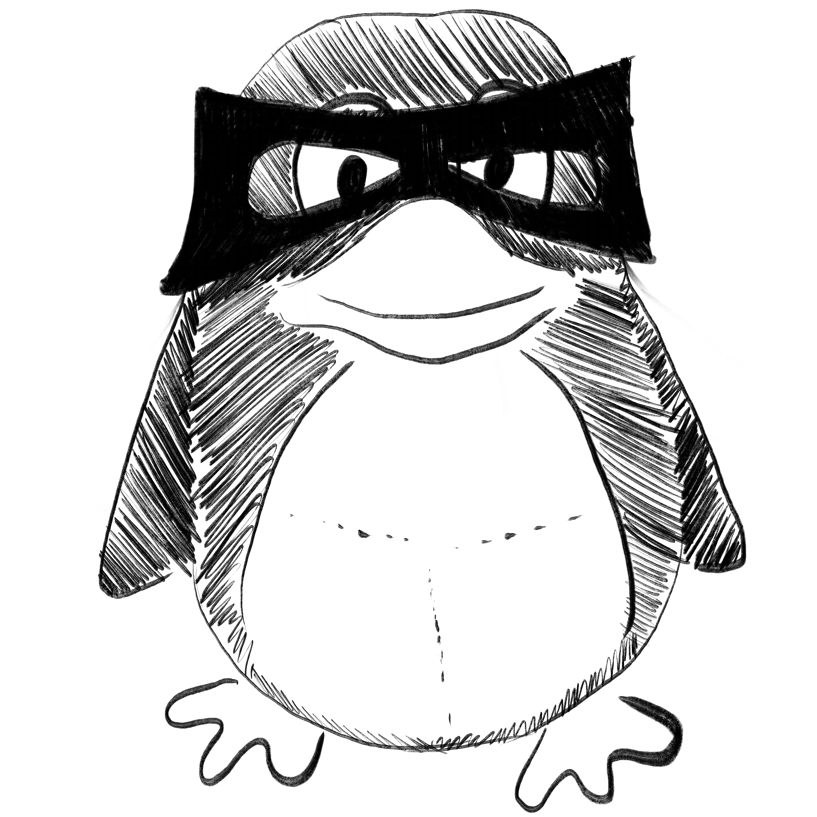
Performance drift in a mortality prediction algorithm among patients with cancer during the SARS-CoV-2 pandemic.
In Journal of the American Medical Informatics Association : JAMIA
Parikh Ravi B, Zhang Yichen, Kolla Likhitha, Chivers Corey, Courtright Katherine R, Zhu Jingsan, Navathe Amol S, Chen Jinbo
2022-Nov-21
SARS-CoV-2, algorithm drift, cancer, machine learning, mortality
SARS-CoV-2 seroprevalence, cumulative infections, and immunity to symptomatic infection - A multistage national household survey and modelling study, Dominican Republic, June-October 2021.
In Lancet regional health. Americas
Background :
Methods :
Findings :
Interpretation :
Funding :
Nilles Eric J, Paulino Cecilia Then, de St Aubin Michael, Restrepo Angela Cadavid, Mayfield Helen, Dumas Devan, Finch Emilie, Garnier Salome, Etienne Marie Caroline, Iselin Louisa, Duke William, Jarolim Petr, Oasan Timothy, Yu Jingyou, Wan Huahua, Peña Farah, Iihoshi Naomi, Abdalla Gabriela, Lopez Beatriz, Cruz Lucia de la, Henríquez Bernarda, Espinosa-Bode Andres, Puello Yosanly Cornelio, Durski Kara, Baldwin Margaret, Baez Amado Alejandro, Merchant Roland C, Barouch Dan H, Skewes-Ramm Ronald, Gutiérrez Emily Zielinski, Kucharski Adam, Lau Colleen L
2022-Dec
Novel favipiravir pattern-based learning model for automated detection of specific language impairment disorder using vowels.
In Neural computing & applications
Barua Prabal Datta, Aydemir Emrah, Dogan Sengul, Erten Mehmet, Kaysi Feyzi, Tuncer Turker, Fujita Hamido, Palmer Elizabeth, Acharya U Rajendra
2022-Nov-13
Favipiravir pattern, Molecular graph-based feature extraction, Specific language impairment, Vowel-based disease diagnosis
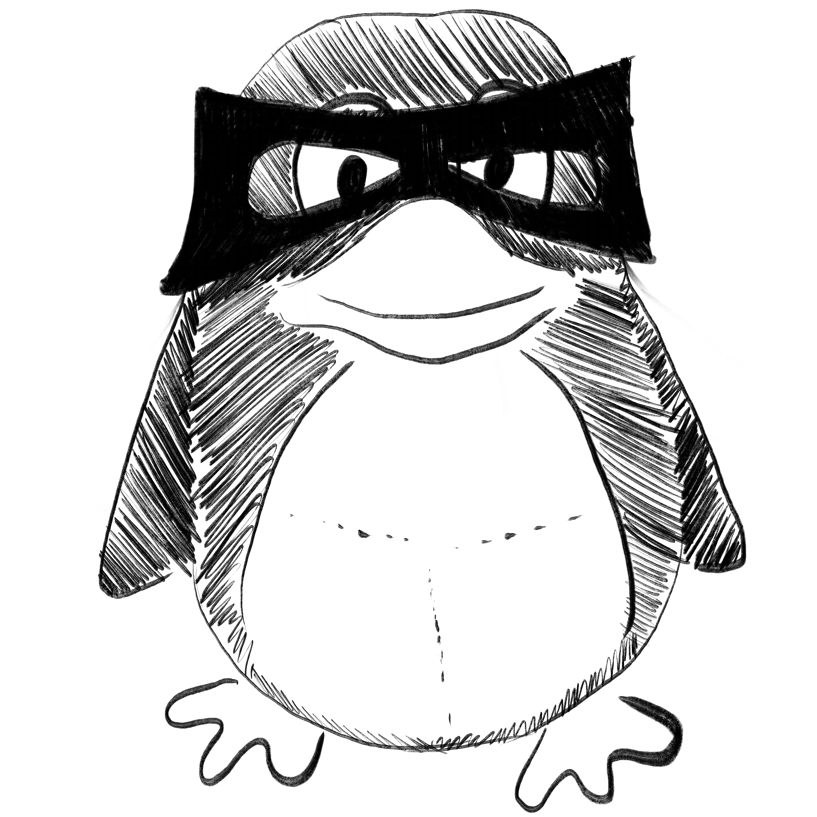
Framework for detection of probable clues to predict misleading information proliferated during COVID-19 outbreak.
In Neural computing & applications
Varshney Deepika, Vishwakarma Dinesh Kumar
2022-Nov-13
COVID-19, Fake news detection, Information pollution
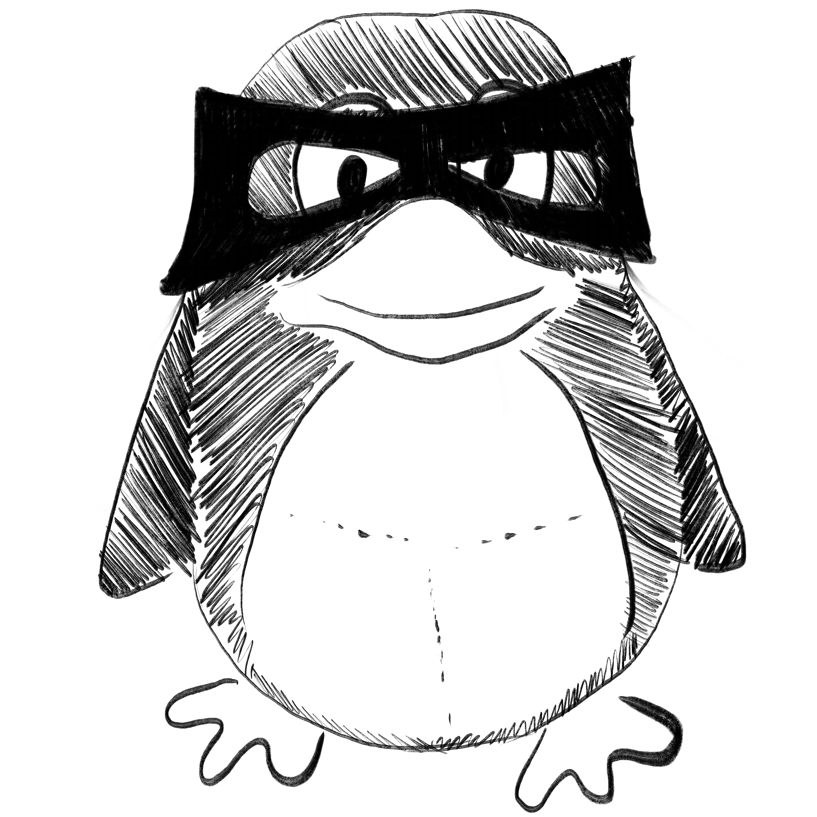
The COVID-19 Pandemic and Mental Health Concerns on Twitter in the United States.
In Health data science
Background :
Methods :
Results :
Conclusions :
Zhang Senqi, Sun Li, Zhang Daiwei, Li Pin, Liu Yue, Anand Ajay, Xie Zidian, Li Dongmei
2022
COVID-19 classification using chest X-ray images based on fusion-assisted deep Bayesian optimization and Grad-CAM visualization.
In Frontiers in public health
Hamza Ameer, Attique Khan Muhammad, Wang Shui-Hua, Alhaisoni Majed, Alharbi Meshal, Hussein Hany S, Alshazly Hammam, Kim Ye Jin, Cha Jaehyuk
2022
Bayesian optimization, corona virus, deep learning, fusion, hyperparameters, multi-filters contrast enhancement
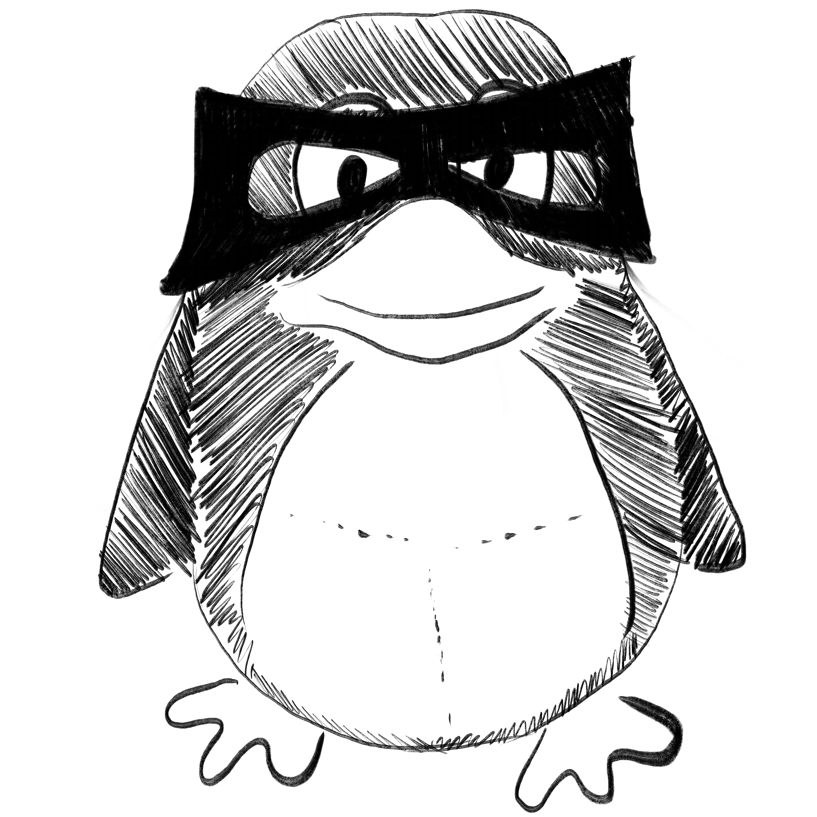
How Does Misinformation and Capricious Opinions Impact the Supply Chain - A Study on the Impacts During the Pandemic.
In Annals of operations research
Kar Arpan Kumar, Tripathi Shalini Nath, Malik Nishtha, Gupta Shivam, Sivarajah Uthayasankar
2022-Nov-07
Consumer buying behavior, Consumer spending, Fake news, Hoarding, Supply chain disruptions
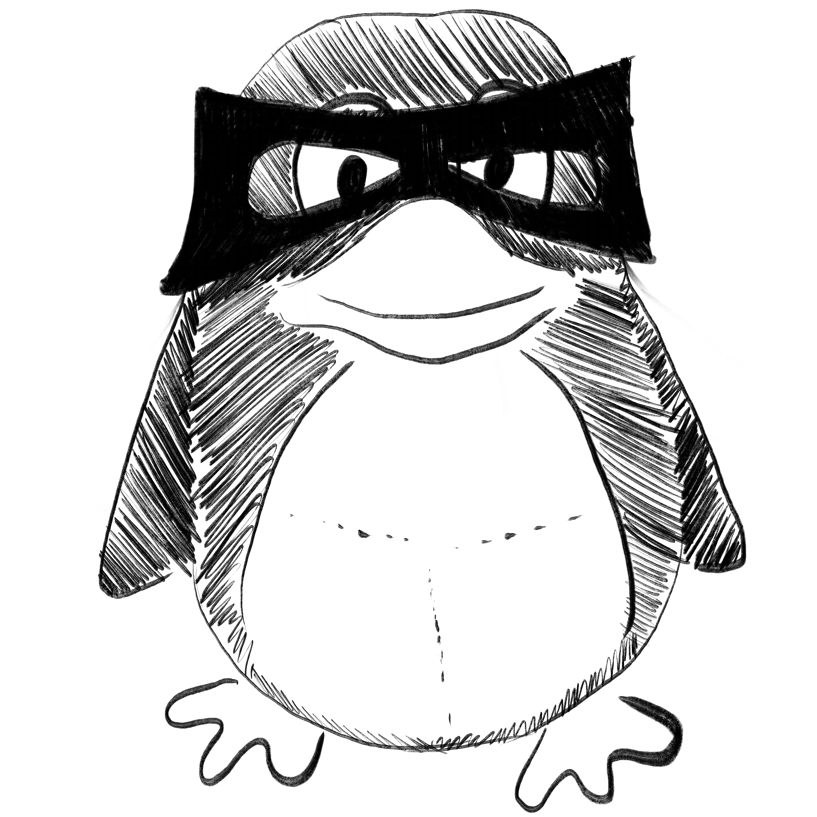
Were ride-hailing fares affected by the COVID-19 pandemic? Empirical analyses in Atlanta and Boston.
In Transportation
Silveira-Santos Tulio, González Ana Belén Rodríguez, Rangel Thais, Pozo Rubén Fernández, Vassallo Jose Manuel, Díaz Juan José Vinagre
2022-Nov-10
COVID-19, Dynamic Pricing, Machine Learning, Ride-Hailing, Time Series Forecasting, Transport Policy
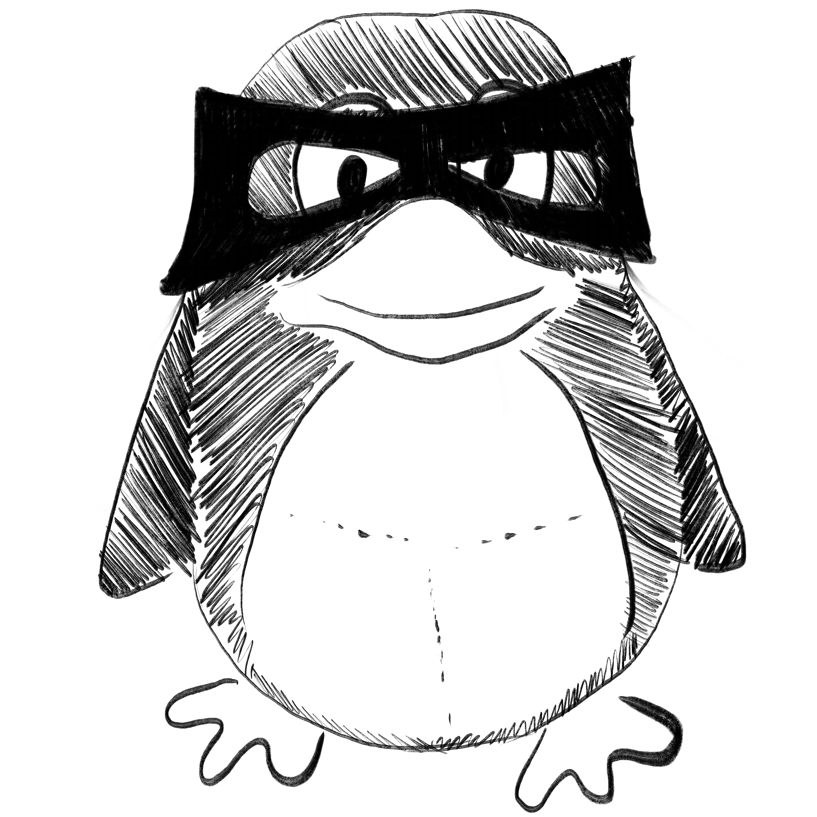
Face-mask-aware Facial Expression Recognition based on Face Parsing and Vision Transformer.
In Pattern recognition letters
Yang Bo, Wu Jianming, Ikeda Kazushi, Hattori Gen, Sugano Masaru, Iwasawa Yusuke, Matsuo Yutaka
2022-Dec
41A05, 41A10, 65D05, 65D17, Covid-19, Deep learning, Face mask, Face parsing, Facial expression recognition, Vision transformer
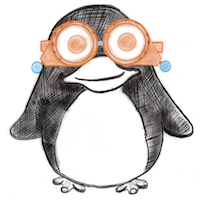
The Role of Technology in Ophthalmic Surgical Education During COVID-19.
In Current surgery reports
Purpose of Review :
Recent Findings :
Summary :
Hu Katherine S, Pettey Jeff, SooHoo Jeffrey R
2022-Nov-14
Ophthalmology training, Remote learning, Surgical simulators, Virtual education, Wet lab curriculum
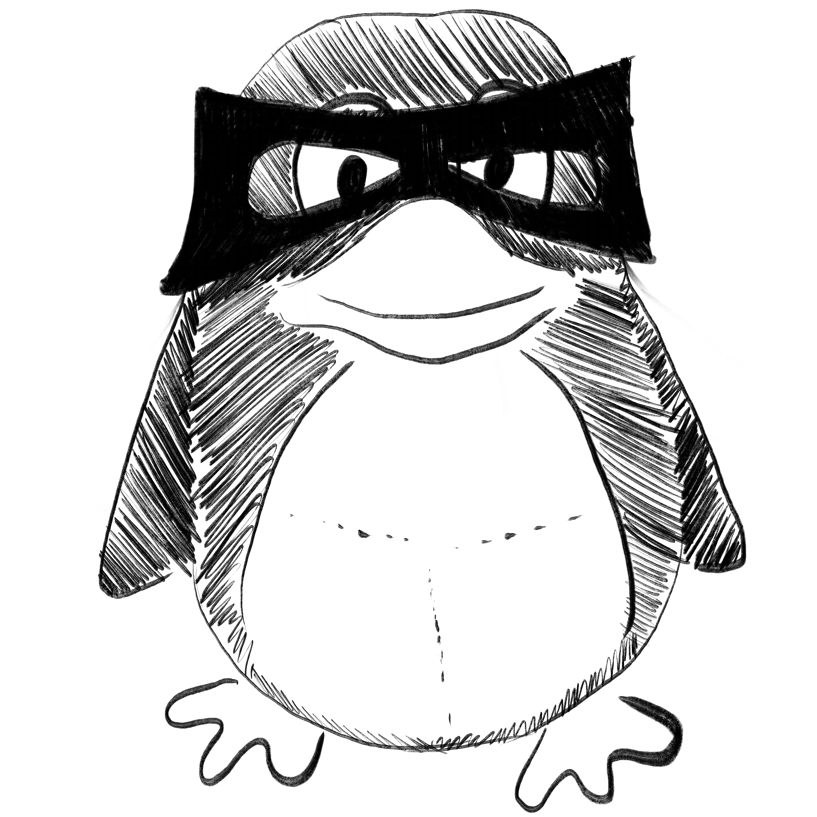
ETCNN: Extra Tree and Convolutional Neural Network-based Ensemble Model for COVID-19 Tweets Sentiment Classification.
In Pattern recognition letters
Umer Muhammad, Sadiq Saima, Karamti Hanen, Abdulmajid Eshmawi Ala’, Nappi Michele, Usman Sana Muhammad, Ashraf Imran
2022-Dec
COVID-19, Ensemble model, Health informatics, Neuroinformatics, Sentiment analysis
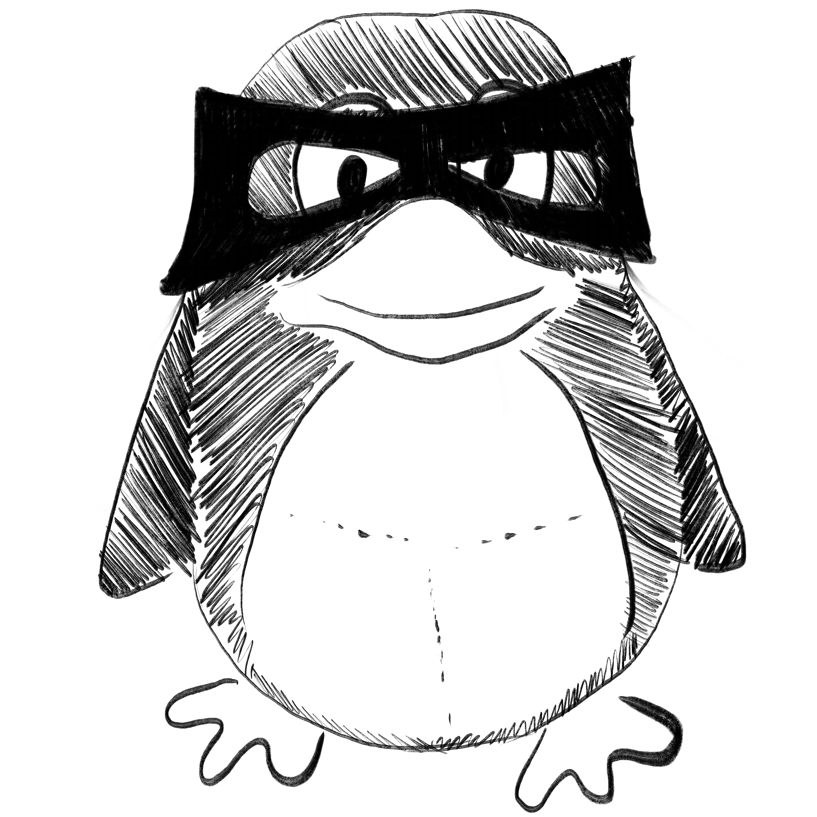
Fruit-CoV: An efficient vision-based framework for speedy detection and diagnosis of SARS-CoV-2 infections through recorded cough sounds.
In Expert systems with applications
Nguyen Long H, Pham Nhat Truong, Do Van Huong, Nguyen Liu Tai, Nguyen Thanh Tin, Nguyen Hai, Nguyen Ngoc Duy, Nguyen Thanh Thi, Nguyen Sy Dzung, Bhatti Asim, Lim Chee Peng
2023-Mar-01
COVID-19, Deep learning, Delta variant, EfficientNet, Log-Mel spectrogram, Machine vision, Neural network, PANNs, Recorded cough sounds, Remote detection, SARS-CoV-2 infections, Self-testing service, Sound classification, Speedy detection, Wavegram
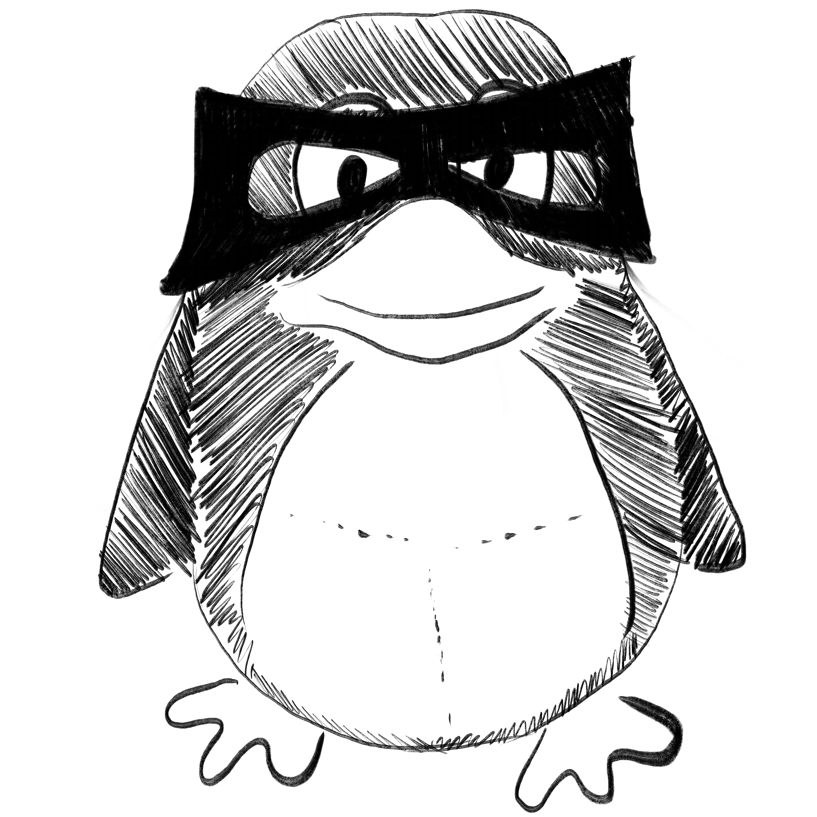
Cross-cultural factors influencing the adoption of virtual reality for practical learning.
In Universal access in the information society
Monteiro Diego, Ma Teng, Li Yue, Pan Zhigeng, Liang Hai-Ning
2022-Nov-15
COVID-19, Cross-cultural, Practical learning, Survey, Technology acceptance, Training, Virtual reality
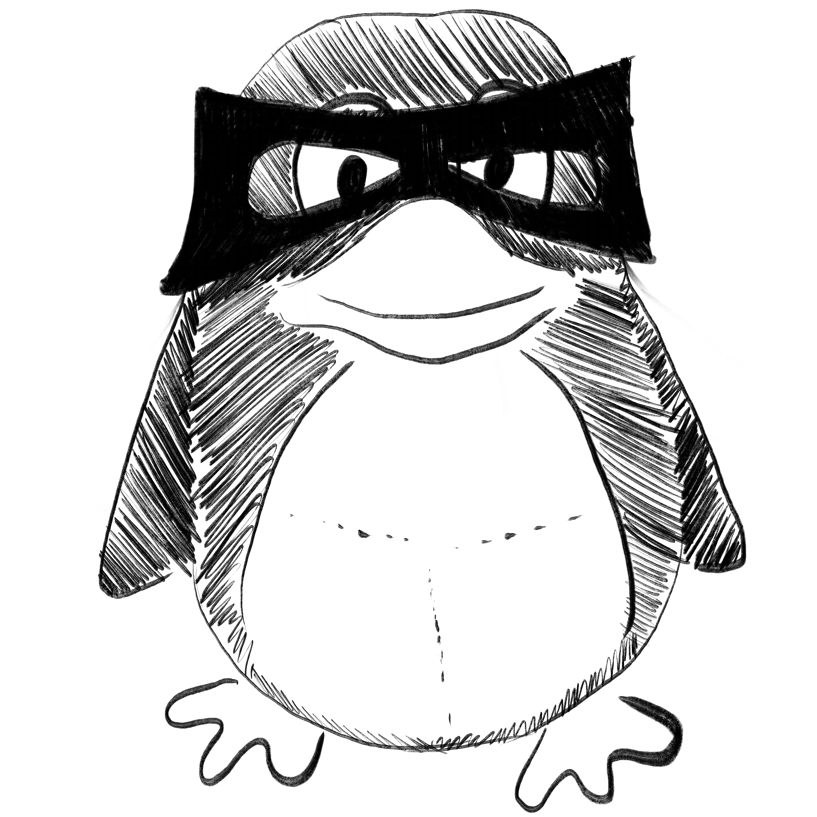
Is indoor and outdoor greenery associated with fewer depressive symptoms during COVID-19 lockdowns? A mechanistic study in Shanghai, China.
In Building and environment
Zhang Jinguang, Browning Matthew H E M, Liu Jie, Cheng Yingyi, Zhao Bing, Dadvand Payam
2023-Jan
Fear of COVID-19, Loneliness, Machine learning, Nature exposure, Visual access
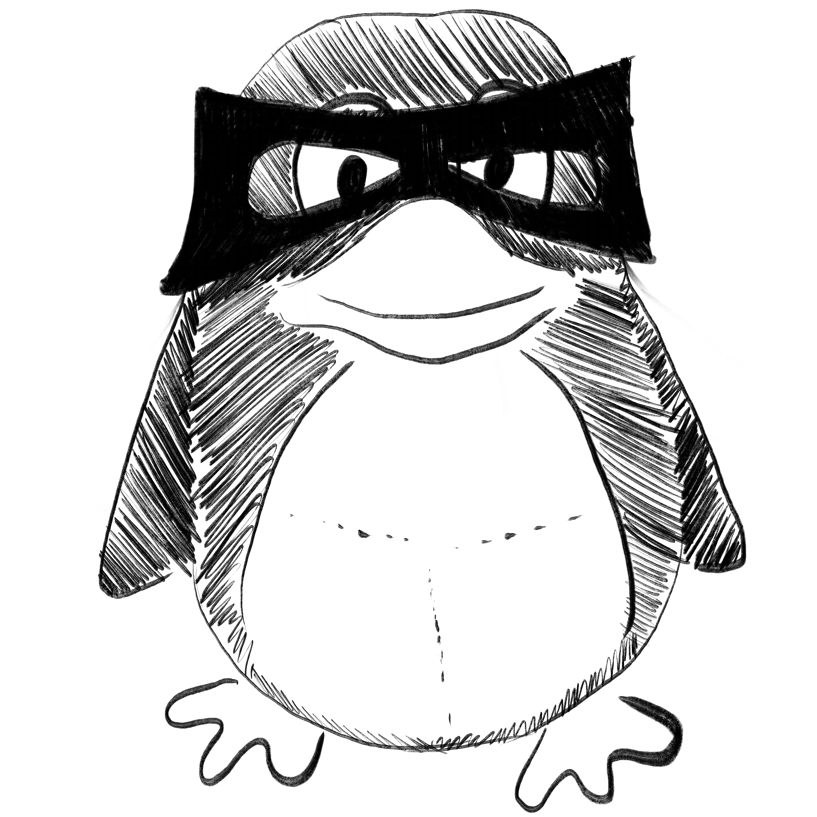
Time-delayed modelling of the COVID-19 dynamics with a convex incidence rate.
In Informatics in medicine unlocked
Babasola Oluwatosin, Kayode Oshinubi, Peter Olumuyiwa James, Onwuegbuche Faithful Chiagoziem, Oguntolu Festus Abiodun
2022
34D20, 37N25, 39A60, 92B05, COVID-19, Convex incidence rate, Delay differential equation, SEIR epidemic model, Stability
Emotion Analysis of COVID-19 Vaccines Based on a Fuzzy Convolutional Neural Network.
In Cognitive computation
Qiu Dong, Yu Yang, Chen Lei
2022-Nov-16
COVID-19 vaccines, Fuzzy convolutional neural network, Fuzzy emotion vector, Fuzzy logic, Sentiment analysis
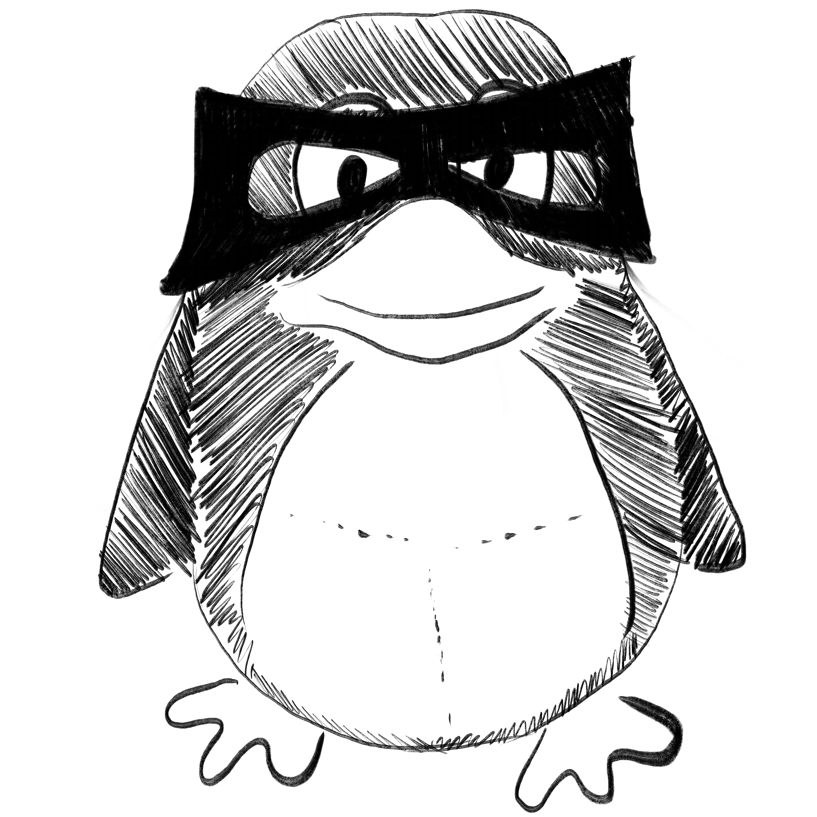
Enhanced Framework for COVID-19 Prediction with Computed Tomography Scan Images using Dense Convolutional Neural Network and Novel Loss Function.
In Computers & electrical engineering : an international journal
Motwani Anand, Shukla Piyush Kumar, Pawar Mahesh, Kumar Manoj, Ghosh Uttam, Numay Waleed Al, Nayak Soumya Ranjan
2022-Nov-14
COVID-19, Chest CT-images, Classification, Deep Learning, Dense-Convolutional Neural Network, Loss function, Optimization, Prediction, SARS-CoV-2
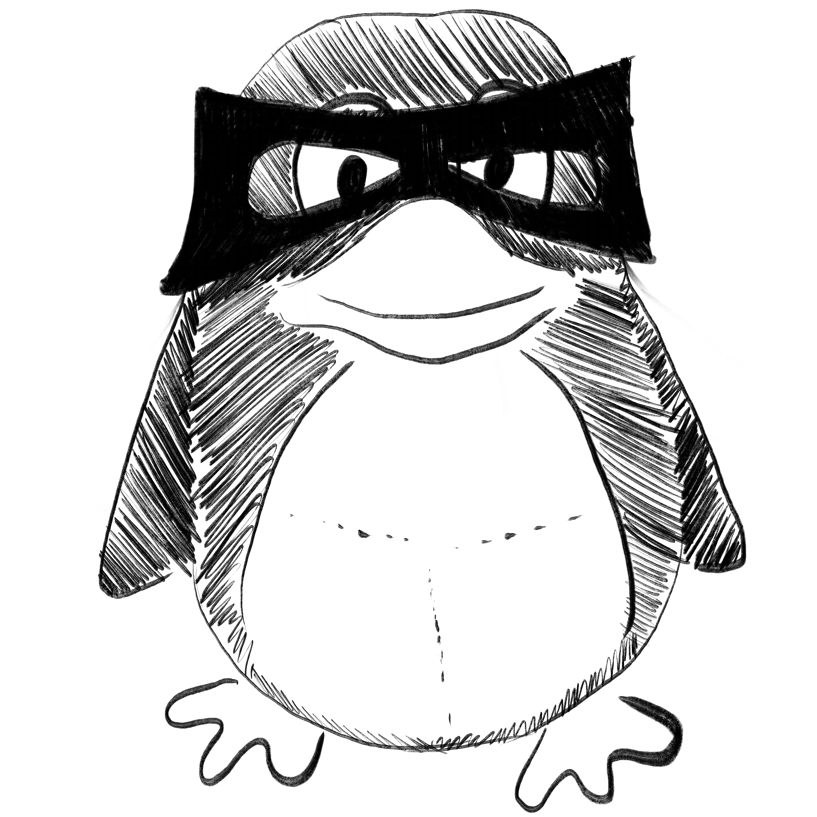
Comparative Evaluation of the Multilayer Perceptron Approach with Conventional ARIMA in Modeling and Prediction of COVID-19 Daily Death Cases.
In Journal of healthcare engineering
Qureshi Moiz, Daniyal Muhammad, Tawiah Kassim
2022
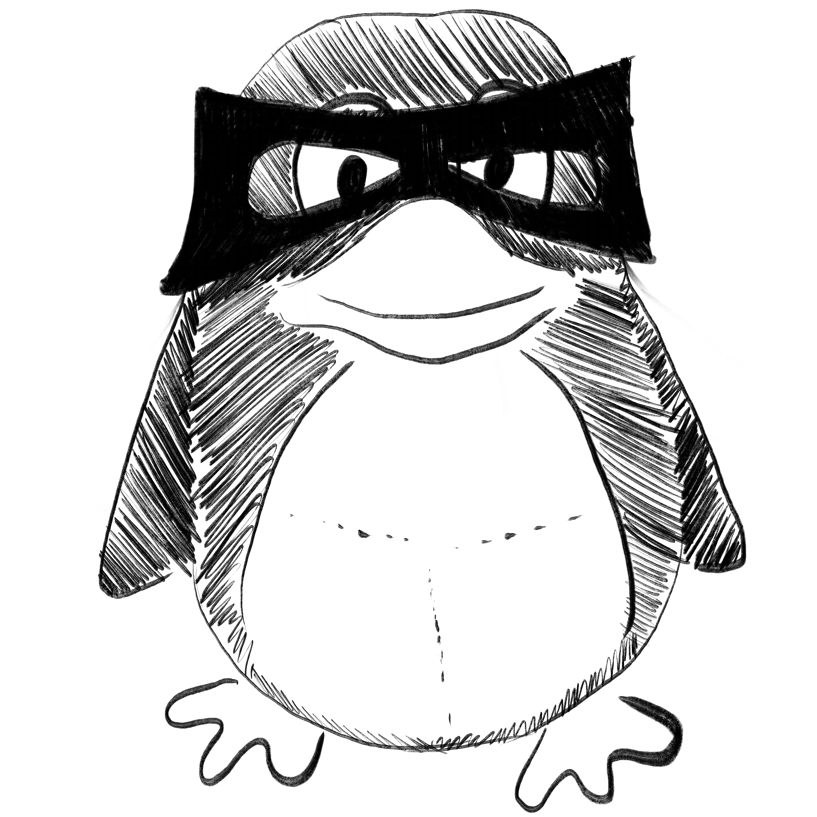
Automatic social distance estimation for photographic studies: Performance evaluation, test benchmark, and algorithm.
In Machine learning with applications
Seker Mert, Männistö Anssi, Iosifidis Alexandros, Raitoharju Jenni
2022-Nov-09
Human Pose Estimation, Performance Evaluation, Person detection, Proxemics, Social distance estimation, Test Benchmark
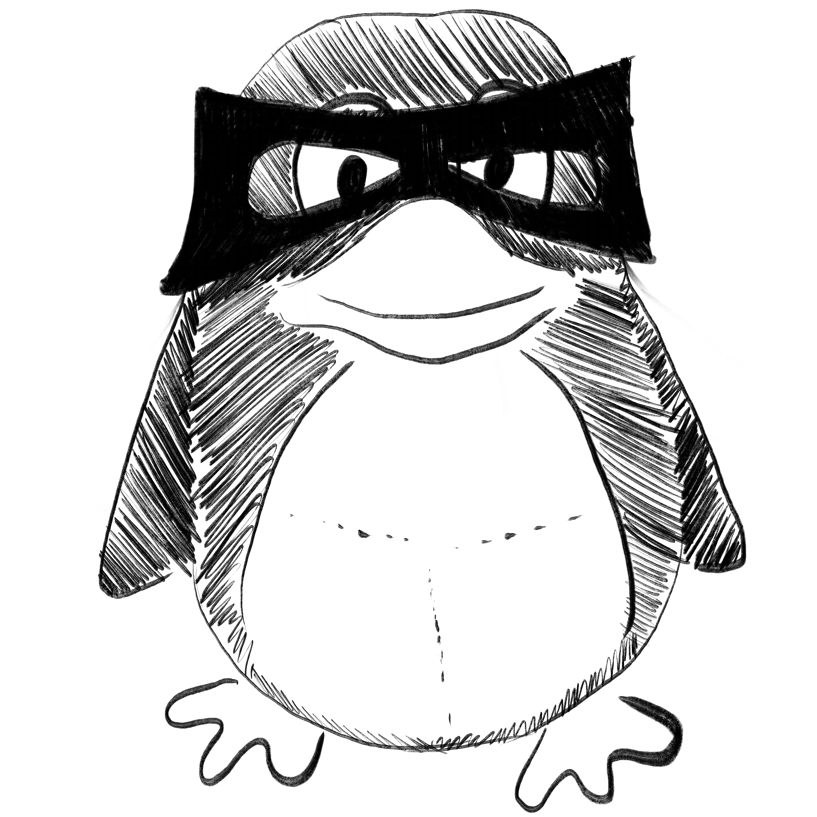
An automated multi-web platform voting framework to predict misleading information proliferated during COVID-19 outbreak using ensemble method.
In Data & knowledge engineering
Varshney Deepika, Vishwakarma Dinesh Kumar
2022-Nov-11
COVID-19, Fake news, Google, Machine learning, Misleading information, Multi-web platforms, YouTube
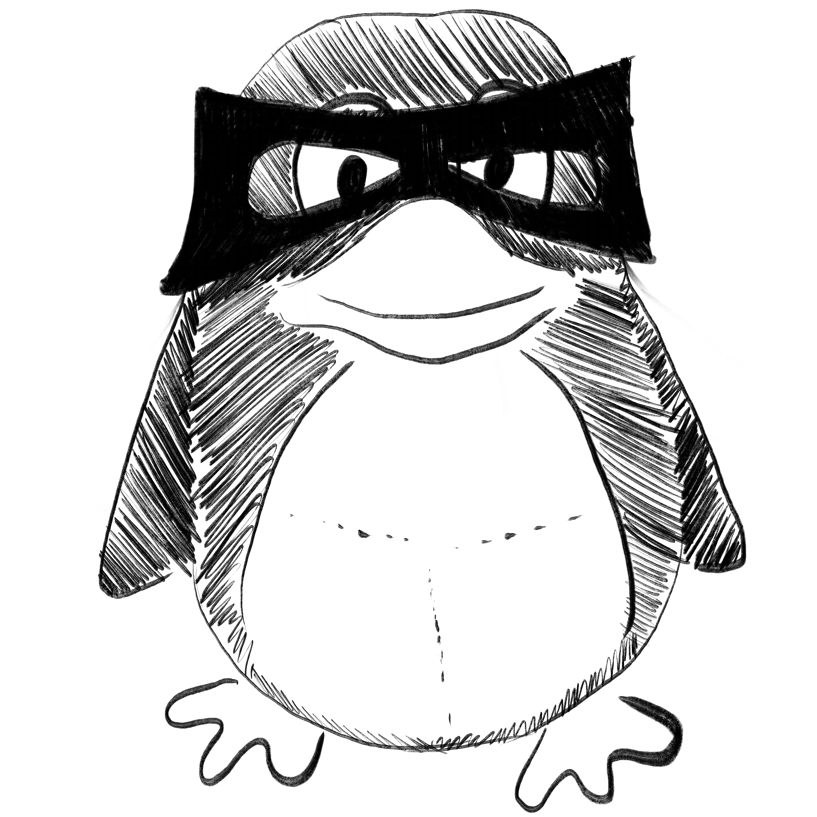
Bridging the Gap between Target-Based and Cell-Based Drug Discovery with a Graph Generative Multitask Model.
In Journal of chemical information and modeling
Hu Fan, Wang Dongqi, Huang Huazhen, Hu Yishen, Yin Peng
2022-Nov-19
Assessment of Oncology Patient Engagement and Interest in Virtual Mind-Body Programming: Moving Toward Personalization of Virtual Care.
In JCO oncology practice
PURPOSE :
METHODS :
RESULTS :
CONCLUSION :
Hung Tony K W, Latte-Naor Shelly, Li Yuelin, Kuperman Gilad J, Seluzicki Christina, Pendleton Eva, Pfister David G, Mao Jun J
2022-Nov-18
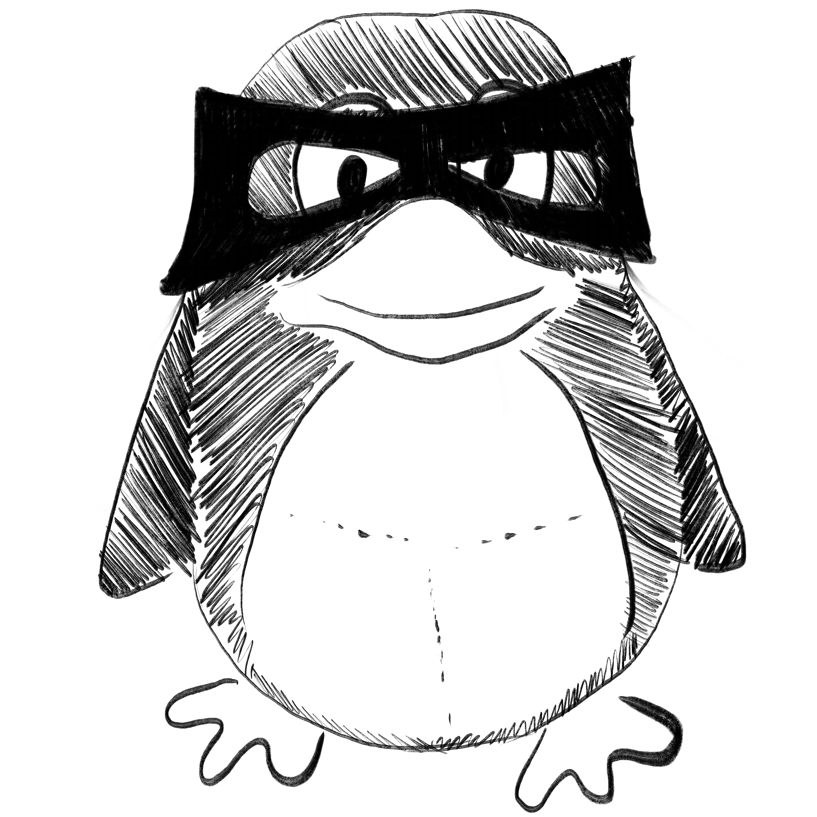
Artificial intelligence-based analytics for impacts of COVID-19 and online learning on college students' mental health.
In PloS one ; h5-index 176.0
Rezapour Mostafa, Elmshaeuser Scott K
2022
Utilizing an organizational development framework as a road map for creating a technology-driven agile curriculum in predoctoral dental education.
In Journal of dental education
Tadinada Aditya, Gul Gulsun, Godwin Lauren, Al Sakka Yacoub, Crain Geralyn, Stanford Clark M, Johnson Jeffrey
2022-Nov-18
computer-assisted instruction, curriculum innovation, dental education, institutional/organizational development, patient simulation
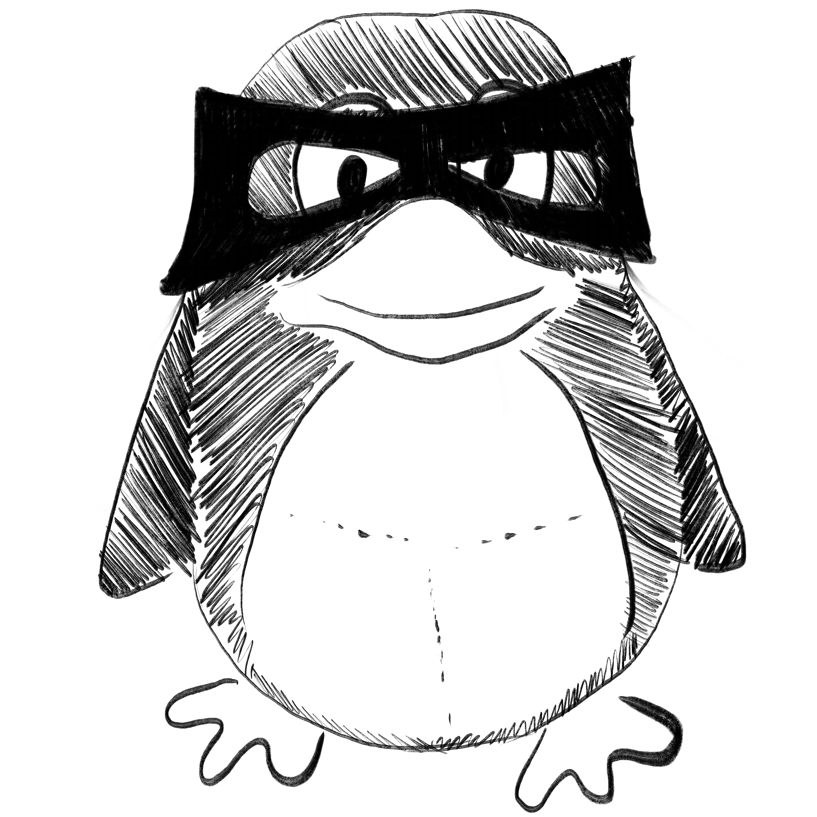
Perceived usefulness of COVID-19 tools for contact tracing among contact tracers in Korea.
In Epidemiology and health
Objectives :
Methods :
Results :
Conclusion :
Gong Seonyeong, Moon Jong Youn, Jung Jaehun
2022-Nov-15
COVID-19, Contact tracing, Entry log, KI-Pass
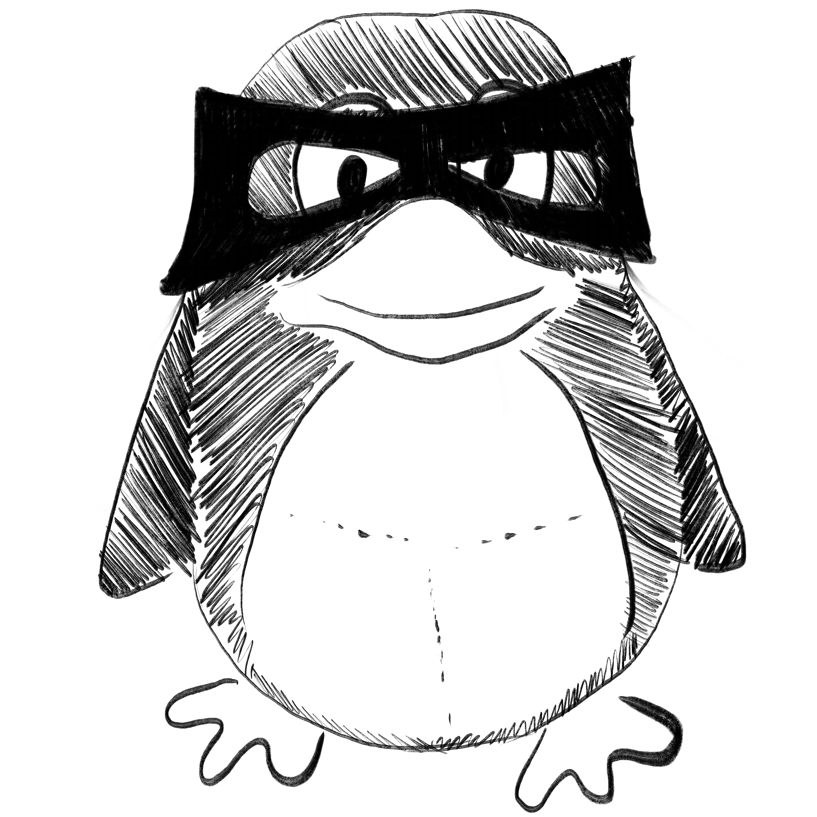
Cell-type annotation with accurate unseen cell-type identification using multiple references
bioRxiv Preprint
Yixuan, X.; Mengguo, W.; Luonan, C.; Xiaofei, Z.
2022-11-18
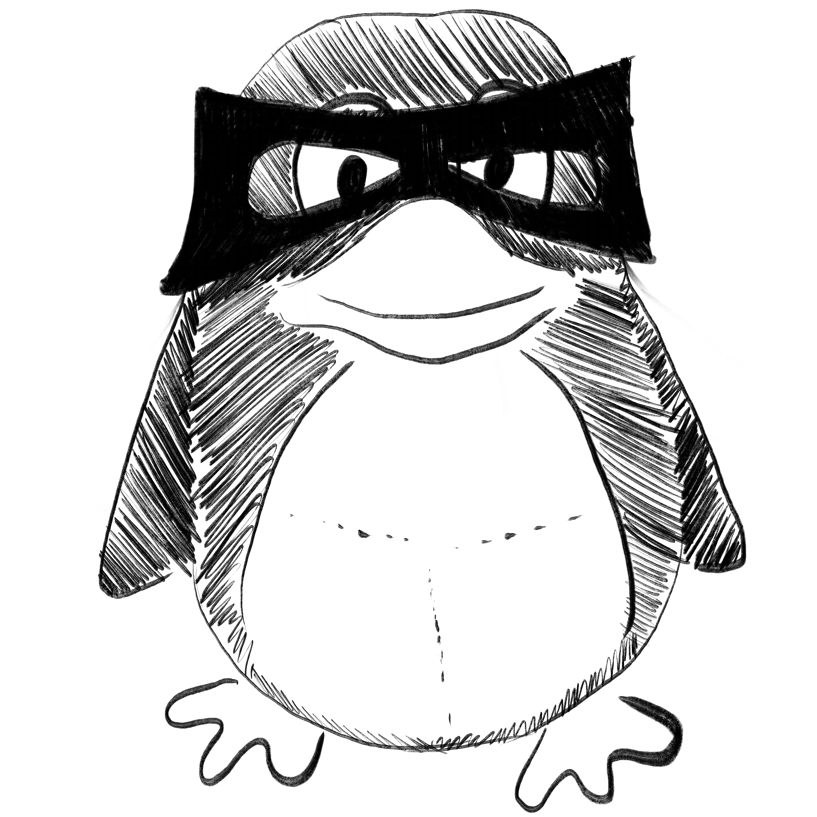
Persistent Laplacian projected Omicron BA.4 and BA.5 to become new dominating variants.
In Computers in biology and medicine
Chen Jiahui, Qiu Yuchi, Wang Rui, Wei Guo-Wei
2022-Nov-02
Deep learning, Evolution, Infectivity, Persistent Laplacian, SARS-CoV-2
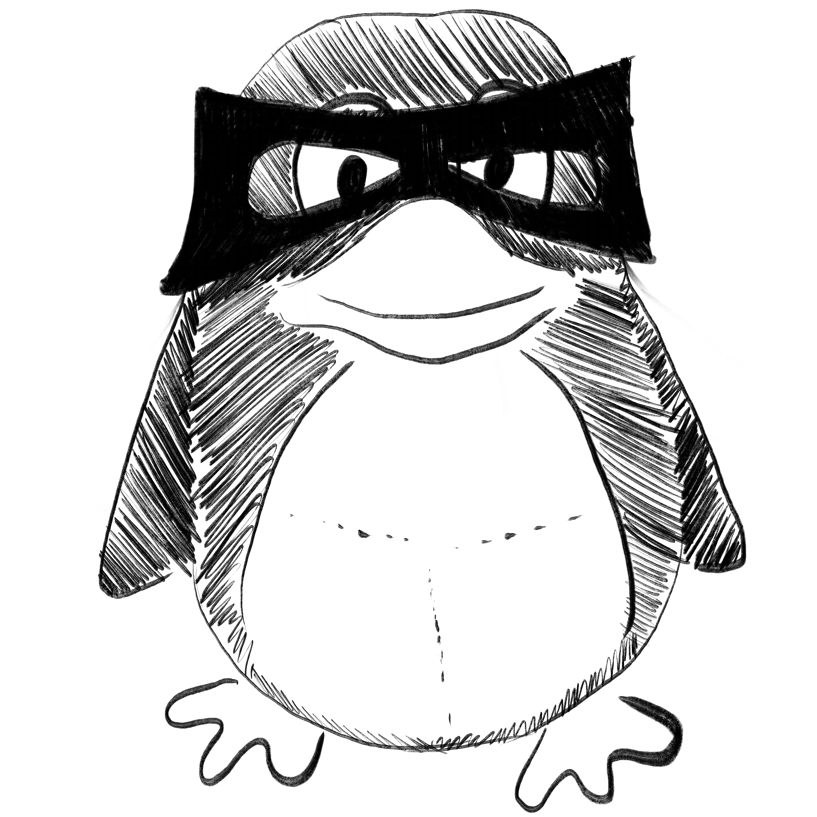
Recommendations for Successful Implementation of the Use of Vocal Biomarkers for Remote Monitoring of COVID-19 and Long COVID in Clinical Practice and Research.
In Interactive journal of medical research
Fischer Aurelie, Elbeji Abir, Aguayo Gloria, Fagherazzi Guy
2022-Nov-15
COVID-19, COVID-19 symptoms, Long COVID, artificial intelligence, digital health, digital health monitoring, digital health solution, health care application, health monitoring, health technology, remote monitoring, remote patient monitoring, vocal biomarker, voice, voice-based technology
Cardiovascular CT, MRI, and PET/CT in 2021: Review of Key Articles.
In Radiology ; h5-index 91.0
Tzimas Georgios, Ryan David T, Murphy David J, Leipsic Jonathon A, Dodd Jonathan D
2022-Nov-15
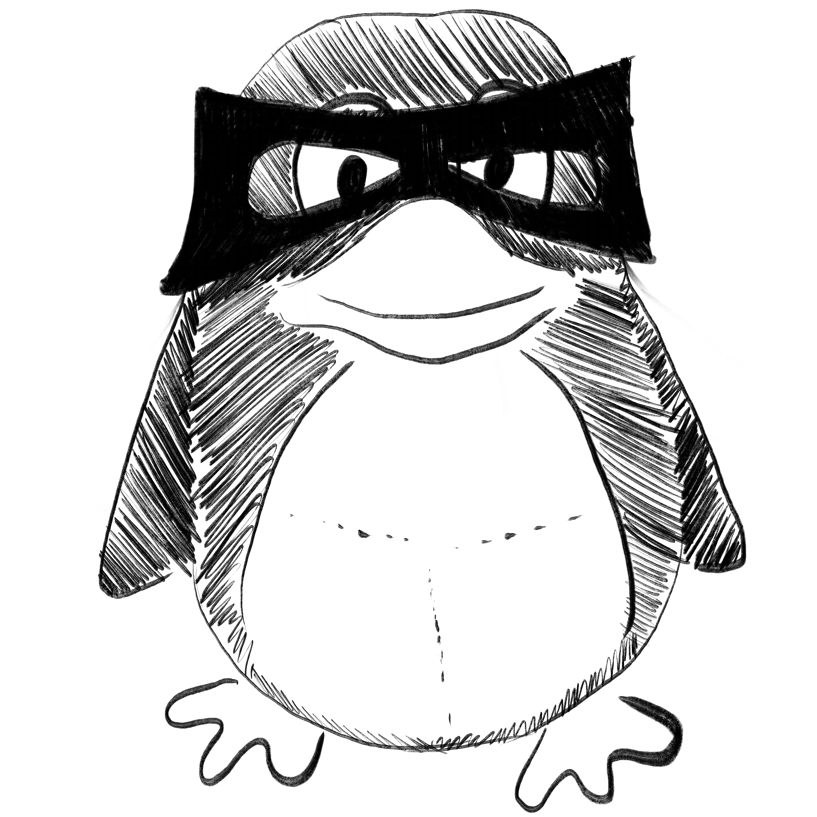
Symptom Clusters Seen in Adult COVID-19 Recovery Clinic Care Seekers.
In Journal of general internal medicine ; h5-index 57.0
BACKGROUND :
OBJECTIVE :
DESIGN :
PATIENTS :
MAIN MEASURES :
KEY RESULTS :
CONCLUSIONS :
TRIAL REGISTRATION :
Danesh Valerie, Arroliga Alejandro C, Bourgeois James A, Boehm Leanne M, McNeal Michael J, Widmer Andrew J, McNeal Tresa M, Kesler Shelli R
2022-Nov-14
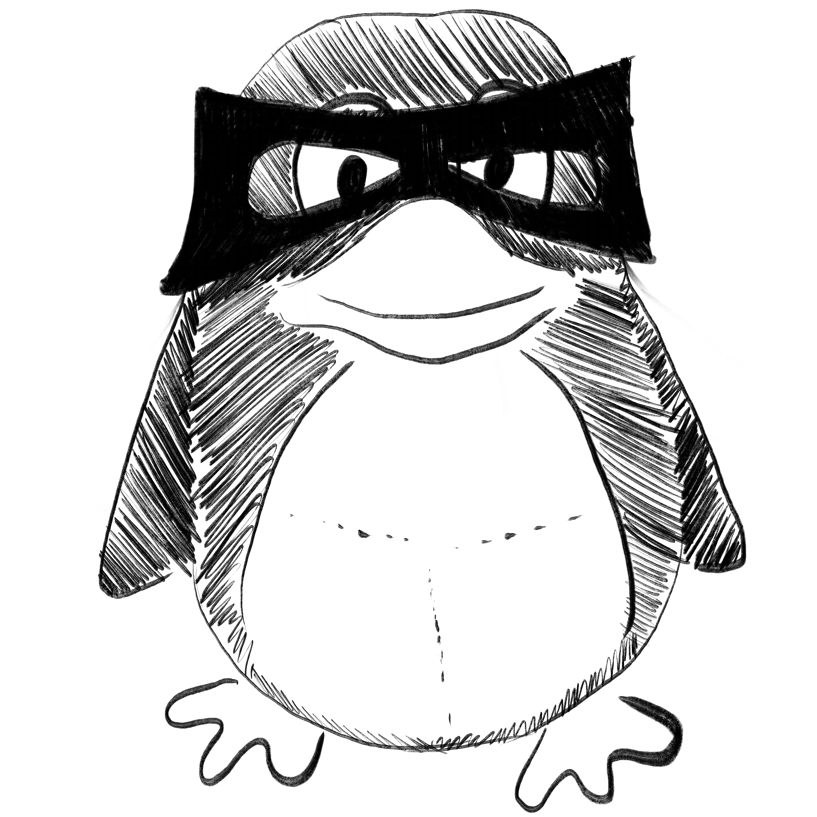
A Machine Learning Approach to Identify Predictors of Severe COVID-19 Outcome in Patients With Rheumatoid Arthritis.
In Pain physician ; h5-index 45.0
BACKGROUND :
OBJECTIVES :
STUDY DESIGN :
SETTING :
METHODS :
RESULTS :
LIMITATIONS :
CONCLUSIONS :
Burns Sara M, Woodworth TIffany S, Icten Zeynep, Honda Trenton, Manjourides Justin
2022-Nov
** RA, SARS-CoV-2, corticosteroid use\r, machine learning, physical therapy, predictive modeling, real-world data, real-world evidence, rheumatoid arthritis, COVID-19**
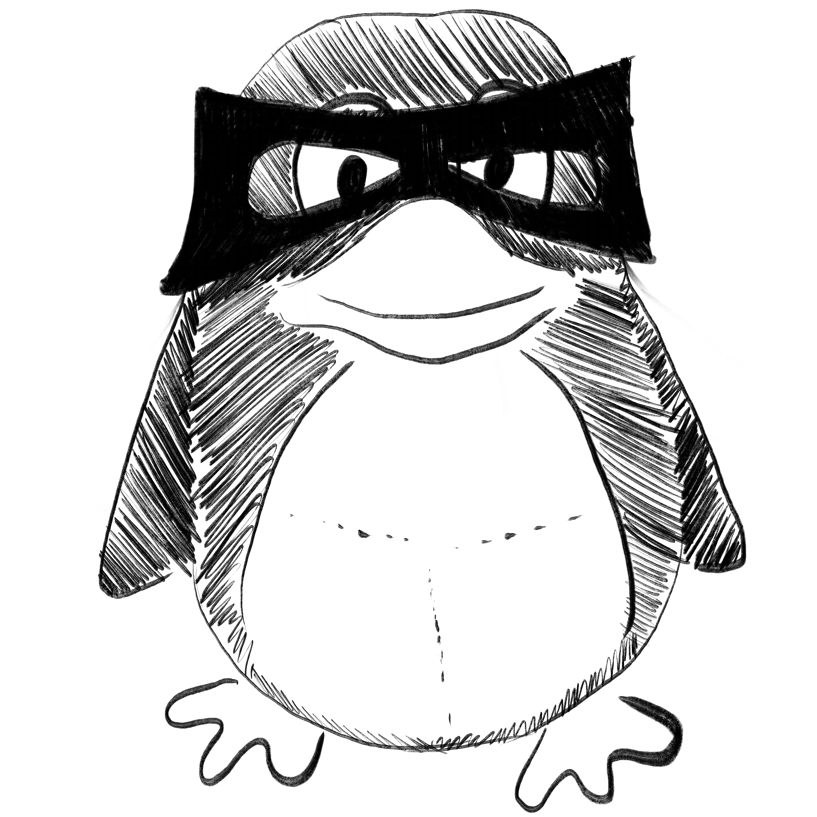
CNN Features and Optimized Generative Adversarial Network for COVID-19 Detection from Chest X-Ray Images.
In Critical reviews in biomedical engineering
Kalpana Gotlur, Durga A Kanaka, Karuna G
2022
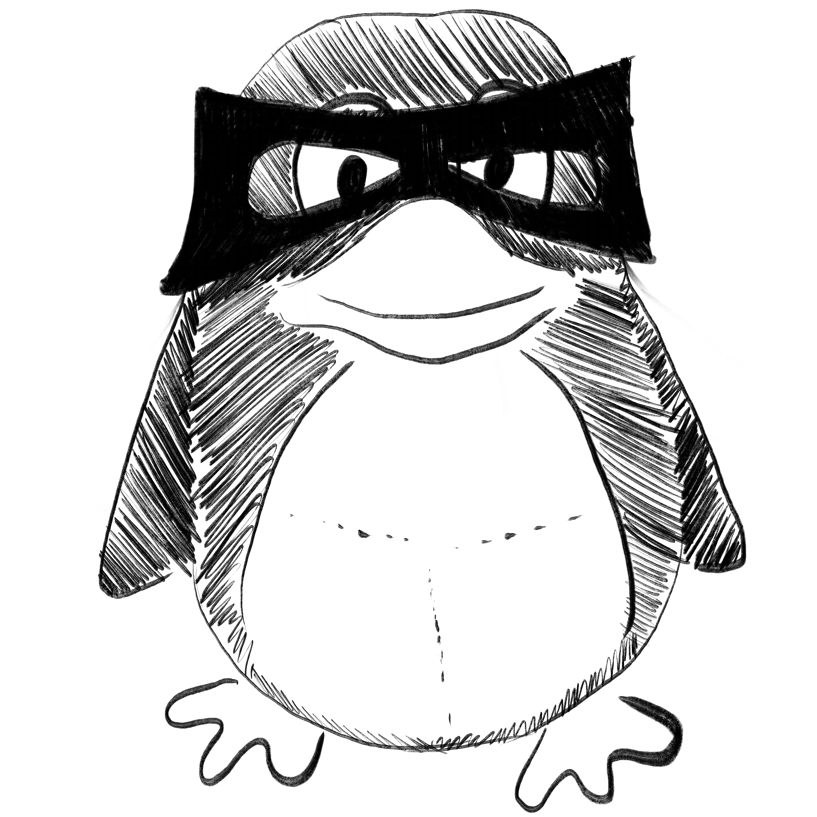
Interpretable tourism volume forecasting with multivariate time series under the impact of COVID-19.
In Neural computing & applications
Wu Binrong, Wang Lin, Tao Rui, Zeng Yu-Rong
2022-Nov-04
COVID-19, Deep learning, Interpretable tourism demand forecasting, Variational mode decomposition
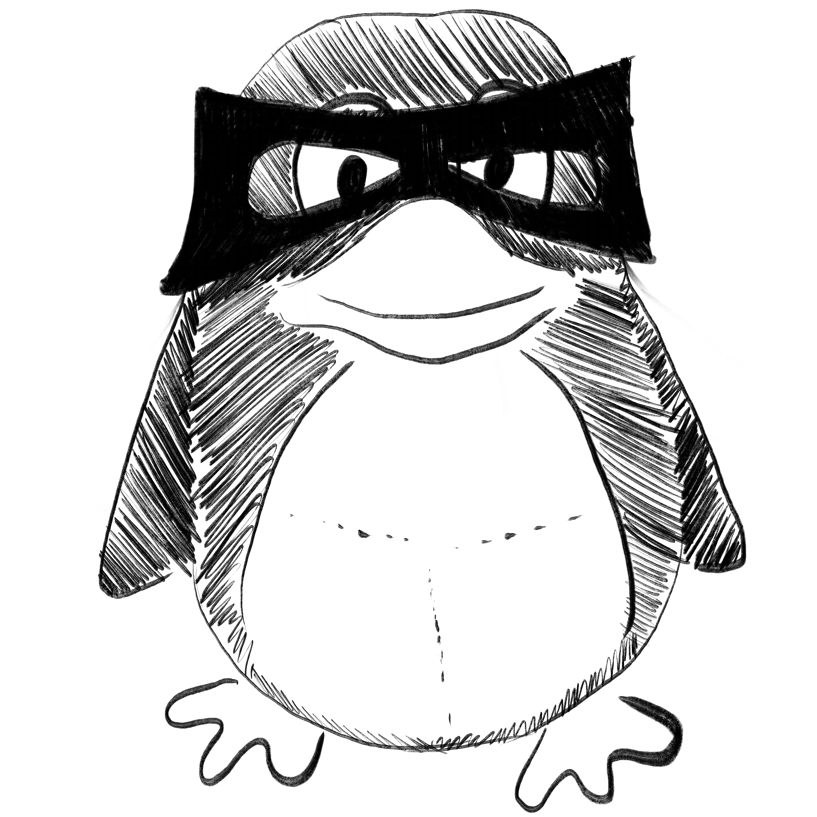
A survey on deep learning applied to medical images: from simple artificial neural networks to generative models.
In Neural computing & applications
Celard P, Iglesias E L, Sorribes-Fdez J M, Romero R, Vieira A Seara, Borrajo L
2022-Nov-04
Artificial neural networks, Computer vision, Convolutional neural networks, Generative adversarial networks, Medical imaging, Variational autoencoders
Practice of big data and artificial intelligence in epidemic surveillance and containment.
In Intelligent medicine
Jiao Zengtao, Ji Hanran, Yan Jun, Qi Xiaopeng
2022-Nov-05
Artificial intelligence, Big data, Early warning, Epidemic prevention and control, Epidemiological investigation
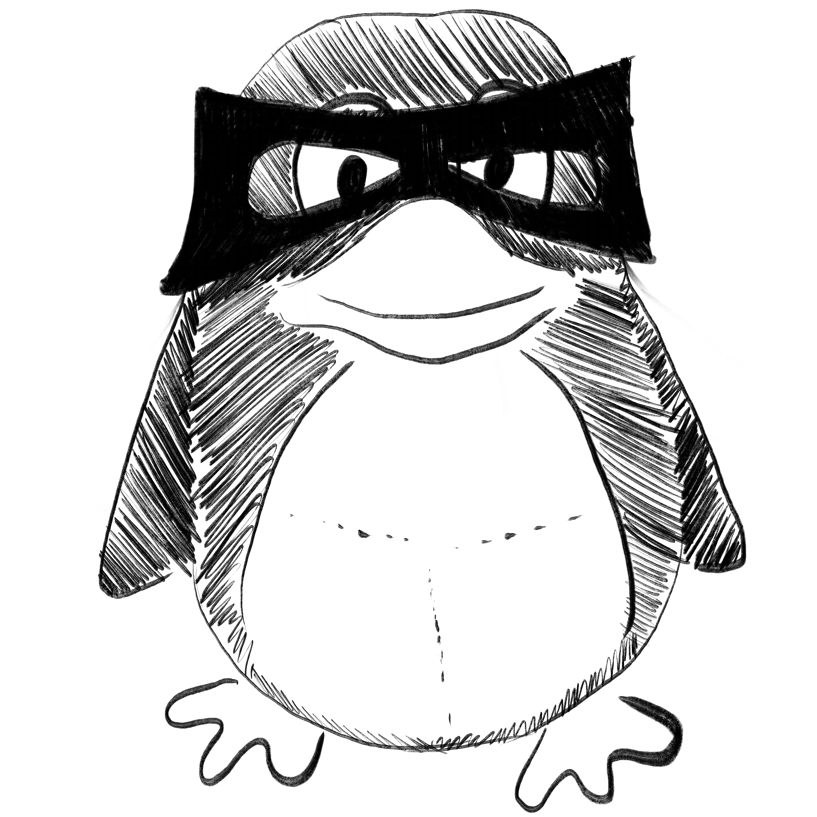
Designing Resilient Manufacturing Systems using Cross Domain Application of Machine Learning Resilience.
In Procedia CIRP
Mukherjee Avik, Glatt Moritz, Mustafa Waleed, Kloft Marius, Aurich Jan C
2022
adverserial attacks, adverserial training, deep neural networks, discrete-event simulation environment, machine learning, manufacturing system, resilience, supply network
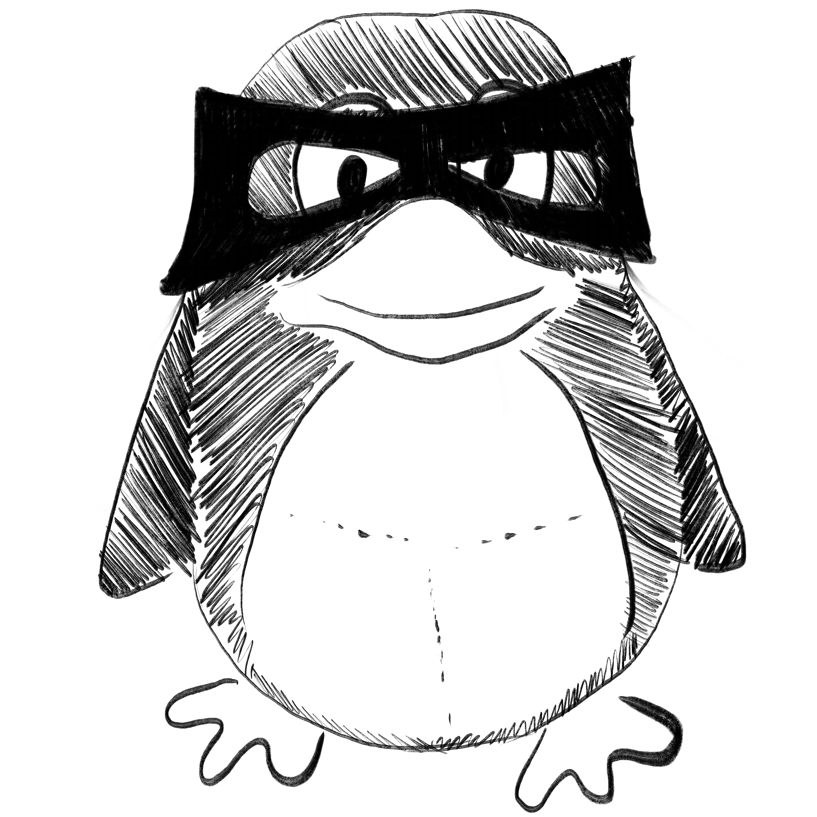
Estimating Discontinuous Time-Varying Risk Factors and Treatment Benefits for COVID-19 with Interpretable ML
ArXiv Preprint
Benjamin Lengerich, Mark E. Nunnally, Yin Aphinyanaphongs, Rich Caruana
2022-11-15
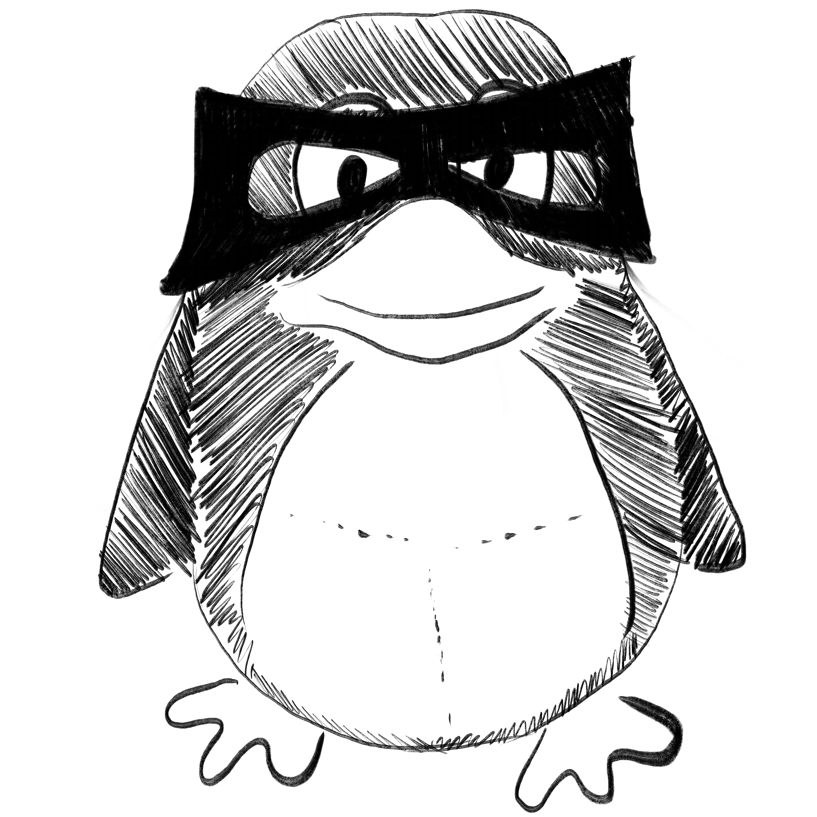
CXR-Net: A Multitask Deep Learning Network for Explainable and Accurate Diagnosis of COVID-19 Pneumonia from Chest X-ray Images.
In IEEE journal of biomedical and health informatics
Zhang Xin, Han Liangxiu, Sobeih Tam, Han Lianghao, Dempsey Nina, Lechareas Symeon, Tridente Ascanio, Chen Haoming, White Stephen, Zhang Daoqiang
2022-Nov-09
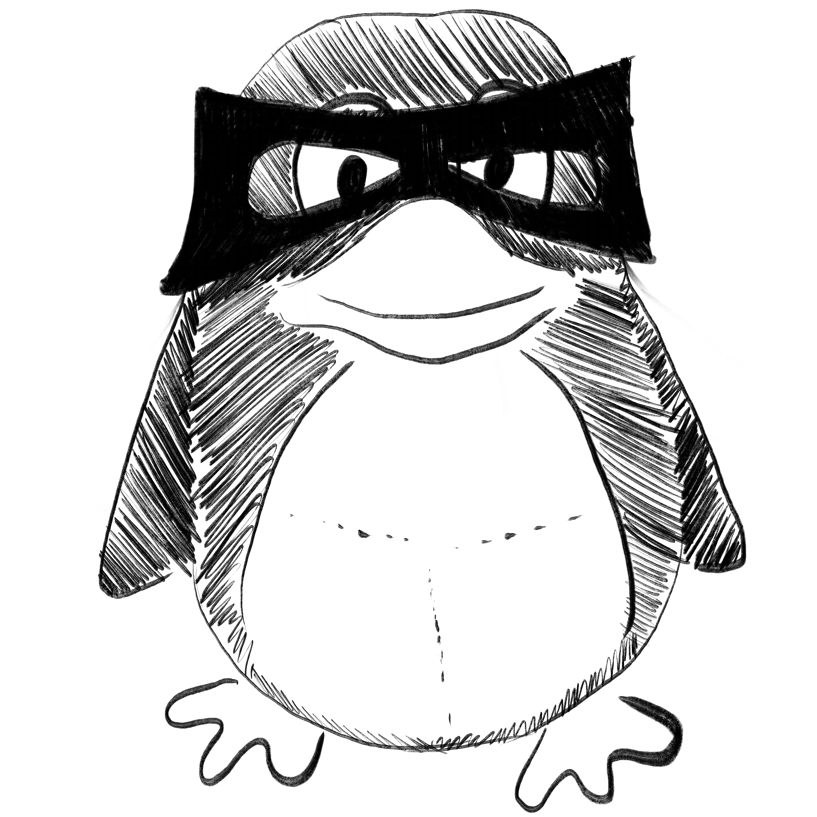
LitCovid in 2022: an information resource for the COVID-19 literature.
In Nucleic acids research ; h5-index 217.0
Chen Qingyu, Allot Alexis, Leaman Robert, Wei Chih-Hsuan, Aghaarabi Elaheh, Guerrerio John J, Xu Lilly, Lu Zhiyong
2022-Nov-09
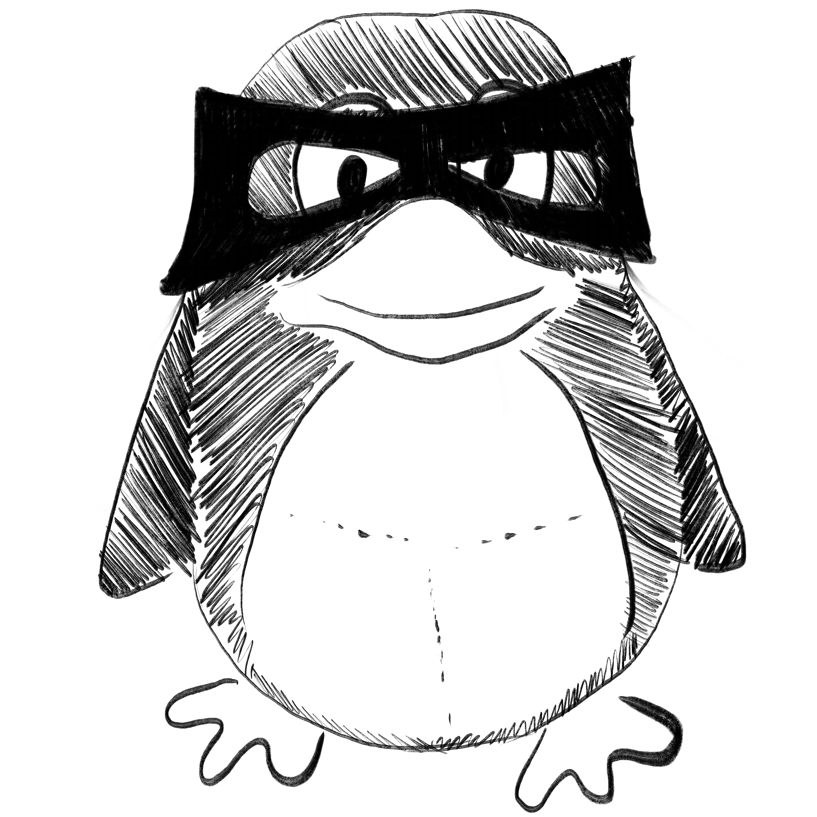
Predicting factors affecting the intention to use a 3PL during the COVID-19 pandemic: A machine learning ensemble approach.
In Heliyon
German Josephine D, Ong Ardvin Kester S, Perwira Redi Anak Agung Ngurah, Robas Kirstien Paola E
2022-Nov
Artificial neural network, Behavioral intention, Random forest classifier, Third party logistics
COVID-19 Health Beliefs Regarding Mask Wearing and Vaccinations on Twitter: Deep Learning Approach.
In JMIR infodemiology
Background :
Objective :
Methods :
Results :
Conclusions :
Ke Si Yang, Neeley-Tass E Shannon, Barnes Michael, Hanson Carl L, Giraud-Carrier Christophe, Snell Quinn
COVID-19, Health Belief Model, Twitter, content analysis, deep learning, health belief, infodemic, infodemiology, machine learning, mask, misinformation, vaccination, vaccine data set
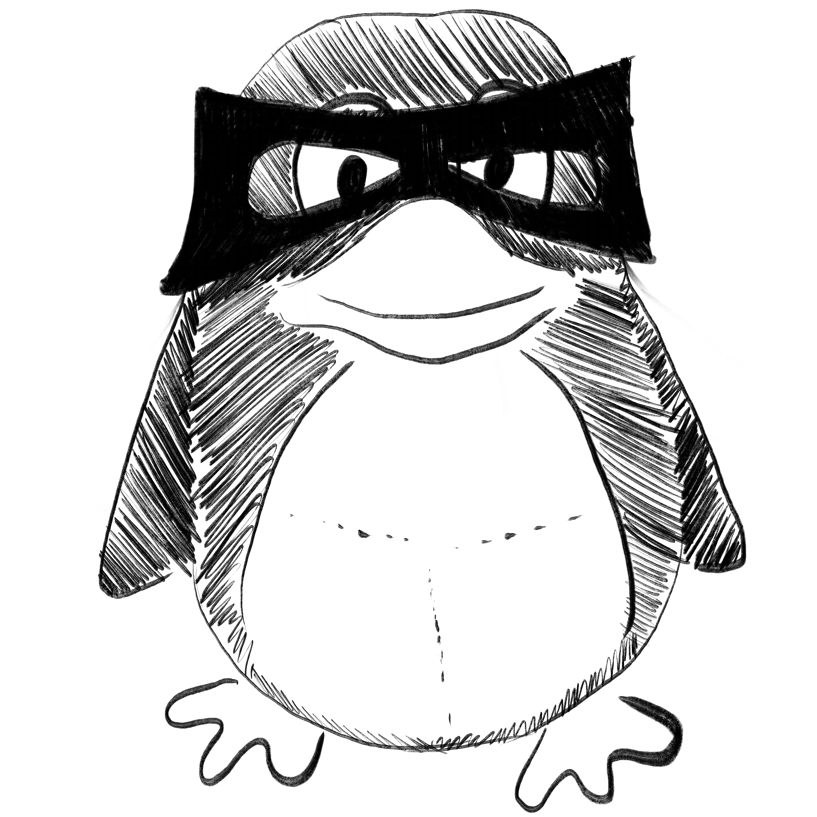
Improved Deep Convolutional Neural Networks using Chimp Optimization Algorithm for Covid19 Diagnosis from the X-Ray Images.
In Expert systems with applications
Cai Chengfeng, Gou Bingchen, Khishe Mohammad, Mohammadi Mokhtar, Rashidi Shima, Moradpour Reza, Mirjalili Seyedali
2022-Nov-04
COVID-19 diagnosis, Chest X-Rays, Chimp Optimization Algorithm, Convolutional Neural Networks, Deep Learning
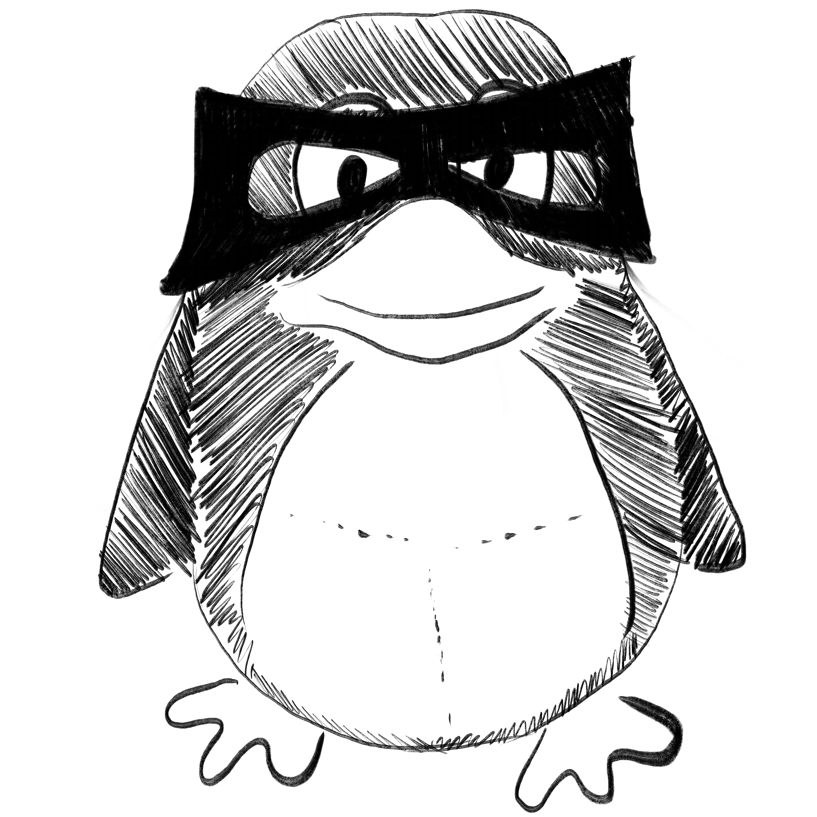
Preparations for the Assessment of COVID-19 Infection and Long-Term Cardiovascular Risk.
In Korean circulation journal
Jung Jaehun
2022-Nov
COVID-19, COVID-19 vaccination, Cardiovascular risk, Long-COVID
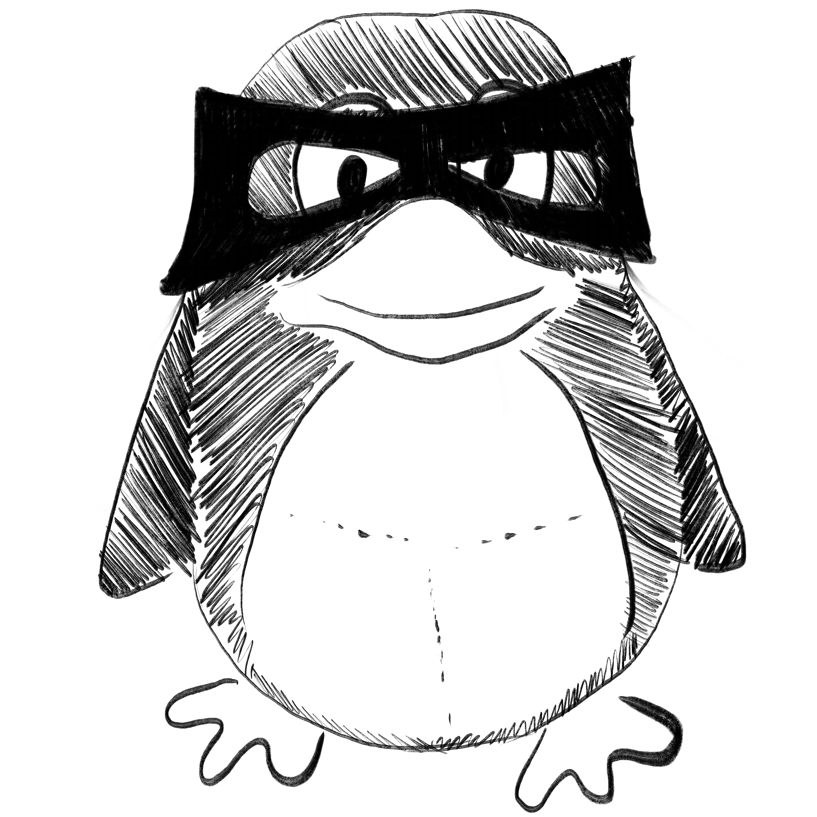
Intelligent COVID-19 screening platform based on breath analysis.
In Journal of breath research
BACKGROUND :
METHODS :
RESULTS :
CONCLUSION :
Xue Cuili, Xu Xiaohong, Liu Zexi, Zhang Yuna, Xu Yuli, Niu Jiaqi, Jin Han, Xiong Wujun, Cui Daxiang
2022-Nov-08
COVID-19 diagnosis, Portable GC-MS, breath analysis, support vector machine, volatile organic compound
Exploring public opinion about telehealth during COVID-19 by social media analytics.
In Journal of telemedicine and telecare ; h5-index 28.0
Pool Javad, Namvar Morteza, Akhlaghpour Saeed, Fatehi Farhad
2022-Dec
COVID-19, Telehealth, machine learning, social media analytics, telemedicine
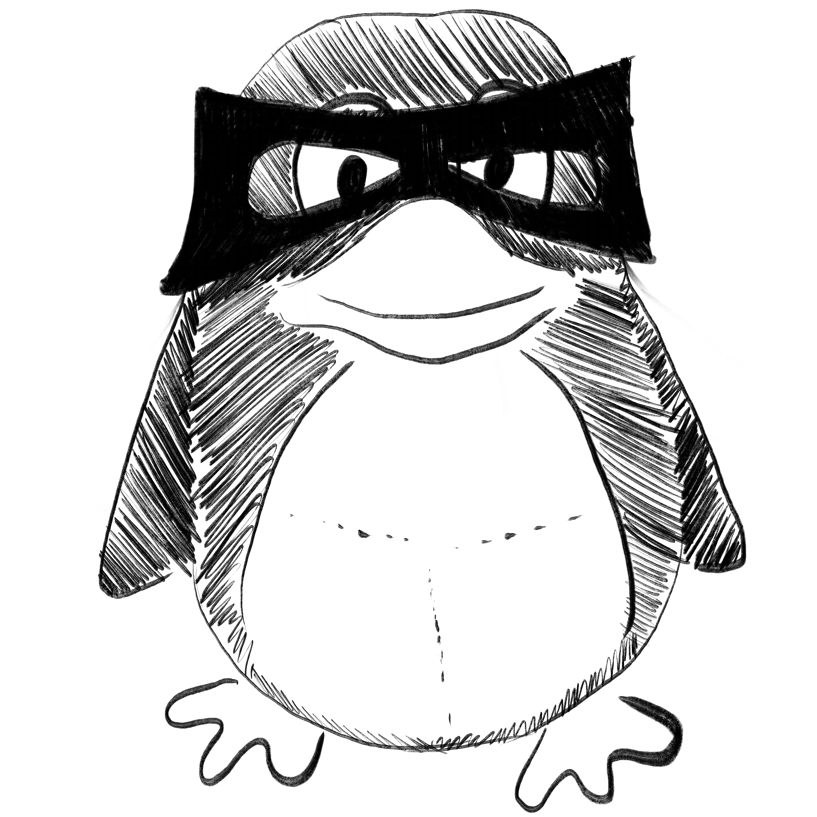
Personalized Prediction of Response to Smartphone-Delivered Meditation Training: Randomized Controlled Trial.
In Journal of medical Internet research ; h5-index 88.0
BACKGROUND :
OBJECTIVE :
METHODS :
RESULTS :
CONCLUSIONS :
TRIAL REGISTRATION :
Webb Christian A, Hirshberg Matthew J, Davidson Richard J, Goldberg Simon B
2022-Nov-08
machine learning, meditation, mobile phone, mobile technology, precision medicine, prediction, smartphone app
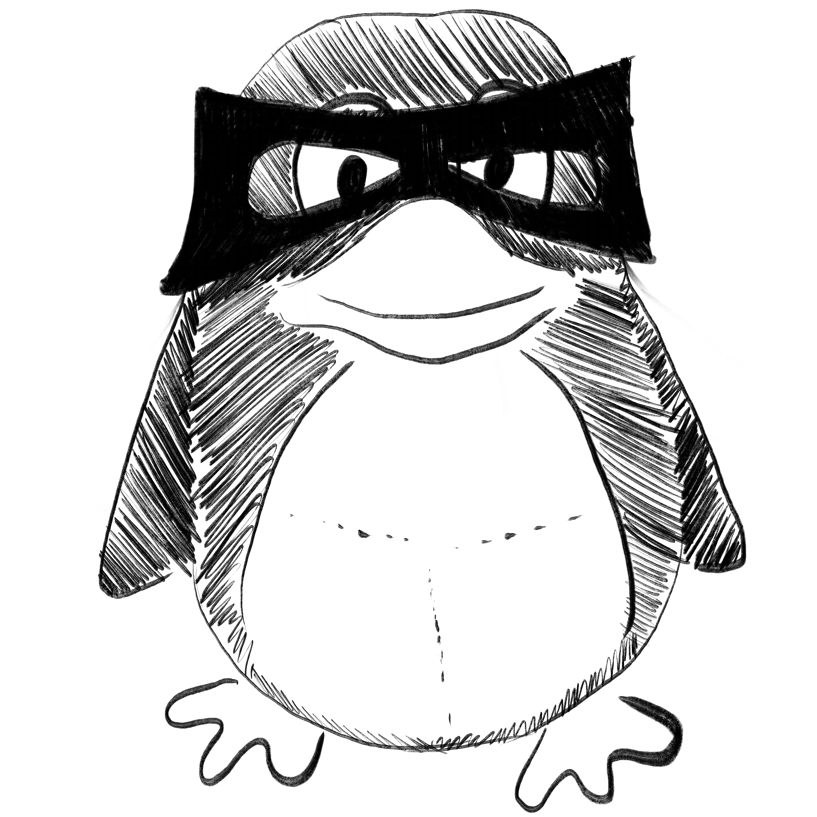
The positive energy of netizens: development and application of fine-grained sentiment lexicon and emotional intensity model.
In Current psychology (New Brunswick, N.J.)
Pan Wenhao, Han Yingying, Li Jinjin, Zhang Emily, He Bikai
2022-Nov-03
COVID-19 pandemic, Fine-grained sentiment lexicon, Positive energy, Social media analysis
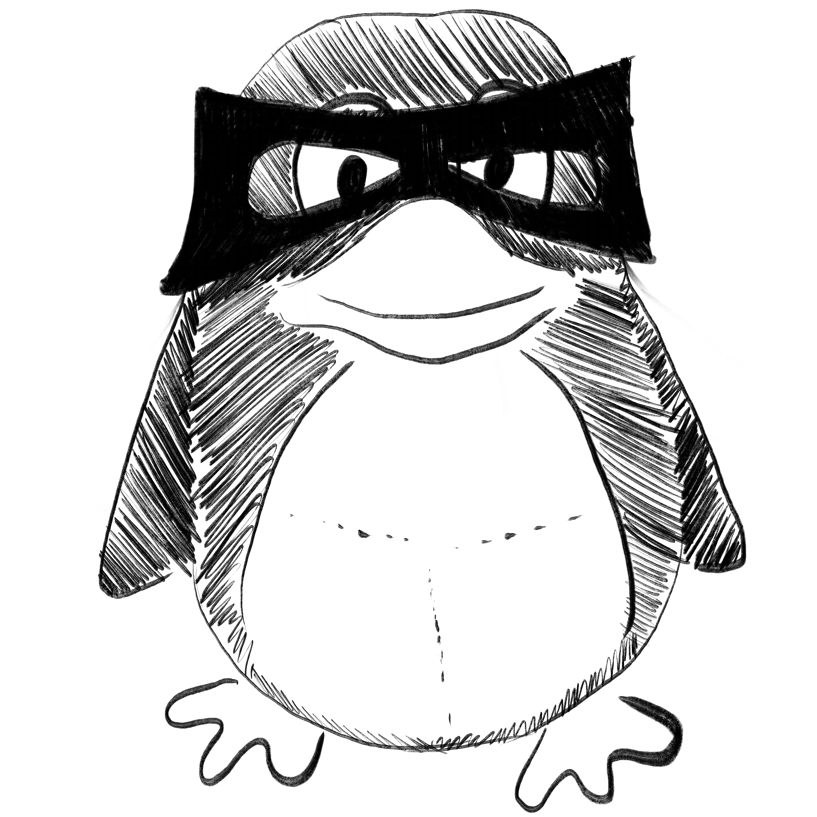
IoT-Based COVID-19 Diagnosing and Monitoring Systems: A Survey.
In IEEE access : practical innovations, open solutions
Anjum Nasreen, Alibakhshikenari Mohammad, Rashid Junaid, Jabeen Fouzia, Asif Amna, Mohamed Ehab Mahmoud, Falcone Francisco
2022
COVID-19 pandemic, Internet of Things (IoTs), artificial intelligence (AI), coronavirus, machine learning algorithms
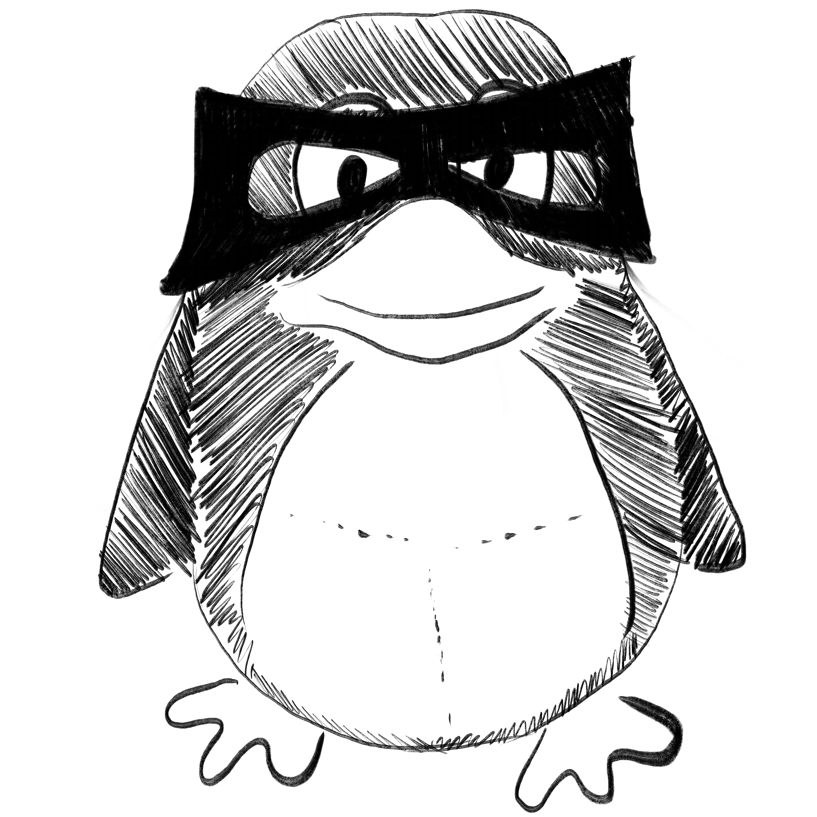
The paradigm and future value of the metaverse for the intervention of cognitive decline.
In Frontiers in public health
Zhou Hao, Gao Jian-Yi, Chen Ying
2022
“Alzheimers disease”, cognitive decline, digital twin, mental health, metaverse in medicine, virtual reality
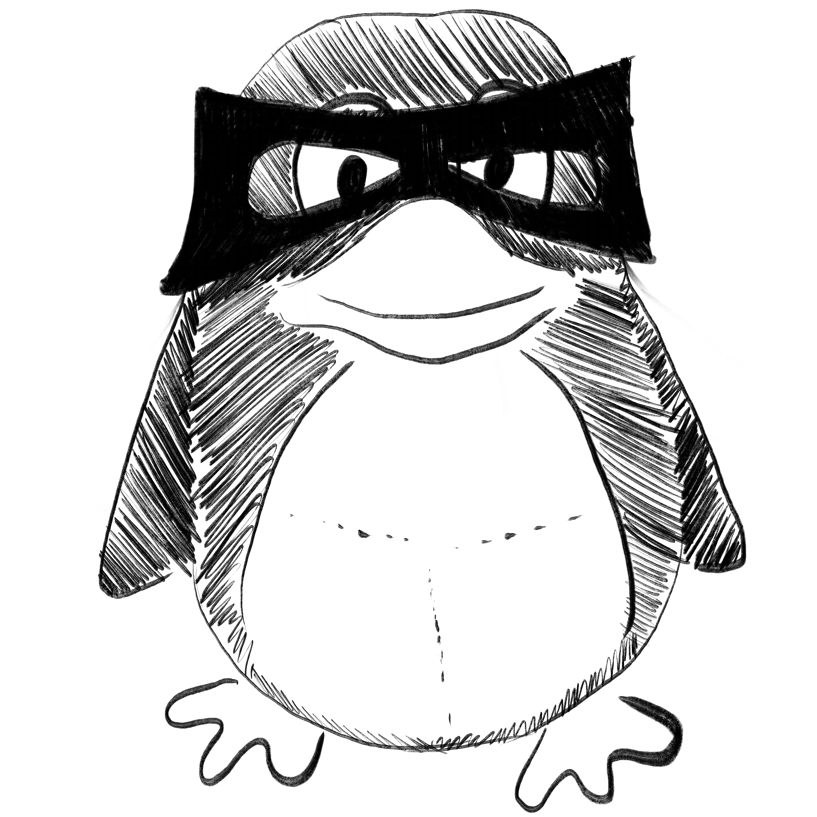
Interpreting global variations in the toll of COVID-19: The case for context and nuance in hypothesis generation and testing.
In Frontiers in public health
Key points :
Methods :
Results :
Conclusions/implications :
Stein Roger M, Katz David L
2022
COVID, health economics, health policy, lifestyle factors, machine learning, obesity, pandemic, statistical methods
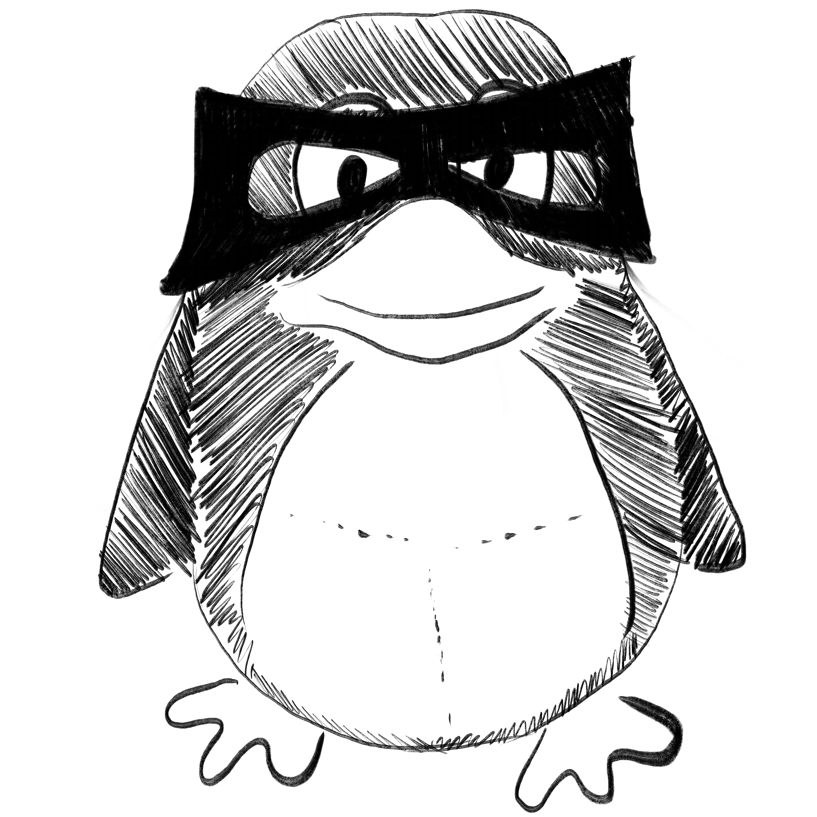
Ensemble learning-based feature selection for phosphorylation site detection.
In Frontiers in genetics ; h5-index 62.0
Liu Songbo, Cui Chengmin, Chen Huipeng, Liu Tong
2022
SARS-cov-2, ensemble learning (EN), feature selection (FS), marchine-learning, phosphorylation site
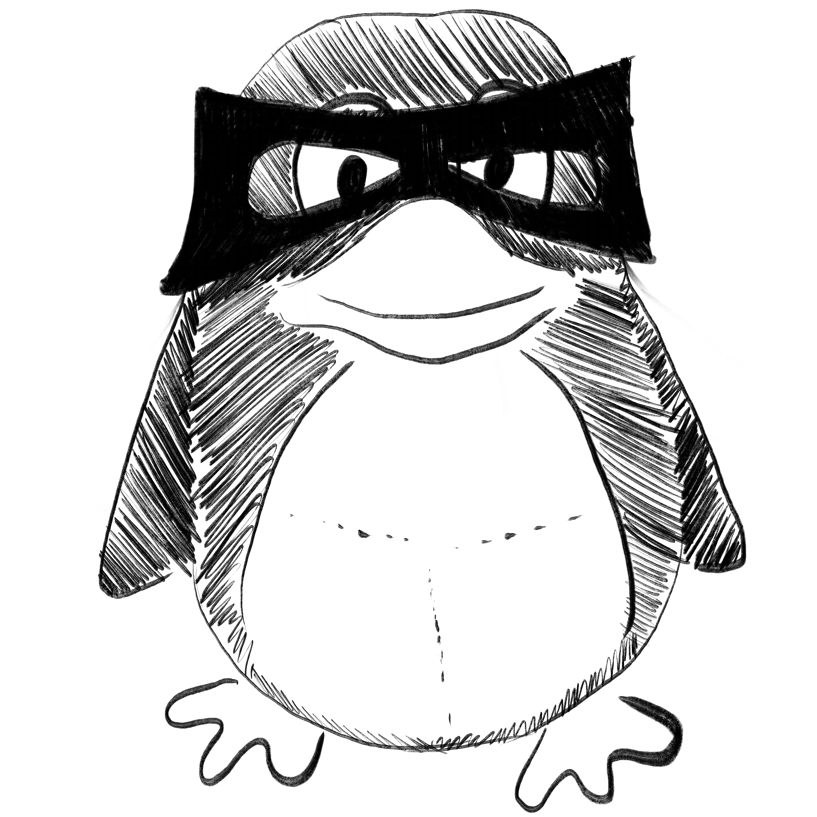
Attention based parameter estimation and states forecasting of COVID-19 pandemic using modified SIQRD Model.
In Chaos, solitons, and fractals
Khan Junaid Iqbal, Ullah Farman, Lee Sungchang
2022-Oct-31
COVID-19, Compartment model, Control theory, Deep learning
Leveraging OpenStreetMap and Multimodal Remote Sensing Data with Joint Deep Learning for Wastewater Treatment Plants Detection.
In International journal of applied earth observation and geoinformation : ITC journal
Li Hao, Zech Johannes, Hong Danfeng, Ghamisi Pedram, Schultz Michael, Zipf Alexander
2022-Jun
GeoAI, OpenStreetMap, SDG 6, multi-task learning, multimodal, object detection, volunteered geographic information, wastewater treatment
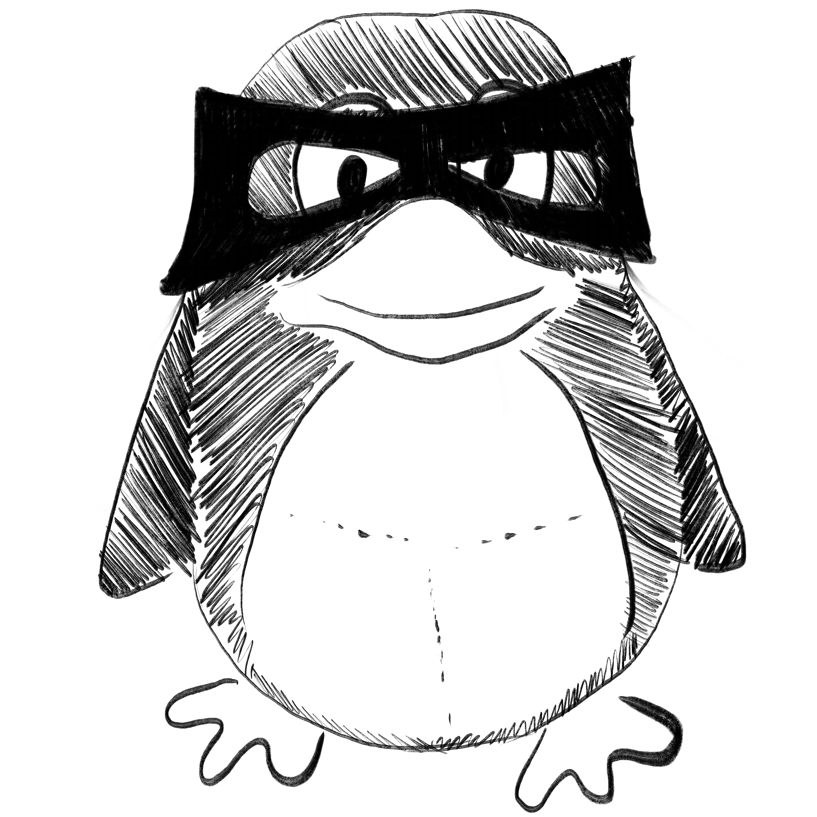
Socially facilitative robots for older adults to alleviate social isolation: A participatory design workshop approach in the US and Japan.
In Frontiers in psychology ; h5-index 92.0
Fraune Marlena R, Komatsu Takanori, Preusse Harrison R, Langlois Danielle K, Au Rachel H Y, Ling Katrina, Suda Shogo, Nakamura Kiko, Tsui Katherine M
2022
Japan, US, cross-cultural study, experience-grounded participatory design, human-robot interaction, older adults, social isolation, social robots
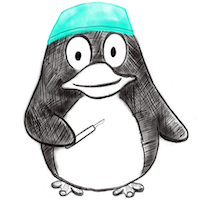
"The show must go on": Aftermath of Covid-19 on anesthesiology residency programs.
In Saudi journal of anaesthesia
Jaju Rishabh, Saxena Medhavi, Paliwal Naveen, Bihani Pooja, Tharu Vidya
Academic, Covid-19, anesthesia, burnout, resident training
COVID-19 Outbreak Forecasting Based on Vaccine Rates and Tweets Classification.
In Computational intelligence and neuroscience
Didi Y, Walha A, Ben Halima M, Wali A
2022
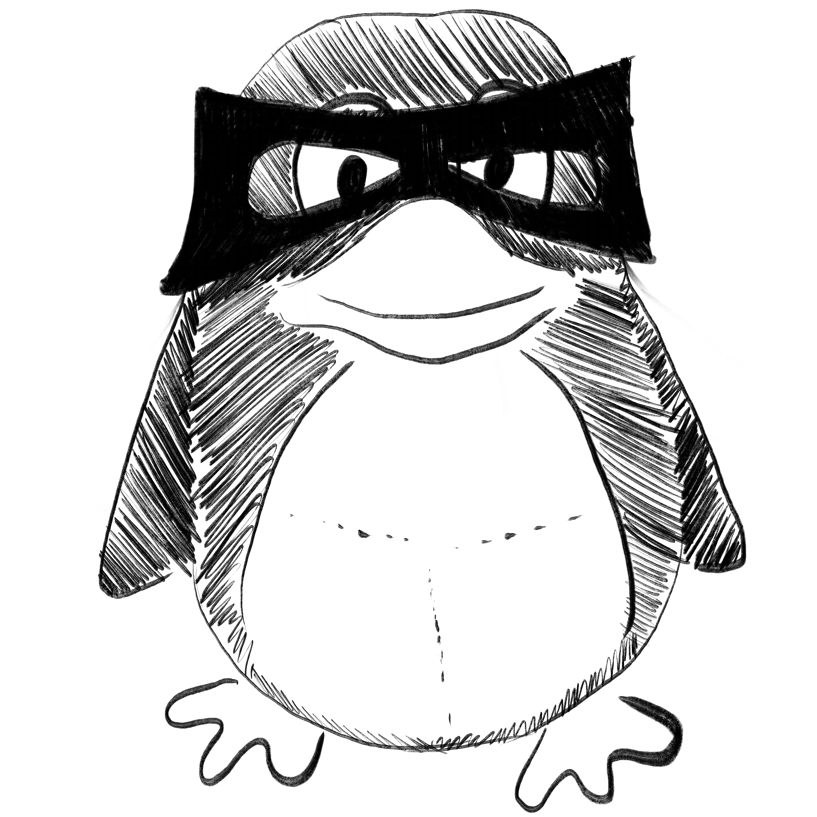
Dual-Proxy Modeling for Masked Face Recognition.
In Procedia computer science
Shuhui Wang, Xiaochen Mao
2022
Dual-Proxy, Masked face recognition, deep learning, neural network
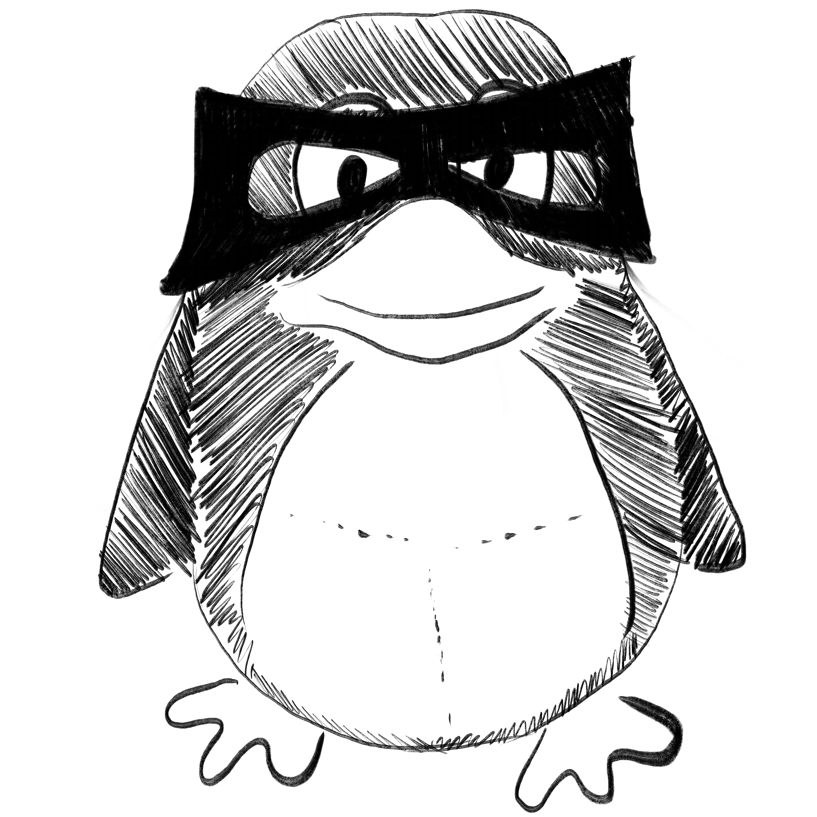
Care for the Mind Amid Chronic Diseases: An Interpretable AI Approach Using IoT
ArXiv Preprint
Jiaheng Xie, Xiaohang Zhao, Xiang Liu, Xiao Fang
2022-11-08
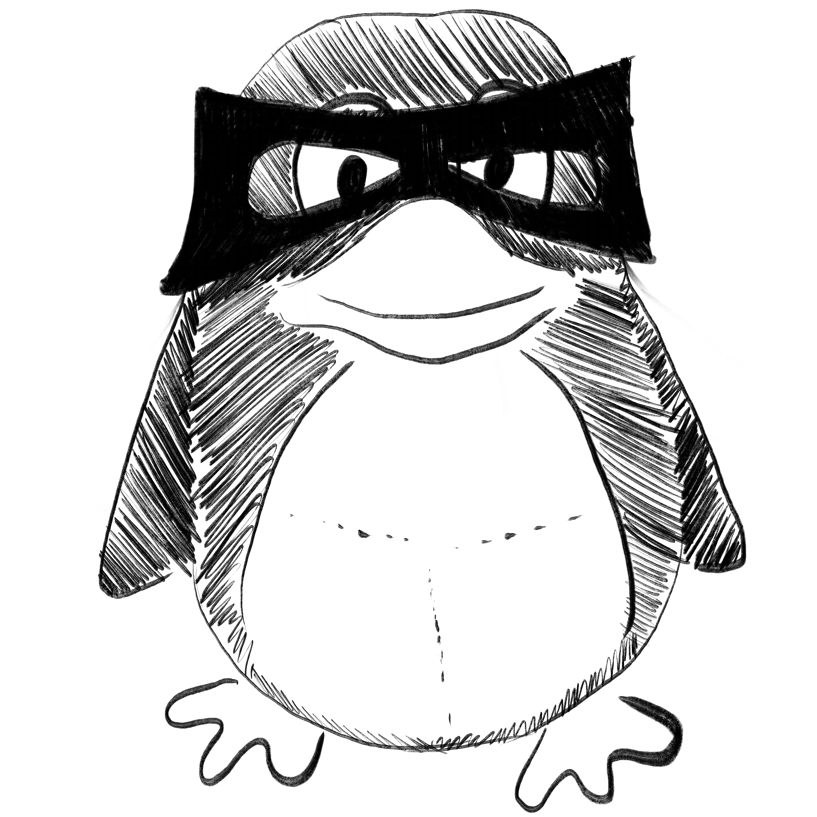
Insight into the nonlinear effect of COVID-19 on well-being in China: Commuting, a vital ingredient.
In Journal of transport & health
Background :
Methods :
Results :
Conclusions :
Dong Yinan, Sun Yilin, D Waygood E Owen, Wang Bobin, Huang Pei, Naseri Hamed
2022-Oct-31
COVID-19, Commuting behavior, Machine learning, Social interaction, Well-being
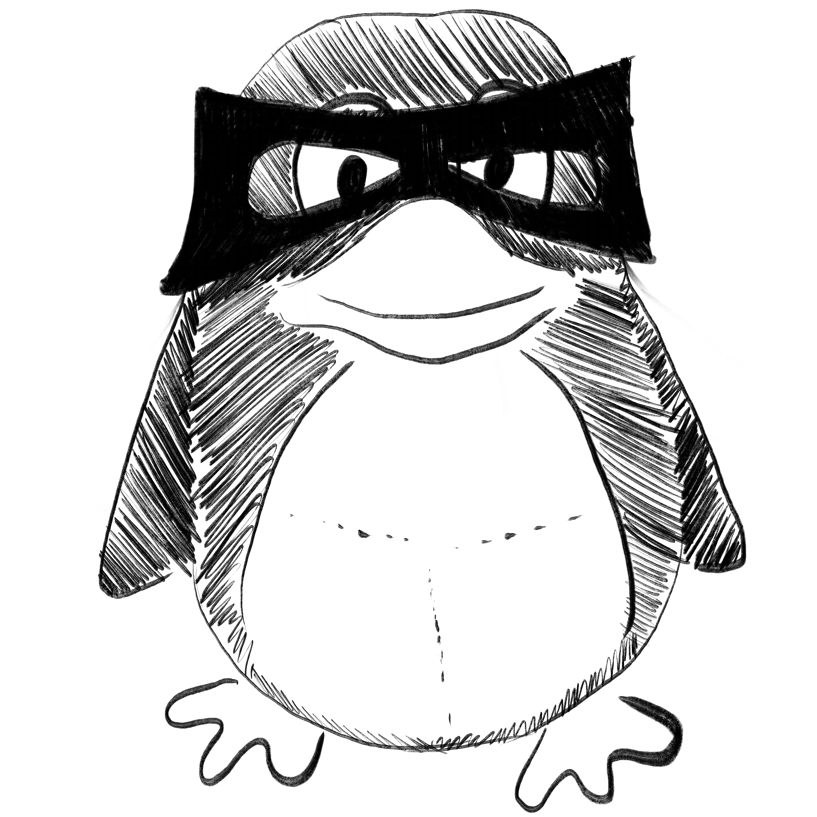
A Critical Review of Global Digital Divide and the Role of Technology in Healthcare.
In Cureus
Reddy Himabindu, Joshi Shiv, Joshi Abhishek, Wagh Vasant
2022-Sep
digital divide, digital health, e-health, healthcare access, healthcare technology, inequity
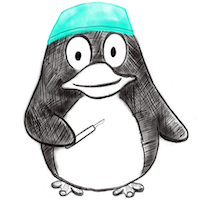
Homogeneous ensemble models for predicting infection levels and mortality of COVID-19 patients: Evidence from China.
In Digital health
Background :
Methods :
Results :
Conclusions :
Wang Jiafeng, Zhou Xianlong, Hou Zhitian, Xu Xiaoya, Zhao Yueyue, Chen Shanshan, Zhang Jun, Shao Lina, Yan Rong, Wang Mingshan, Ge Minghua, Hao Tianyong, Tu Yuexing, Huang Haijun
COVID-19, Ensemble model, electronic health records, machine learning, prediction models
Mental health and chest CT scores mediate the relationship between COVID-19 vaccination status and seroconversion time: A cross-sectional observational study in B.1.617.2 (Delta) infection patients.
In Frontiers in public health
Background :
Methods :
Results :
Conclusion :
Zhang Wen, Chen Qian, Dai Jinghong, Lu Jiaming, Li Jie, Yi Yongxiang, Fu Linqing, Li Xin, Liu Jiani, Liufu Jinlong, Long Cong, Zhang Bing
2022
B.1.617.2 Delta variant, COVID-19, SARS-CoV-2, mental health, seroconversion time, vaccination
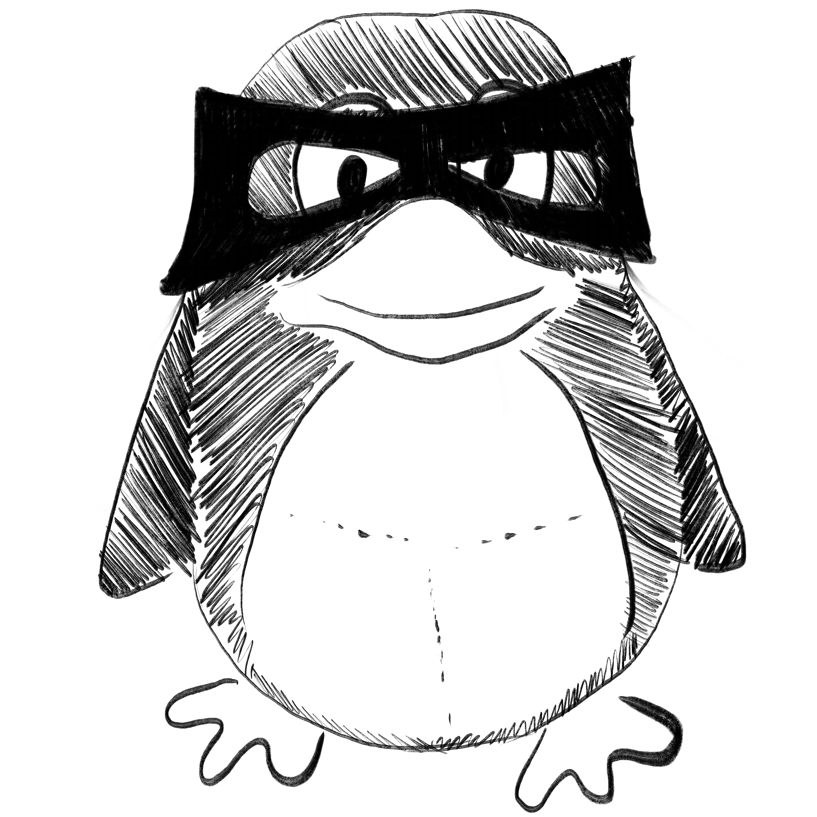
CELLS: A Parallel Corpus for Biomedical Lay Language Generation
ArXiv Preprint
Yue Guo, Wei Qiu, Gondy Leroy, Sheng Wang, Trevor Cohen
2022-11-07
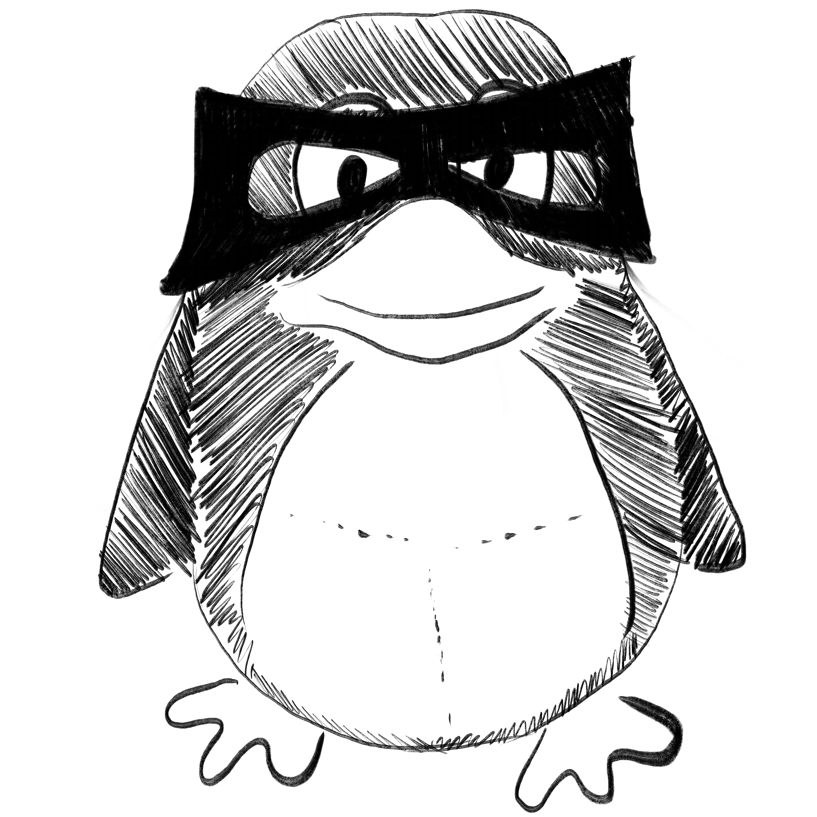
Prediction of COVID-19 patients in danger of death using radiomic features of portable chest radiographs.
In Journal of medical radiation sciences
INTRODUCTION :
METHODS :
RESULTS :
CONCLUSIONS :
Nakashima Maoko, Uchiyama Yoshikazu, Minami Hirotake, Kasai Satoshi
2022-Nov-05
Artificial intelligence, COVID-19, portable chest X-ray, prognosis prediction, radiomics
Machine Learning Successfully Detects Patients with COVID-19 Prior to PCR Results and Predicts Their Survival Based on Standard Laboratory Parameters in an Observational Study.
In Infectious diseases and therapy
INTRODUCTION :
METHODS :
RESULTS :
CONCLUSIONS :
Styrzynski Filip, Zhakparov Damir, Schmid Marco, Roqueiro Damian, Lukasik Zuzanna, Solek Julia, Nowicki Jakub, Dobrogowski Milosz, Makowska Joanna, Sokolowska Milena, Baerenfaller Katja
2022-Nov-04
COVID-19 prognosis, Laboratory parameters, Machine learning, Predictive features, SARS-CoV-2 diagnosis
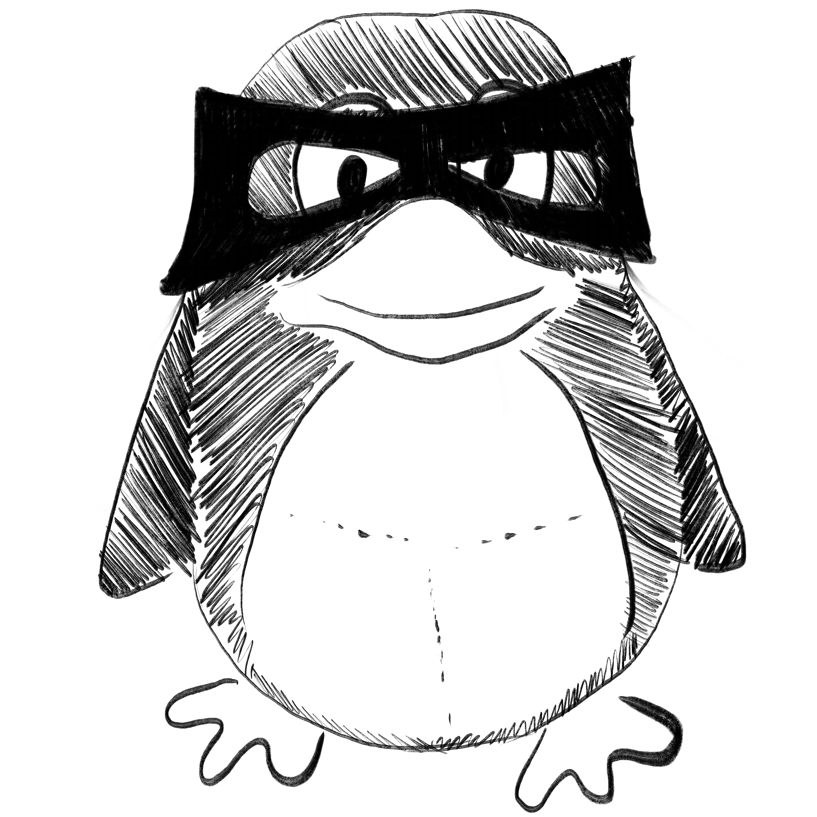
Detecting COVID-19 from digitized ECG printouts using 1D convolutional neural networks.
In PloS one ; h5-index 176.0
Nguyen Thao, Pham Hieu H, Le Khiem H, Nguyen Anh-Tu, Thanh Tien, Do Cuong
2022
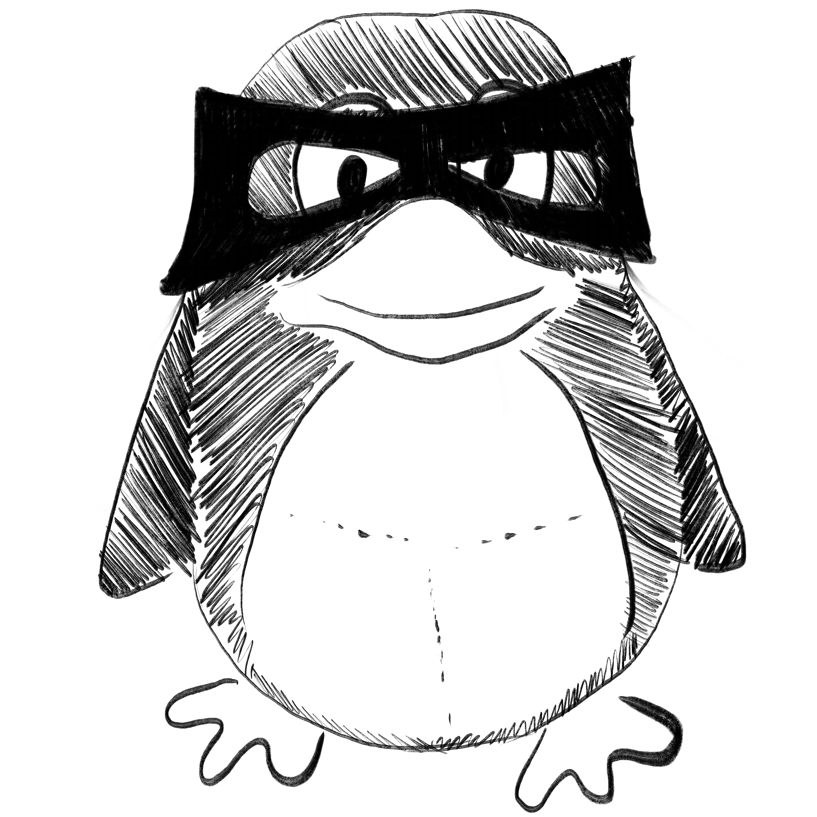
Emerging Technologies Used in Health Management and Efficiency Improvement During Different Contact Tracing Phases Against COVID-19 Pandemic.
In IEEE reviews in biomedical engineering
Gendy Maggie Ezzat Gaber, Yuce Mehmet Rasit
2022-Nov-04
Assessment of COVID-19 lung involvement on computed tomography by deep-learning-, threshold-, and human reader-based approaches-an international, multi-center comparative study.
In Quantitative imaging in medicine and surgery
Background :
Methods :
Results :
Conclusions :
Fervers Philipp, Fervers Florian, Jaiswal Astha, Rinneburger Miriam, Weisthoff Mathilda, Pollmann-Schweckhorst Philip, Kottlors Jonathan, Carolus Heike, Lennartz Simon, Maintz David, Shahzad Rahil, Persigehl Thorsten
2022-Nov
Coronavirus disease 2019 (COVID-19), X-ray computed, biomarkers, linear models, pneumonia, tomography
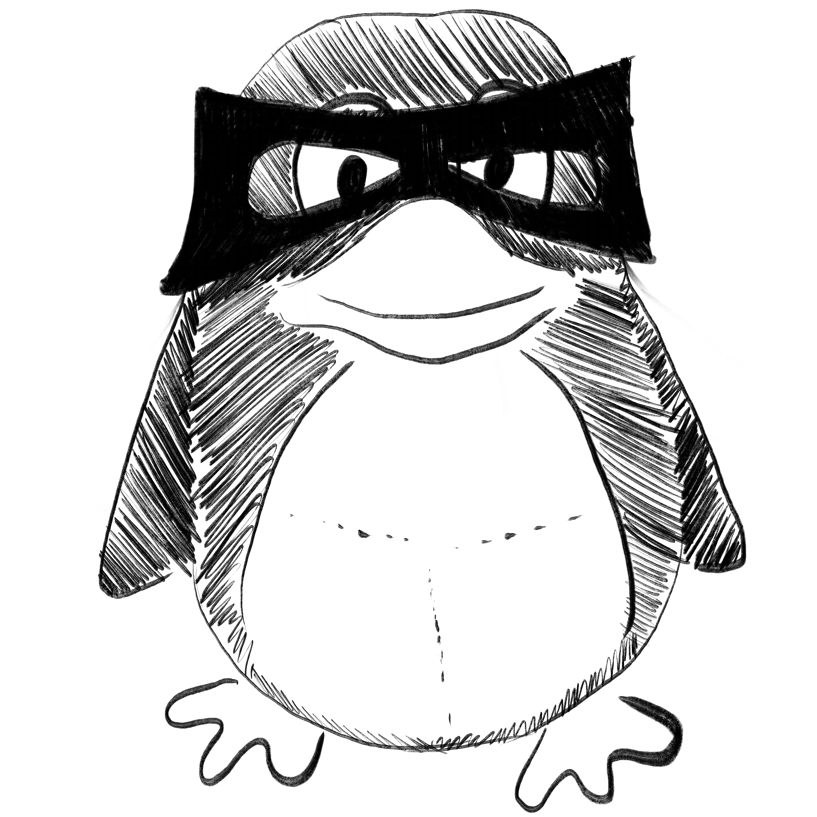
Pre-hospital prediction of adverse outcomes in patients with suspected COVID-19: Development, application and comparison of machine learning and deep learning methods.
In Computers in biology and medicine
BACKGROUND :
METHOD :
RESULTS :
CONCLUSIONS :
Hasan M, Bath P A, Marincowitz C, Sutton L, Pilbery R, Hopfgartner F, Mazumdar S, Campbell R, Stone T, Thomas B, Bell F, Turner J, Biggs K, Petrie J, Goodacre S
2022-Aug-28
Artificial neural networks, COVID-19, Emergency services, Extreme gradient boosting, Logistic regression, Stacking ensemble, Support vector machine
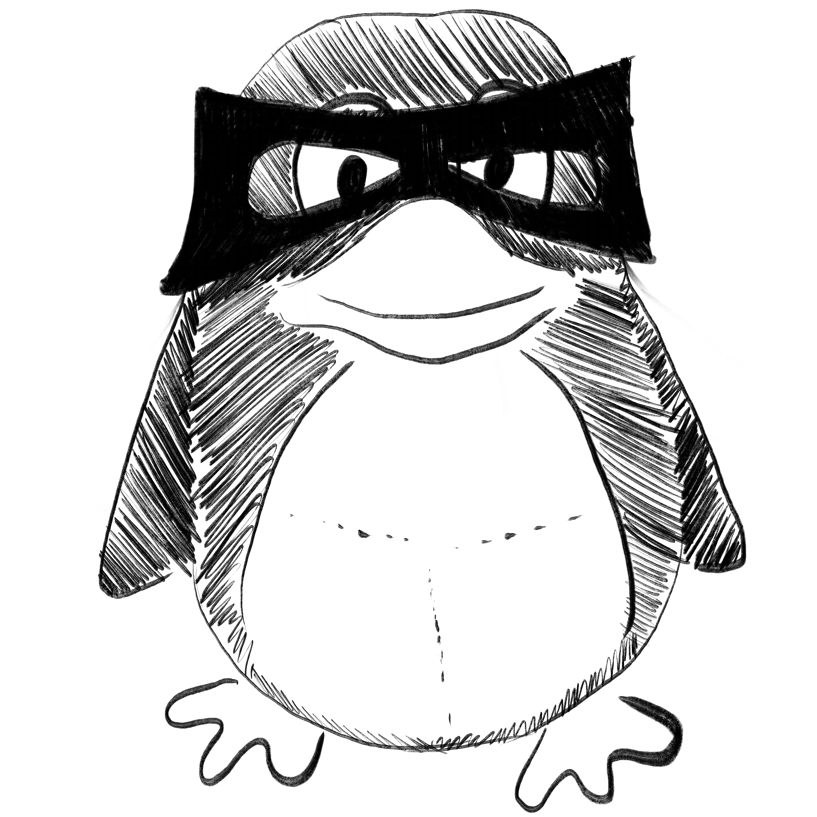
D3AI-Spike: A deep learning platform for predicting binding affinity between SARS-CoV-2 spike receptor binding domain with multiple amino acid mutations and human angiotensin-converting enzyme 2.
In Computers in biology and medicine
Han Jiaxin, Liu Tingting, Zhang Xinben, Yang Yanqing, Shi Yulong, Li Jintian, Ma Minfei, Zhu Weiliang, Gong Likun, Xu Zhijian
2022-Oct-25
COVID-19, D3AI-Spike, Deep learning, ELISA, Protein-protein interaction
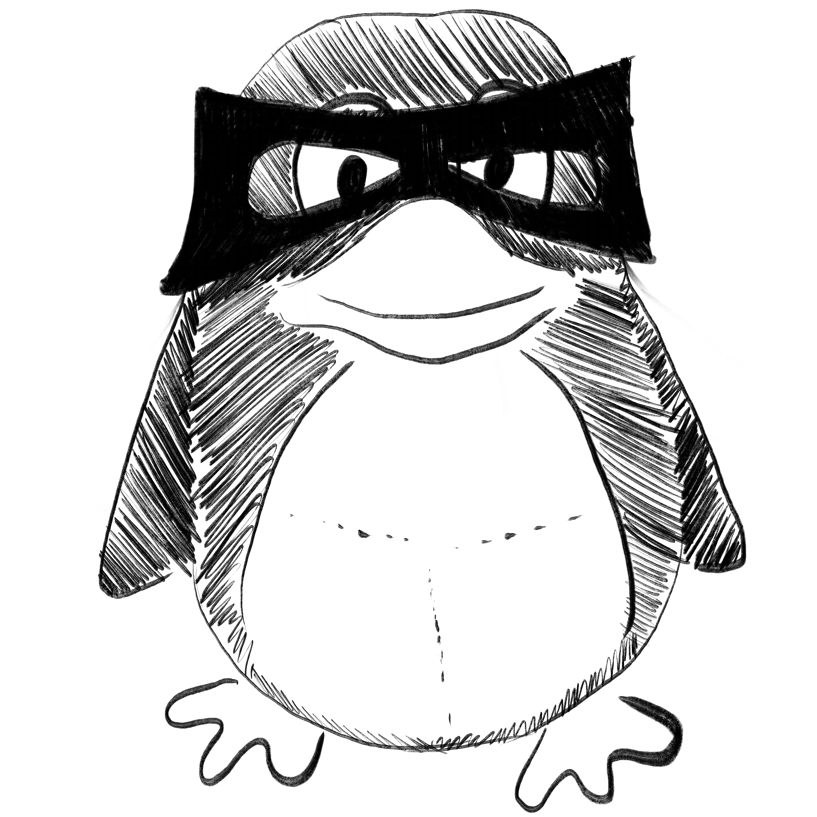
Superhuman performance on sepsis MIMIC-III data by distributional reinforcement learning.
In PloS one ; h5-index 176.0
Böck Markus, Malle Julien, Pasterk Daniel, Kukina Hrvoje, Hasani Ramin, Heitzinger Clemens
2022
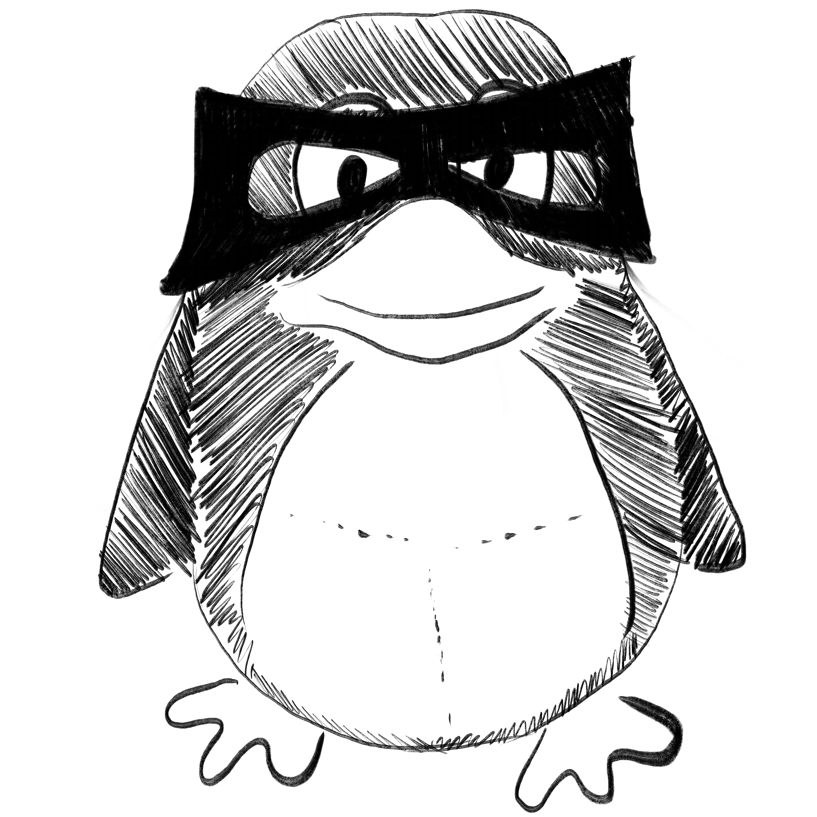
Critical role of information and communication technology in nursing during the COVID-19 pandemic: A qualitative study.
In Journal of nursing management ; h5-index 43.0
AIM :
BACKGROUND :
METHOD :
RESULTS :
CONCLUSION :
IMPLICATIONS FOR NURSING MANAGEMENT :
Yoo Hye Jin, Lee Hyeongsuk
2022-Nov-03
COVID-19, artificial intelligence, information technology, nursing care, patient isolation
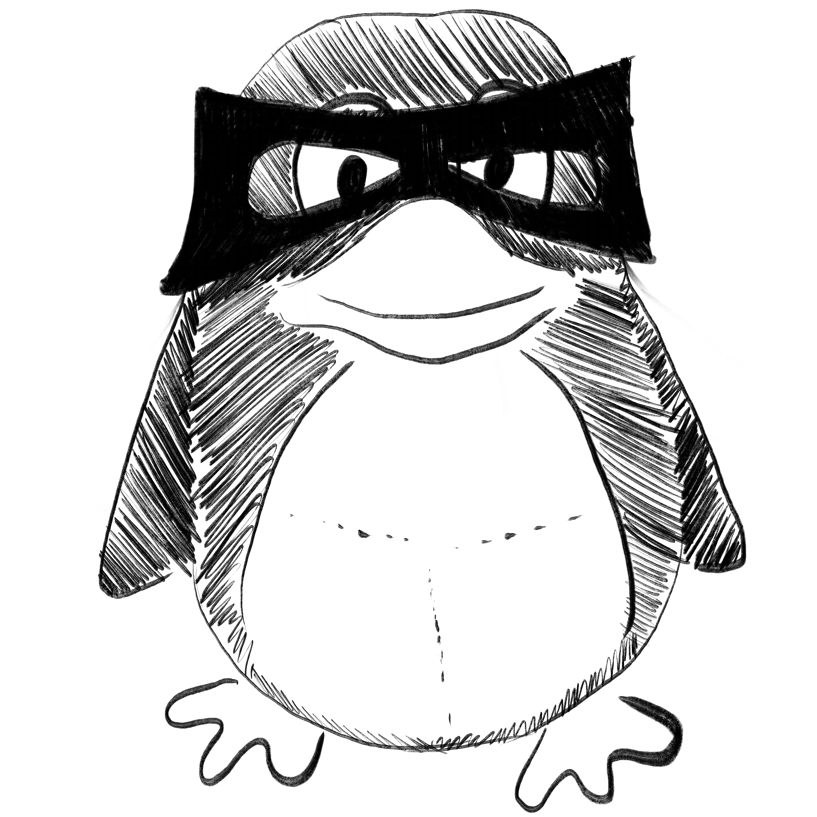
Computationally restoring the potency of a clinical antibody against SARS-CoV-2 Omicron subvariants.
In bioRxiv : the preprint server for biology
Desautels Thomas A, Arrildt Kathryn T, Zemla Adam T, Lau Edmond Y, Zhu Fangqiang, Ricci Dante, Cronin Stephanie, Zost Seth J, Binshtein Elad, Scheaffer Suzanne M, Engdahl Taylor B, Chen Elaine, Goforth John W, Vashchenko Denis, Nguyen Sam, Weilhammer Dina R, Lo Jacky Kai-Yin, Rubinfeld Bonnee, Saada Edwin A, Weisenberger Tracy, Lee Tek-Hyung, Whitener Bradley, Case James B, Ladd Alexander, Silva Mary S, Haluska Rebecca M, Grzesiak Emilia A, Bates Thomas W, Petersen Brenden K, Thackray Larissa B, Segelke Brent W, Lillo Antonietta Maria, Sundaram Shivshankar, Diamond Michael S, Crowe James E, Carnahan Robert H, Faissol Daniel M
2022-Oct-24
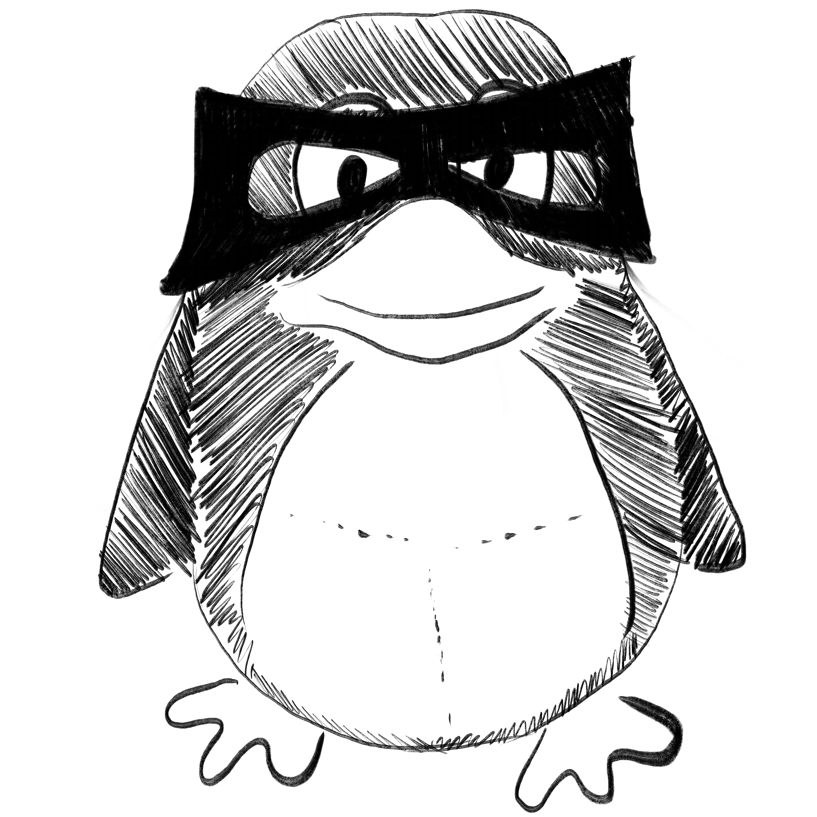
A novel deep learning-based method for COVID-19 pneumonia detection from CT images.
In BMC medical informatics and decision making ; h5-index 38.0
BACKGROUND :
AIMS :
METHODS :
RESULTS :
CONCLUSION :
Luo Ju, Sun Yuhao, Chi Jingshu, Liao Xin, Xu Canxia
2022-Nov-02
Artificial intelligence, COVID-19, CT image, Community acquired pneumonia, Deep learning
An immune dysfunction score for stratification of patients with acute infection based on whole-blood gene expression.
In Science translational medicine ; h5-index 138.0
Cano-Gamez Eddie, Burnham Katie L, Goh Cyndi, Allcock Alice, Malick Zunaira H, Overend Lauren, Kwok Andrew, Smith David A, Peters-Sengers Hessel, Antcliffe David, McKechnie Stuart, Scicluna Brendon P, van der Poll Tom, Gordon Anthony C, Hinds Charles J, Davenport Emma E, Knight Julian C, Webster Nigel, Galley Helen, Taylor Jane, Hall Sally, Addison Jenni, Roughton Sian, Tennant Heather, Guleri Achyut, Waddington Natalia, Arawwawala Dilshan, Durcan John, Short Alasdair, Swan Karen, Williams Sarah, Smolen Susan, Mitchell-Inwang Christine, Gordon Tony, Errington Emily, Templeton Maie, Venatesh Pyda, Ward Geraldine, McCauley Marie, Baudouin Simon, Higham Charley, Soar Jasmeet, Grier Sally, Hall Elaine, Brett Stephen, Kitson David, Wilson Robert, Mountford Laura, Moreno Juan, Hall Peter, Hewlett Jackie, McKechnie Stuart, Garrard Christopher, Millo Julian, Young Duncan, Hutton Paula, Parsons Penny, Smiths Alex, Faras-Arraya Roser, Soar Jasmeet, Raymode Parizade, Thompson Jonathan, Bowrey Sarah, Kazembe Sandra, Rich Natalie, Andreou Prem, Hales Dawn, Roberts Emma, Fletcher Simon, Rosbergen Melissa, Glister Georgina, Cuesta Jeronimo Moreno, Bion Julian, Millar Joanne, Perry Elsa Jane, Willis Heather, Mitchell Natalie, Ruel Sebastian, Carrera Ronald, Wilde Jude, Nilson Annette, Lees Sarah, Kapila Atul, Jacques Nicola, Atkinson Jane, Brown Abby, Prowse Heather, Krige Anton, Bland Martin, Bullock Lynne, Harrison Donna, Mills Gary, Humphreys John, Armitage Kelsey, Laha Shond, Baldwin Jacqueline, Walsh Angela, Doherty Nicola, Drage Stephen, Ortiz-Ruiz de Gordoa Laura, Lowes Sarah, Higham Charley, Walsh Helen, Calder Verity, Swan Catherine, Payne Heather, Higgins David, Andrews Sarah, Mappleback Sarah, Hind Charles, Garrard Chris, Watson D, McLees Eleanor, Purdy Alice, Stotz Martin, Ochelli-Okpue Adaeze, Bonner Stephen, Whitehead Iain, Hugil Keith, Goodridge Victoria, Cawthor Louisa, Kuper Martin, Pahary Sheik, Bellingan Geoffrey, Marshall Richard, Montgomery Hugh, Ryu Jung Hyun, Bercades Georgia, Boluda Susan, Bentley Andrew, Mccalman Katie, Jefferies Fiona, Knight Julian, Davenport Emma, Burnham Katie, Maugeri Narelle, Radhakrishnan Jayachandran, Mi Yuxin, Allcock Alice, Goh Cyndi
2022-Nov-02
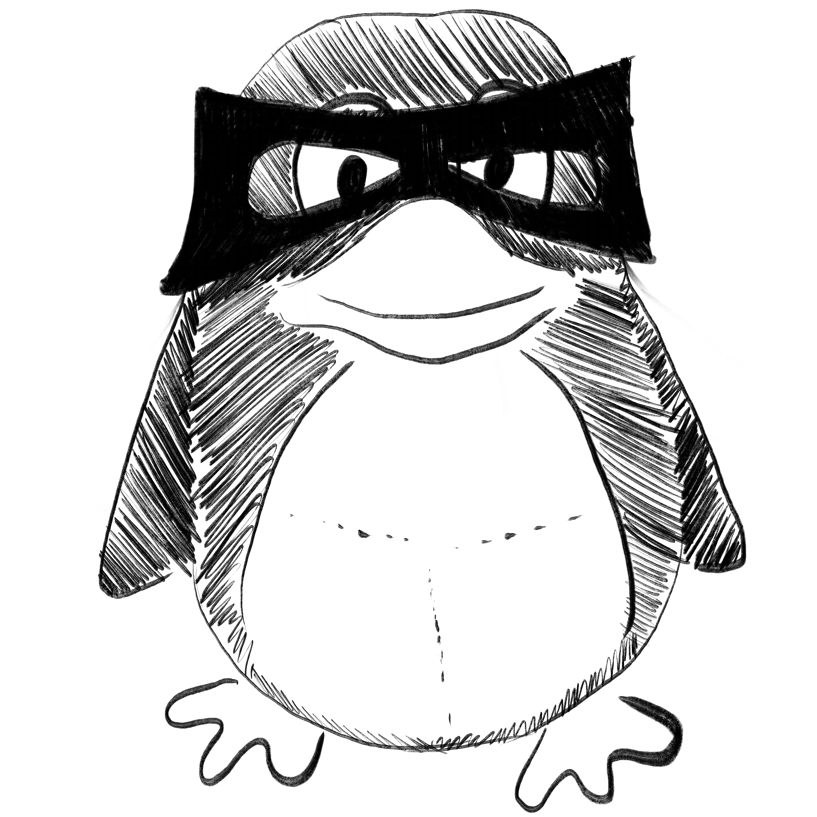
Application of Supervised Machine Learning Techniques to Forecast the COVID-19 U.S. Recession and Stock Market Crash.
In Computational economics
Malladi Rama K
2022-Oct-26
Financial econometrics, Forecasting, Machine learning, Recession, Stock market crash
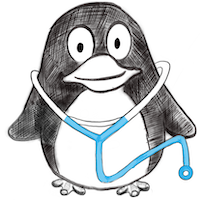
The Impact of COVID-19 on the Behaviors and Attitudes of Children and Adolescents: A Cross-Sectional Study.
In Cureus
Annam Swetha, Fleming Maria F, Gulraiz Azouba, Zafar Muhammad Talha, Khan Saif, Oghomitse-Omene Princess T, Saleemuddin Sana, Patel Parth, Ahsan Zainab, Qavi Muhammad Saqlain S
2022-Sep
adolescent, child and adolescent psychiatry, child attitudes, child behaviour, covid-19, mental health
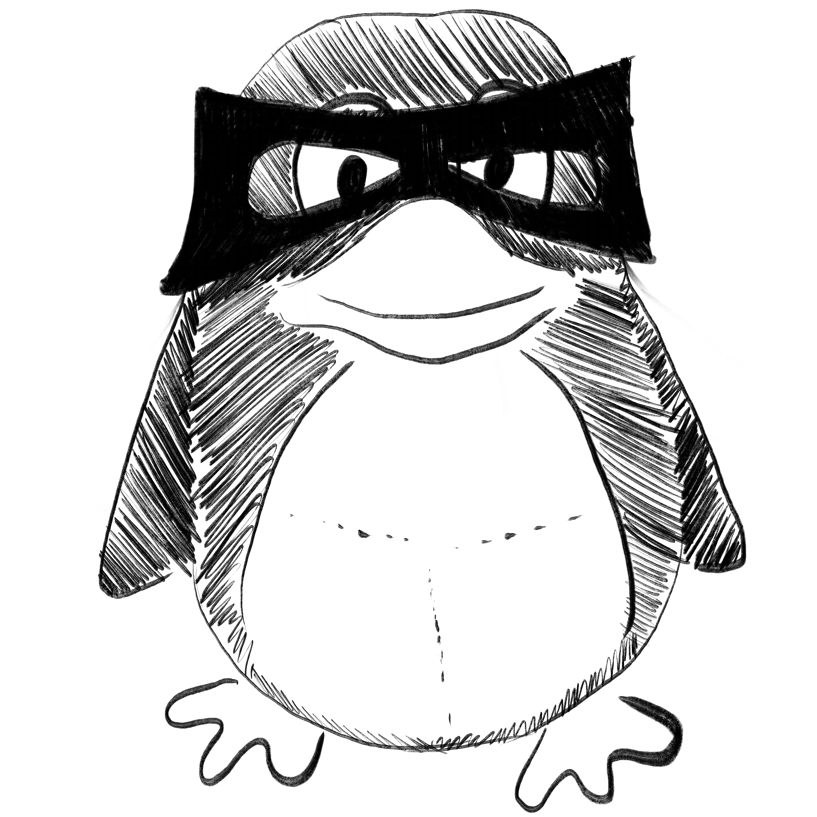
The role of cryptocurrencies in predicting oil prices pre and during COVID-19 pandemic using machine learning.
In Annals of operations research
Ibrahim Bassam A, Elamer Ahmed A, Abdou Hussein A
2022-Oct-28
Bitcoin, COVID-19, Crude oil, Cryptocurrencies, Machine learning, Neural networks
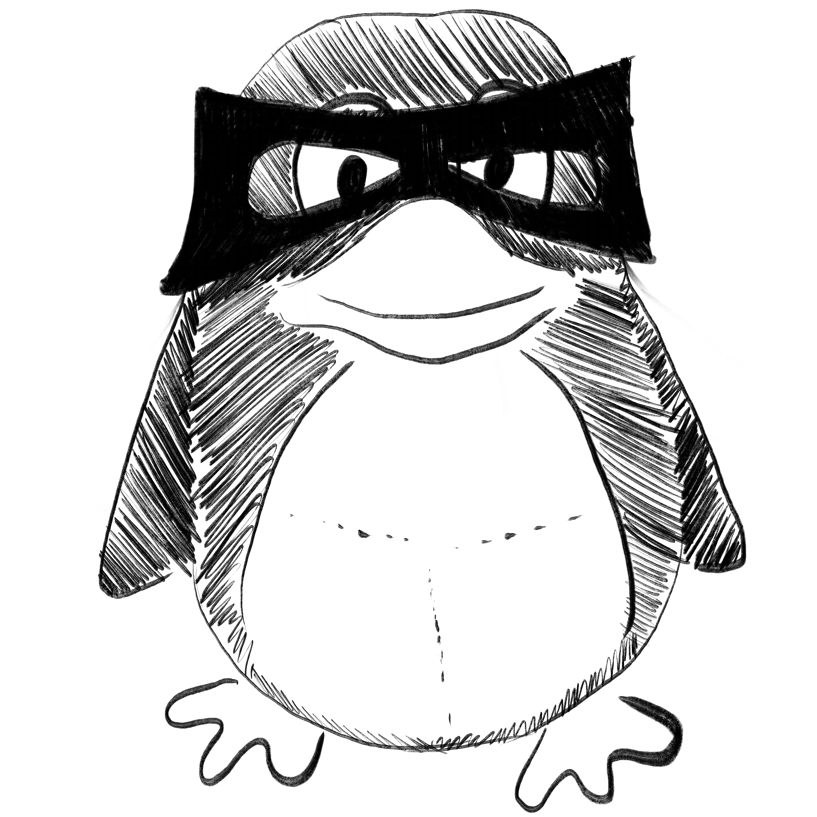
Interpretable tourism demand forecasting with temporal fusion transformers amid COVID-19.
In Applied intelligence (Dordrecht, Netherlands)
Wu Binrong, Wang Lin, Zeng Yu-Rong
2022-Oct-27
COVID-19, Deep learning, Interpretable tourism demand forecasting, Text mining
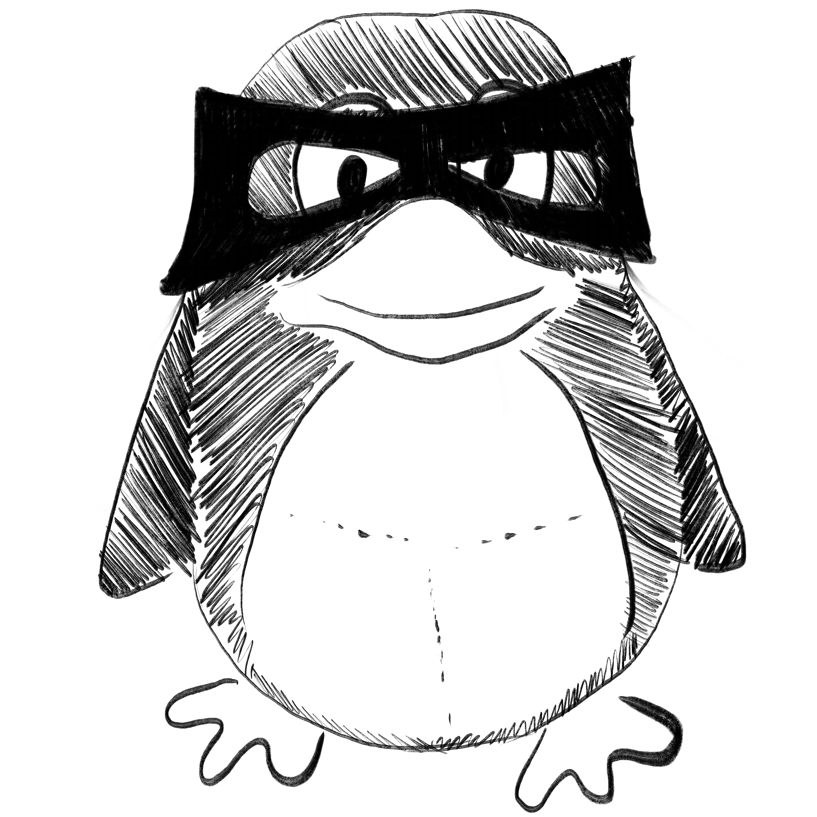
The m7G Modification Level and Immune Infiltration Characteristics in Patients with COVID-19.
In Journal of multidisciplinary healthcare
Purpose :
Patients and Methods :
Results :
Conclusion :
Lu Lingling, Zheng Jiaolong, Liu Bang, Wu Haicong, Huang Jiaofeng, Wu Liqing, Li Dongliang
2022
7-methylguanosine, COVID-19, SARS-CoV-2, immune cells, nomogram, risk
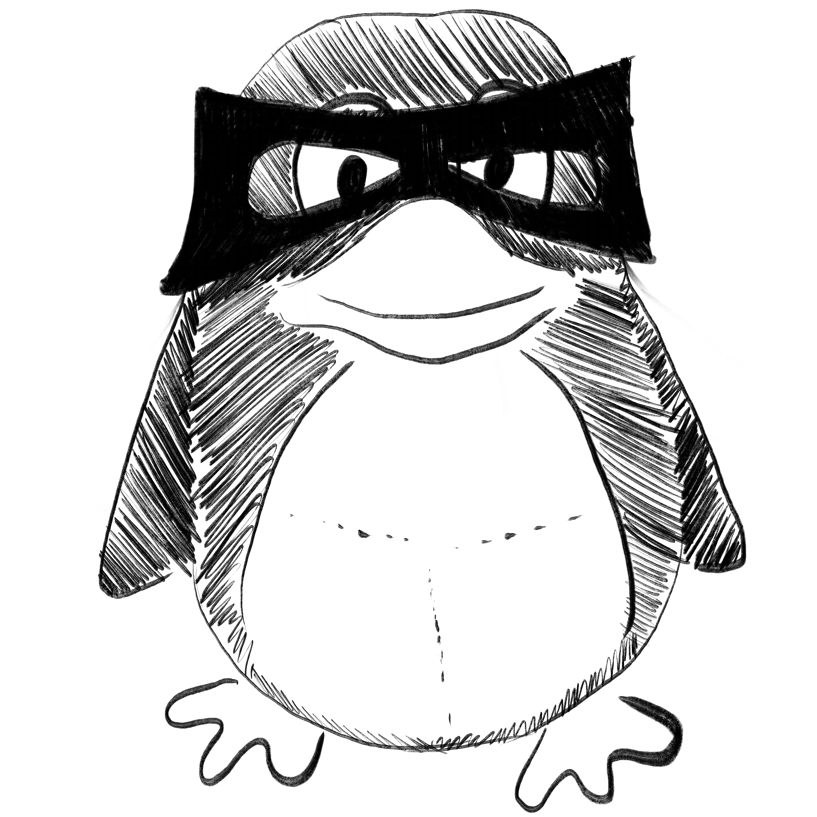
A comparison of Covid-19 cases and deaths in Turkey and in other countries.
In Network modeling and analysis in health informatics and bioinformatics
Çağlar Oğuzhan, Özen Figen
2022
Artificial neural network, Covid-19, Decision tree, Linear regression, Polynomial regression, Support vector regression
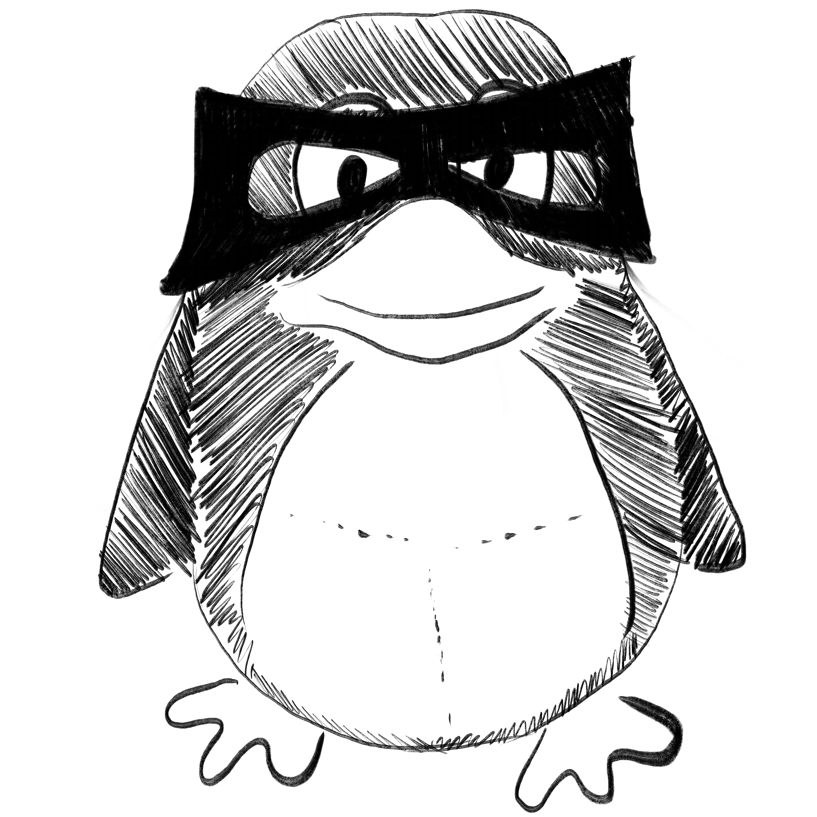
A robust semantic lung segmentation study for CNN-based COVID-19 diagnosis.
In Chemometrics and intelligent laboratory systems : an international journal sponsored by the Chemometrics Society
Aslan Muhammet Fatih
2022-Dec-15
AlexNet, COVID-19, Convolutional neural networks, DeepLabV3+, Semantic segmentation, Support vector machine
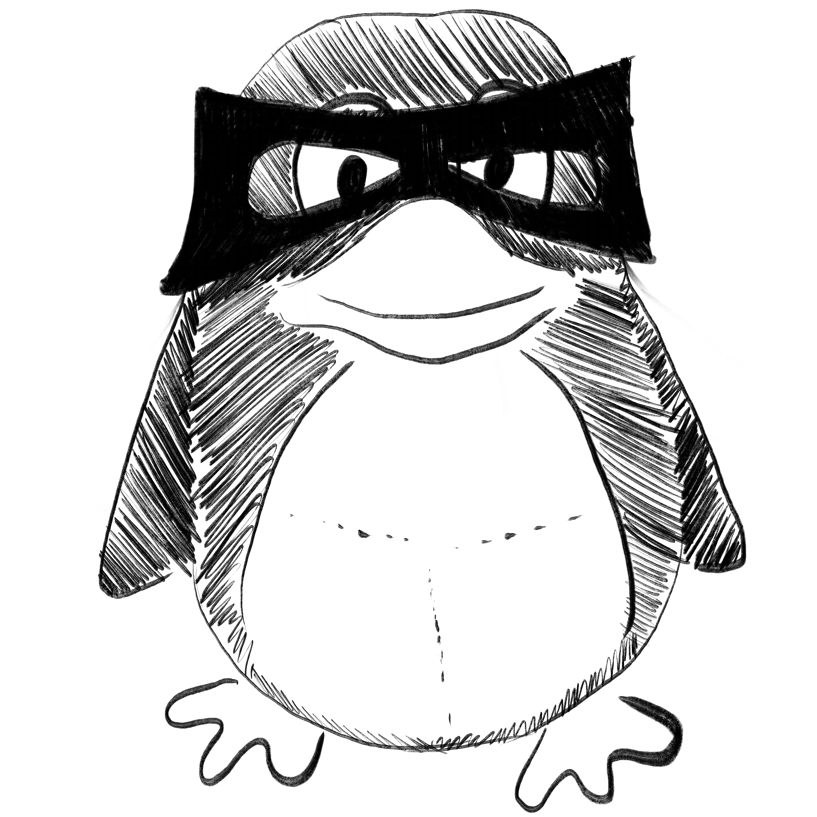
Predictive models for COVID-19 detection using routine blood tests and machine learning.
In Heliyon
Kistenev Yury V, Vrazhnov Denis A, Shnaider Ekaterina E, Zuhayri Hala
2022-Oct
Blood tests, COVID-19, Machine learning
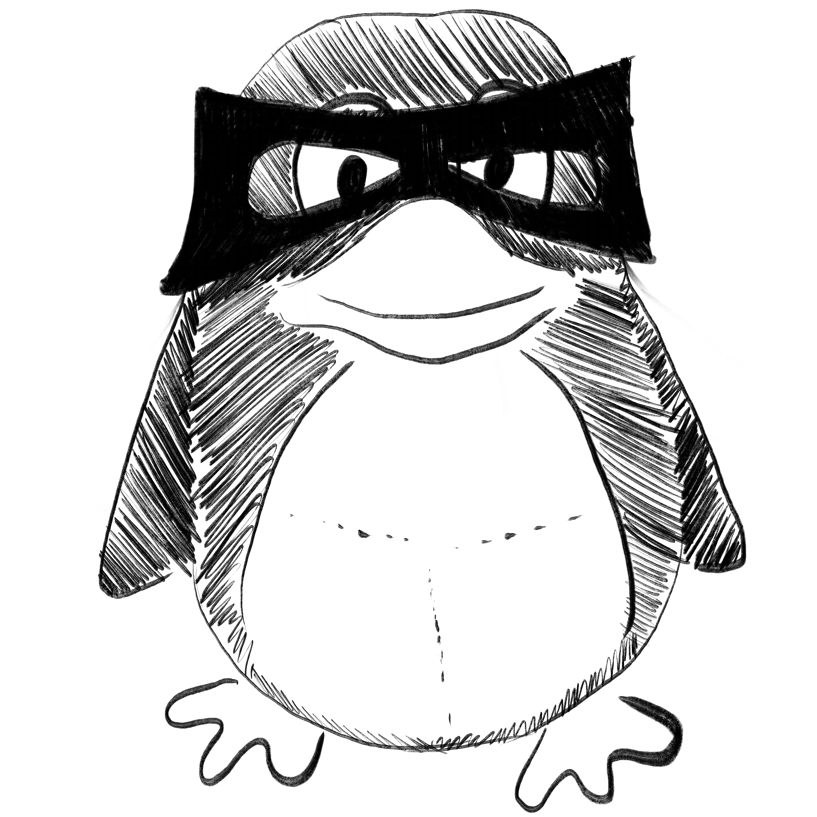
Defending against adversarial attacks on Covid-19 classifier: A denoiser-based approach.
In Heliyon
Kansal Keshav, Krishna P Sai, Jain Parshva B, R Surya, Honnavalli Prasad, Eswaran Sivaraman
2022-Oct
Adversarial attacks, Deep neural network, Denoiser, FGSM, HGD, Machine learning, PGD
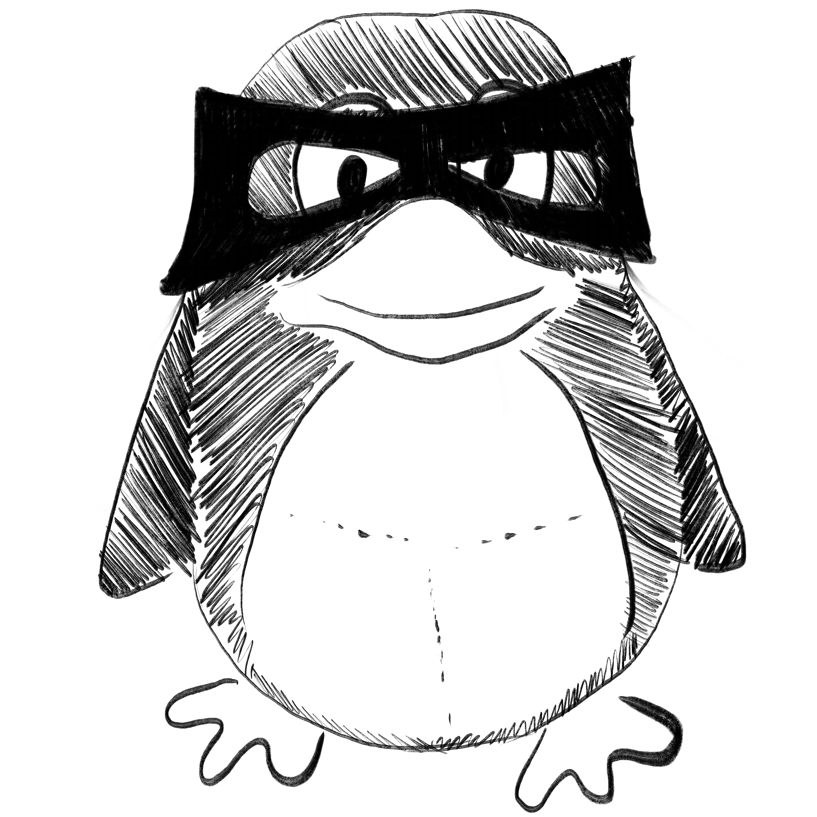
Masked Facial Recognition in Security Systems Using Transfer Learning.
In SN computer science
Ramgopal M, Roopesh M Sai, Chowdary M Veeranna, Madhav M, Shanmuga K
2023
Convolutional neural networks (CNN), Face recognition, Machine learning (ML), Object detection model, Transfer learning model
The Recent Advances of Mobile Healthcare in Cardiology Practice.
In Acta informatica medica : AIM : journal of the Society for Medical Informatics of Bosnia & Herzegovina : casopis Drustva za medicinsku informatiku BiH
Background :
Objective :
Methods :
Results and Discussion :
Conclusion :
Kulbayeva Shynar, Tazhibayeva Karlygash, Seiduanova Laura, Smagulova Indira, Mussina Aiman, Tanabayeva Shynar, Fakhradiyev Ildar, Saliev Timur
2022-Sep
cardiology, mobile app, mobile applications, telemedicine
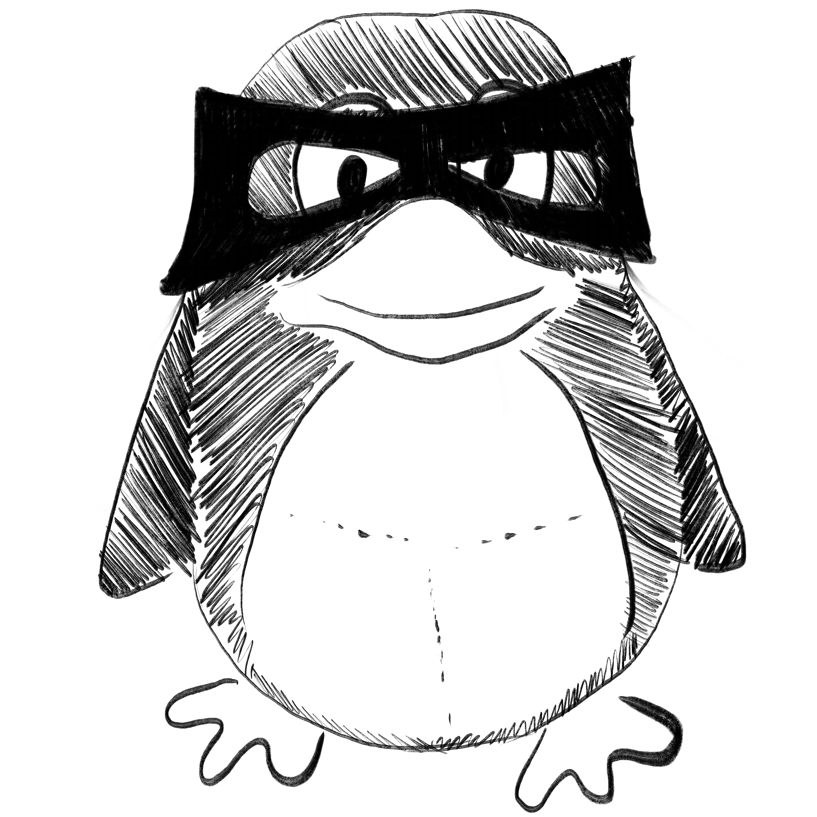
Digital phenotyping for classification of anxiety severity during COVID-19.
In Frontiers in digital health
Nguyen Binh, Ivanov Martin, Bhat Venkat, Krishnan Sri
2022
COVID-19, anxiety, digital phenotyping, machine learning, mental health
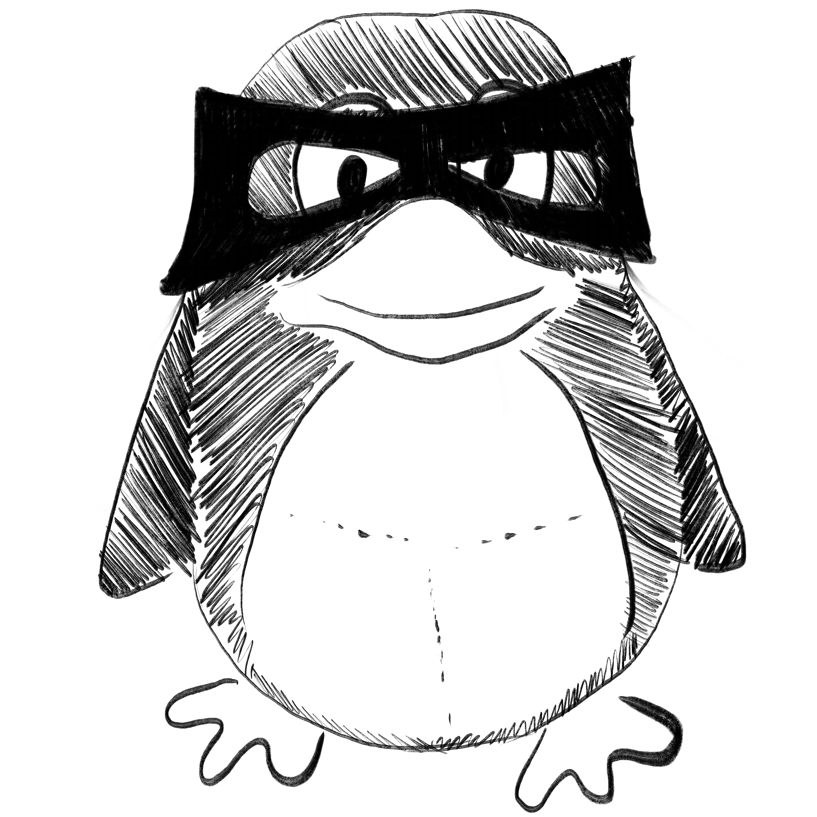
Learning effective embedding for automated COVID-19 prediction from chest X-ray images.
In Multimedia systems
T N Sree Ganesh, Satish Rishi, Sridhar Rajeswari
2022-Oct-26
AlexNet, COVID-19 prediction, Convolution neural network, Medical image classification, Multitask learning, Siamese neural network, Transfer learning, VGG16
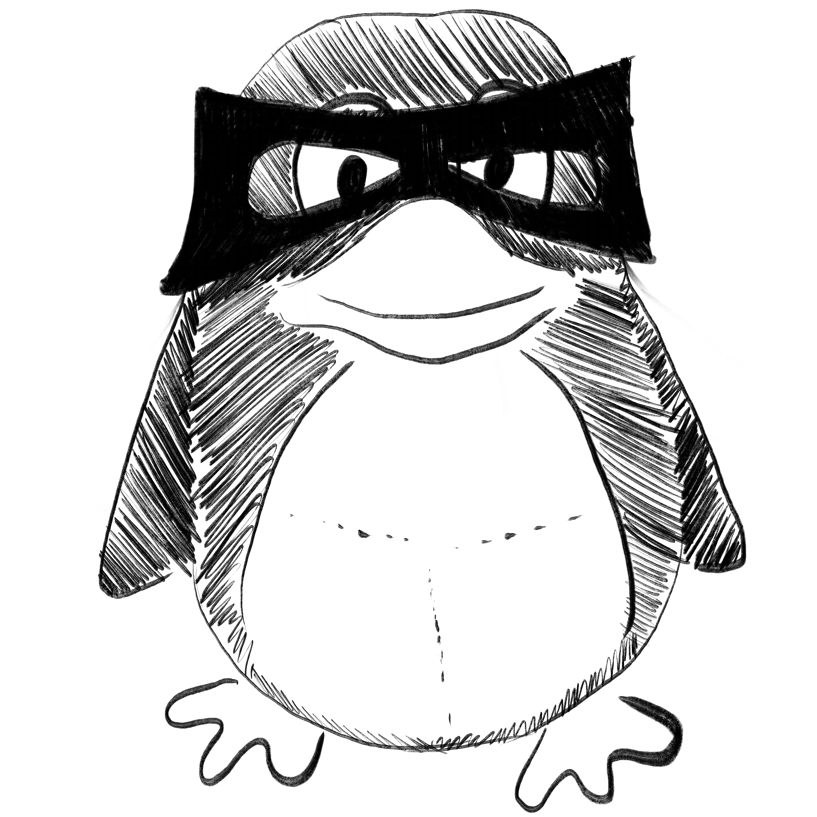
Modelling and forecasting new cases of Covid-19 in Nigeria: Comparison of regression, ARIMA and machine learning models.
In Scientific African
Busari S I, Samson T K
2022-Nov
ARIMA, Forecasting, Machine learning, Time series
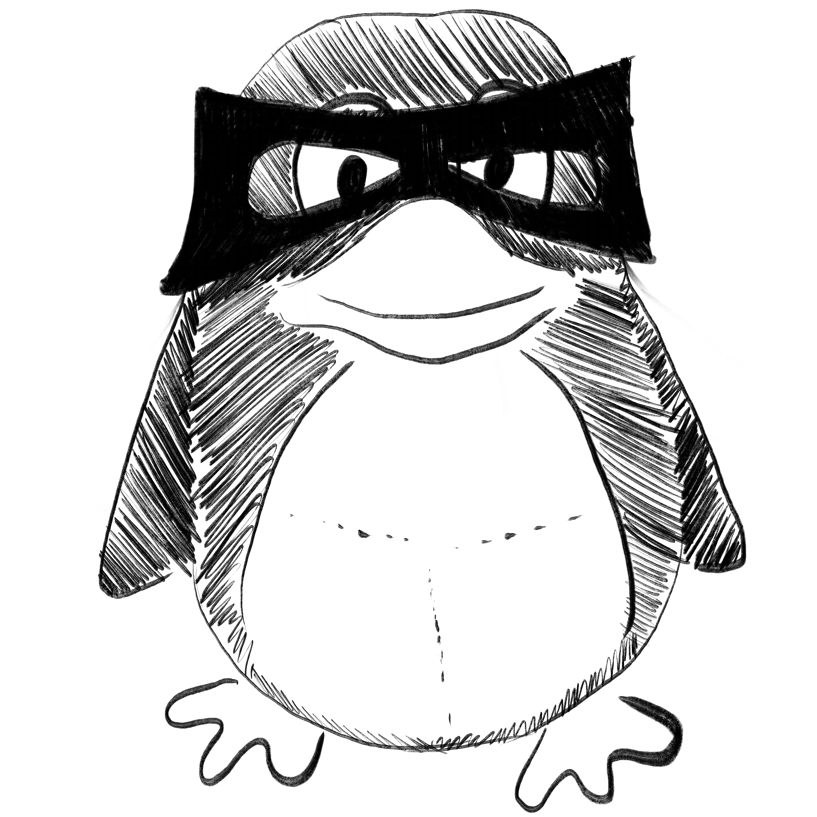
Ultrasensitive NO Sensor Based on a Nickel Single-Atom Electrocatalyst for Preliminary Screening of COVID-19.
In ACS sensors
Zhou Wei, Tan Yi, Ma Jing, Wang Xiao, Yang Li, Li Zhen, Liu Chengcheng, Wu Hao, Sun Lei, Deng Weiqiao
2022-Oct-31
COVID-19, Ni-N2O2/AB, mini-exhaled nitric oxide sensor, preliminary screening, single-atom catalysts
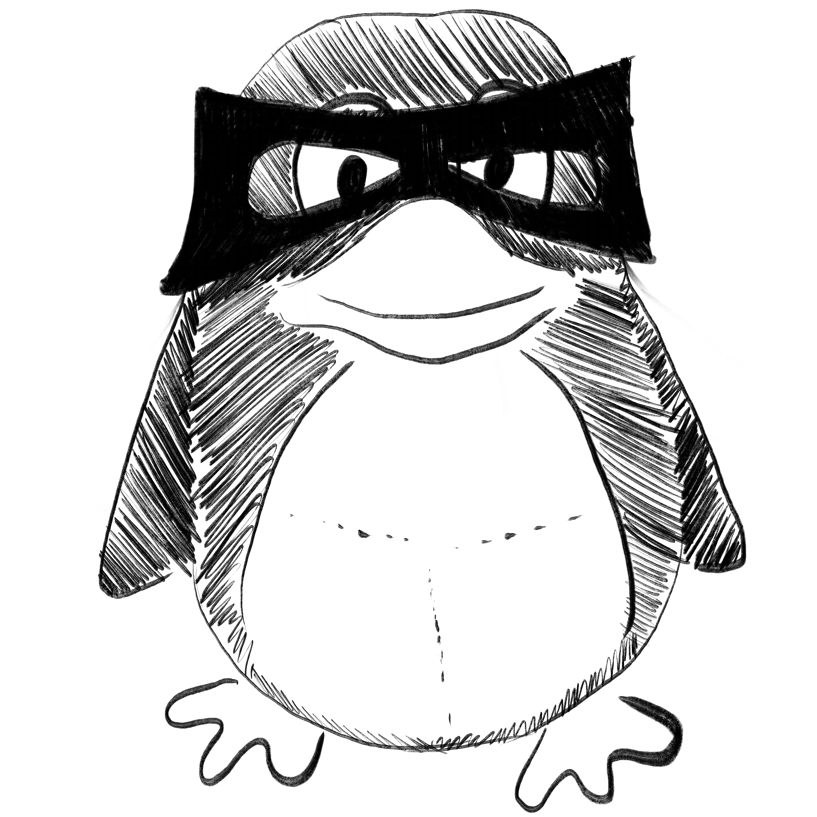
Artificial intelligence-based approaches for traditional fermented alcoholic beverages' development: review and prospect.
In Critical reviews in food science and nutrition ; h5-index 70.0
Yu Huakun, Liu Shuangping, Qin Hui, Zhou Zhilei, Zhao Hongyuan, Zhang Suyi, Mao Jian
2022-Oct-31
Traditional fermented alcoholic beverages, artificial intelligence, big data, fermentation regulation, microbial community
Severity detection of COVID-19 infection with machine learning of clinical records and CT images.
In Technology and health care : official journal of the European Society for Engineering and Medicine
BACKGROUND :
OBJECTIVE :
METHOD :
RESULTS :
CONCLUSION :
Zhu Fubao, Zhu Zelin, Zhang Yijun, Zhu Hanlei, Gao Zhengyuan, Liu Xiaoman, Zhou Guanbin, Xu Yan, Shan Fei
2022-Oct-21
COVID-19, CT radiomics features, Severity detection, clinical features, imbalance classification
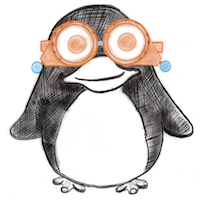
Acceptance and Perception of Artificial Intelligence Usability in Eye Care (APPRAISE) for Ophthalmologists: A Multinational Perspective.
In Frontiers in medicine
Background :
Methods :
Results :
Conclusion :
Gunasekeran Dinesh V, Zheng Feihui, Lim Gilbert Y S, Chong Crystal C Y, Zhang Shihao, Ng Wei Yan, Keel Stuart, Xiang Yifan, Park Ki Ho, Park Sang Jun, Chandra Aman, Wu Lihteh, Campbel J Peter, Lee Aaron Y, Keane Pearse A, Denniston Alastair, Lam Dennis S C, Fung Adrian T, Chan Paul R V, Sadda SriniVas R, Loewenstein Anat, Grzybowski Andrzej, Fong Kenneth C S, Wu Wei-Chi, Bachmann Lucas M, Zhang Xiulan, Yam Jason C, Cheung Carol Y, Pongsachareonnont Pear, Ruamviboonsuk Paisan, Raman Rajiv, Sakamoto Taiji, Habash Ranya, Girard Michael, Milea Dan, Ang Marcus, Tan Gavin S W, Schmetterer Leopold, Cheng Ching-Yu, Lamoureux Ecosse, Lin Haotian, van Wijngaarden Peter, Wong Tien Y, Ting Daniel S W
2022
artificial intelligence (AI), implementation, ophthalmology, regulation, translation
A comprehensive review on variants of SARS-CoVs-2: Challenges, solutions and open issues.
In Computer communications
Deepanshi Budhiraja, Ishan Garg, Deepak Kumar, Neeraj Sharma
2022-Oct-26
COVID-19, Deep learning, Game theory, Machine learning, SARS-CoV-2, Variants
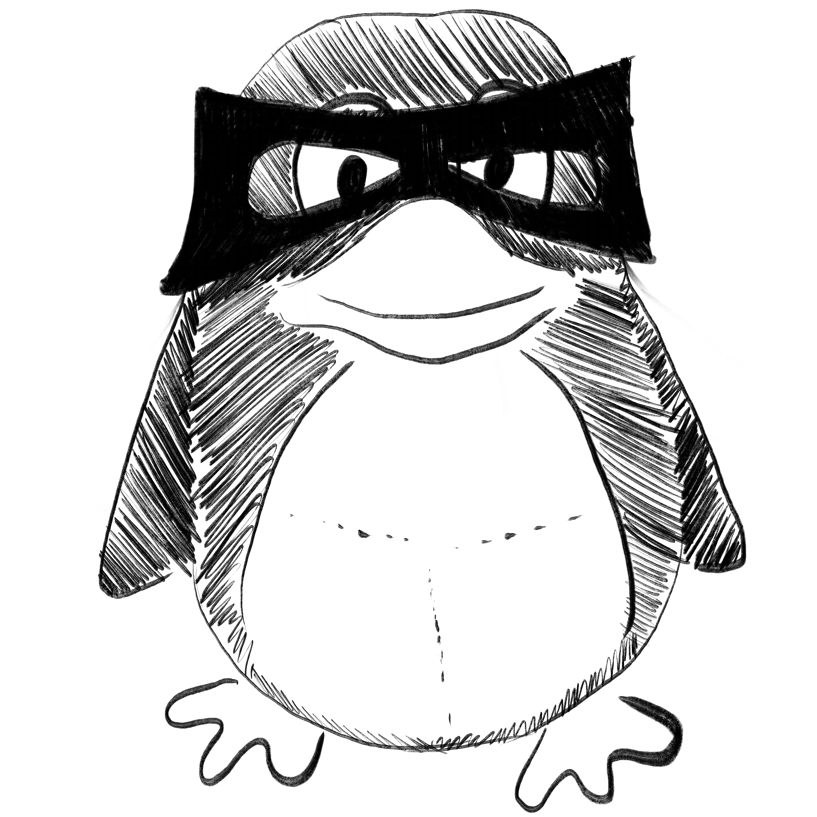
Ensemble hybrid model for Hindi COVID-19 text classification with metaheuristic optimization algorithm.
In Multimedia tools and applications
Jain Vipin, Kashyap Kanchan Lata
2022-Oct-24
COVID-19, Deep learning, Ensemble learning, Grey wolf, Optimization, Sentiment
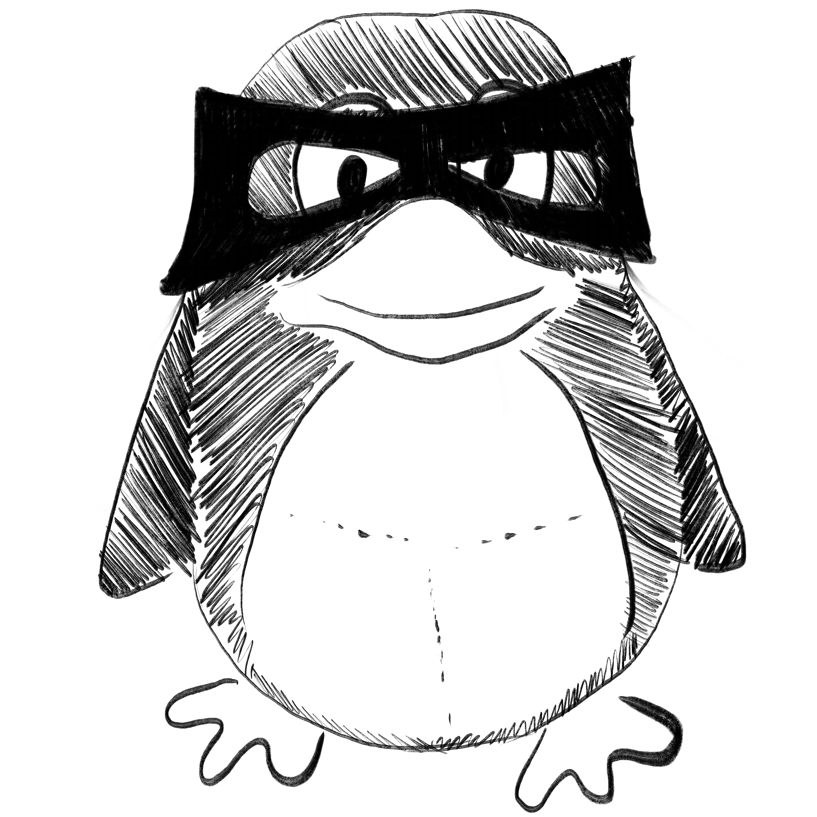
A hybrid LBP-DCNN based feature extraction method in YOLO: An application for masked face and social distance detection.
In Multimedia tools and applications
Oztel Ismail, Yolcu Oztel Gozde, Akgun Devrim
2022-Oct-21
Covid-19, Deep learning, Face mask detection, Human detection
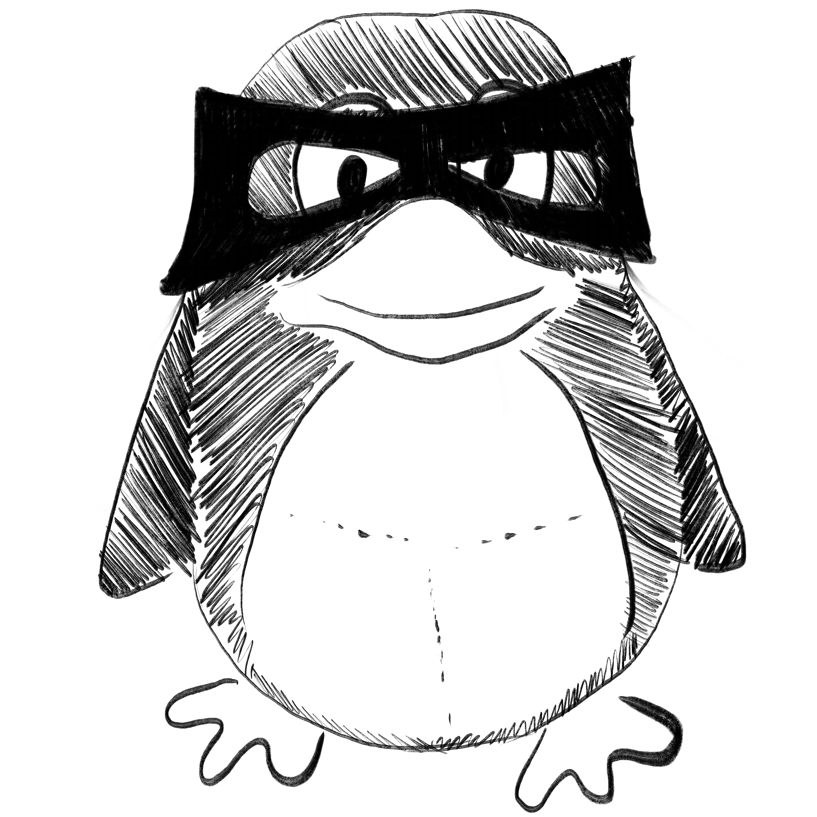
From luxury to necessity: Progress of touchless interaction technology.
In Technology in society
Iqbal Muhammad Zahid, Campbell Abraham G
2021-Nov
Contactless, Gestures, Human computer interaction, Touchless interaction, Touchless technology, Zero UI, Zero touch
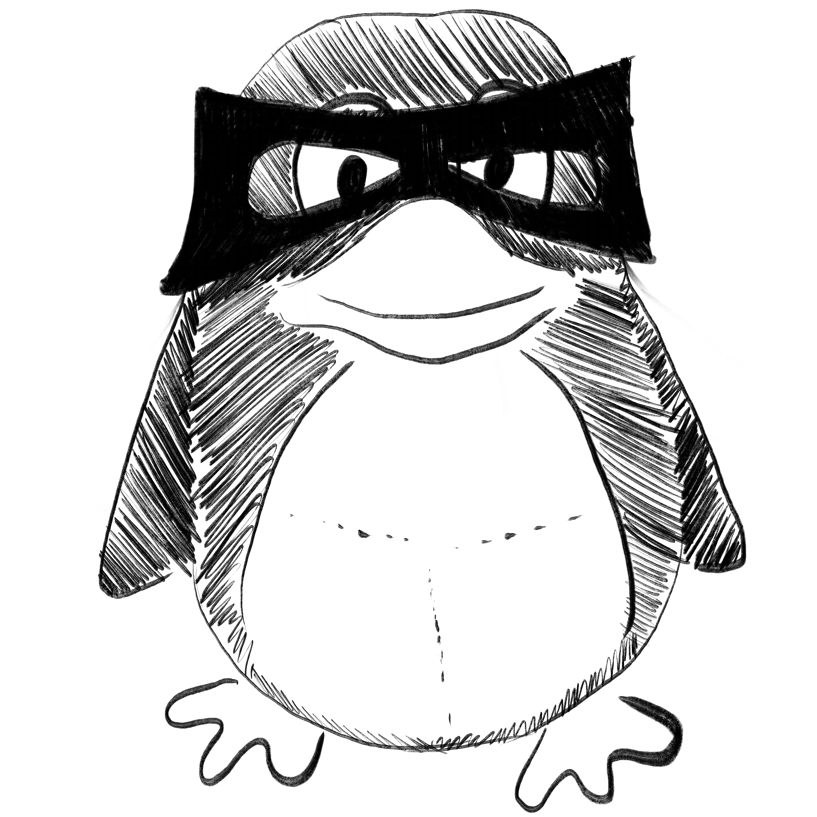
Boosted crow search algorithm for handling multi-threshold image problems with application to X-ray images of COVID-19.
In Expert systems with applications
Zhao Songwei, Wang Pengjun, Heidari Ali Asghar, Zhao Xuehua, Chen Huiling
2023-Mar-01
2D histogram, COVID-19, Crow search algorithm, Multi-threshold image segmentation, Optimization, Renyi’s entropy
Application of artificial intelligence in diagnosing COVID-19 disease symptoms on chest X-rays: A systematic review.
In International journal of medical sciences
Kufel Jakub, Bargieł Katarzyna, Koźlik Maciej, Czogalik Łukasz, Dudek Piotr, Jaworski Aleksander, Cebula Maciej, Gruszczyńska Katarzyna
2022
COVID-19, artificial intelligence, chest X-rays, convolutional neural network, diagnostic imaging
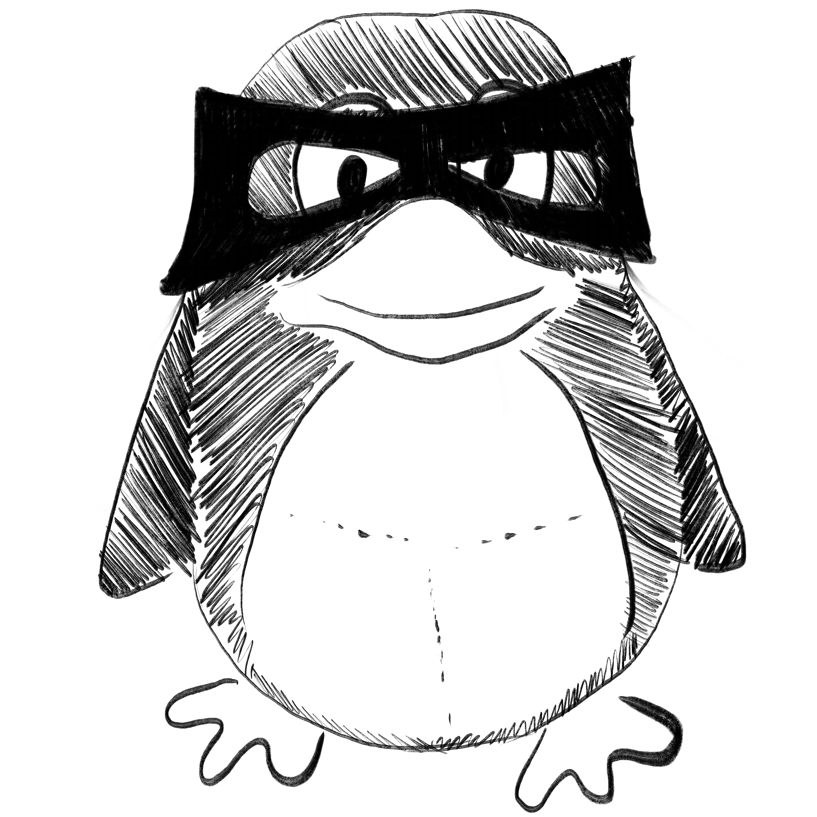
Towards Smart Diagnostic Methods for COVID-19: Review of Deep Learning for Medical Imaging.
In IPEM-translation
Moghaddam Marjan Jalali, Ghavipour Mina
2022-Oct-26
Artificial Intelligence, CT-Scan, Classification, Segmentation, X-ray
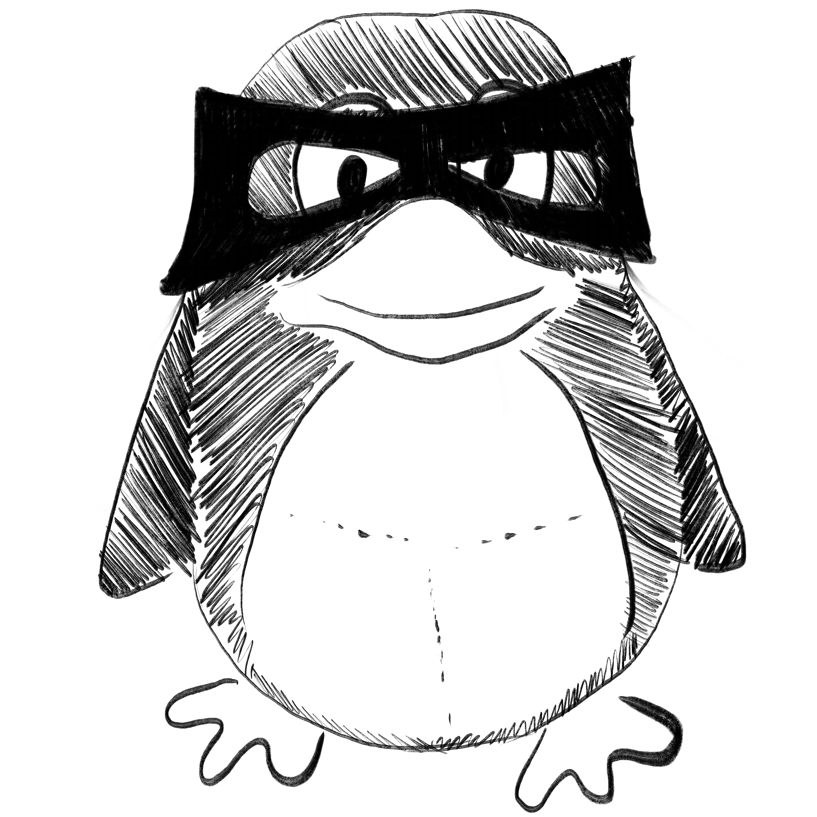
A deep transfer learning-based convolution neural network model for COVID-19 detection using Computed tomography scan images for medical applications.
In Advances in engineering software (Barking, London, England : 1992)
Kathamuthu Nirmala Devi, Subramaniam Shanthi, Le Q H, Muthusamy Suresh, Panchal Hitesh, Sundararajan Suma Christal Mary, Alruabie Ali Jawad, Zahra Musaddak Maher Abdul
2022-Oct-24
CNN, Covid-19, Deep Learning, DenseNet121, InceptionV3, ResNet-50, Transfer learning, VGG16, VGG19
Deep-learning-based hepatic fat assessment (DeHFt) on non-contrast chest CT and its association with disease severity in COVID-19 infections: A multi-site retrospective study.
In EBioMedicine
BACKGROUND :
METHODS :
FINDINGS :
INTERPRETATION :
FUNDING :
Modanwal Gourav, Al-Kindi Sadeer, Walker Jonathan, Dhamdhere Rohan, Yuan Lei, Ji Mengyao, Lu Cheng, Fu Pingfu, Rajagopalan Sanjay, Madabhushi Anant
2022-Oct-26
COVID-19, Hepatic steatosis, NAFLD
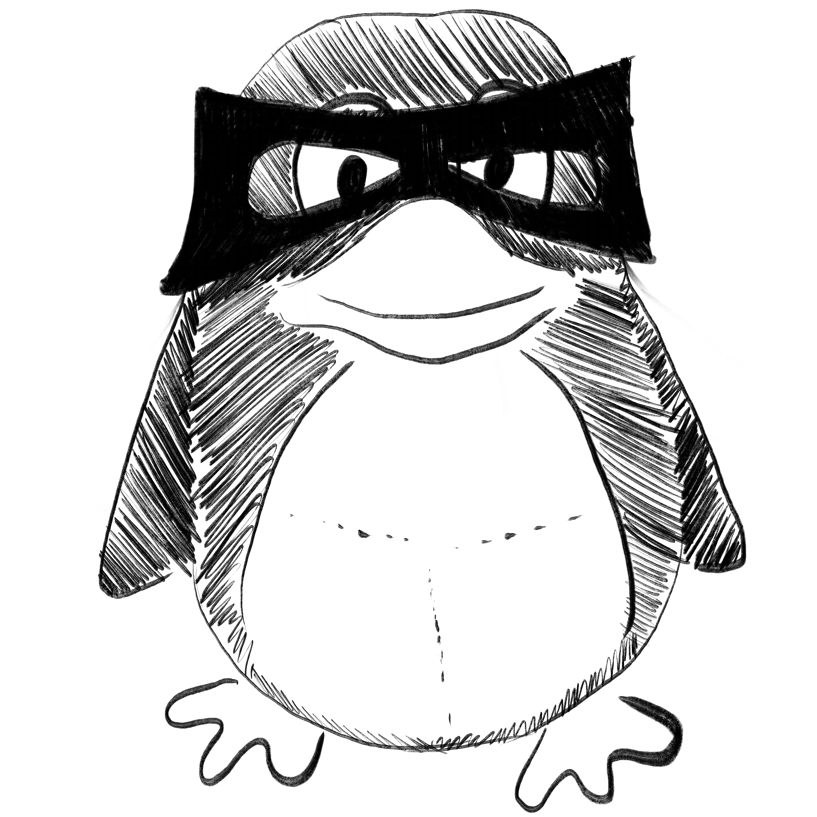
Multisite Evaluation of Prediction Models for Emergency Department Crowding Before and During the COVID-19 Pandemic.
In Journal of the American Medical Informatics Association : JAMIA
OBJECTIVE :
MATERIALS AND METHODS :
RESULTS :
DISCUSSION AND CONCLUSION :
Smith Ari J, Patterson Brian W, Pulia Michael S, Mayer John, Schwei Rebecca J, Nagarajan Radha, Liao Frank, Shah Manish N, Boutilier Justin J
2022-Oct-29
COVID-19, Emergency medicine, data drift, emergency department boarding, machine learning
An exploration of challenges associated with machine learning for time series forecasting of COVID-19 community spread using wastewater-based epidemiological data.
In The Science of the total environment
Vaughan Liam, Zhang Muyang, Gu Haoran, Rose Joan, Naughton Colleen, Medema Gertjan, Allan Vajra, Roiko Anne, Blackall Linda, Zamyadi Arash
2022-Oct-25
COVID-19, Machine learning, Time series forecasting, Wastewater-based epidemiology
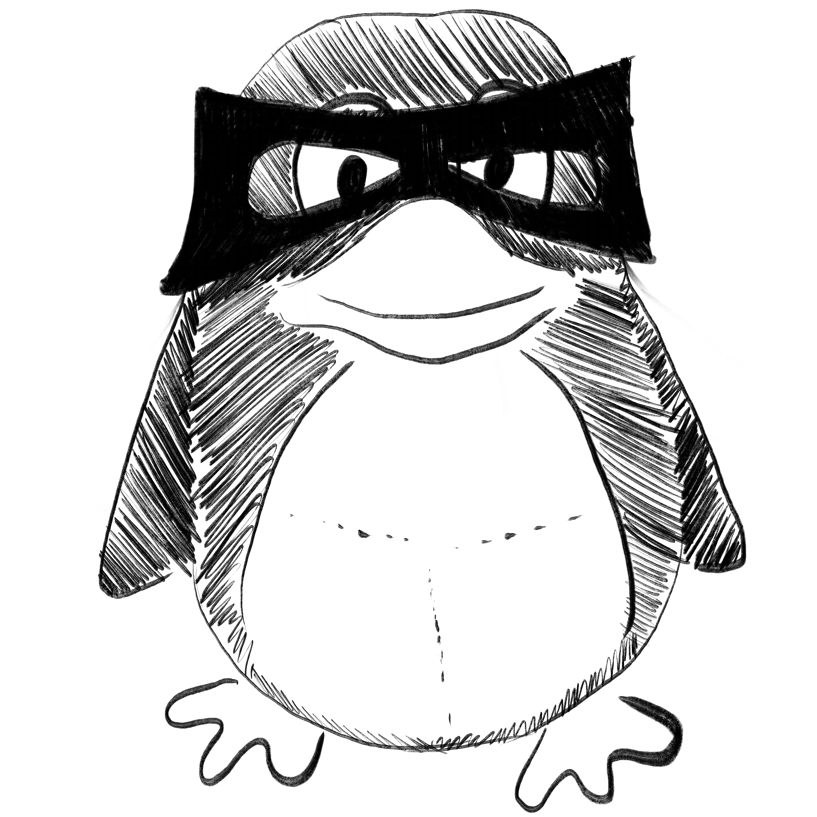
Assessing data gathering of chatbot based symptom checkers - a clinical vignettes study.
In International journal of medical informatics ; h5-index 49.0
BACKGROUND :
OBJECTIVES :
METHODS :
RESULTS :
CONCLUSION :
Ben-Shabat Niv, Sharvit Gal, Meimis Ben, Ben Joya Daniel, Sloma Ariel, Kiderman David, Shabat Aviv, Tsur Avishai M, Watad Abdulla, Amital Howard
2022-Oct-22
Artificial intelligence, Chatbots, Computer-assisted diagnosis, Data-gathering, Diagnosis, Medical interview, Symptom checker, Telemedicine, Triage
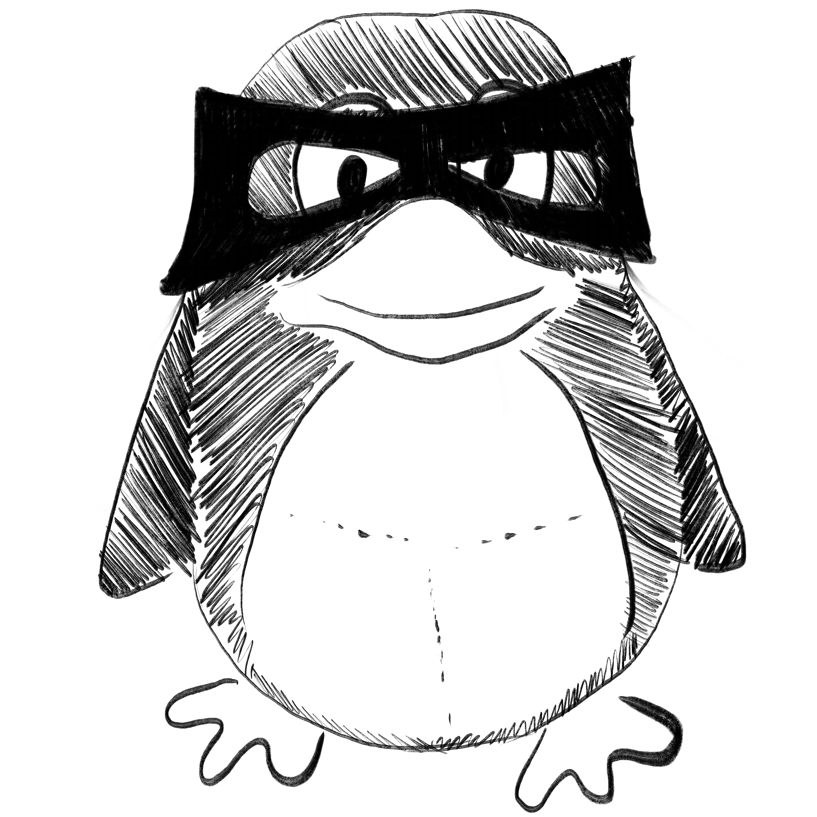
Genome-wide detection of human variants that disrupt intronic branchpoints.
In Proceedings of the National Academy of Sciences of the United States of America
Zhang Peng, Philippot Quentin, Ren Weicheng, Lei Wei-Te, Li Juan, Stenson Peter D, Palacín Pere Soler, Colobran Roger, Boisson Bertrand, Zhang Shen-Ying, Puel Anne, Pan-Hammarström Qiang, Zhang Qian, Cooper David N, Abel Laurent, Casanova Jean-Laurent
2022-Nov
branchpoint, disease genetics, intronic variant, software, splicing
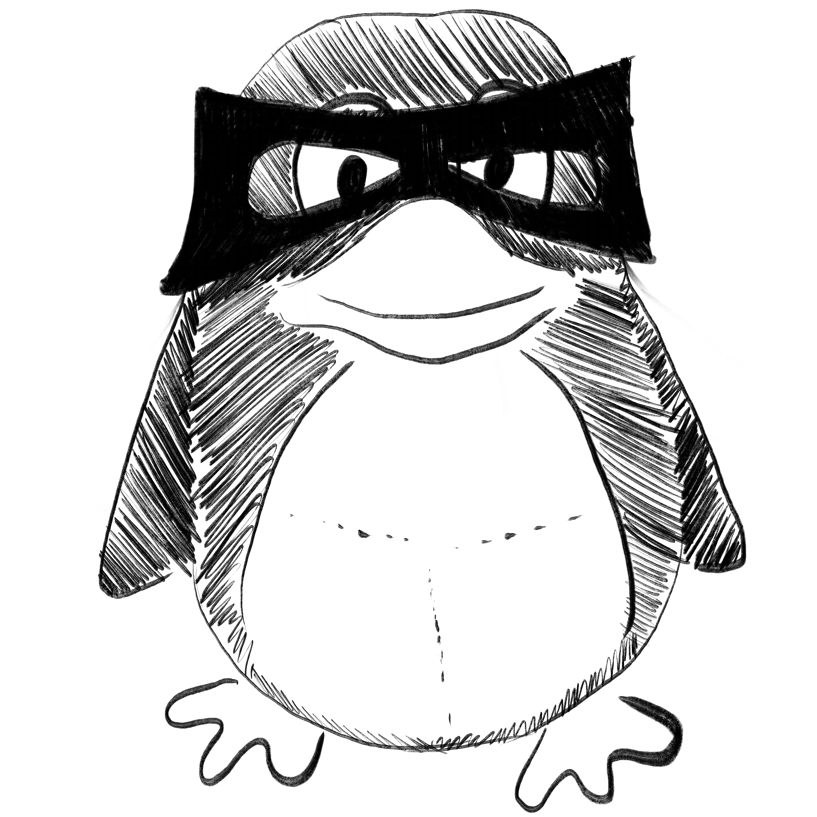
Artificial Intelligence and Deep Learning Assisted Rapid Diagnosis of COVID-19 from Chest Radiographical Images: A Survey.
In Contrast media & molecular imaging
Sinwar Deepak, Dhaka Vijaypal Singh, Tesfaye Biniyam Alemu, Raghuwanshi Ghanshyam, Kumar Ashish, Maakar Sunil Kr, Agrawal Sanjay
2022
Contrastive learning and subtyping of post-COVID-19 lung computed tomography images.
In Frontiers in physiology
Li Frank, Zhang Xuan, Comellas Alejandro P, Hoffman Eric A, Yang Tianbao, Lin Ching-Long
2022
PASC, cluster analysis, computed tomography, contrastive learning, long Covid, post-COVID-19, small airways disease
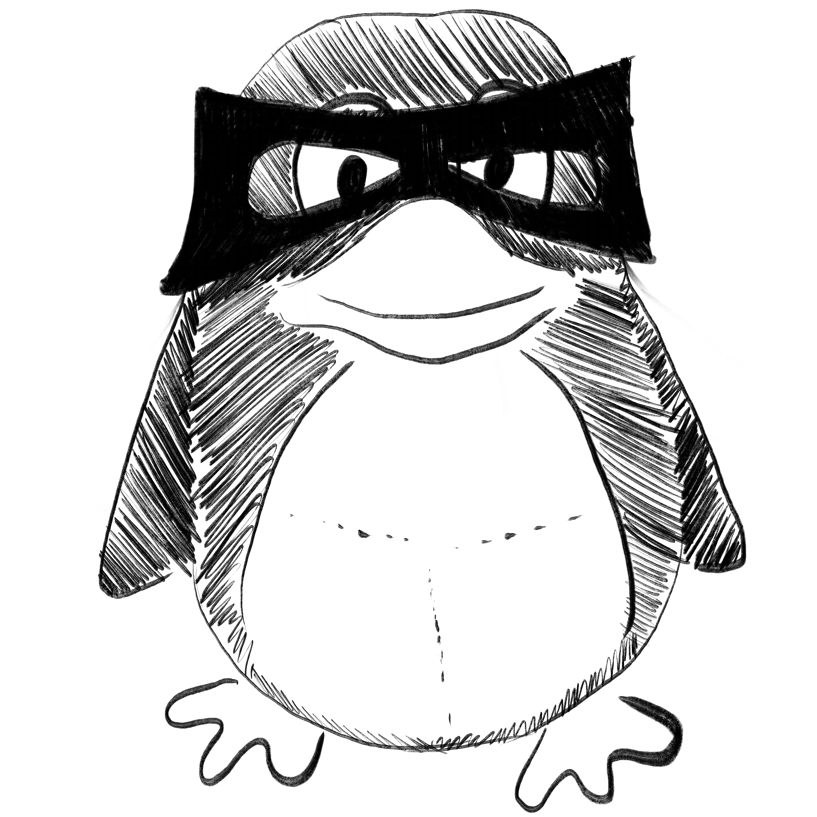
A multi-task FP-GNN framework enables accurate prediction of selective PARP inhibitors.
In Frontiers in pharmacology
Ai Daiqiao, Wu Jingxing, Cai Hanxuan, Zhao Duancheng, Chen Yihao, Wei Jiajia, Xu Jianrong, Zhang Jiquan, Wang Ling
2022
PARP, deep learning, interpretability, multi-task FP-GNN, online webserver
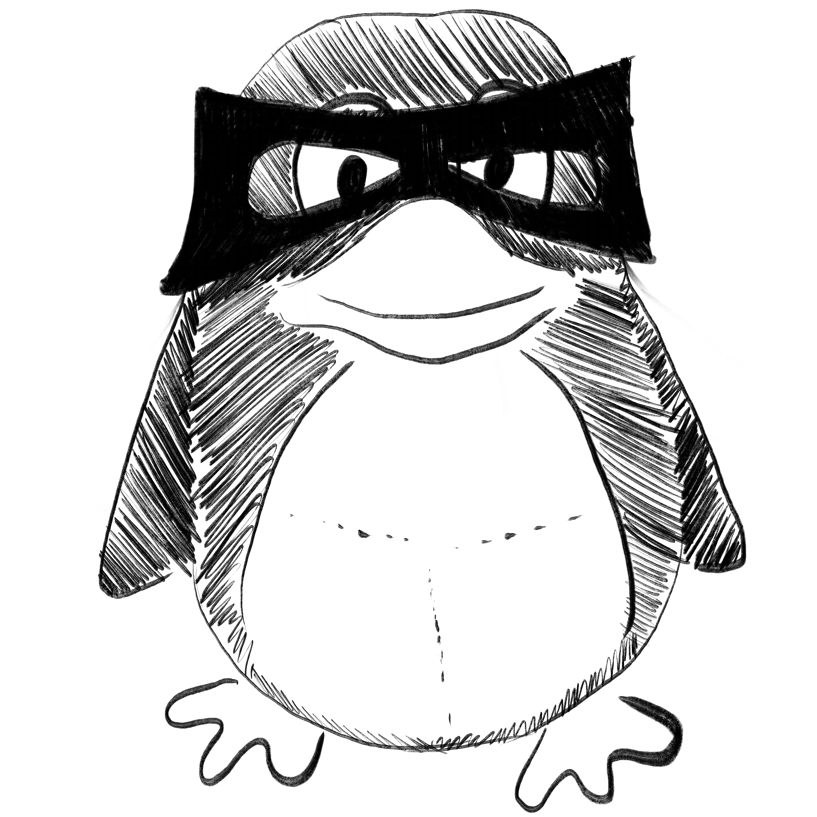
We are not ready yet: limitations of state-of-the-art disease named entity recognizers.
In Journal of biomedical semantics ; h5-index 23.0
BACKGROUND :
RESULTS :
CONCLUSIONS :
Kühnel Lisa, Fluck Juliane
2022-Oct-27
BERT, Manual Curation, Text mining, bioNLP
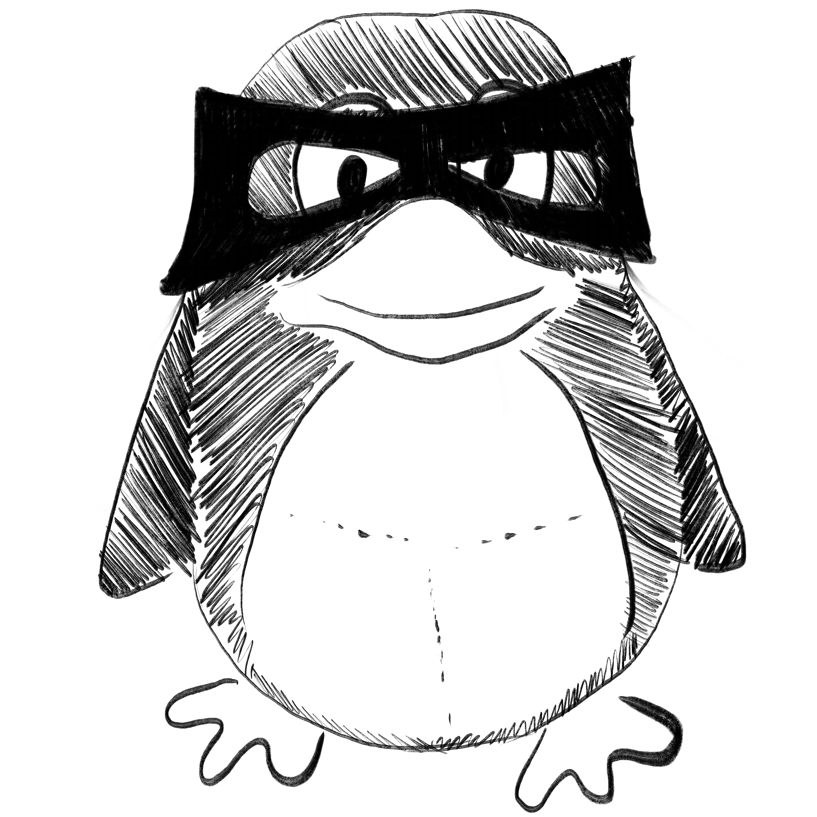
Vitamin D deficiency and SARS-CoV-2 infection: Big-data analysis from March 2020 to March 2021. D-COVID study
bioRxiv Preprint
Anguita, N.
2022-10-28
Machine learning predicts the short-term requirement for invasive ventilation among Australian critically ill COVID-19 patients.
In PloS one ; h5-index 176.0
OBJECTIVE(S) :
DESIGN :
PARTICIPANTS :
MAIN OUTCOME MEASURES :
RESULTS :
CONCLUSION :
Karri Roshan, Chen Yi-Ping Phoebe, Burrell Aidan J C, Penny-Dimri Jahan C, Broadley Tessa, Trapani Tony, Deane Adam M, Udy Andrew A, Plummer Mark P
2022
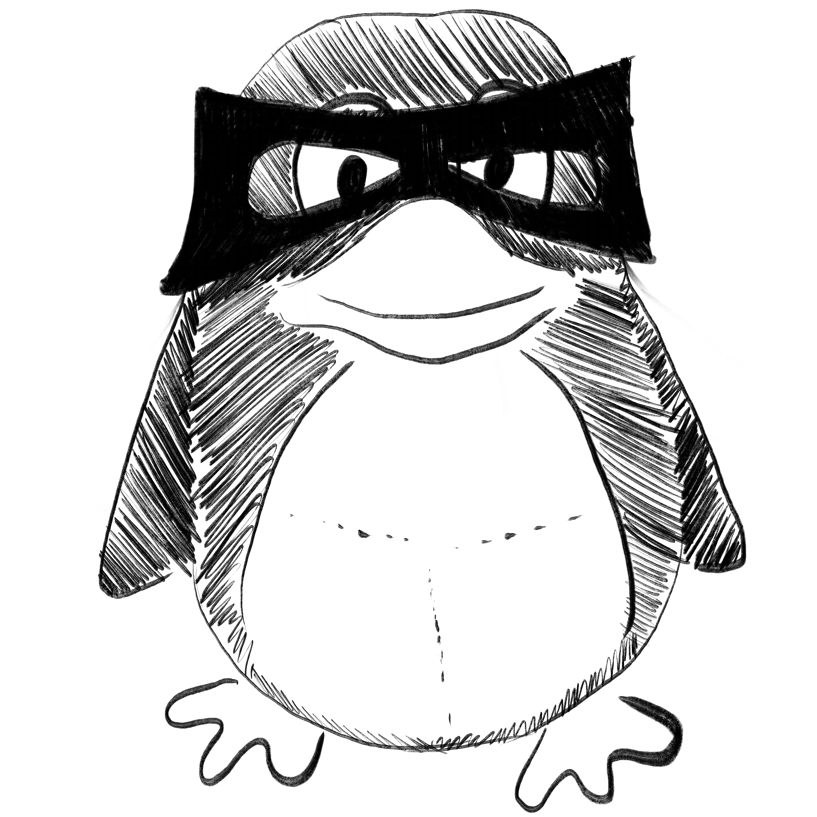
Pseudo-Label Guided Image Synthesis for Semi-Supervised COVID-19 Pneumonia Infection Segmentation.
In IEEE transactions on medical imaging ; h5-index 74.0
Lyu Fei, Ye Mang, Carlsen Jonathan Frederik, Erleben Kenny, Darkner Sune, Yuen Pong C
2022-Oct-26
Valuation of Costs in Health Economics During Financial and Economic Crises: A Case Study from Lebanon.
In Applied health economics and health policy
Dahham Jalal, Kremer Ingrid, Hiligsmann Mickaël, Hamdan Kamal, Nassereddine Abdallah, Evers Silvia M A A, Rizk Rana
2022-Oct-26
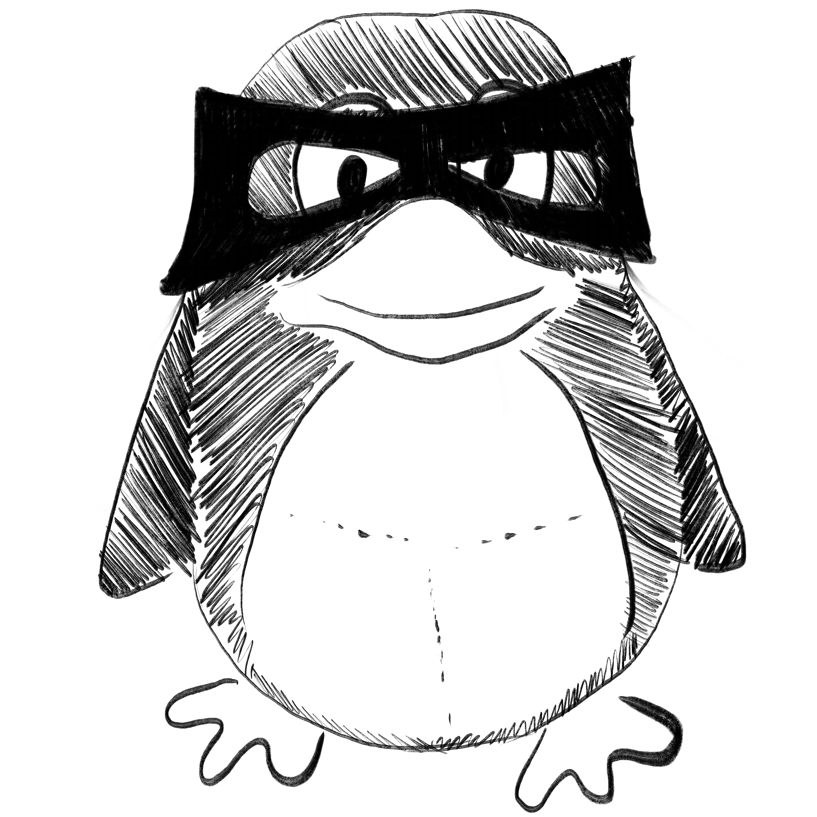
[The role of artificial intelligence in assessing the progression of fibrosing lung diseases].
In Terapevticheskii arkhiv
INTRODUCTION :
RESULTS :
CONCLUSION :
Speranskaia A A
2022-Mar-15
artificial intelligence, computer tomography, progressive fibrosing interstitial lung diseases
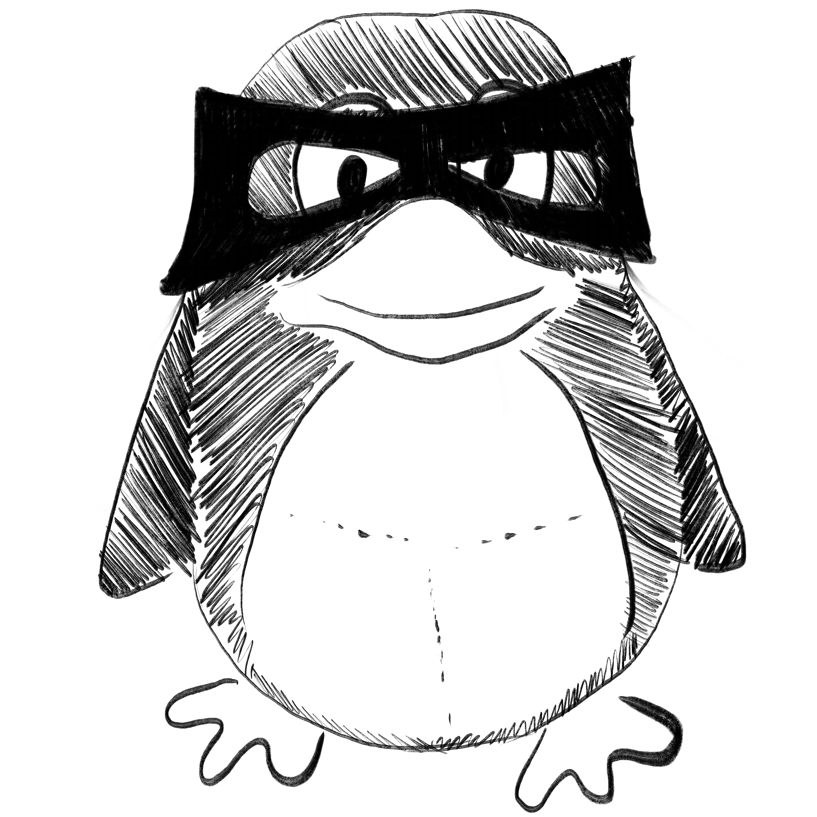
Comprehensive Survey of Machine Learning Systems for COVID-19 Detection.
In Journal of imaging
Alsaaidah Bayan, Al-Hadidi Moh’d Rasoul, Al-Nsour Heba, Masadeh Raja, AlZubi Nael
2022-Sep-30
COVID-19, CT images, augmentation, deep learning, diagnosis, machine learning, pneumonia
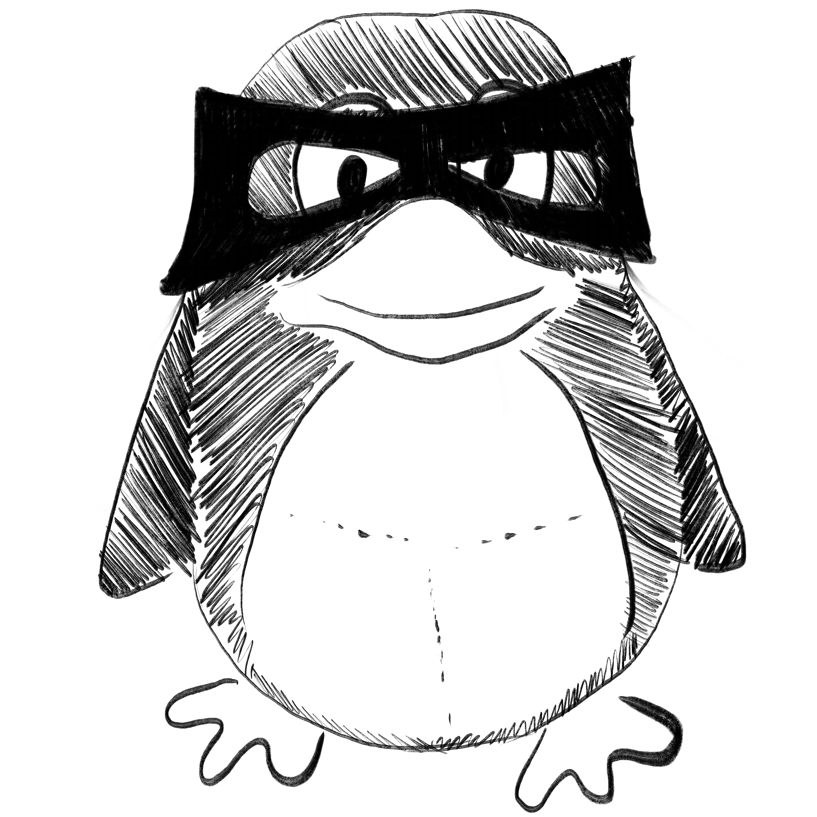
Deep Transfer Learning for COVID-19 Detection and Lesion Recognition Using Chest CT Images.
In Computational and mathematical methods in medicine
Zhang Sai, Yuan Guo-Chang
2022
A novel infrasound and audible machine-learning approach to the diagnosis of COVID-19.
In ERJ open research
Background :
Methods :
Results :
Conclusions :
Dori Guy, Bachner-Hinenzon Noa, Kasim Nour, Zaidani Haitem, Perl Sivan Haia, Maayan Shlomo, Shneifi Amin, Kian Yousef, Tiosano Tuvia, Adler Doron, Adir Yochai
2022-Oct
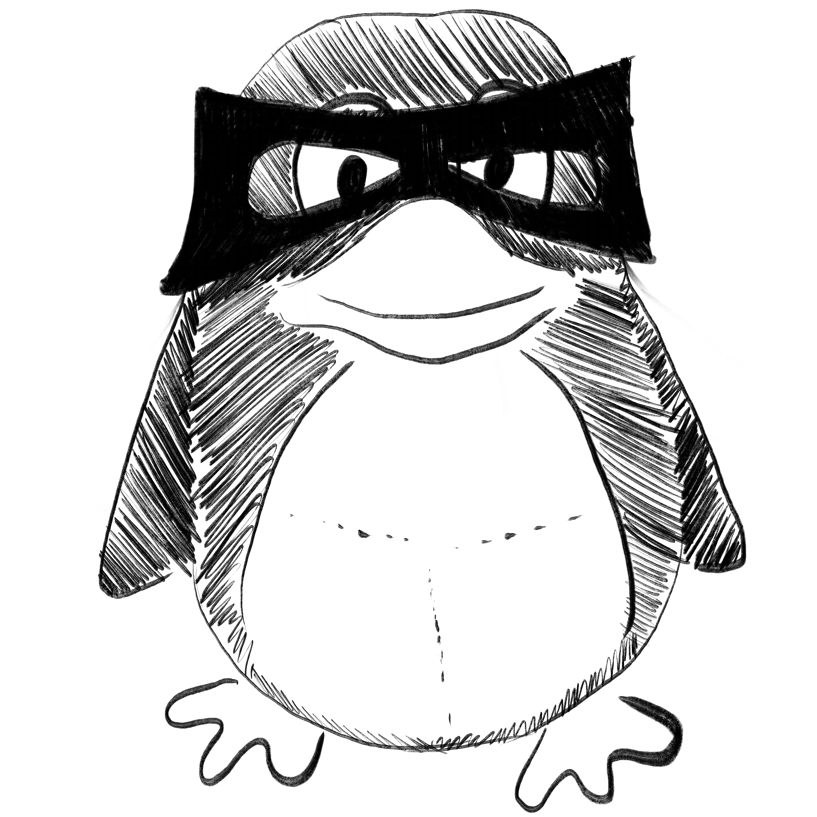
BND-VGG-19: A deep learning algorithm for COVID-19 identification utilizing X-ray images.
In Knowledge-based systems
Cao Zili, Huang Junjian, He Xing, Zong Zhaowen
2022-Oct-21
COVID-19, Classification, Diagnosis, VGG-19, X-ray image
COVID-19 Vaccination Rates of People Who Use Drugs - Chengdu City, Sichuan Province, China, November 2021 - February 2022.
In China CDC weekly
What is already known about this topic? :
What is added by this report? :
What are the implications for public health practice? :
Du Erri, Jiang Pengyu, Zhang Chaowei, Zhang Shan, Yan Xiangyu, Li Yongjie, Jia Zhongwei
2022-Sep-16
COVID-19, people who use drugs, vaccination
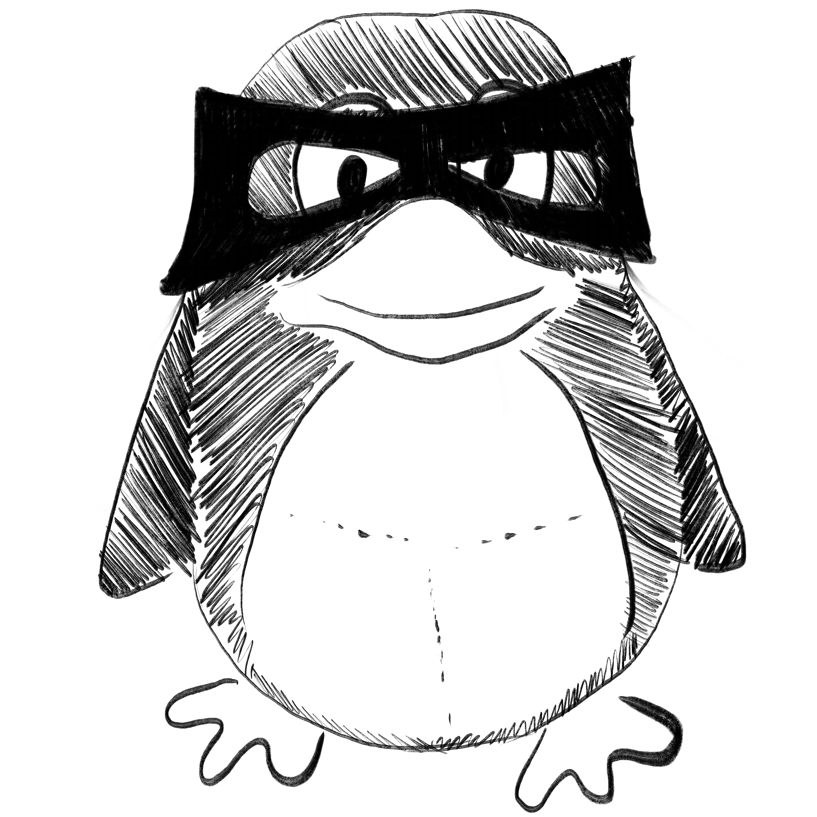
Can Artificial Intelligence Detect Monkeypox from Digital Skin Images?
bioRxiv Preprint
Islam, T.; Hussain, M.; Chowdhury, F. U. H.; Islam, B. M. R.
2022-10-27
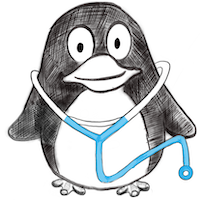
Natural Language Processing CAM Algorithm Improves Delirium Detection Compared With Conventional Methods.
In American journal of medical quality : the official journal of the American College of Medical Quality
Pagali Sandeep R, Kumar Rakesh, Fu Sunyang, Sohn Sunghwan, Yousufuddin Mohammed
2022-Oct-26
Deep learning modelling of public's sentiments towards temporal evolution of COVID-19 transmission.
In Applied soft computing
Wang Ying, Chew Alvin Wei Ze, Zhang Limao
2022-Oct-20
COVID-19 transmission, Deep learning, Global sentiment evolution, Natural language processing, Text sentiment classification, Twitter data
COVICT: an IoT based architecture for COVID-19 detection and contact tracing.
In Journal of ambient intelligence and humanized computing
Wahid Mirza Anas, Bukhari Syed Hashim Raza, Daud Ahmad, Awan Saeed Ehsan, Raja Muhammad Asif Zahoor
2022-Oct-20
COVID-19, Contact tracing, D2D communication, Early identification, Internet of things, Pandemic, Smart lockdown
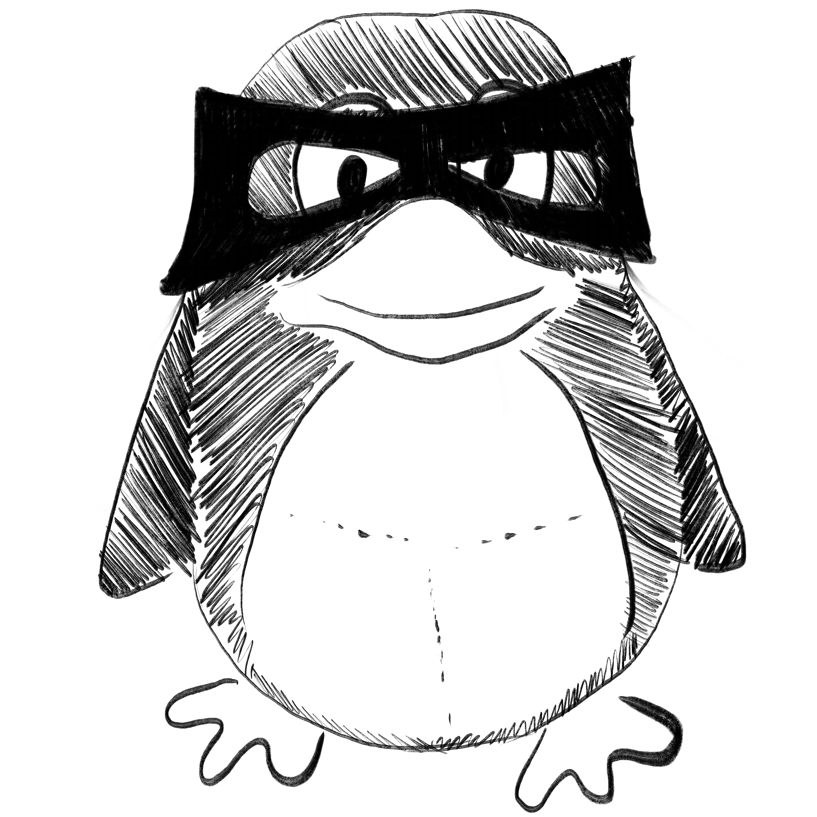
A machine learning study of COVID-19 serology and molecular tests and predictions.
In Smart health (Amsterdam, Netherlands)
Elkin Magdalyn E, Zhu Xingquan
2022-Oct-20
68T05, 68T50, 92C50, 92C55, 92C60, COVID-19, Classification, Machine Learning, Molecular test, Serology test, Symptoms
Diagnostic performance of corona virus disease 2019 chest computer tomography image recognition based on deep learning: Systematic review and meta-analysis.
In Medicine
BACKGROUND :
METHODS :
RESULTS :
CONCLUSIONS :
Wang Qiaolan, Ma Jingxuan, Zhang Luoning, Xie Linshen
2022-Oct-21
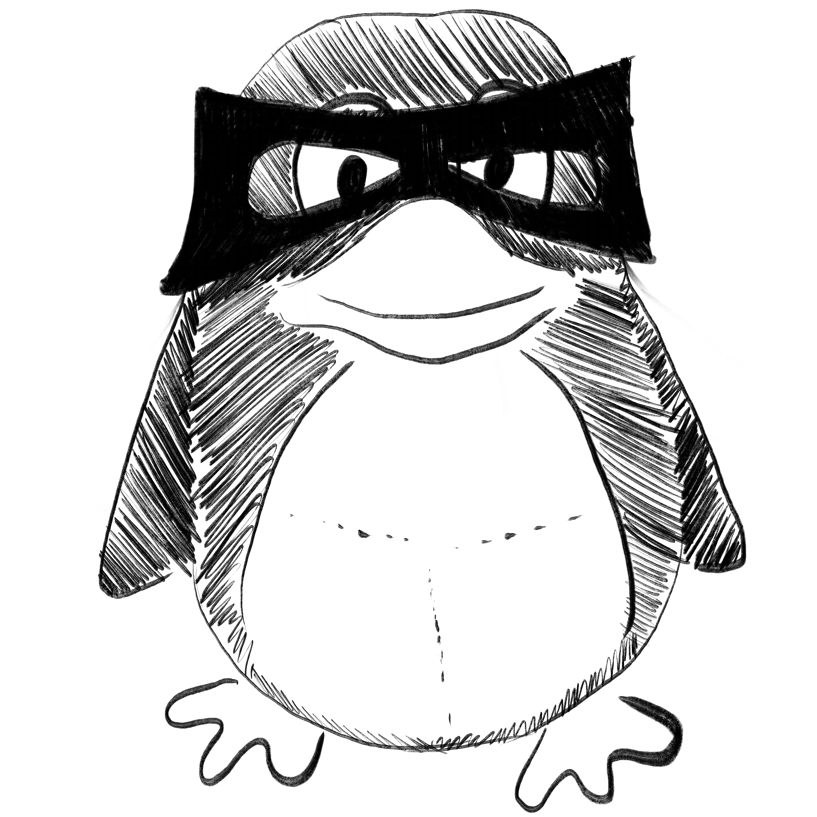
Automated diagnosis of COVID-19 using radiological modalities and Artificial Intelligence functionalities: A retrospective study based on chest HRCT database.
In Biomedical signal processing and control
Background and Objective :
Methods :
Results :
Conclusion :
Bhattacharjya Upasana, Sarma Kandarpa Kumar, Medhi Jyoti Prakash, Choudhury Binoy Kumar, Barman Geetanjali
2022-Oct-18
COVID-19, Consolidation, Crazy –paving, Deep learning, Ground glass opacities, Halo Sign, Machine Learning
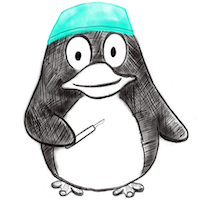
Unsupervised clustering reveals phenotypes of AKI in ICU COVID-19 patients.
In Frontiers in medicine
Background :
Methods :
Results :
Conclusion :
Legouis David, Criton Gilles, Assouline Benjamin, Le Terrier Christophe, Sgardello Sebastian, Pugin Jérôme, Marchi Elisa, Sangla Frédéric
2022
AKI, COVID-19, clustering, critical care, machine learning
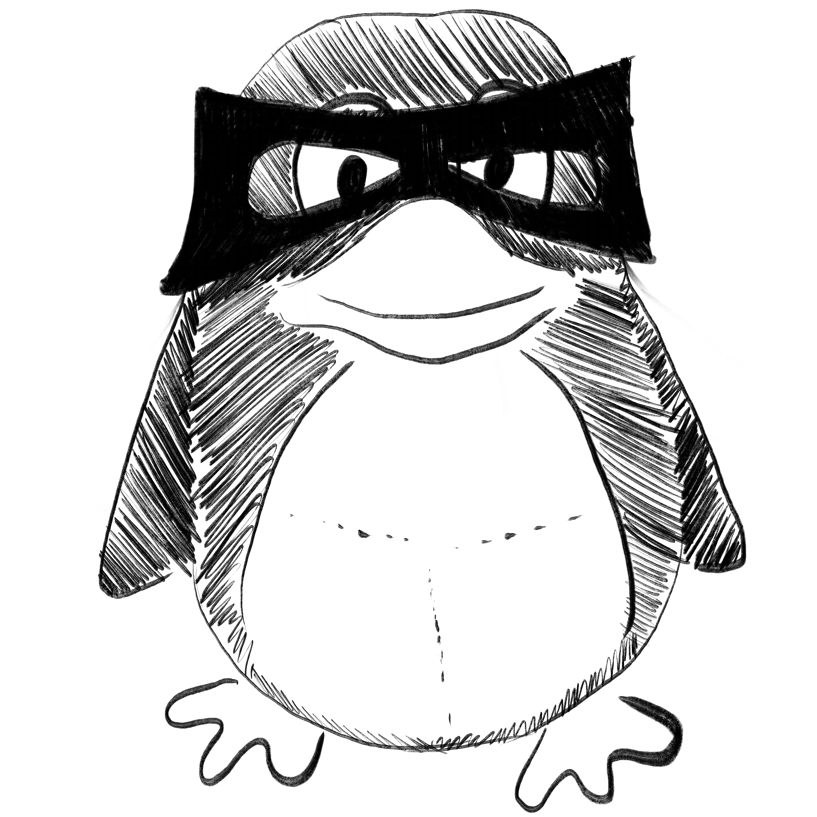
Real-Time Analysis of Predictors of COVID-19 Infection Spread in Countries in the European Union Through a New Tool.
In International journal of public health
Balogh Aniko, Harman Anna, Kreuter Frauke
2022
COVID-19 non-pharmaceutical interventions, COVID-19 prediction, COVID-19 virus variants, comparative analyses, interactive visualization, machine learning, social epidemiology, time series cross-validation
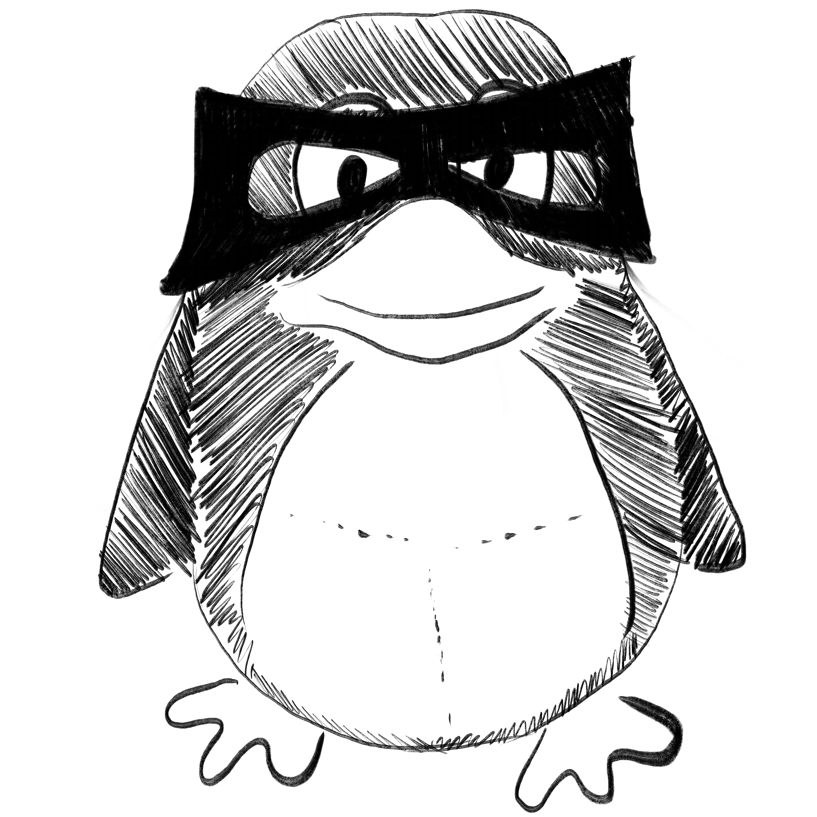
Search queries related to COVID-19 based on keyword extraction.
In Procedia computer science
Background :
Methods :
Results :
Conclusions :
Kelebercová Lívia, Munk Michal
2022
Fake News Detection, Google Trends, Keyword Extraction, Natural Language Processing
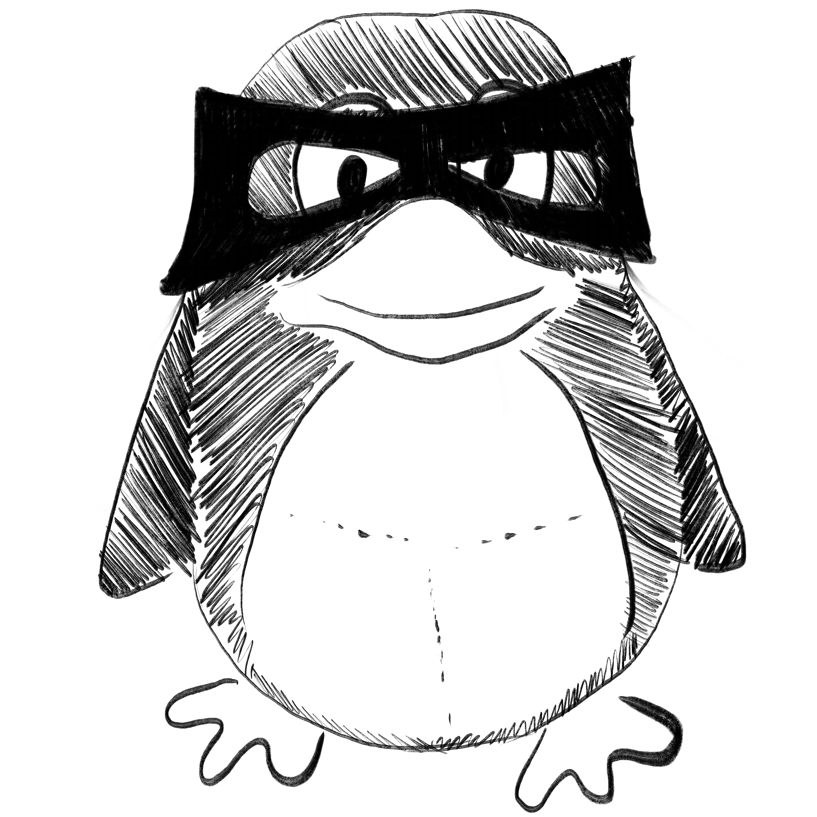
The Deep Learning-Based Framework for Automated Predicting COVID-19 Severity Score.
In Procedia computer science
Zheng Yongchang, Dong Hongwei
2022
COVID-19, CXRs, deep learning method, estimate severity, feature extraction
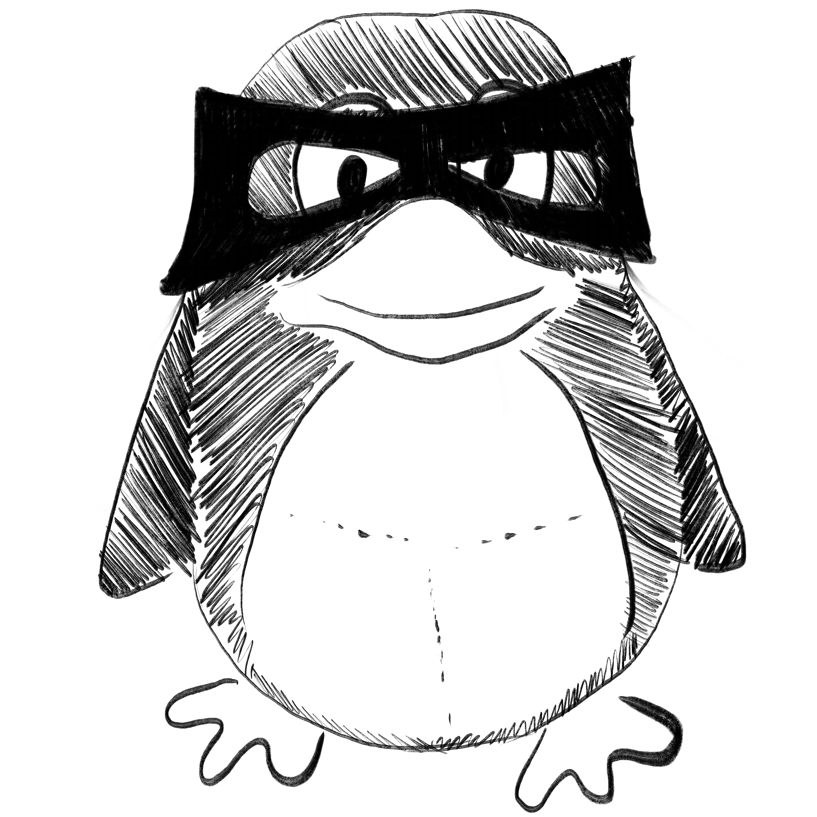
Machine Learning Models for Predicting Short-Long Length of Stay of COVID-19 Patients.
In Procedia computer science
Olivato Matteo, Rossetti Nicholas, Gerevini Alfonso E, Chiari Mattia, Putelli Luca, Serina Ivan
2022
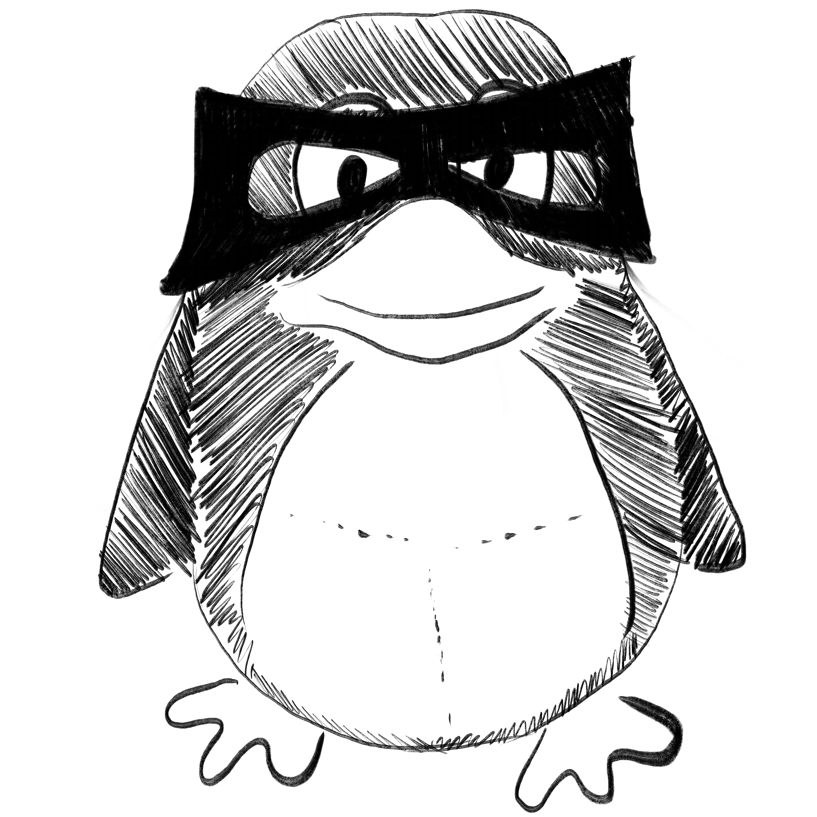
The Influence of Environmental Factors on the Spread of COVID-19 in Italy.
In Procedia computer science
Loreggia Andrea, Passarelli Anna, Pini Maria Silvia
2022
Air Quality Effects, COVID-19 Pandemic, Correlation Analysis, Machine Learning
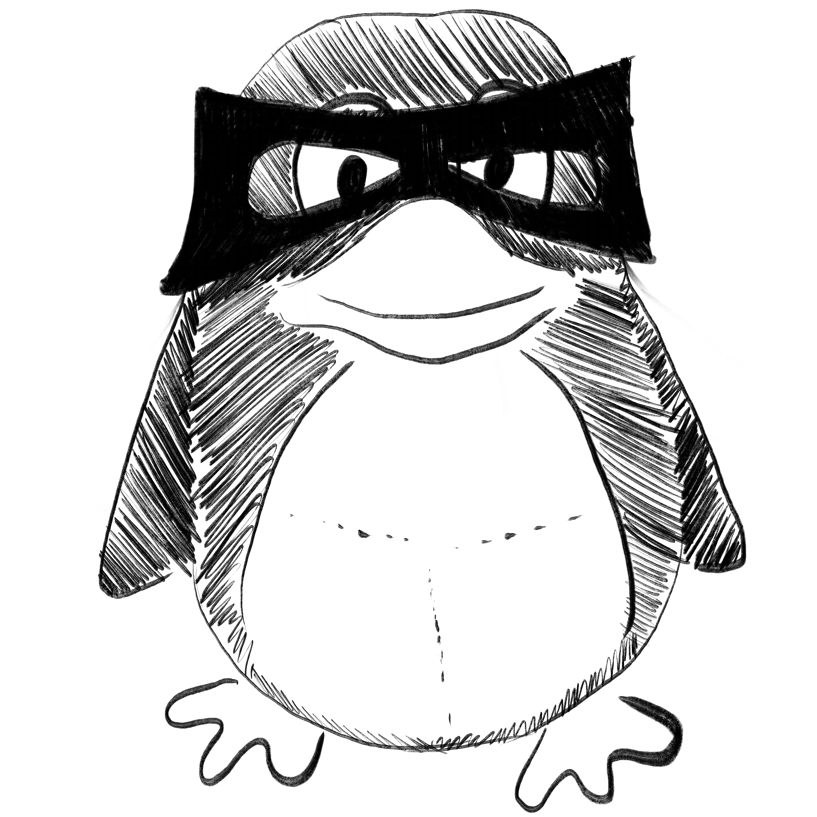
An explainable COVID-19 detection system based on human sounds.
In Smart health (Amsterdam, Netherlands)
Li Huining, Chen Xingyu, Qian Xiaoye, Chen Huan, Li Zhengxiong, Bhattacharjee Soumyadeep, Zhang Hanbin, Huang Ming-Chun, Xu Wenyao
2022-Oct-19
Accurate, Acoustic, COVID-19, Explainable
CovidViT: a novel neural network with self-attention mechanism to detect Covid-19 through X-ray images.
In International journal of machine learning and cybernetics
Yang Hang, Wang Liyang, Xu Yitian, Liu Xuhua
2022-Oct-19
Covid-19, Deep learning, Self-attention, Transformers
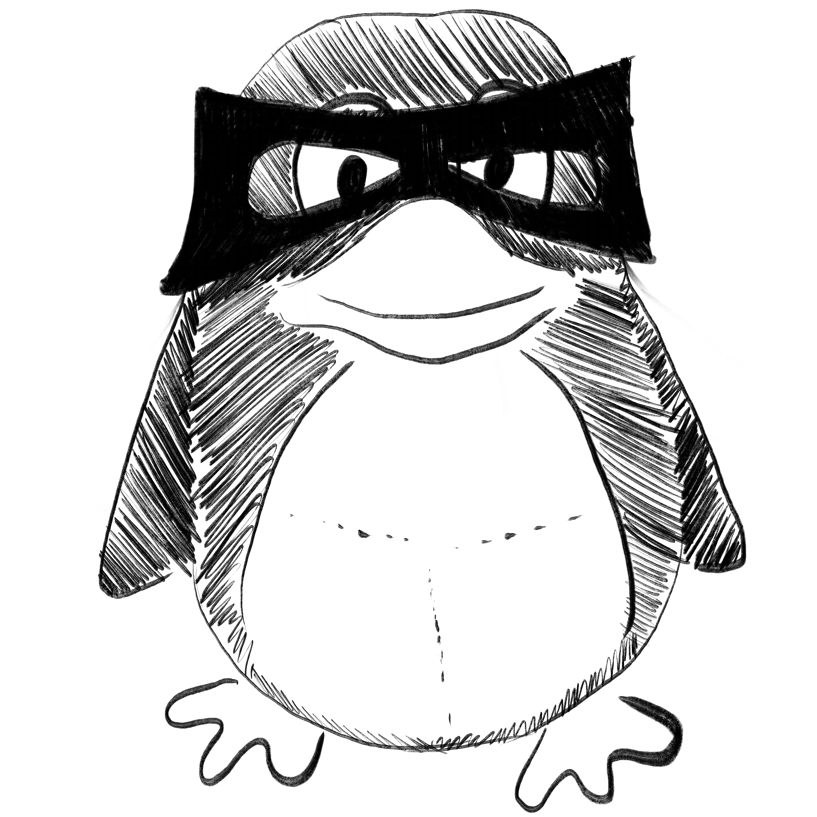
A Hybrid Deep Transfer Learning Model With Kernel Metric for COVID-19 Pneumonia Classification Using Chest CT Images.
In IEEE/ACM transactions on computational biology and bioinformatics
Li Jianyuan, Luo Xiong, Ma Huimin, Zhao Wenbing
2022-Oct-24
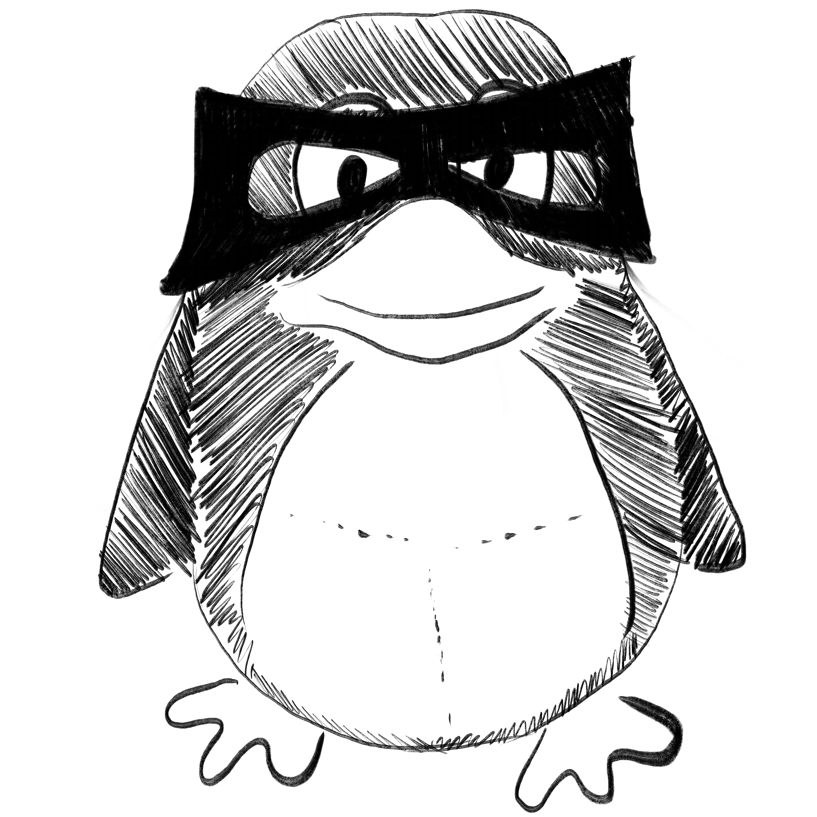
Understanding How the Design and Implementation of Online Consultations Affect Primary Care Quality: Systematic Review of Evidence With Recommendations for Designers, Providers, and Researchers.
In Journal of medical Internet research ; h5-index 88.0
BACKGROUND :
OBJECTIVE :
METHODS :
RESULTS :
CONCLUSIONS :
TRIAL REGISTRATION :
Darley Sarah, Coulson Tessa, Peek Niels, Moschogianis Susan, van der Veer Sabine N, Wong David C, Brown Benjamin C
2022-Oct-24
COVID-19, OC, care provider, general practice, health care professional, health outcome, pandemic, patient care, primary care, primary health care, remote consultation, systematic review, telemedicine, triage, workforce
Associations between the use of aspirin or other antiplatelet drugs and all-cause mortality among patients with COVID-19: A meta-analysis.
In Frontiers in pharmacology
Su Wanting, Miao He, Guo Zhaotian, Chen Qianhui, Huang Tao, Ding Renyu
2022
COVID-19, antiplatelet drug, aspirin, meta-analysis, mortality
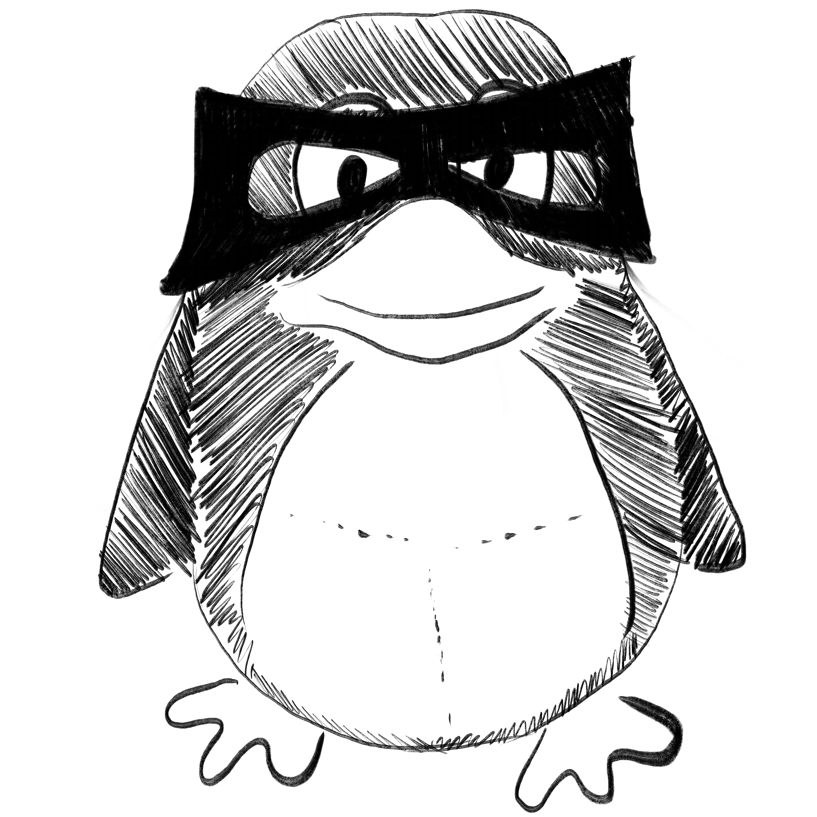
Effect of Disulfide Bridge on the Binding of SARS-CoV-2 Fusion Peptide to Cell Membrane: A Coarse-Grained Study.
In ACS omega
Shen Hujun, Wu Zhenhua
2022-Oct-18
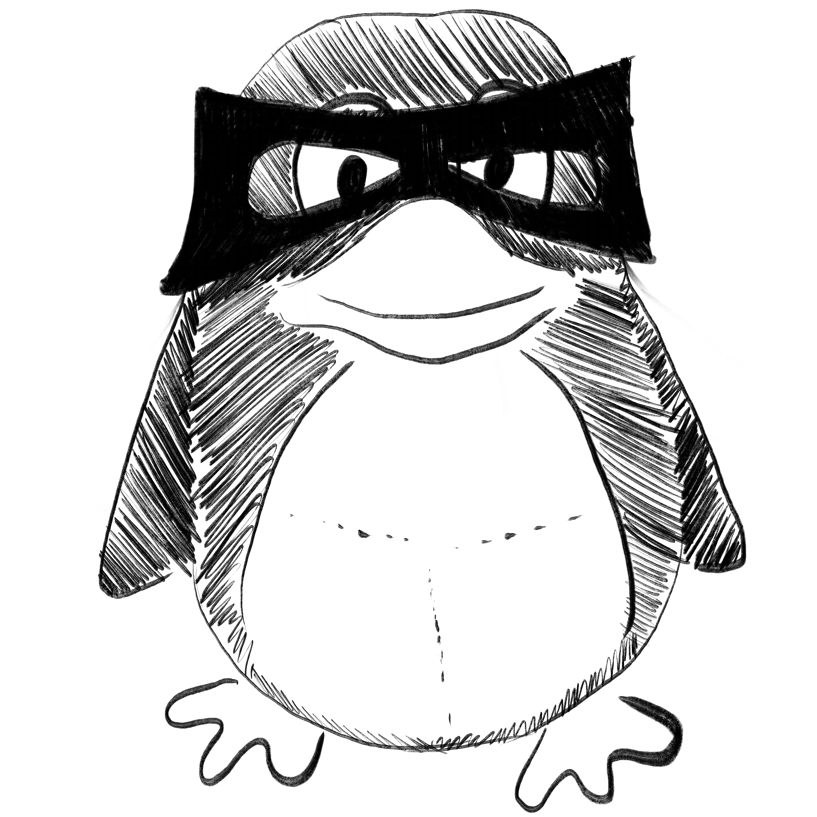
Ambient air pollutants concentration prediction during the COVID-19: A method based on transfer learning.
In Knowledge-based systems
Chen Shuixia, Xu Zeshui, Wang Xinxin, Zhang Chenxi
2022-Oct-17
Ambient air pollutants concentration prediction, COVID-19, Machine learning, Transfer learning
McS-Net: Multi-class Siamese network for severity of COVID-19 infection classification from lung CT scan slices.
In Applied soft computing
Ahuja Sakshi, Panigrahi Bijaya Ketan, Dey Neelanjan, Taneja Arpit, Gandhi Tapan Kumar
2022-Oct-17
CNN, COVID-19 infection, CT scan, Siamese network
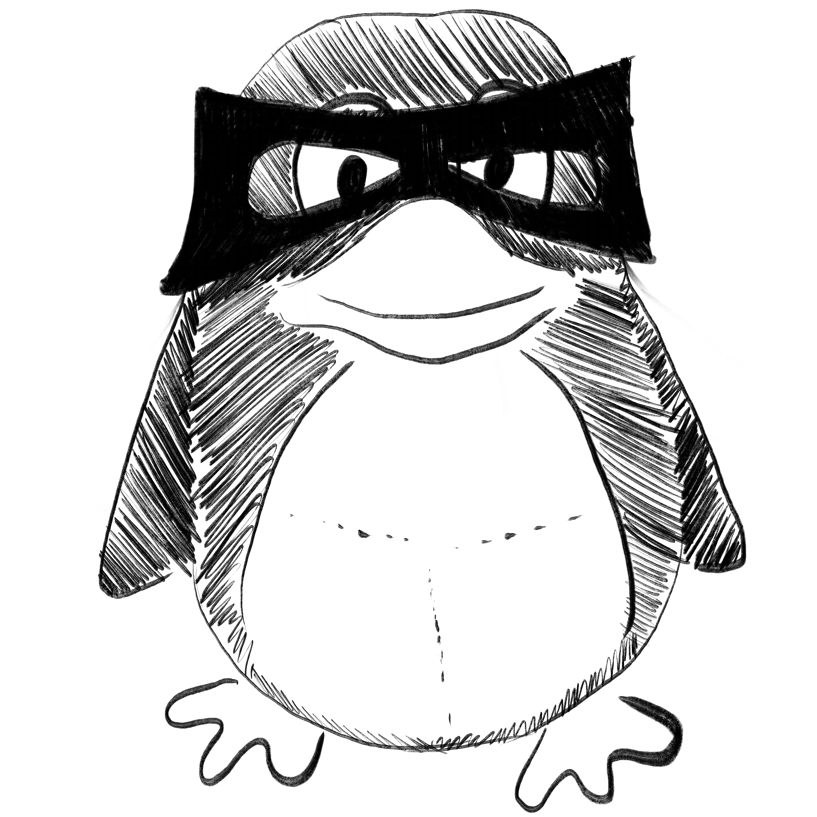
Predicting infectious disease for biopreparedness and response: A systematic review of machine learning and deep learning approaches.
In One health (Amsterdam, Netherlands)
Keshavamurthy Ravikiran, Dixon Samuel, Pazdernik Karl T, Charles Lauren E
2022-Dec
Deep learning, Disease forecast, Disease prediction, Infectious diseases, Machine learning, Systematic review
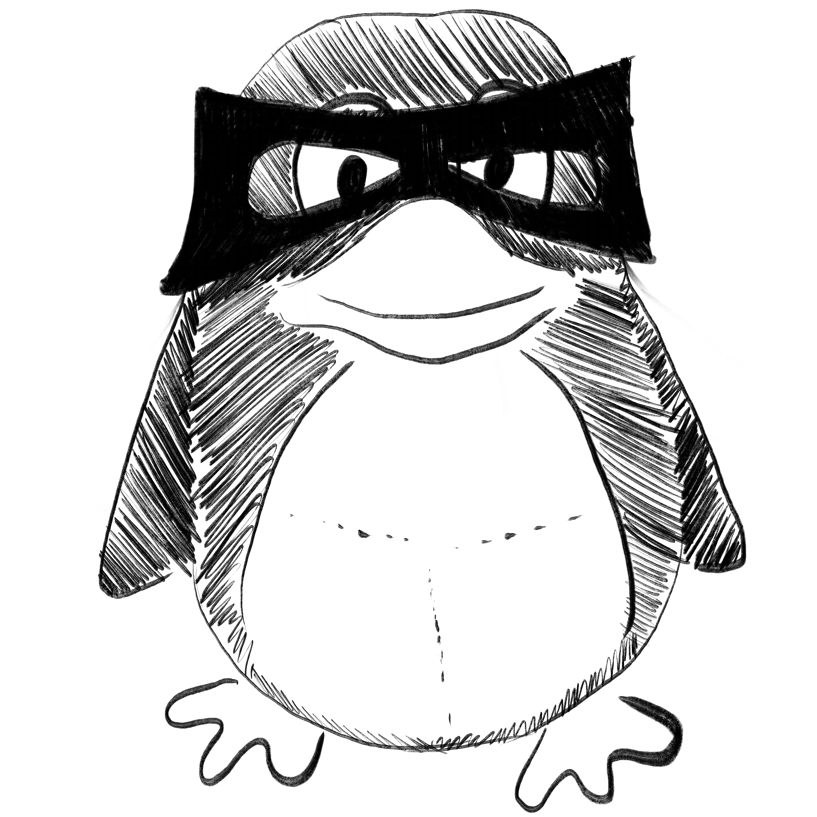
Computationally restoring the potency of a clinical antibody against SARS-CoV-2 Omicron subvariants
bioRxiv Preprint
Desautels, T. A.; Arrildt, K. T.; Zemla, A. T.; Lau, E. Y.; Zhu, F.; Ricci, D.; Cronin, S.; Zost, S.; Binshtein, E.; Scheaffer, S. M.; Engdahl, T. B.; Chen, E.; Goforth, J. W.; Vashchenko, D.; Nguyen, S.; Weilhammer, D. R.; Lo, J. K.-Y.; Rubinfeld, B.; Saada, E. A.; Weisenberger, T.; Lee, T.-H.; Whitener, B.; Case, J. B.; Ladd, A.; Silva, M. S.; Haluska, R. M.; Grzesiak, E. A.; Bates, T. W.; Petersen, B. K.; Thackray, L. B.; Segelke, B. W.; Lillo, A. M.; Sundaram, S.; Diamond, M. S.; Crowe, J. E.; Carnahan, R. H.; Faissol, D. M.
2022-10-24
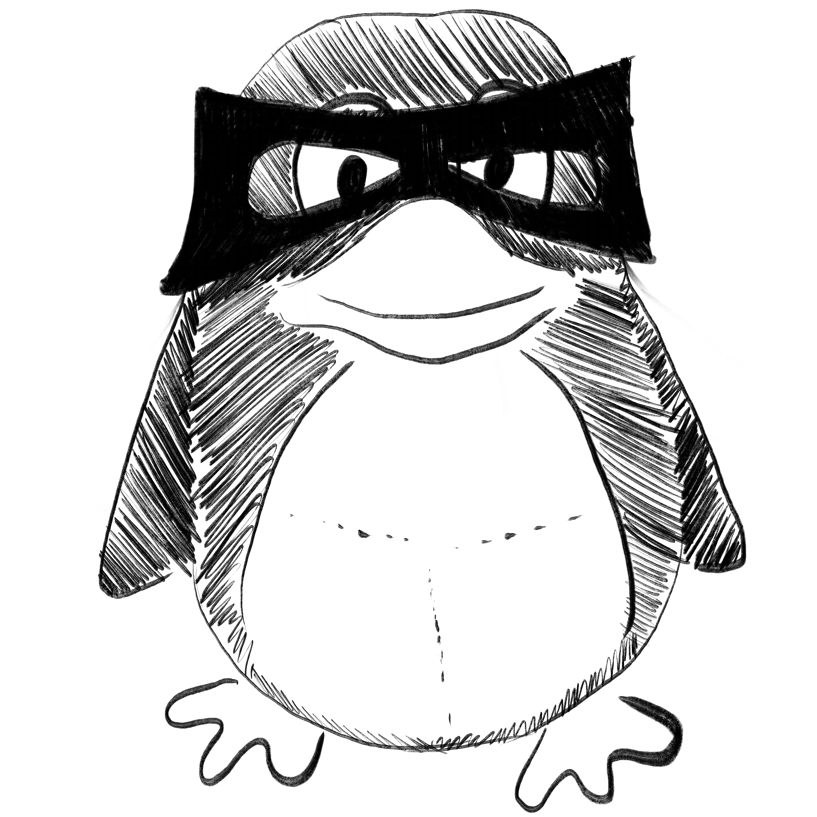
Effects of long-term COVID-19 confinement and music stimulation on mental state and brain activity of young people.
In Neuroscience letters
Luo Lina, Shan Mianjia, Zu Yangmin, Chen Yufang, Bu Lingguo, Wang Lejun, Ni Ming, Niu Wenxin
2022-Oct-19
Cognitive neuroscience, Functional brain connectivity, Lockdown, Music stimulation, Negative emotions, Prefrontal cortex activation
A storm in a teacup -- A biomimetic lung microphysiological system in conjunction with a deep-learning algorithm to monitor lung pathological and inflammatory reactions.
In Biosensors & bioelectronics
Chen Zaozao, Huang Jie, Zhang Jing, Xu Zikang, Li Qiwei, Ouyang Jun, Yan Yuchuan, Sun Shiqi, Ye Huan, Wang Fei, Zhu Jianfeng, Wang Zhangyan, Chao Jie, Pu Yuepu, Gu Zhongze
2022-Oct-01
COVID-19, Cytokine storm, Lung-on-a-chip, Microfluidics
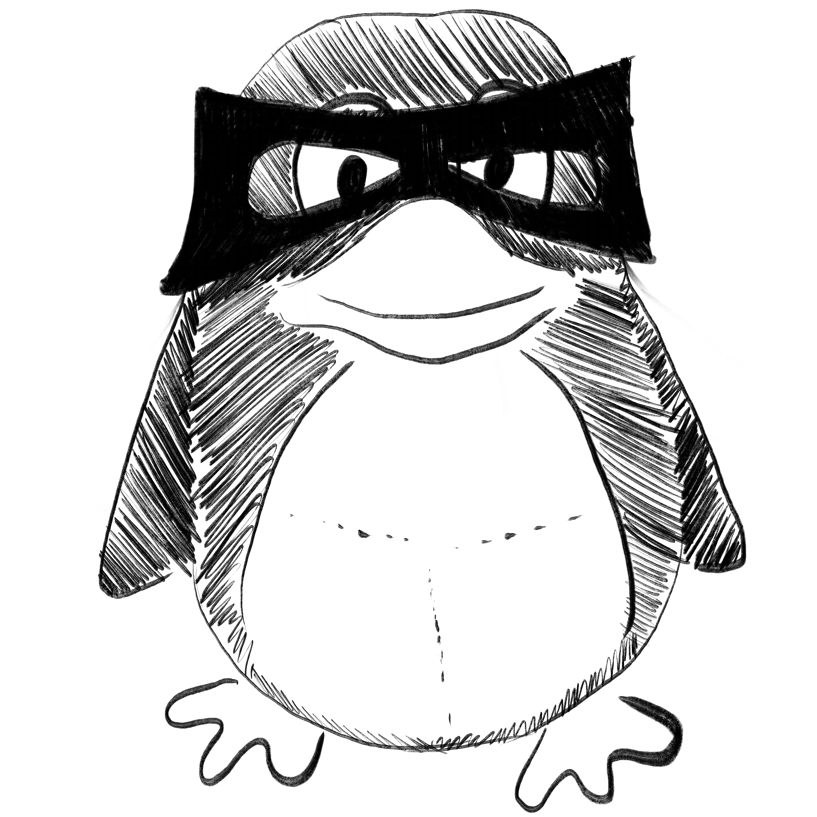
Systematic Review of Advanced AI Methods for Improving Healthcare Data Quality In Post COVID-19 Era.
In IEEE reviews in biomedical engineering
Isgut Monica, Gloster Logan, Choi Katherine, Venugopalan Janani, Wang May D
2022-Oct-21
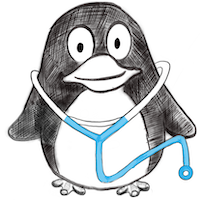
Development and validation of multivariable prediction models of serological response to SARS-CoV-2 vaccination in kidney transplant recipients.
In Frontiers in immunology ; h5-index 100.0
Osmanodja Bilgin, Stegbauer Johannes, Kantauskaite Marta, Rump Lars Christian, Heinzel Andreas, Reindl-Schwaighofer Roman, Oberbauer Rainer, Benotmane Ilies, Caillard Sophie, Masset Christophe, Kerleau Clarisse, Blancho Gilles, Budde Klemens, Grunow Fritz, Mikhailov Michael, Schrezenmeier Eva, Ronicke Simon
2022
COVID-19, clinical decision support, immunosuppression therapy, kidney transplantation, vaccination
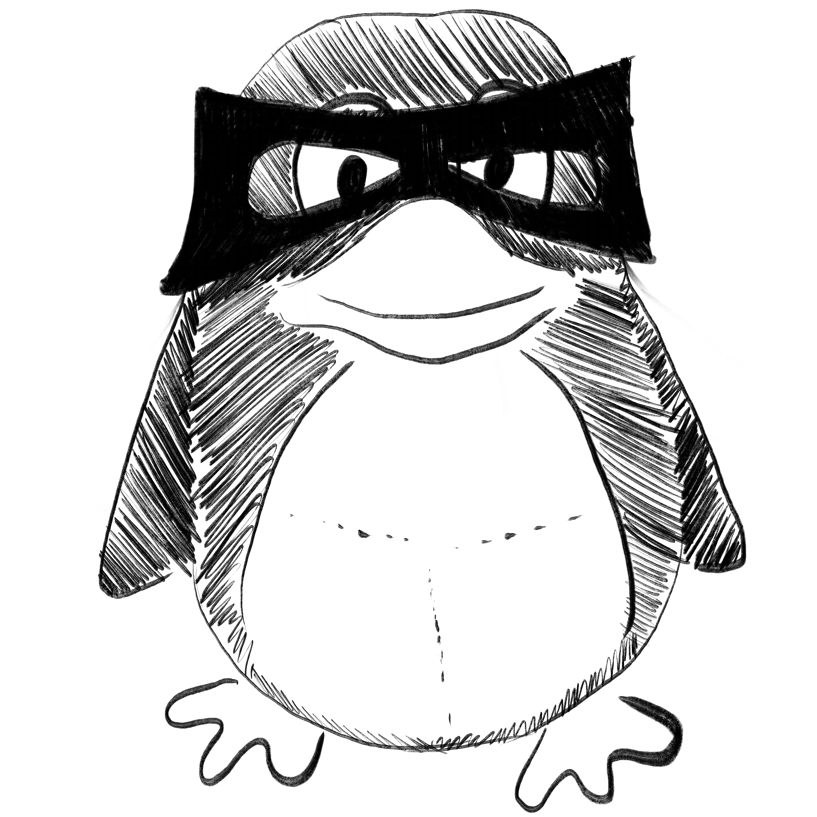
Artificial intelligence assisted acute patient journey.
In Frontiers in artificial intelligence
Nazir Talha, Mushhood Ur Rehman Muhammad, Asghar Muhammad Roshan, Kalia Junaid S
2022
AI-based clinical decision support system, Automated EMR summary, acute patient journey, artificial intelligence, electronic-triage, health IoT
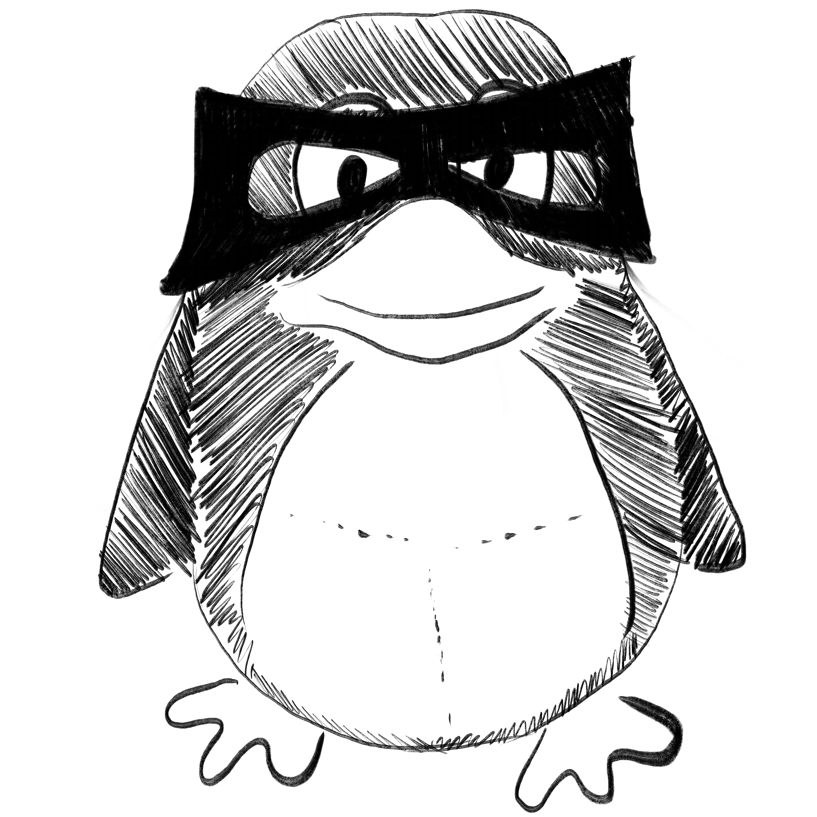
Deep learning models-based CT-scan image classification for automated screening of COVID-19.
In Biomedical signal processing and control
Gupta Kapil, Bajaj Varun
2023-Feb
COVID-19, CT-scan images, Deep learning, Transfer learning
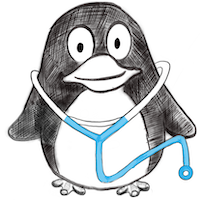
Machine learning in the diagnosis of asthma phenotypes during coronavirus disease 2019 pandemic.
In Clinical and translational allergy
Background :
Methods :
Results :
Conclusions :
Gawlewicz-Mroczka Agnieszka, Pytlewski Adam, Celejewska-Wójcik Natalia, Ćmiel Adam, Gielicz Anna, Sanak Marek, Mastalerz Lucyna
2022-Oct
COVID‐19 pandemic, machine learning, nonsteroidal anti‐inflammatory drug (NSAID)–exacerbated respiratory disease (NERD), nonsteroidal anti‐inflammatory drug tolerant asthma (NTA), oral aspirin challenge
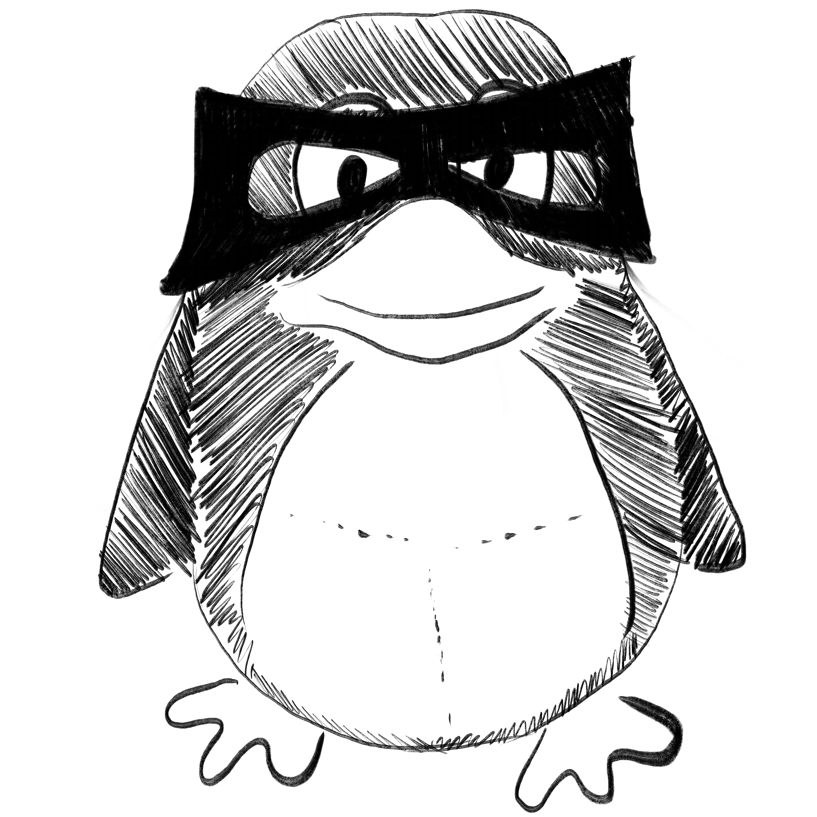
Smartphone-based Platform Assisted by Artificial Intelligence for Reading and Reporting Rapid Diagnostic Tests: Application to SARS-CoV-2 Lateral Flow Immunoassays.
In JMIR public health and surveillance
BACKGROUND :
OBJECTIVE :
METHODS :
RESULTS :
CONCLUSIONS :
Bermejo-Peláez David, Marcos-Mencía Daniel, Álamo Elisa, Pérez-Panizo Nuria, Mousa Adriana, Dacal Elena, Lin Lin, Vladimirov Alexander, Cuadrado Daniel, Mateos-Nozal Jesús, Galán Juan Carlos, Romero-Hernandez Beatriz, Cantón Rafael, Luengo-Oroz Miguel, Rodriguez-Dominguez Mario
2022-Oct-13
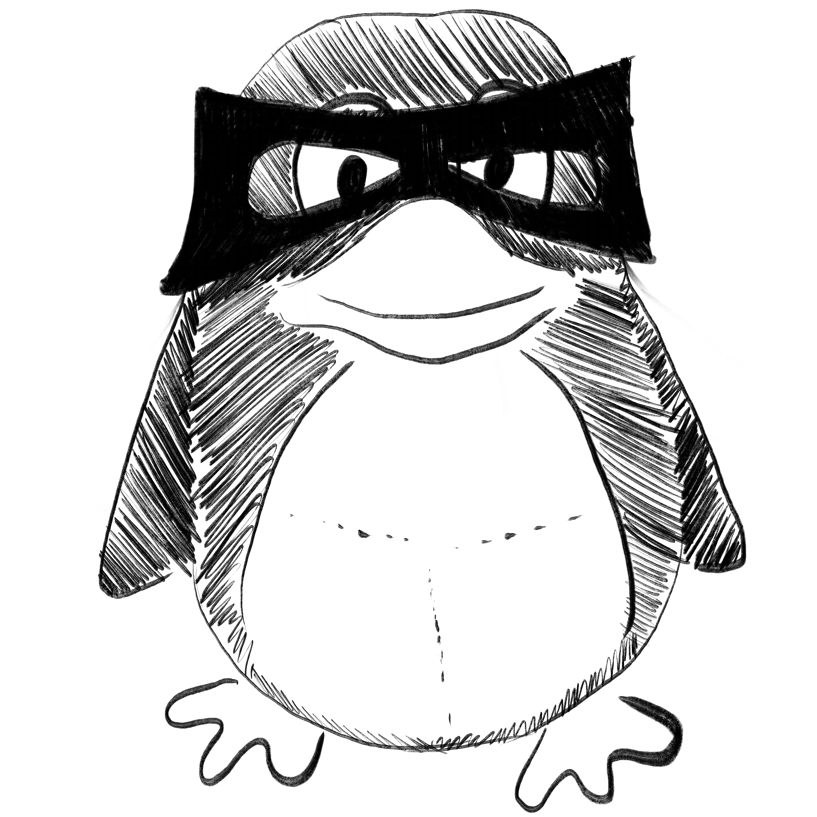
Discovery and analytical validation of a vocal biomarker to monitor anosmia and ageusia in patients with Covid-19: Cross-sectional study.
In JMIR medical informatics ; h5-index 23.0
BACKGROUND :
OBJECTIVE :
METHODS :
RESULTS :
CONCLUSIONS :
CLINICALTRIAL :
Higa Eduardo, Zhang Lu, Elbéji Abir, Fischer Aurélie, Aguayo Gloria A, Nazarov Petr V, Fagherazzi Guy
2022-Sep-07
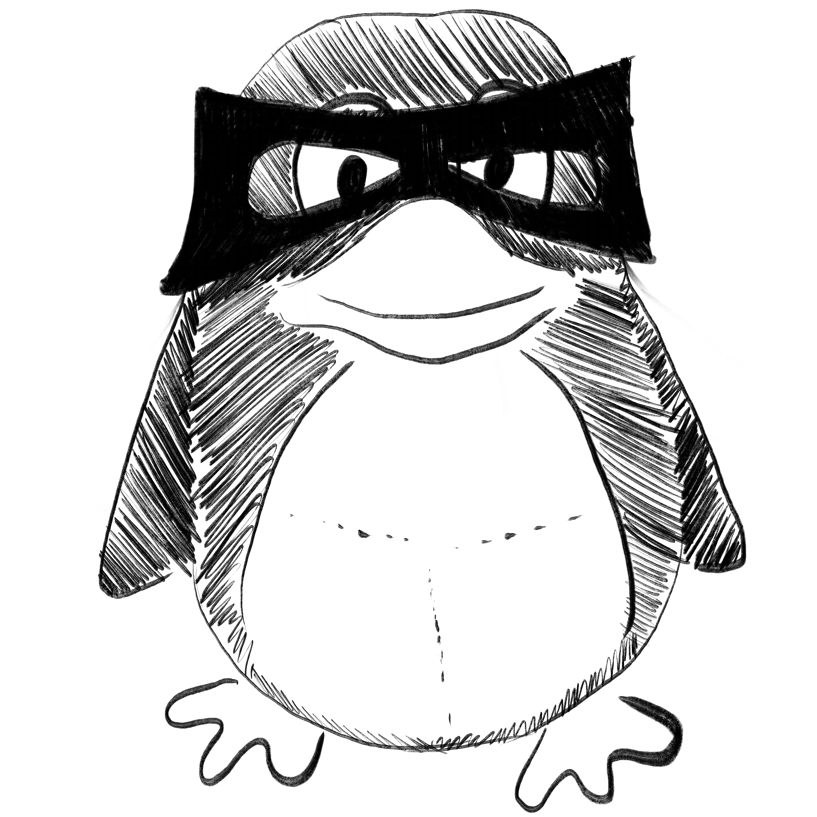
Evaluation of digital economy development level based on multi-attribute decision theory.
In PloS one ; h5-index 176.0
Su Jinqi, Su Ke, Wang Shubin
2022
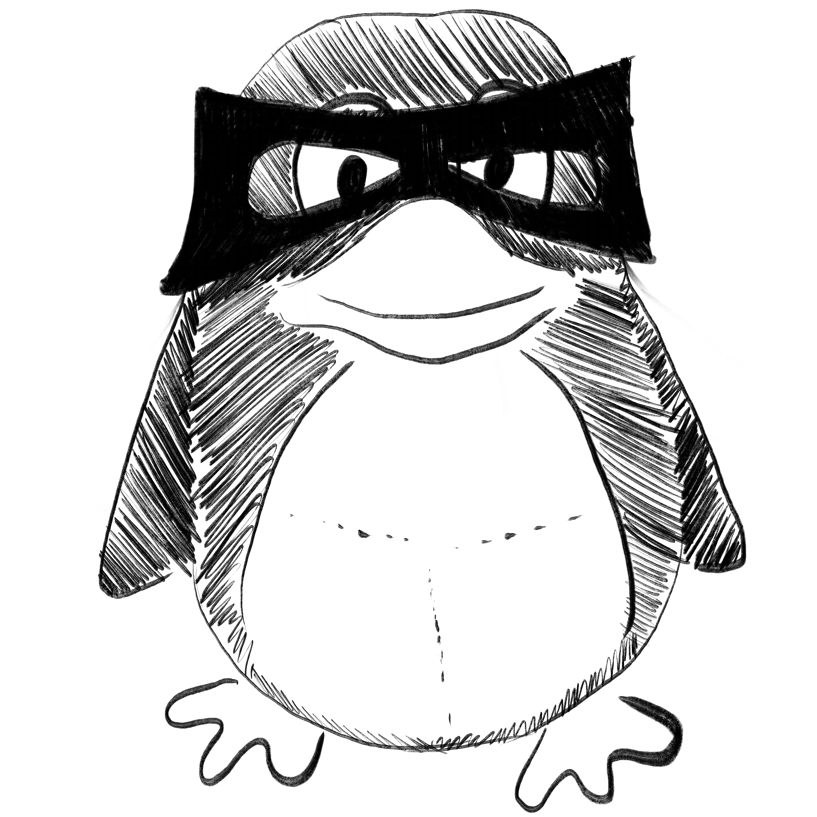
Predicting responders to prone positioning in mechanically ventilated patients with COVID-19 using machine learning.
In Annals of intensive care ; h5-index 37.0
BACKGROUND :
METHODS :
RESULTS :
CONCLUSIONS :
Dam Tariq A, Roggeveen Luca F, van Diggelen Fuda, Fleuren Lucas M, Jagesar Ameet R, Otten Martijn, de Vries Heder J, Gommers Diederik, Cremer Olaf L, Bosman Rob J, Rigter Sander, Wils Evert-Jan, Frenzel Tim, Dongelmans Dave A, de Jong Remko, Peters Marco A A, Kamps Marlijn J A, Ramnarain Dharmanand, Nowitzky Ralph, Nooteboom Fleur G C A, de Ruijter Wouter, Urlings-Strop Louise C, Smit Ellen G M, Mehagnoul-Schipper D Jannet, Dormans Tom, de Jager Cornelis P C, Hendriks Stefaan H A, Achterberg Sefanja, Oostdijk Evelien, Reidinga Auke C, Festen-Spanjer Barbara, Brunnekreef Gert B, Cornet Alexander D, van den Tempel Walter, Boelens Age D, Koetsier Peter, Lens Judith, Faber Harald J, Karakus A, Entjes Robert, de Jong Paul, Rettig Thijs C D, Arbous Sesmu, Vonk Sebastiaan J J, Machado Tomas, Herter Willem E, de Grooth Harm-Jan, Thoral Patrick J, Girbes Armand R J, Hoogendoorn Mark, Elbers Paul W G
2022-Oct-20
Acute respiratory distress syndrome, COVID-19, Mechanical ventilation
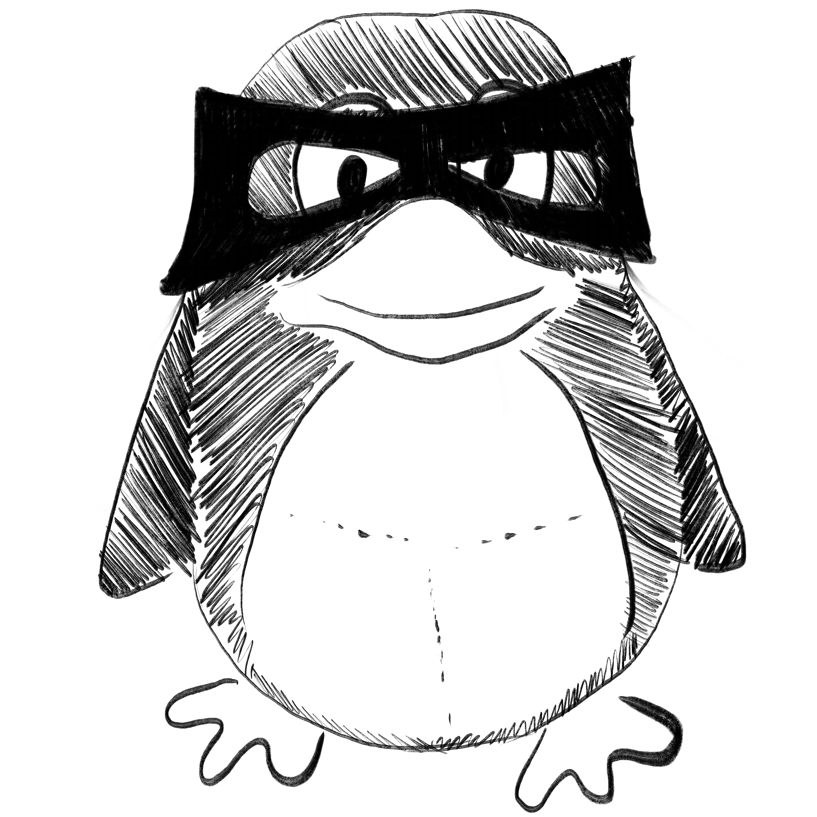
Serological responses to human virome define clinical outcomes of Italian patients infected with SARS-CoV-2.
In International journal of biological sciences
Wang Limin, Candia Julián, Ma Lichun, Zhao Yongmei, Imberti Luisa, Sottini Alessandra, Quiros-Roldan Eugenia, Dobbs Kerry, Burbelo Peter D, Cohen Jeffrey I, Delmonte Ottavia M, Forgues Marshonna, Liu Hui, Matthews Helen F, Shaw Elana, Stack Michael A, Weber Sarah E, Zhang Yu, Lisco Andrea, Sereti Irini, Su Helen C, Notarangelo Luigi D, Wang Xin Wei
2022
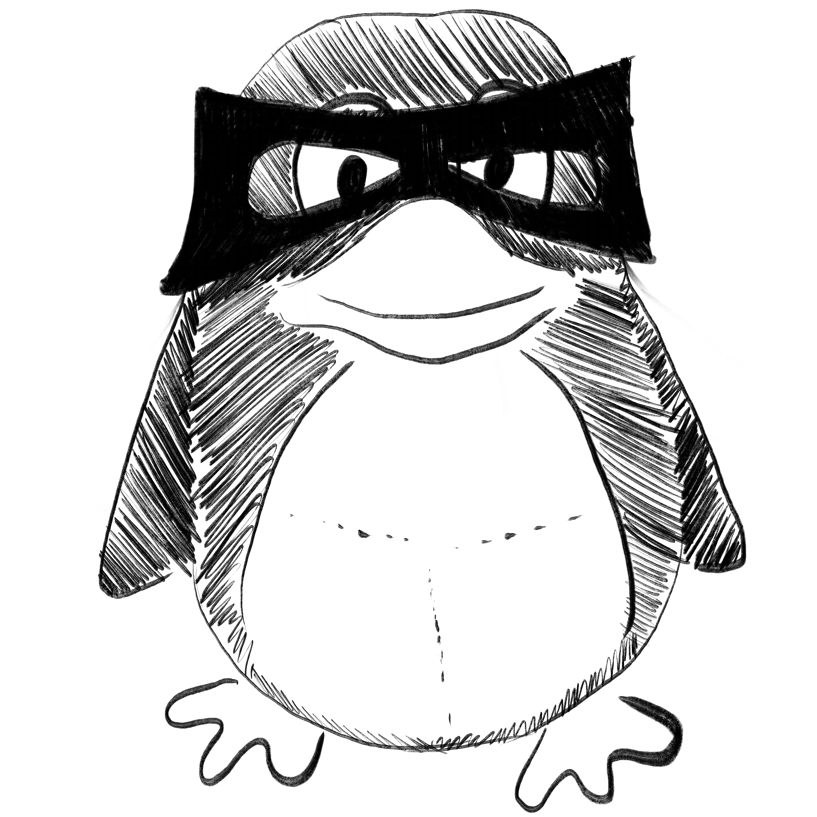
COVID19 Diagnosis Using Chest X-rays and Transfer Learning.
In medRxiv : the preprint server for health sciences
** :
CCS CONCEPTS :
Reference format :
Stubblefield Jonathan, Causey Jason, Dale Dakota, Qualls Jake, Bellis Emily, Fowler Jennifer, Walker Karl, Huang Xiuzhen
2022-Oct-12
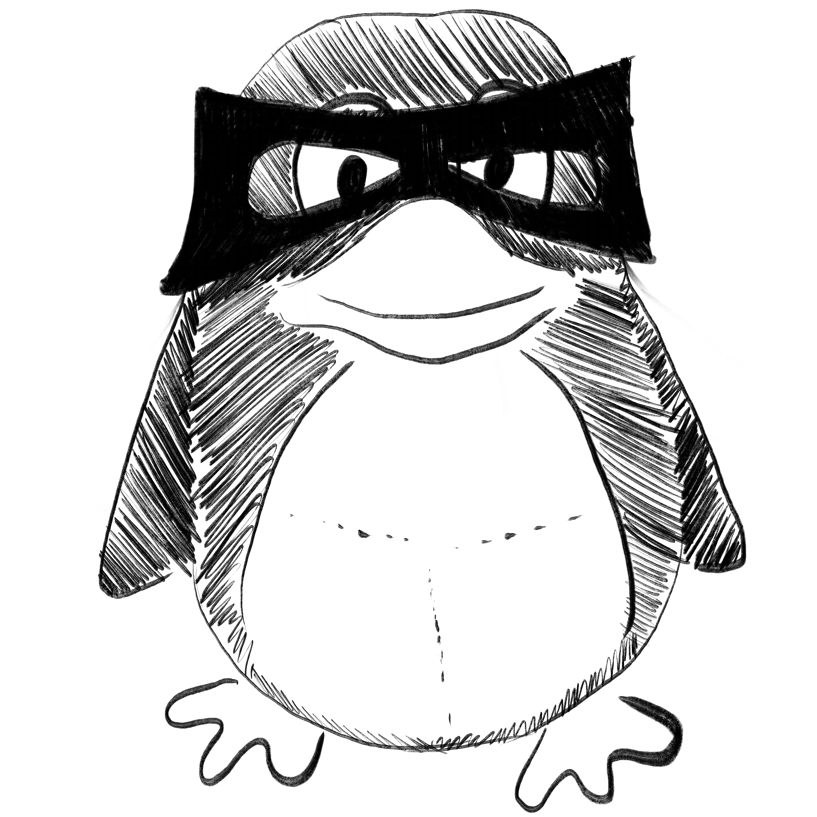
Distinct responses of newly identified monocyte subsets to advanced gastrointestinal cancer and COVID-19.
In Frontiers in immunology ; h5-index 100.0
Rigamonti Alessandra, Castagna Alessandra, Viatore Marika, Colombo Federico Simone, Terzoli Sara, Peano Clelia, Marchesi Federica, Locati Massimo
2022
COVID-19, cancer, immunotherapy, machine learning, monocyte, single-cell transcriptome
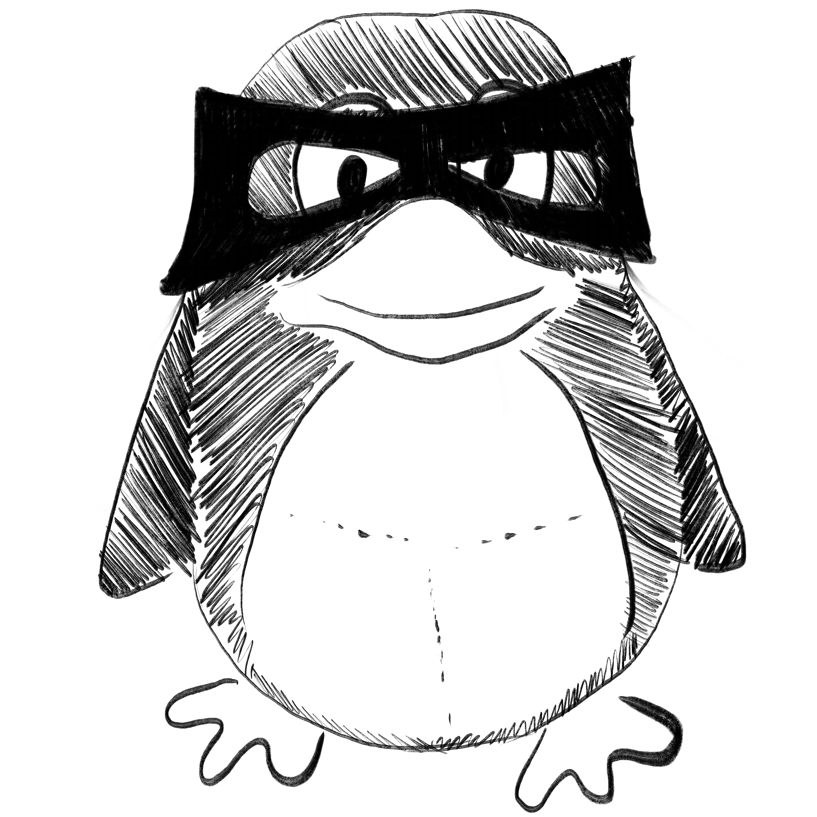
Detection of COVID-19 using deep learning on x-ray lung images.
In PeerJ. Computer science
Odeh AbdAlRahman, Alomar Ayah, Aljawarneh Shadi
2022
COVID-19, Classification, Deep learning, Supervised learning, Transfer learning
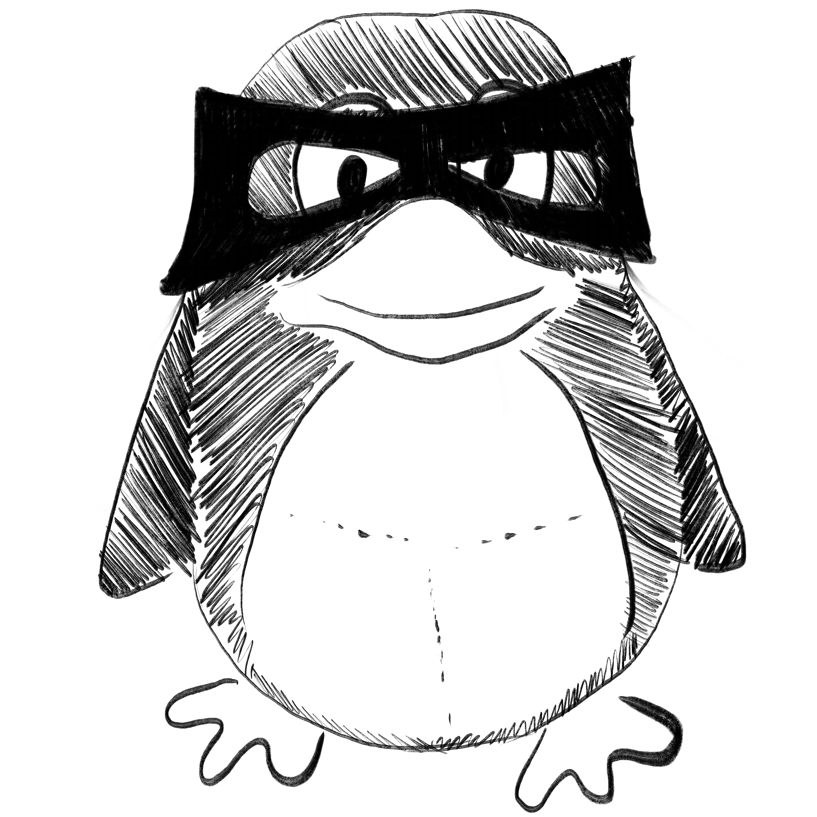
Analyzing perceptions of a global event using CNN-LSTM deep learning approach: the case of Hajj 1442 (2021).
In PeerJ. Computer science
Shambour Mohd Khaled
2022
Convolutional Neural Networks (CNN), Deep learning, Hajj rituals, Long short term memory, Sentiment analysis
Machine Learning Techniques to Explore Clinical Presentations of COVID-19 Disease Severity and to Test the Association with Unhealthy Opioid Use (UOU): Retrospective Cross-sectional Cohort Study.
In JMIR public health and surveillance
BACKGROUND :
OBJECTIVE :
METHODS :
RESULTS :
CONCLUSIONS :
CLINICALTRIAL :
Thompson Hale M, Sharma Brihat, Smith Dale, Bhalla Sameer, Erondu Ihuoma, Hazra Aniruddha, Ilyas Yousaf, Pachwicewicz Paul, Sheth Neeral K, Chhabra Neeraj, Karnik Niranjan S, Afshar Majid
2022-Oct-18
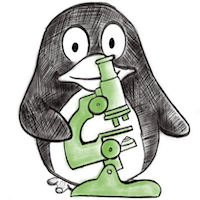
A prediction model for COVID-19 liver dysfunction in patients with normal hepatic biochemical parameters.
In Life science alliance
Bao Jianfeng, Liu Shourong, Liang Xiao, Wang Congcong, Cao Lili, Li Zhaoyi, Wei Furong, Fu Ai, Shi Yingqiu, Shen Bo, Zhu Xiaoli, Zhao Yuge, Liu Hong, Miao Liangbin, Wang Yi, Liang Shuang, Wu Linyan, Huang Jinsong, Guo Tiannan, Liu Fang
2023-Jan
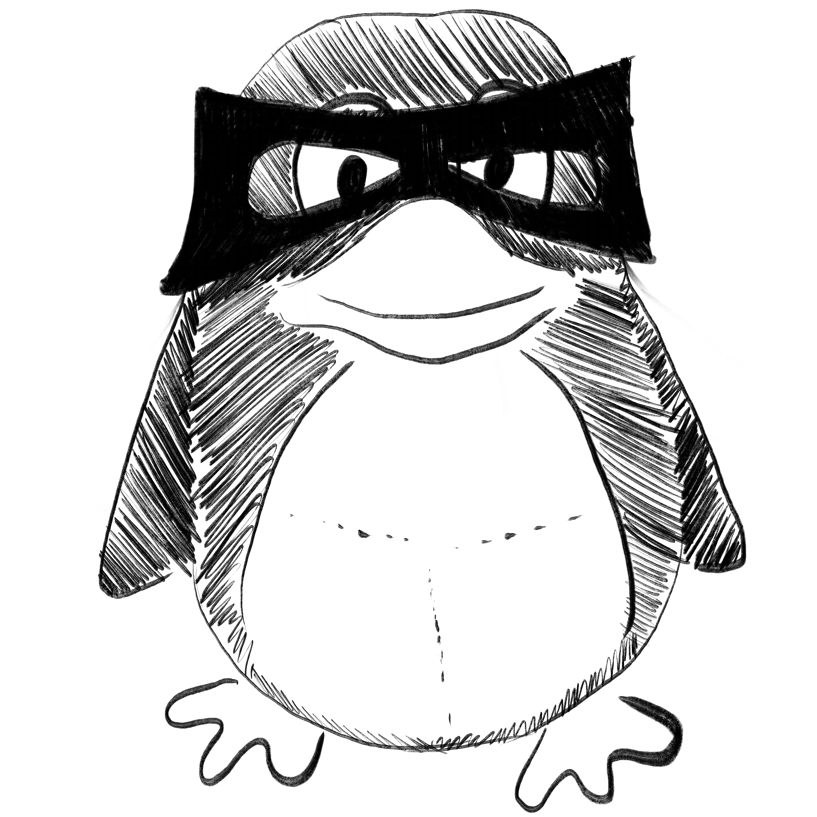
Role of Technology in Detection of COVID-19.
In Cureus
Lohiya Drishti V, Pathak Swanand S
2022-Sep
artificial intelligence, cas system, covid, ct-scan, elisa, molecular investigations, rt-pcr, sars-cov-2, serological test, thermometer thermal scanners
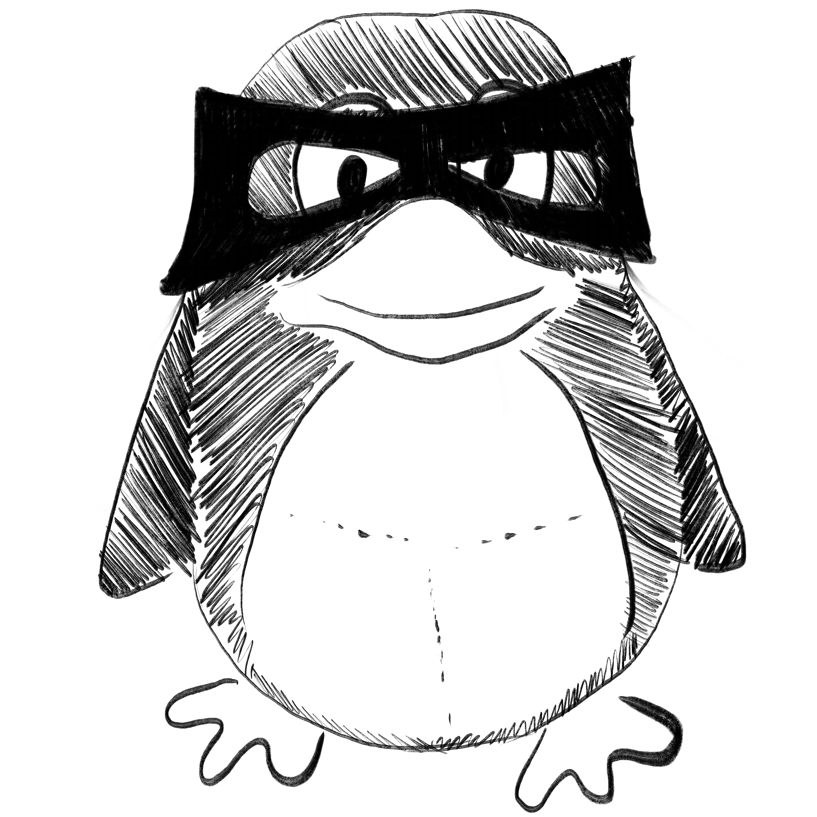
Proximity Detection During Epidemics: Direct UWB TOA Versus Machine Learning Based RSSI.
In International journal of wireless information networks
** :
Graphical Abstract :
Su Zhuoran, Pahlavan Kaveh, Agu Emmanuel, Wei Haowen
2022-Oct-14
BLE, COVID-19, Classical estimation theory, Proximity detection, RSSI features, UWB
Application of machine learning approaches to predict the impact of ambient air pollution on outpatient visits for acute respiratory infections.
In The Science of the total environment
Ravindra Khaiwal, Bahadur Samsher Singh, Katoch Varun, Bhardwaj Sanjeev, Kaur-Sidhu Maninder, Gupta Madhu, Mor Suman
2022-Oct-15
ARI, Air pollution, Machine learning programs, Random forest regression, Risk prediction
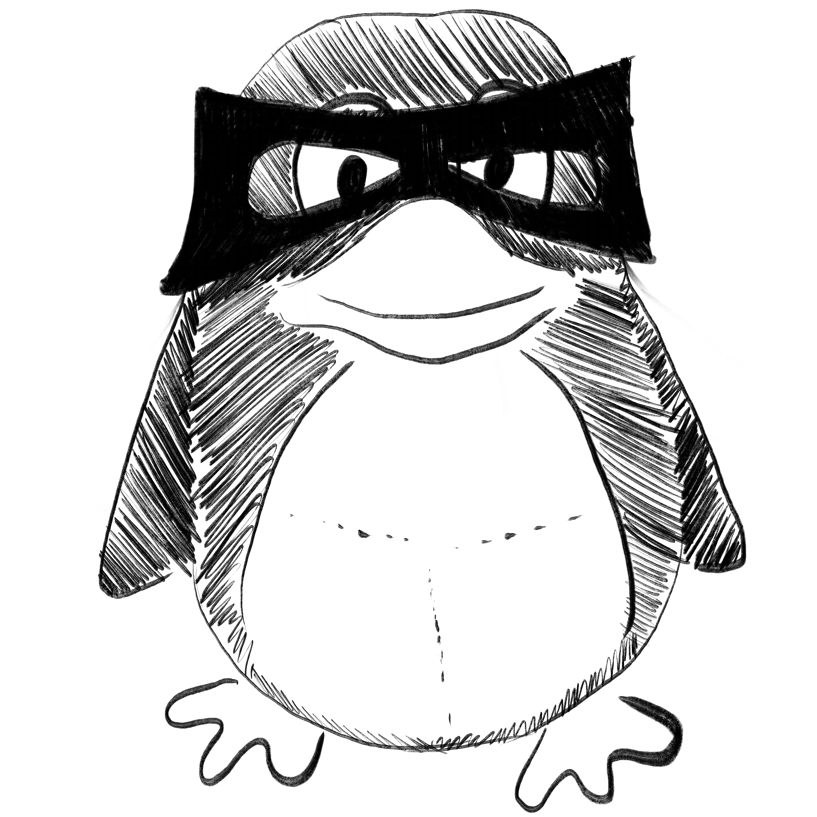
Active deep learning from a noisy teacher for semi-supervised 3D image segmentation: Application to COVID-19 pneumonia infection in CT.
In Computerized medical imaging and graphics : the official journal of the Computerized Medical Imaging Society
Hussain Mohammad Arafat, Mirikharaji Zahra, Momeny Mohammad, Marhamati Mahmoud, Neshat Ali Asghar, Garbi Rafeef, Hamarneh Ghassan
2022-Oct-07
Active learning, COVID-19, Deep learning, Noisy teacher, Pneumonia, Segmentation, Semi-supervised learning
Exploring the influence of COVID-19 on the spread of hand, foot, and mouth disease with an automatic machine learning prediction model.
In Environmental science and pollution research international
Yang Chuan, An Shuyi, Qiao Baojun, Guan Peng, Huang Desheng, Wu Wei
2022-Oct-18
Automatic machine learning, COVID-19, Countermeasures, HFMD, Prediction, Time series
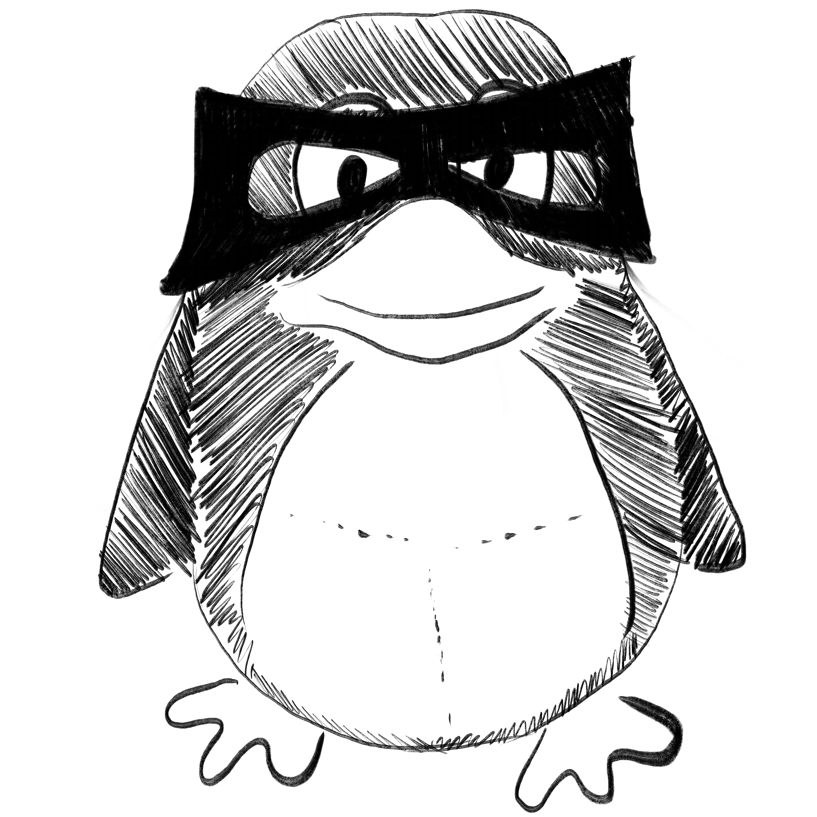
Clinical and Temporal Characterization of COVID-19 Subgroups Using Patient Vector Embeddings of Electronic Health Records.
In Journal of the American Medical Informatics Association : JAMIA
OBJECTIVE :
MATERIALS AND METHODS :
RESULTS :
DISCUSSION :
Ta Casey N, Zucker Jason E, Chiu Po-Hsiang, Fang Yilu, Natarajan Karthik, Weng Chunhua
2022-Oct-18
COVID-19, Cluster analysis, SARS-CoV-2, Unsupervised machine learning
Public Willingness to Engage With COVID-19 Contact Tracing, Quarantine, and Exposure Notification.
In Public health reports (Washington, D.C. : 1974)
OBJECTIVES :
METHODS :
RESULTS :
CONCLUSIONS :
Liccardi Ilaria, Alekseyev Jesslyn, Woltz Vilhelm L Andersen, McLean Jody E, Zurko Mary Ellen
2022-Oct-18
COVID-19, attitudes, contact tracing, exposure notification, health knowledge, practice, quarantine
Struggling With Recovery From Opioids: Who Is at Risk During COVID-19?
In Journal of addiction medicine ; h5-index 27.0
OBJECTIVES :
METHODS :
RESULTS :
CONCLUSIONS :
Keith Diana R, Tegge Allison N, Stein Jeffrey S, Athamneh Liqa N, Craft William H, Chilcoat Howard D, Le Moigne Anne, DeVeaugh-Geiss Angela, Bickel Warren K
2022-Oct-18
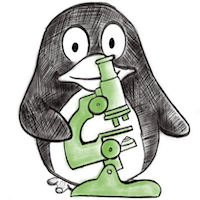
Robustness of Demonstration-based Learning Under Limited Data Scenario
ArXiv Preprint
Hongxin Zhang, Yanzhe Zhang, Ruiyi Zhang, Diyi Yang
2022-10-19
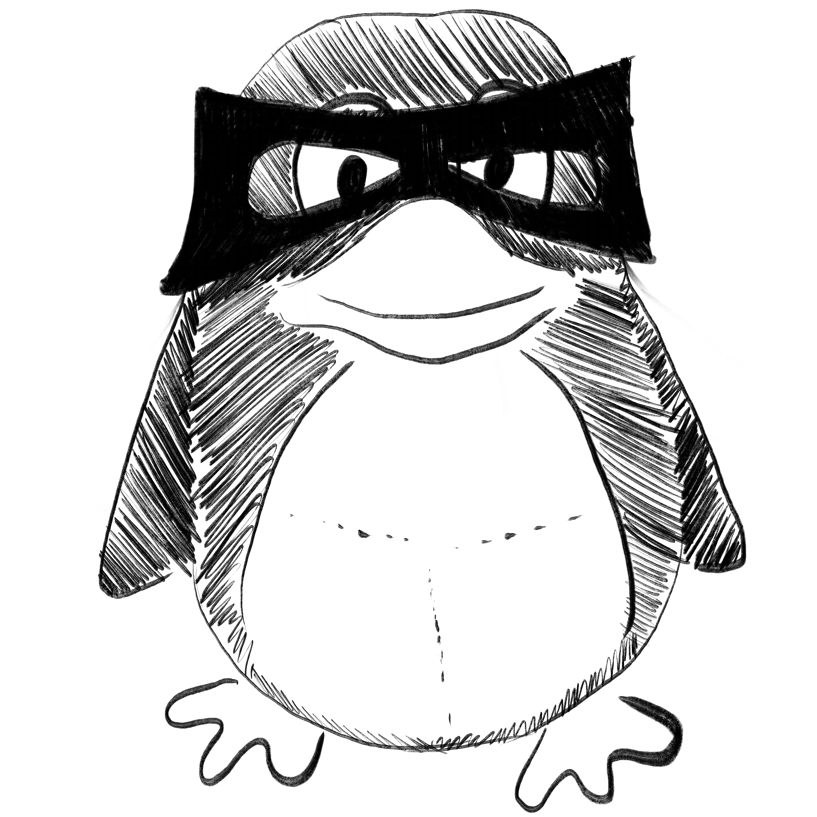
Retraction Note: An adaptive speech signal processing for COVID-19 detection using deep learning approach.
In International journal of speech technology
Al-Dhlan Kawther A
2022-Oct-13
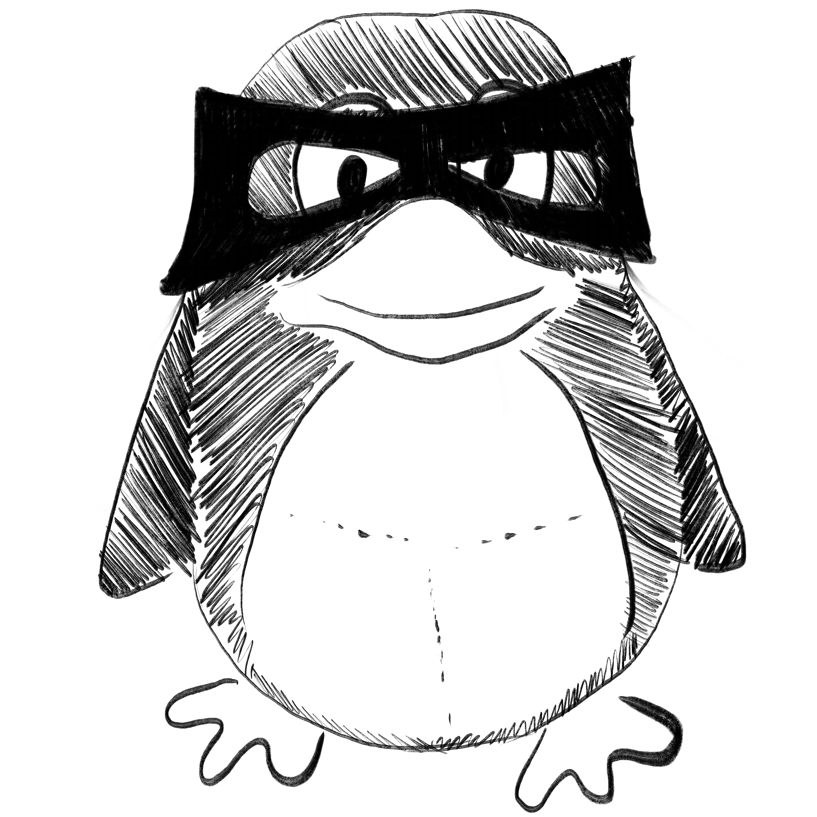
Comparative analysis of deep learning approaches for AgNOR-stained cytology samples interpretation
ArXiv Preprint
João Gustavo Atkinson Amorim, André Victória Matias, Allan Cerentini, Luiz Antonio Buschetto Macarini, Alexandre Sherlley Onofre, Fabiana Botelho Onofre, Aldo von Wangenheim
2022-10-19
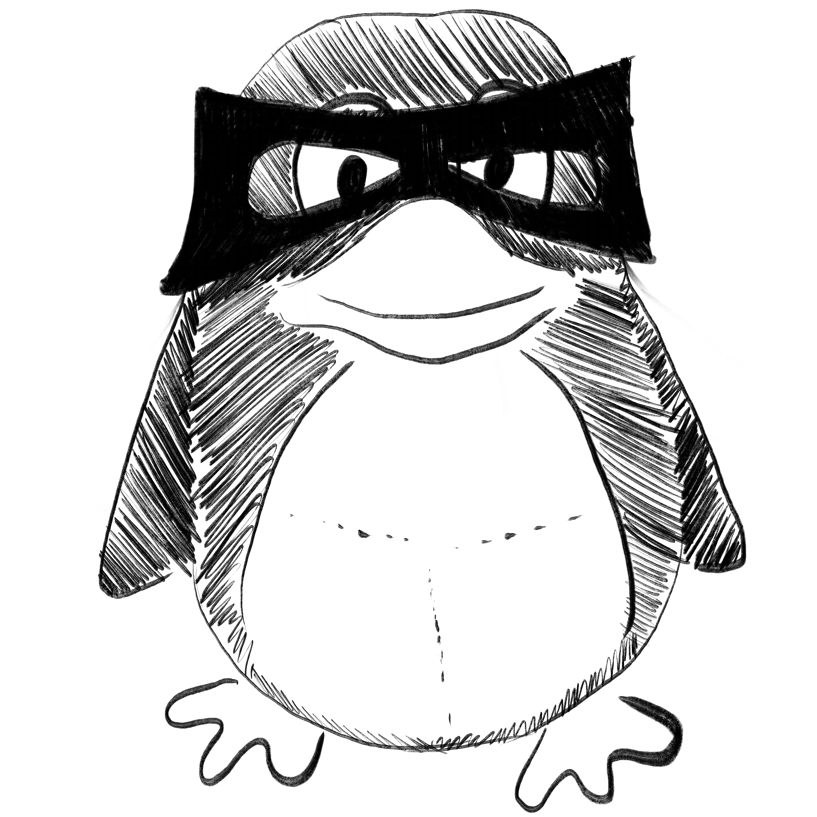
Profitability of Ichimoku-Based Trading Rule in Vietnam Stock Market in the Context of the COVID-19 Outbreak.
In Computational economics
Che-Ngoc Ha, Do-Thi Nga, Nguyen-Trang Thao
2022-Oct-13
COVID-19, Ichimoku cloud, Non-parametric statistics, Return on Investment, Vietnamese stock market
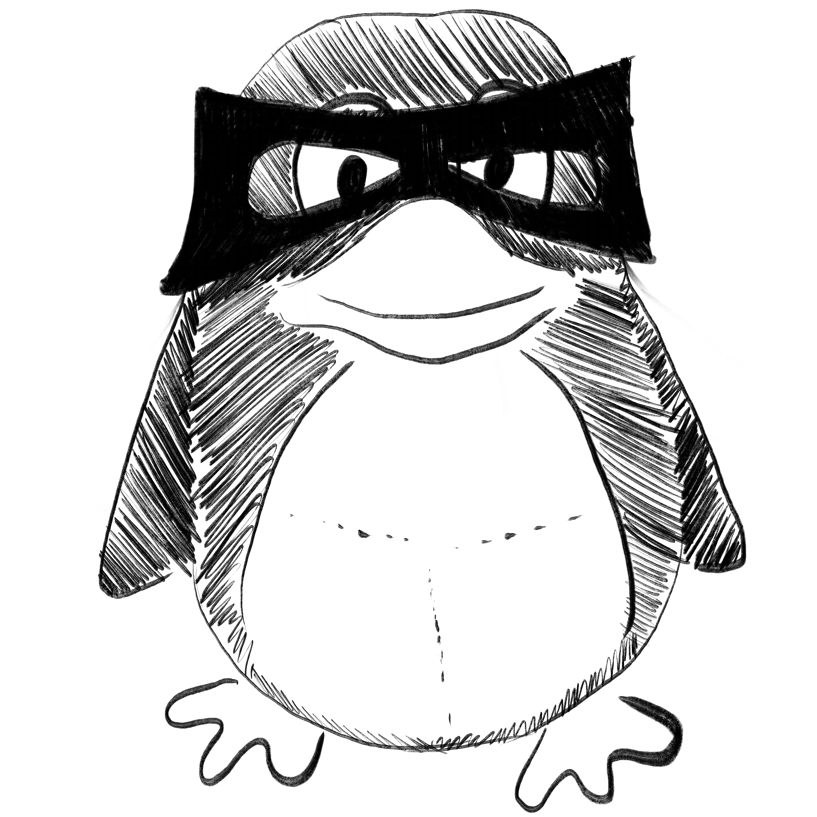
Self-learning locally-optimal hypertuning using maximum entropy, and comparison of machine learning approaches for estimating fatigue life in composite materials
ArXiv Preprint
Ismael Ben-Yelun, Miguel Diaz-Lago, Luis Saucedo-Mora, Miguel Angel Sanz, Ricardo Callado, Francisco Javier Montans
2022-10-19
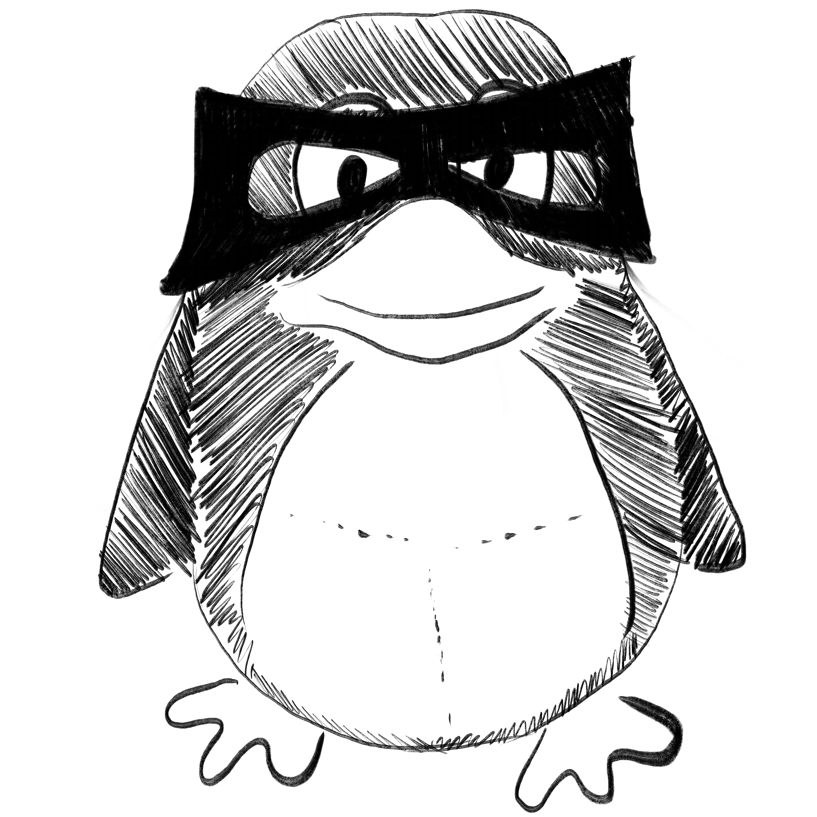
Review Learning: Alleviating Catastrophic Forgetting with Generative Replay without Generator
ArXiv Preprint
Jaesung Yoo, Sunghyuk Choi, Ye Seul Yang, Suhyeon Kim, Jieun Choi, Dongkyeong Lim, Yaeji Lim, Hyung Joon Joo, Dae Jung Kim, Rae Woong Park, Hyeong-Jin Yoon, Kwangsoo Kim
2022-10-17
Identifying pre-existing conditions and multimorbidity patterns associated with in-hospital mortality in patients with COVID-19.
In Scientific reports ; h5-index 158.0
Bucholc Magda, Bradley Declan, Bennett Damien, Patterson Lynsey, Spiers Rachel, Gibson David, Van Woerden Hugo, Bjourson Anthony J
2022-Oct-15
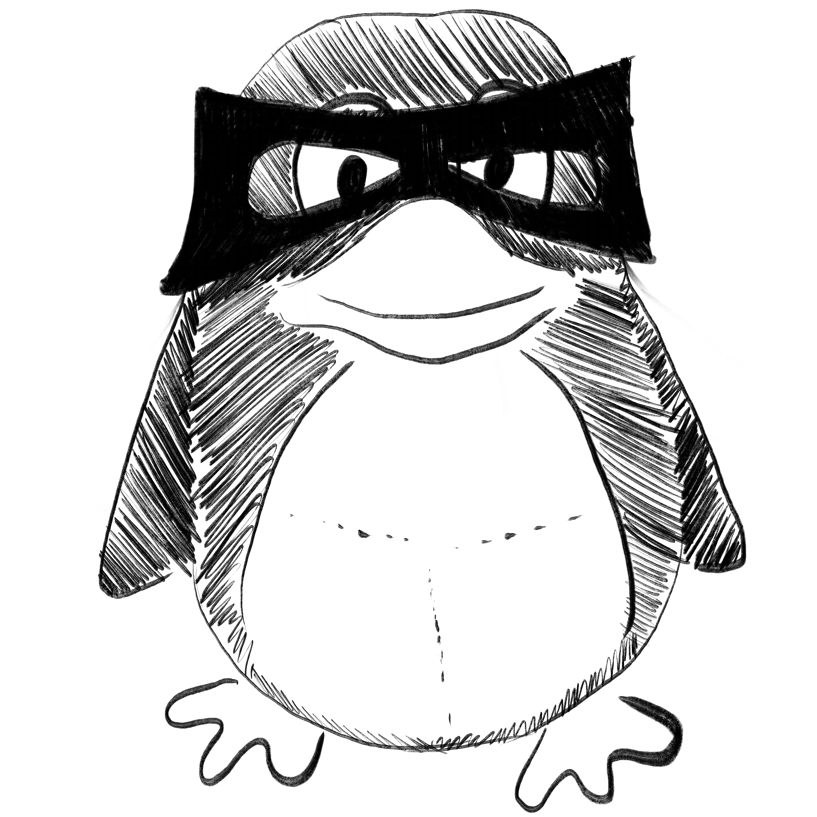
Computer-aided diagnostic for classifying chest X-ray images using deep ensemble learning.
In BMC medical imaging
BACKGROUND :
RESULTS :
CONCLUSIONS :
Visuña Lara, Yang Dandi, Garcia-Blas Javier, Carretero Jesus
2022-Oct-15
CNN, COVID-19 classification, Deep ensemble learning, Grad-CAM, Stacking, Voting
Understanding and revealing the intrinsic impacts of the COVID-19 lockdown on air quality and public health in North China using machine learning.
In The Science of the total environment
Lv Yunqian, Tian Hezhong, Luo Lining, Liu Shuhan, Bai Xiaoxuan, Zhao Hongyan, Zhang Kai, Lin Shumin, Zhao Shuang, Guo Zhihui, Xiao Yifei, Yang Junqi
2022-Oct-10
Air quality, COVID-19, Disease burden, Long-term trends, Meteorological parameters, Random forest
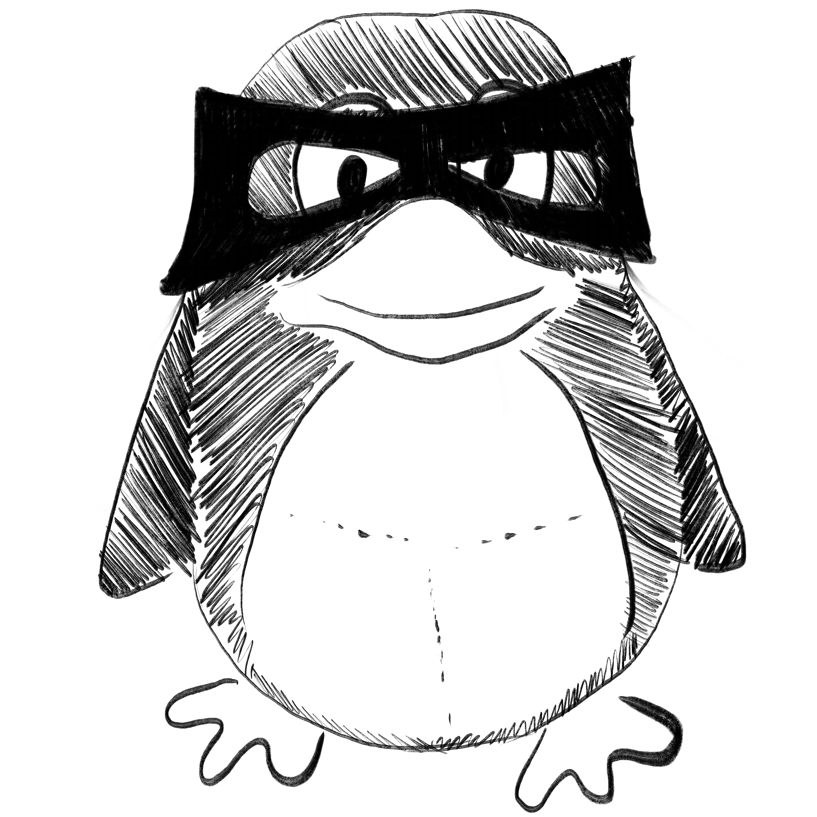
Design and development of hybrid optimization enabled deep learning model for COVID-19 detection with comparative analysis with DCNN, BIAT-GRU, XGBoost.
In Computers in biology and medicine
Dar Jawad Ahmad, Srivastava Kamal Kr, Ahmed Lone Sajaad
2022-Oct-03
(SARS-CoV-2) Covid-19 detection, Fuzzy, Hybrid optimization, Mel frequency cepstral coefficients, Neural network, Spectral centroid, Spectral flatness
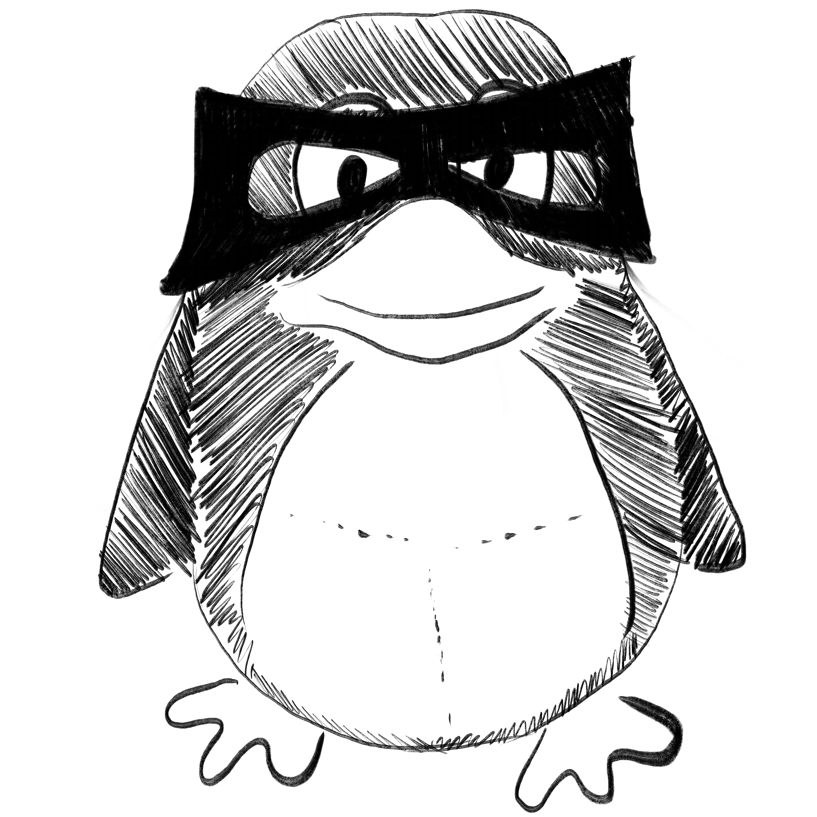
Explanatory classification of CXR images into COVID-19, Pneumonia and Tuberculosis using deep learning and XAI.
In Computers in biology and medicine
Bhandari Mohan, Shahi Tej Bahadur, Siku Birat, Neupane Arjun
2022-Oct-03
COVID-19, Deep learning, Grad-CAM, LIME, Pneumonia, SHAP, Tuberculosis, eXplainable AI
The natural language processing of radiology requests and reports of chest imaging: Comparing five transformer models' multilabel classification and a proof-of-concept study.
In Health informatics journal ; h5-index 25.0
BACKGROUND :
METHODS :
RESULTS :
CONCLUSION :
Olthof Allard W, van Ooijen Peter Ma, Cornelissen Ludo J
chest imaging, data mining, machine learning, natural language processing, radiology
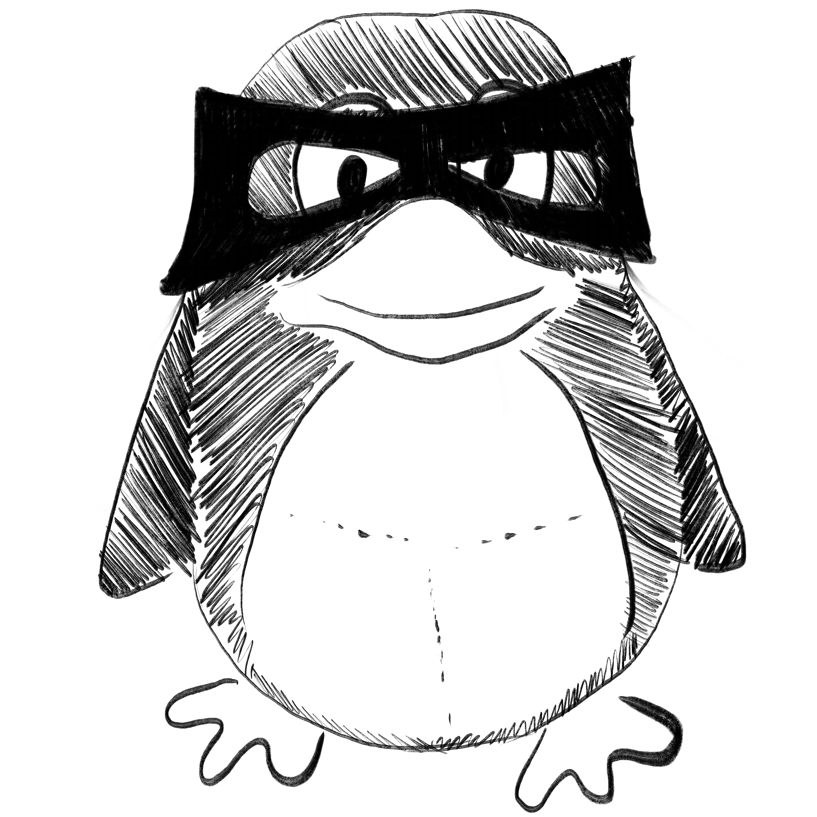
Smart healthcare: A prospective future medical approach for COVID-19.
In Journal of the Chinese Medical Association : JCMA
Yang De-Ming, Chang Tai-Jay, Hung Kai-Feng, Wang Mong-Lien, Cheng Yen-Fu, Chiang Su-Hua, Chen Mei-Fang, Liao Yi-Ting, Lai Wei-Qun, Liang Kung-Hao
2022-Oct-12
Discussions About COVID-19 Vaccination on Twitter in Turkey: Sentiment Analysis.
In Disaster medicine and public health preparedness
OBJECTIVES :
METHODS :
RESULTS :
CONCLUSIONS :
Özsezer Gözde, Mermer Gülengül
2022-Oct-13
COVID-19, Twitter, Vaccine, sentiment analysis
Deep learning techniques for detecting and recognizing face masks: A survey.
In Frontiers in public health
Alturki Rahaf, Alharbi Maali, AlAnzi Ftoon, Albahli Saleh
2022
convolutional neural network, crowd monitoring, face mask, public health, transfer learning
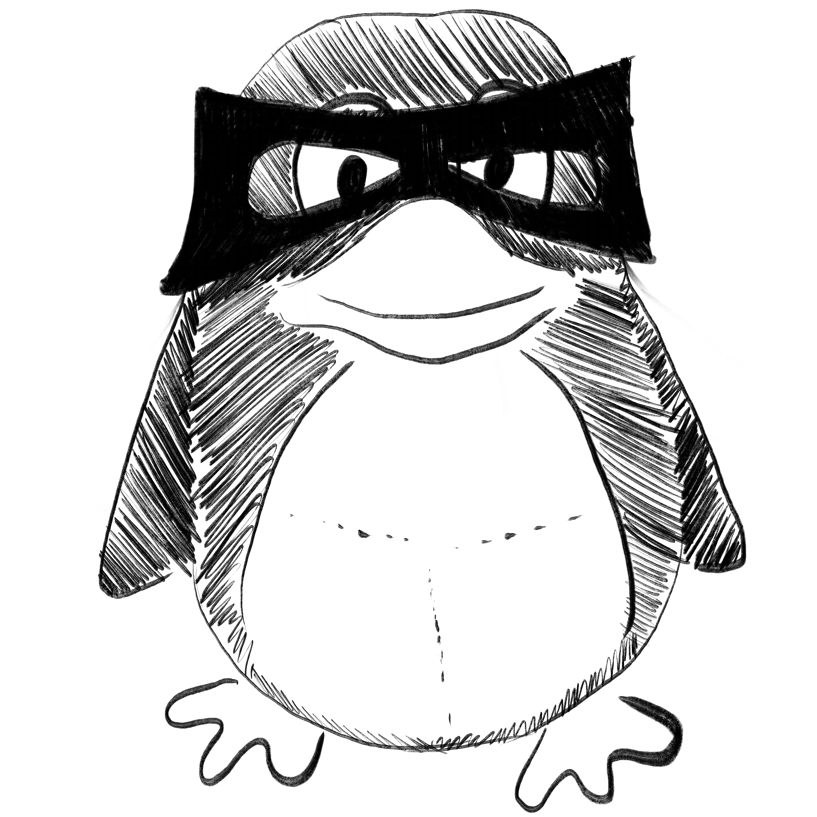
Warfarin anticoagulation management during the COVID-19 pandemic: The role of internet clinic and machine learning.
In Frontiers in pharmacology
Dai Meng-Fei, Li Shu-Yue, Zhang Ji-Fan, Wang Bao-Yan, Zhou Lin, Yu Feng, Xu Hang, Ge Wei-Hong
2022
COVID-19, anticoagulation quality, internet, machine learning, telemedicine, warfarin
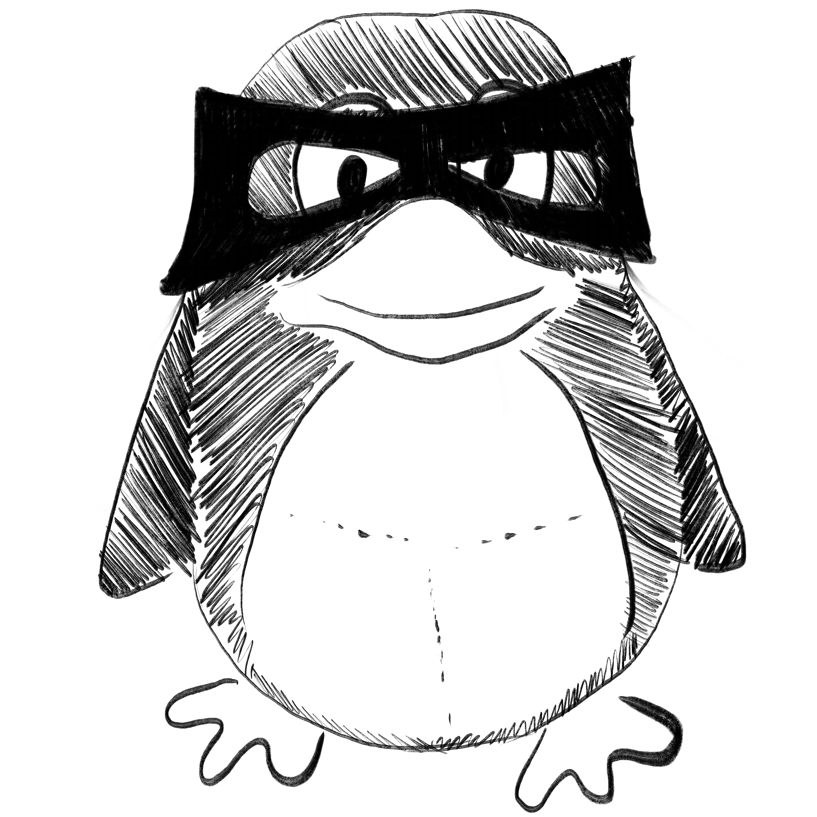
Reduced B cell antigenicity of Omicron lowers host serologic response.
In Cell reports ; h5-index 119.0
Tubiana Jérôme, Xiang Yufei, Fan Li, Wolfson Haim J, Chen Kong, Schneidman-Duhovny Dina, Shi Yi
2022-Sep-28
CP: Immunology, CP: Microbiology, Omicron variant of concern, SARS-CoV-2, antigenicity, computational structural biology, deep learning, spike protein
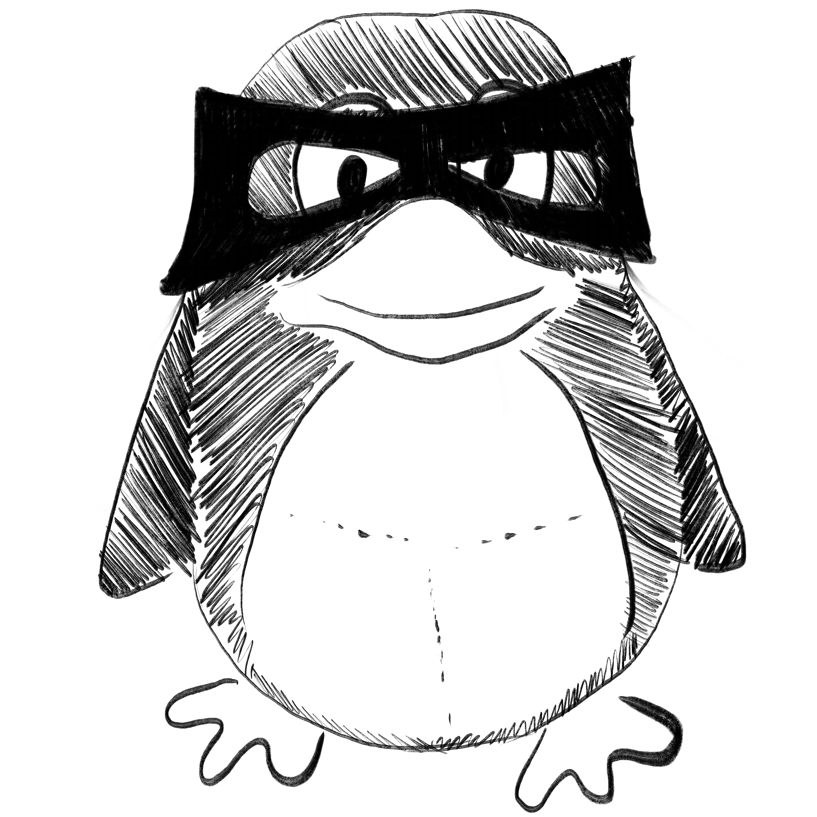
A review about COVID-19 in the MENA region: environmental concerns and machine learning applications.
In Environmental science and pollution research international
Meskher Hicham, Belhaouari Samir Brahim, Thakur Amrit Kumar, Sathyamurthy Ravishankar, Singh Punit, Khelfaoui Issam, Saidur Rahman
2022-Oct-12
Artificial intelligent, COVID-19, Environmental analysis, MENA, Machine learning, Meteorological factors
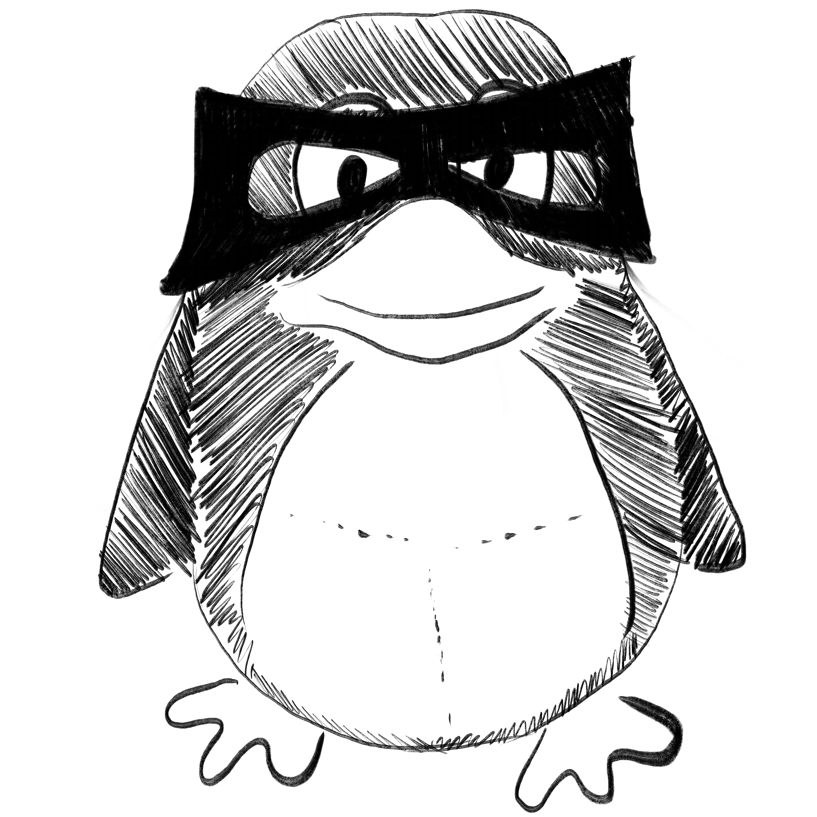
Angiotensin-converting Enzyme-2 (ACE2) Expression in Pediatric Liver Disease.
In Applied immunohistochemistry & molecular morphology : AIMM
Stevens James P, Kolachala Vasantha L, Joshi Gaurav N, Nagpal Sini, Gibson Greg, Gupta Nitika A
2022-Oct-11
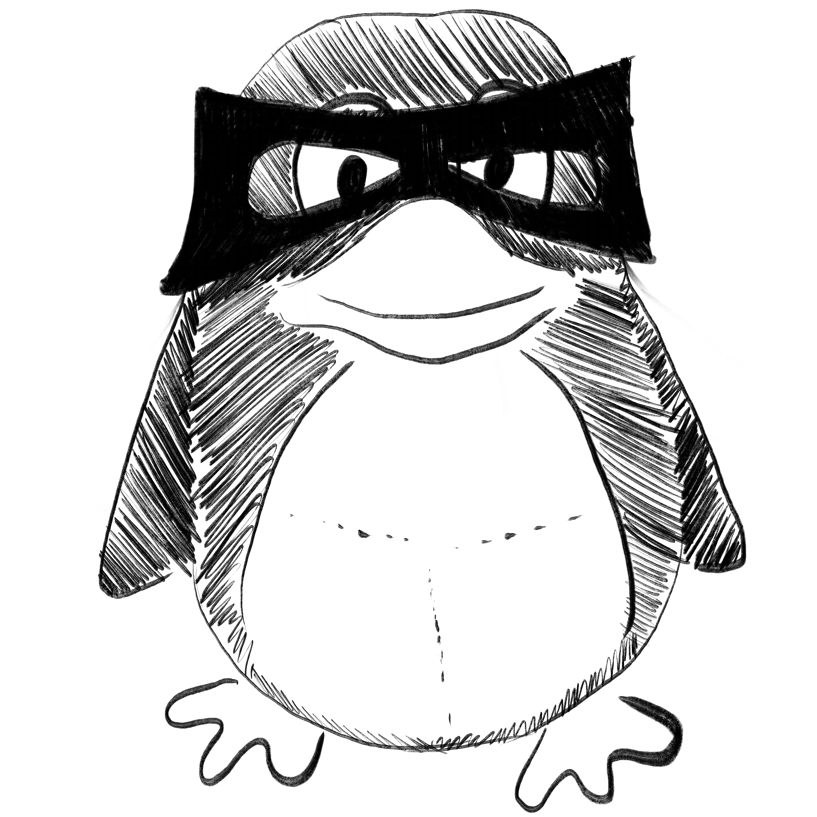
Variation in the ACE2 receptor has limited utility for SARS-CoV-2 host prediction
bioRxiv Preprint
Mollentze, N.; Keen, D.; Munkhbayar, U.; Biek, R.; Streicker, D. G.
2022-10-13
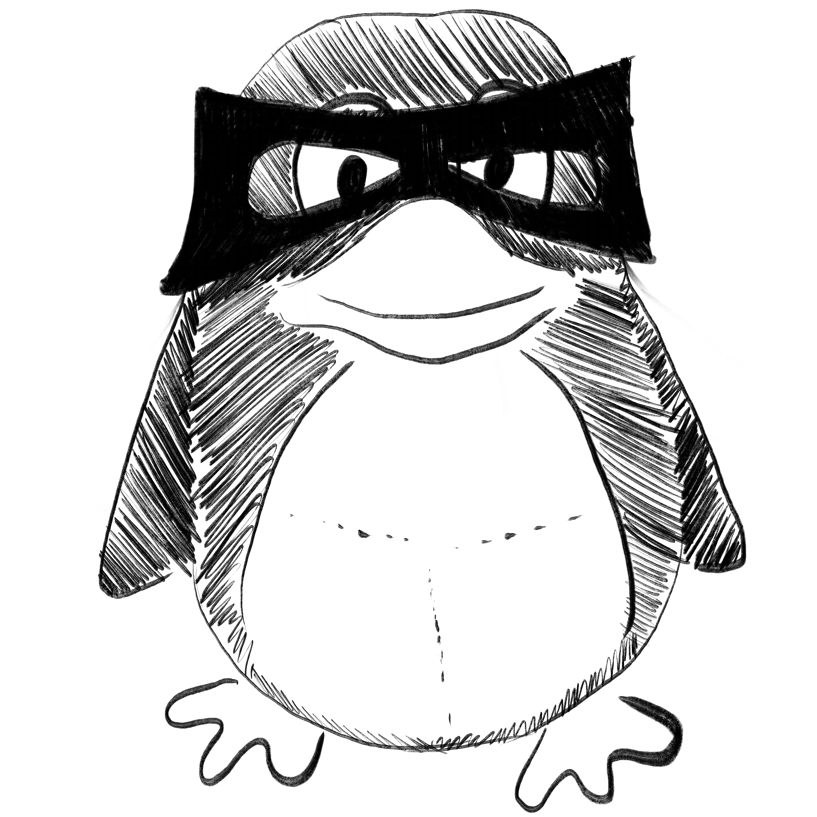
A Large-Scale Annotated Multivariate Time Series Aviation Maintenance Dataset from the NGAFID
ArXiv Preprint
Hong Yang, Travis Desell
2022-10-13
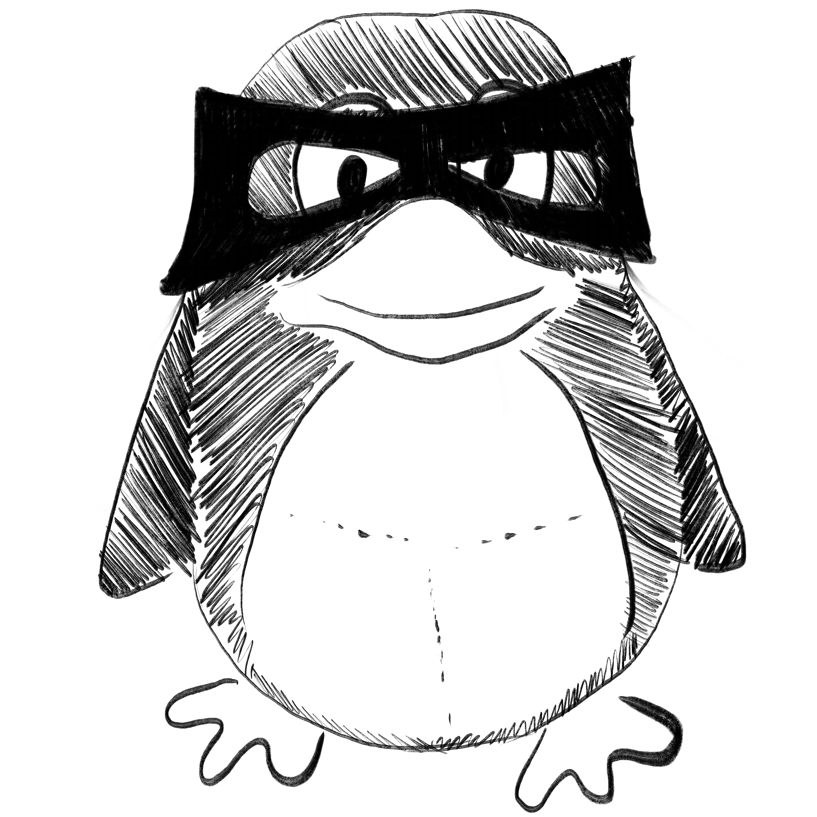
UncertaintyFuseNet: Robust uncertainty-aware hierarchical feature fusion model with Ensemble Monte Carlo Dropout for COVID-19 detection.
In An international journal on information fusion
Abdar Moloud, Salari Soorena, Qahremani Sina, Lam Hak-Keung, Karray Fakhri, Hussain Sadiq, Khosravi Abbas, Acharya U Rajendra, Makarenkov Vladimir, Nahavandi Saeid
2022-Oct-05
COVID-19, Deep learning, Early fusion, Feature fusion, Uncertainty quantification
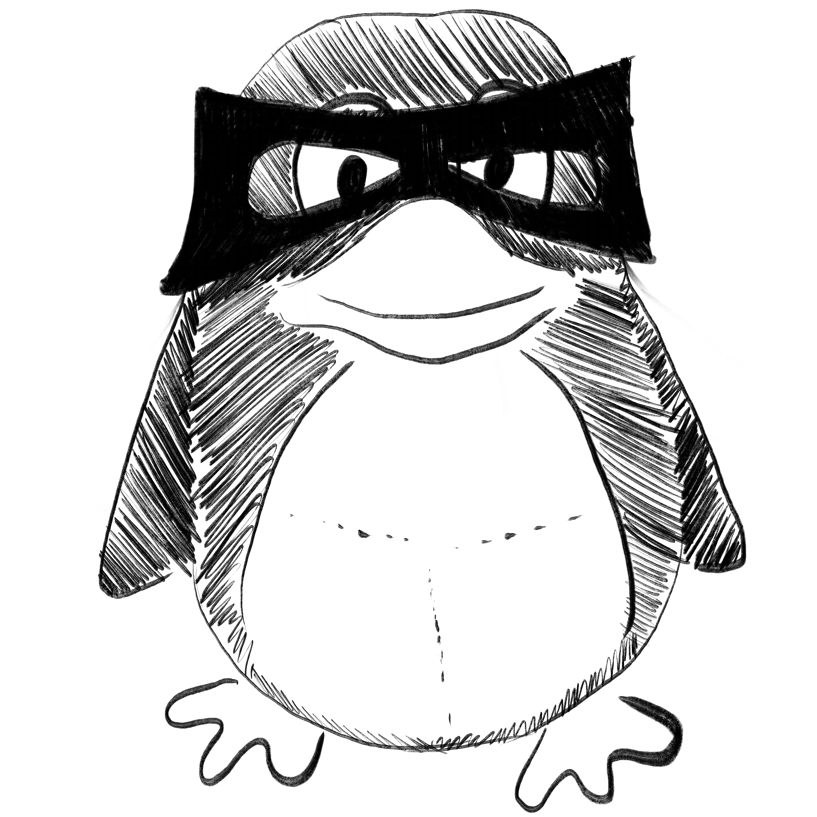
Post-script-Retail forecasting: Research and practice.
In International journal of forecasting
Fildes Robert, Kolassa Stephan, Ma Shaohui
COVID-19, Disruption, Instability, Machine learning, Omni-retailing, Online retail, Structural change
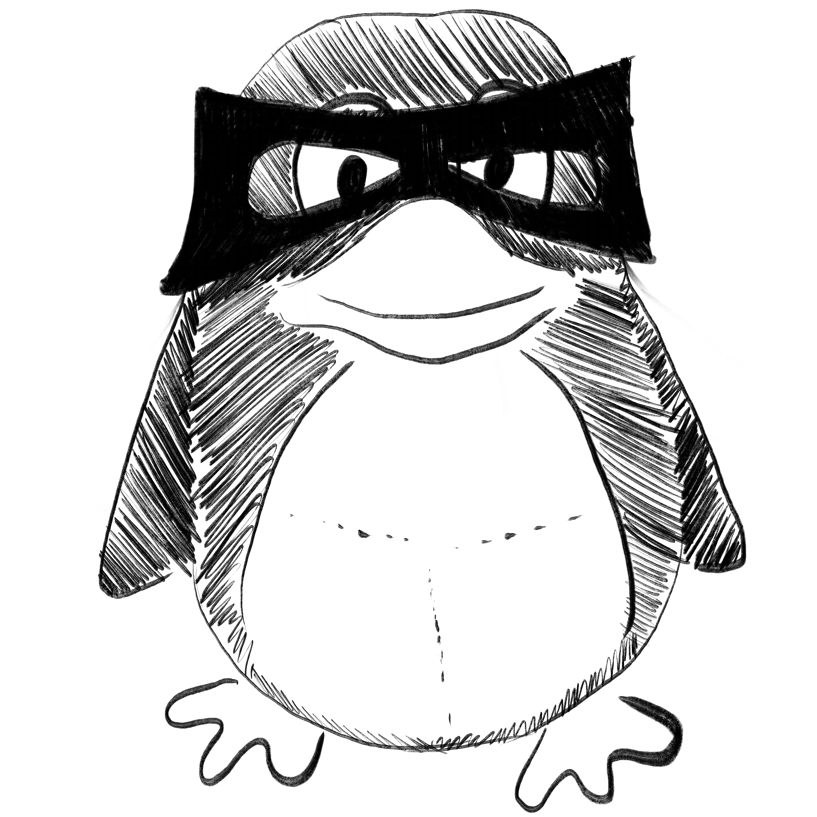
Machine learning and automatic ARIMA/Prophet models-based forecasting of COVID-19: methodology, evaluation, and case study in SAARC countries.
In Stochastic environmental research and risk assessment : research journal
Sardar Iqra, Akbar Muhammad Azeem, Leiva Víctor, Alsanad Ahmed, Mishra Pradeep
2022-Oct-05
Artificial intelligence, Facebook Prophet algorithm, GLM, R software, SARS-CoV-2, South Asian Association for Regional Cooperation countries, Time-series models
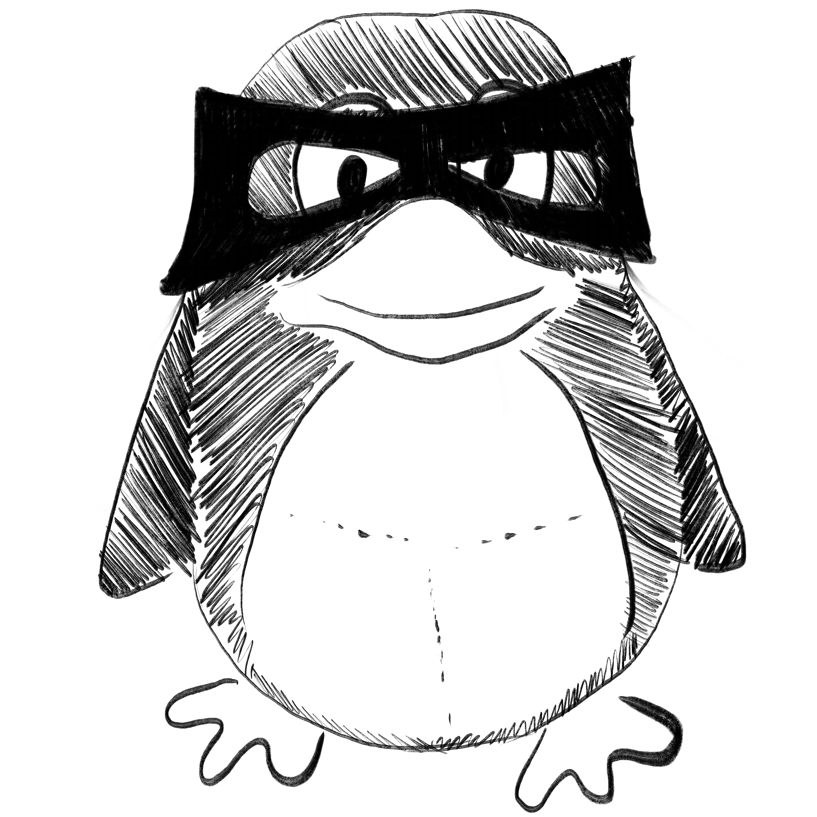
Predicting pattern of coronavirus using X-ray and CT scan images.
In Network modeling and analysis in health informatics and bioinformatics
Khurana Batra Payal, Aggarwal Paras, Wadhwa Dheeraj, Gulati Mehul
2022
CT scan, Convolutional Neural Network (CNN), Coronavirus, Deep learning, Prediction, X-ray
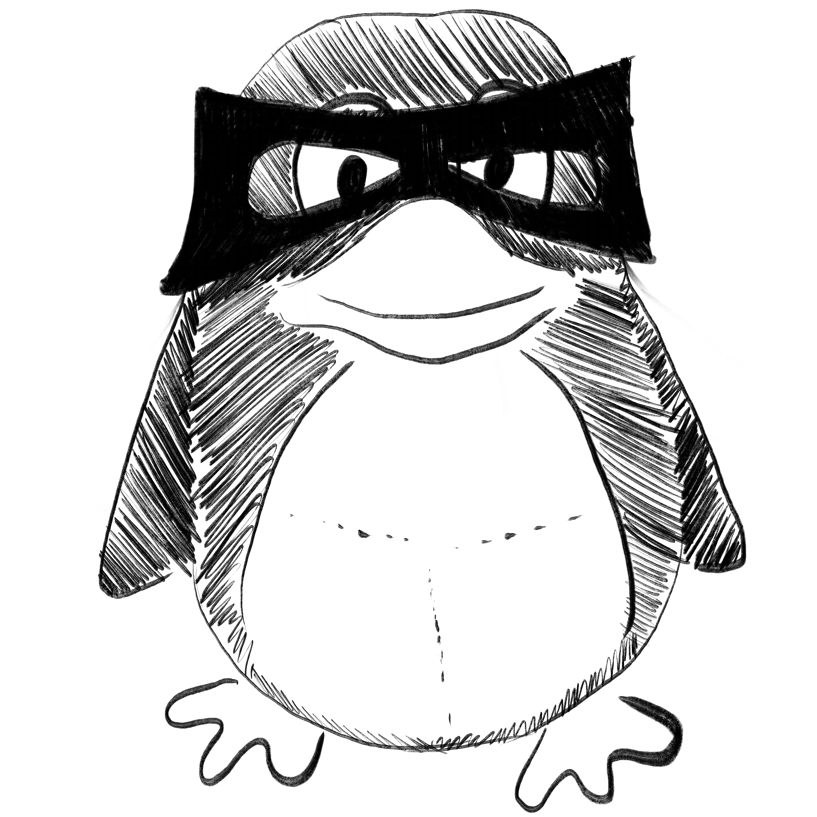
ADL-CDF: A Deep Learning Framework for COVID-19 Detection from CT Scans Towards an Automated Clinical Decision Support System.
In Arabian journal for science and engineering
Saheb Shaik Khasim, Narayanan B, Rao Thota Venkat Narayana
2022-Oct-04
Convolutional neural networks, Covid-19, Deep learning, Medical image analysis, Multi-scale feature selection
Detecting COVID-19 infection status from chest X-ray and CT scan via single transfer learning-driven approach.
In Frontiers in genetics ; h5-index 62.0
Ghose Partho, Alavi Muhaddid, Tabassum Mehnaz, Ashraf Uddin Md, Biswas Milon, Mahbub Kawsher, Gaur Loveleen, Mallik Saurav, Zhao Zhongming
2022
COVID-19, CT scan, classification, deep learning, transfer learning, x-ray
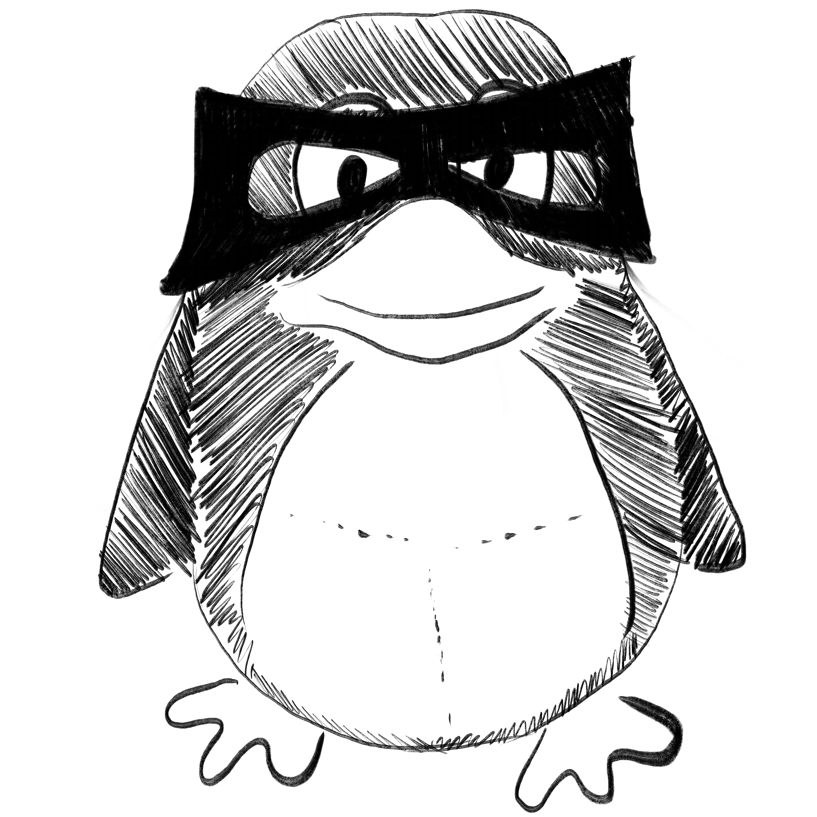
Twitter data sentiment analysis of tourism in Thailand during the COVID-19 pandemic using machine learning.
In Heliyon
Leelawat Natt, Jariyapongpaiboon Sirawit, Promjun Arnon, Boonyarak Samit, Saengtabtim Kumpol, Laosunthara Ampan, Yudha Alfan Kurnia, Tang Jing
2022-Oct
COVID19, Machine learning, Sentiment analysis, Thailand, Tourism, Tweet
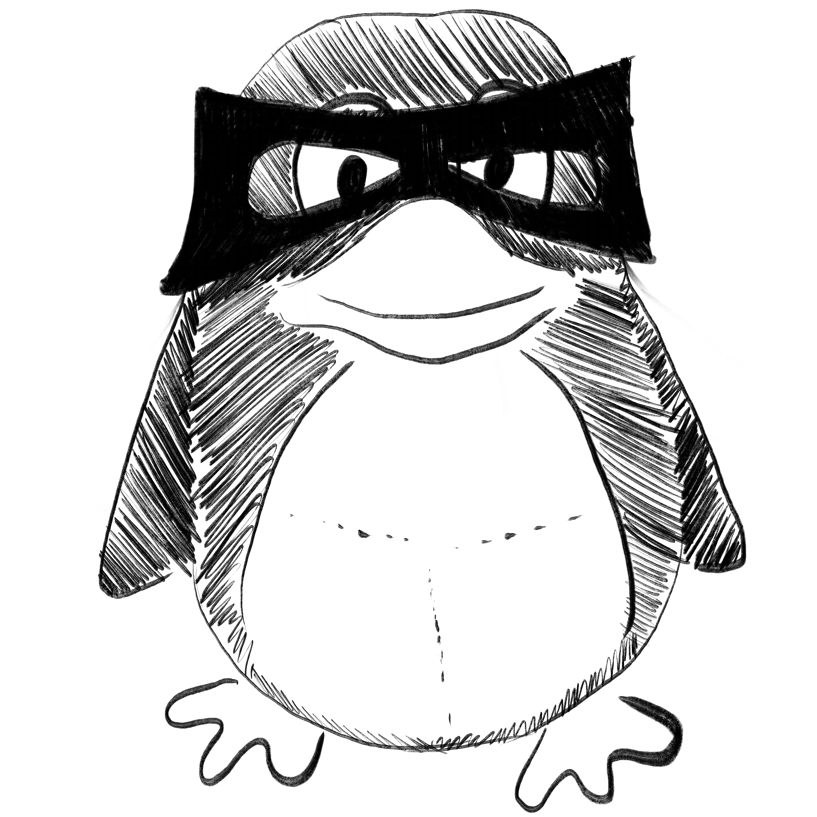
The Commoditization of AI for Molecule Design.
In Artificial intelligence in the life sciences
Urbina Fabio, Ekins Sean
2022-Dec
Artificial intelligence, design-make-test, machine learning, molecule design, recurrent neural networks
Development and validation of chest CT-based imaging biomarkers for early stage COVID-19 screening.
In Frontiers in public health
Liu Xiao-Ping, Yang Xu, Xiong Miao, Mao Xuanyu, Jin Xiaoqing, Li Zhiqiang, Zhou Shuang, Chang Hang
2022
Coronavirus Disease 2019 (COVID-19), artificial intelligence, biomedical imaging application, chest CT image, imaging biomarker, multicentric retrospective study
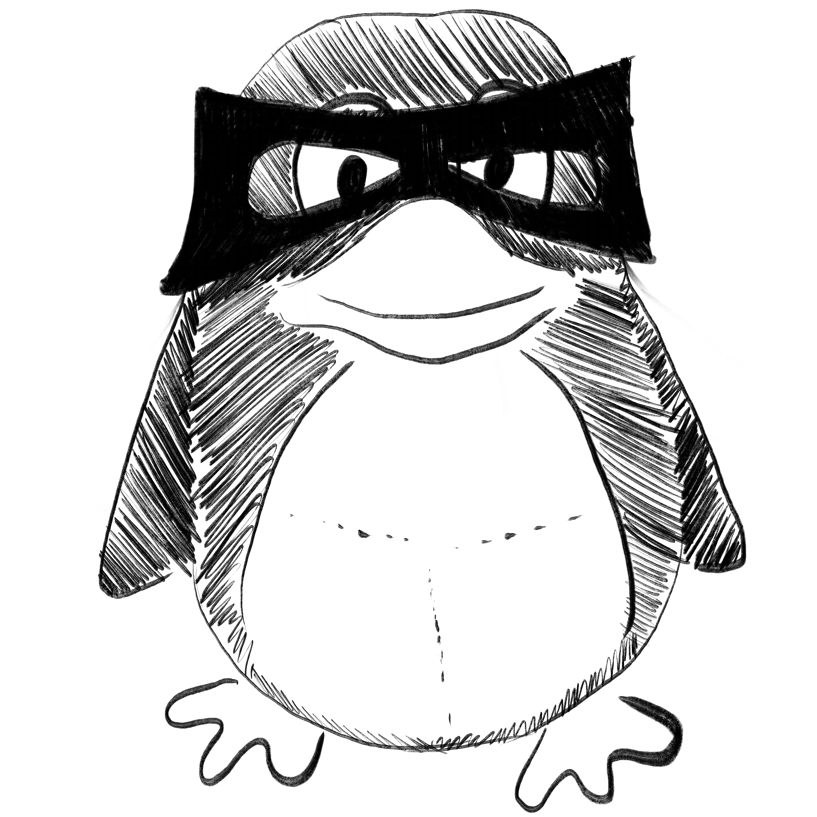
EffViT-COVID: A dual-path network for COVID-19 percentage estimation.
In Expert systems with applications
Chauhan Joohi, Bedi Jatin
2022-Oct-03
COVID-19, Deep network, EfficientNet-B7, Huber loss, Percentage estimation, Vision transformer
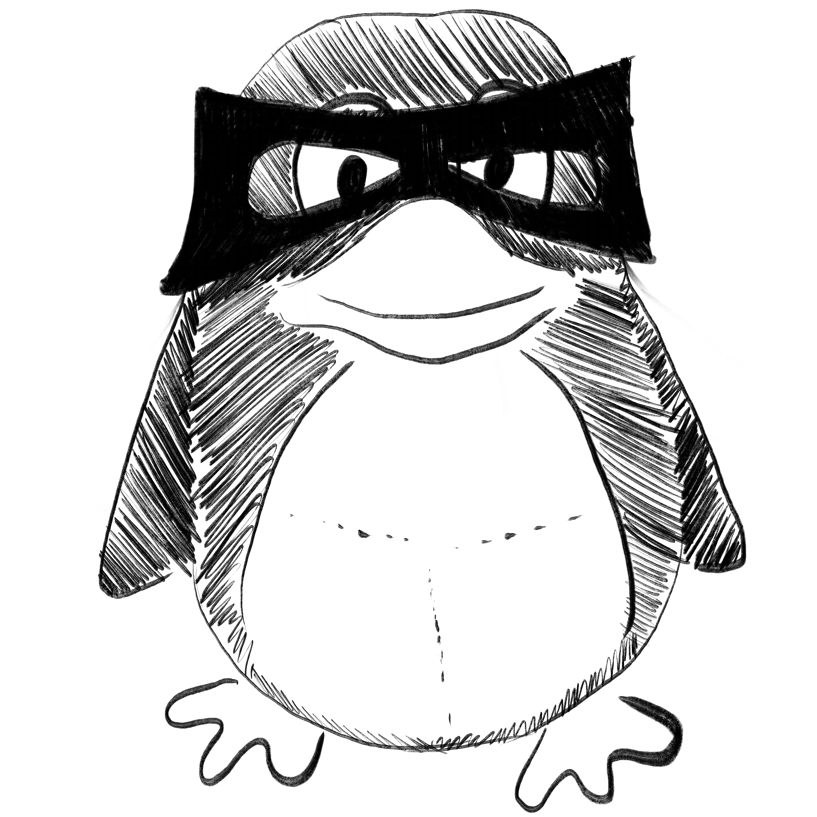
Predicting South Korean adolescents vulnerable to obesity after the COVID-19 pandemic using categorical boosting and shapley additive explanation values: A population-based cross-sectional survey.
In Frontiers in pediatrics
Objective :
Materials and methods :
Results :
Conclusion :
Byeon Haewon
2022
COVID-19 pandemic, CatBoost, adolescent, machine learning, obesity
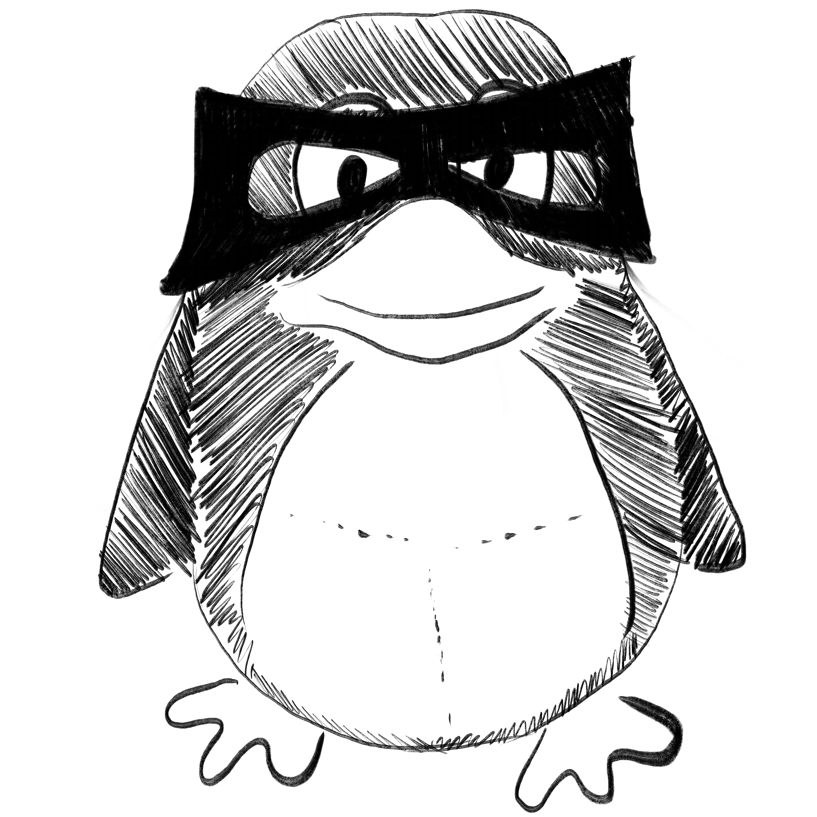
COVID-19-related Nepali Tweets Classification in a Low Resource Setting
ArXiv Preprint
Rabin Adhikari, Safal Thapaliya, Nirajan Basnet, Samip Poudel, Aman Shakya, Bishesh Khanal
2022-10-11
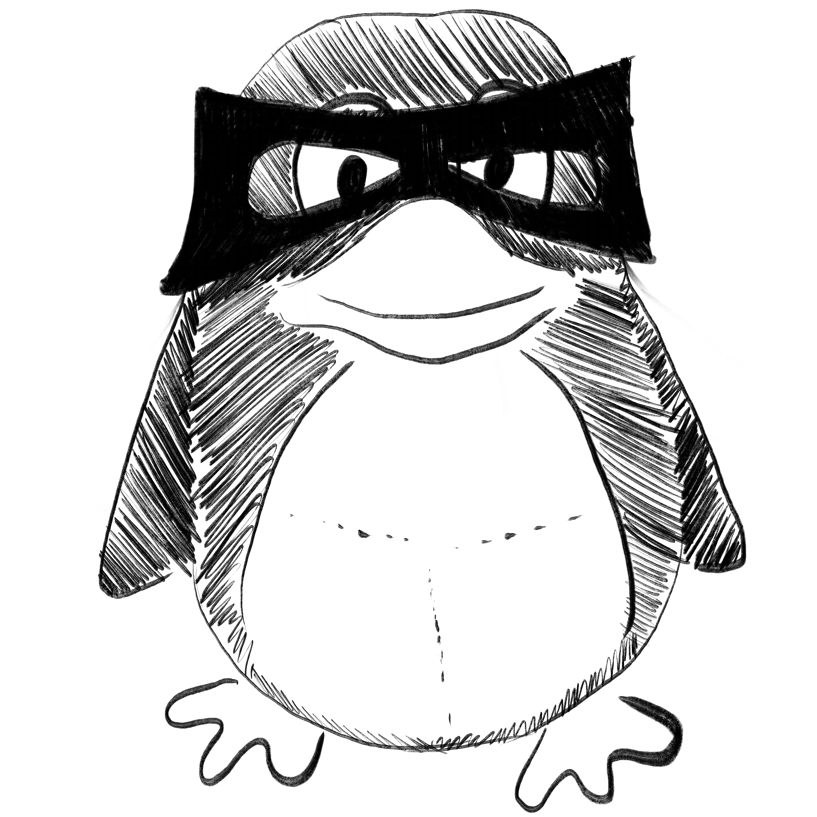
Access to online learning: Machine learning analysis from a social justice perspective.
In Education and information technologies
McIntyre Nora A
2022-Oct-04
COVID-19, Country inequalities, Educational access, Machine learning, Online learning
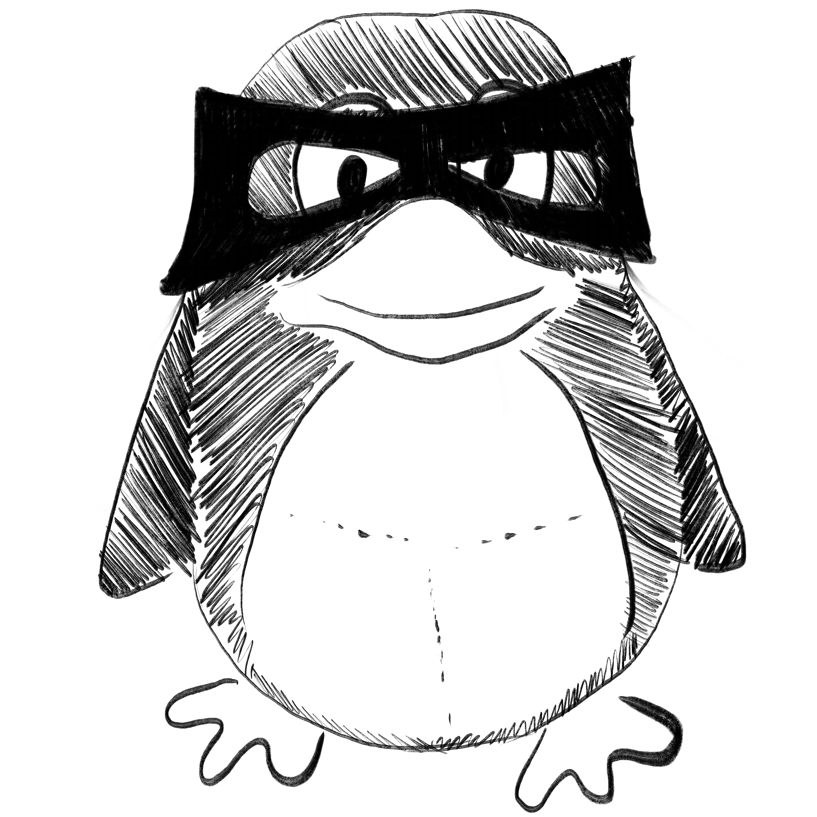
Not Good Times for Lies: Misinformation Detection on the Russia-Ukraine War, COVID-19, and Refugees
ArXiv Preprint
Cagri Toraman, Oguzhan Ozcelik, Furkan Şahinuç, Fazli Can
2022-10-11
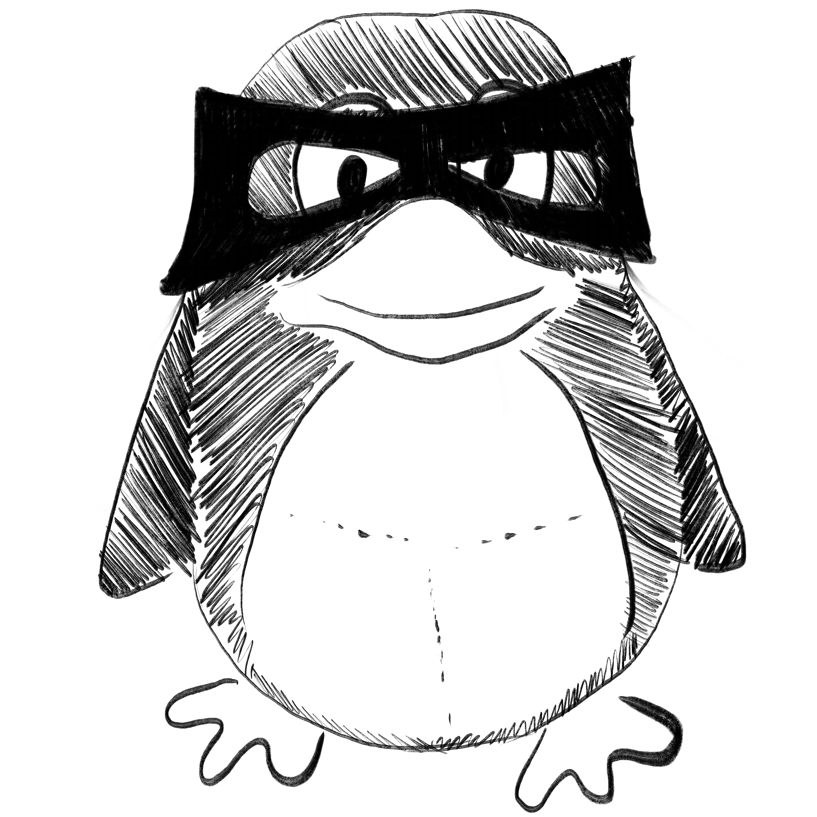
Computer especially AI-assisted drug virtual screening and design in traditional Chinese medicine.
In Phytomedicine : international journal of phytotherapy and phytopharmacology
BACKGROUND :
PURPOSE :
STUDY DESIGN :
METHODS :
RESULT :
CONCLUSIONS :
Lin Yumeng, Zhang You, Wang Dongyang, Yang Bowen, Shen Ying-Qiang
2022-Oct-01
Artificial intelligence (AI), Computer-assisted, Drug screening, Drug design, Natural products, Traditional Chinese medicine (TCM)
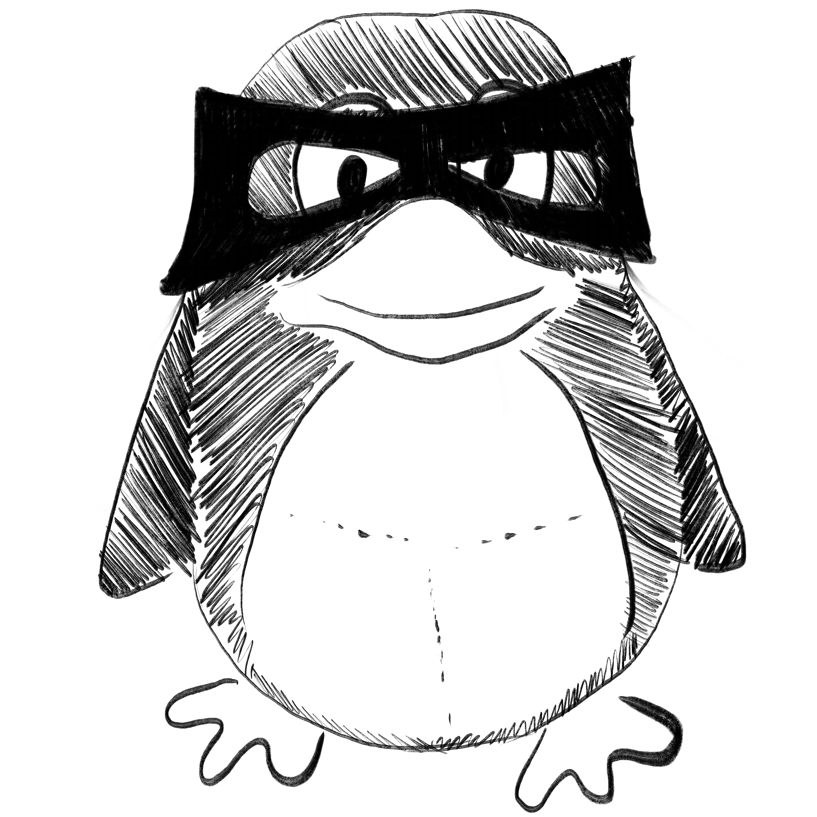
Novel chaotic oppositional fruit fly optimization algorithm for feature selection applied on COVID 19 patients' health prediction.
In PloS one ; h5-index 176.0
Bacanin Nebojsa, Budimirovic Nebojsa, K Venkatachalam, Strumberger Ivana, Alrasheedi Adel Fahad, Abouhawwash Mohamed
2022
Evaluation of Federated Learning Variations for COVID-19 diagnosis using Chest Radiographs from 42 US and European hospitals.
In Journal of the American Medical Informatics Association : JAMIA
OBJECTIVE :
MATERIALS AND METHODS :
RESULTS :
CONCLUSION :
Peng Le, Luo Gaoxiang, Walker Andrew, Zaiman Zachary, Jones Emma K, Gupta Hemant, Kersten Kristopher, Burns John L, Harle Christopher A, Magoc Tanja, Shickel Benjamin, Steenburg Scott D, Loftus Tyler, Melton Genevieve B, Gichoya Judy Wawira, Sun Ju, Tignanelli Christopher J
2022-Oct-10
Artificial Intelligence, COVID-19, Computer Vision, Federated Learning
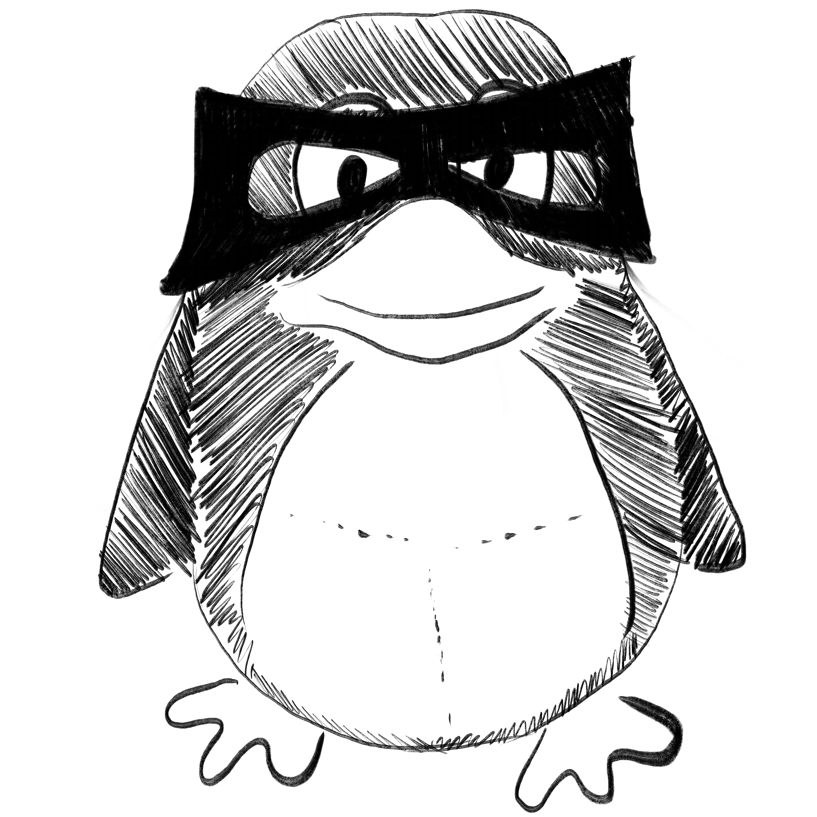
MO-MEMES: A method for accelerating virtual screening using multi-objective Bayesian optimization.
In Frontiers in medicine
Mehta Sarvesh, Goel Manan, Priyakumar U Deva
2022
Bayesian optimization, High throughout screening, chemical space exploration, drug discovery, machine learning, virtual screening
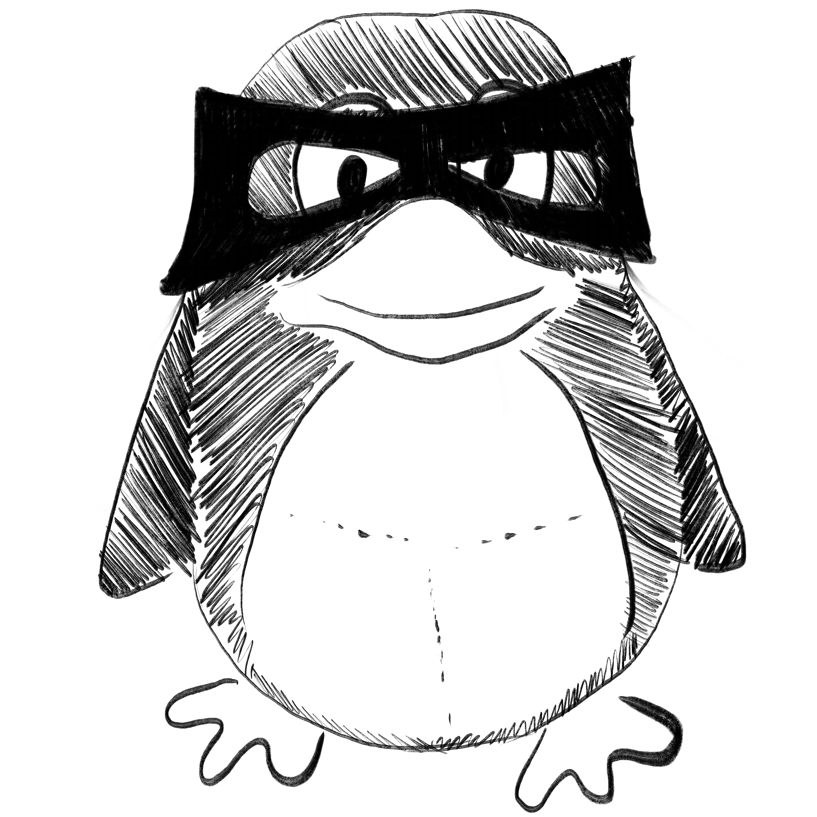
An implementation of a hybrid method based on machine learning to identify biomarkers in the Covid-19 diagnosis using DNA sequences.
In Chemometrics and intelligent laboratory systems : an international journal sponsored by the Chemometrics Society
Das Bihter
2022-Nov-15
Big data analysis, Biomedical signal processing, Covid-19, Linear algebra, Machine learning
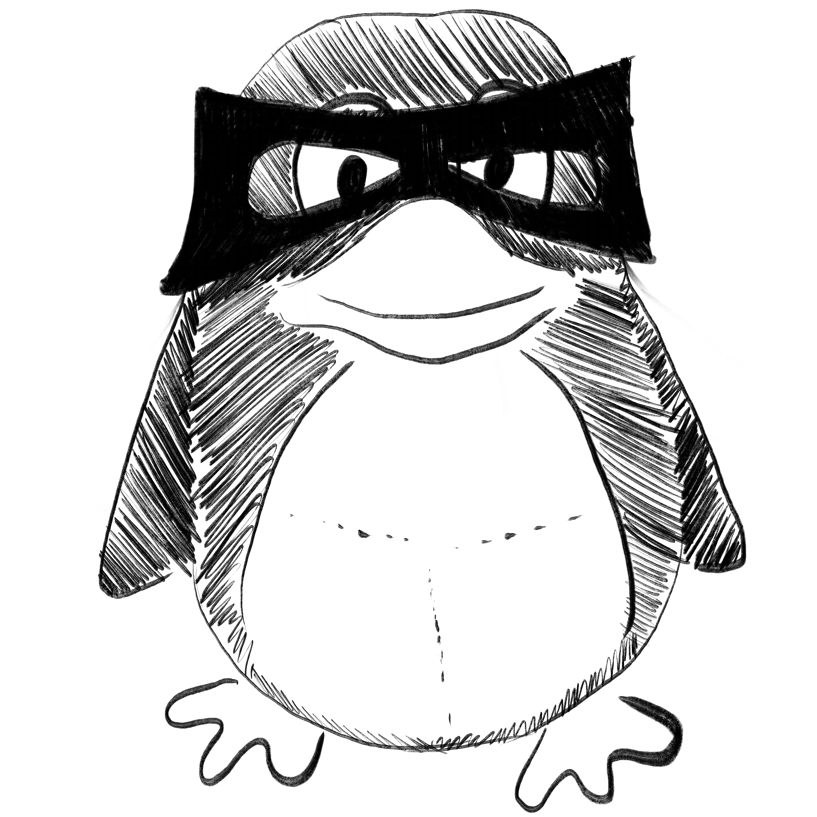
Audits and COVID-19: A paradigm shift in the making.
In Business horizons
Castka Pavel, Searcy Cory
2021-Nov-18
Audit, Certification, Inspection, Technology, Testing
Identification of methylation signatures and rules for predicting the severity of SARS-CoV-2 infection with machine learning methods.
In Frontiers in microbiology
Liu Zhiyang, Meng Mei, Ding ShiJian, Zhou XiaoChao, Feng KaiYan, Huang Tao, Cai Yu-Dong
2022
SARS-CoV-2, classification rule, machine learning, methylation, severity
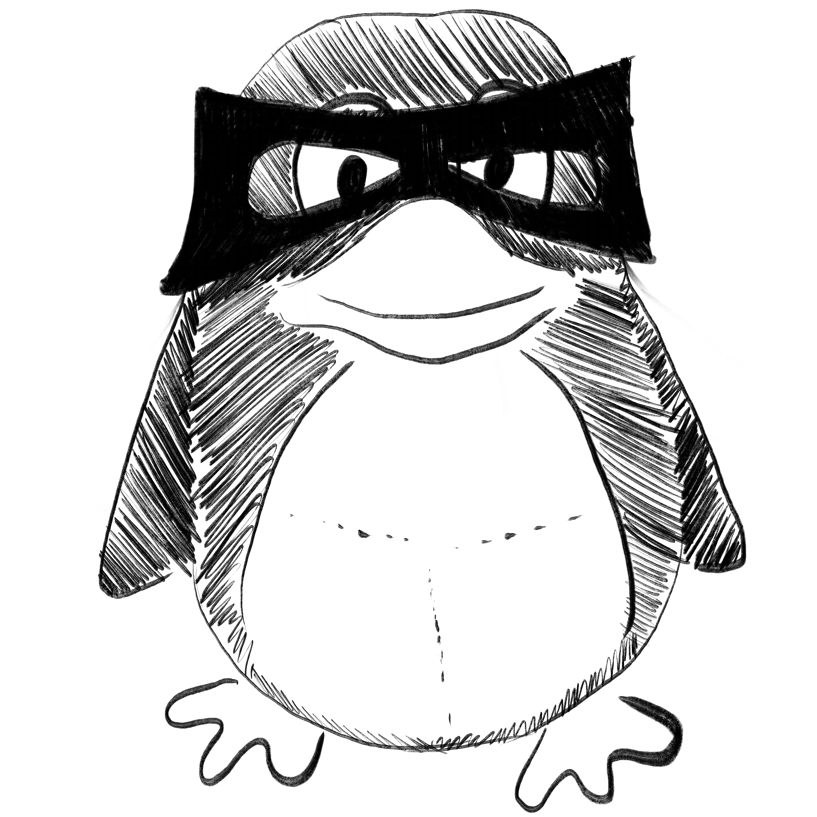
Machine learning-based derivation and external validation of a tool to predict death and development of organ failure in hospitalized patients with COVID-19.
In Scientific reports ; h5-index 158.0
Xu Yixi, Trivedi Anusua, Becker Nicholas, Blazes Marian, Ferres Juan Lavista, Lee Aaron, Conrad Liles W, Bhatraju Pavan K
2022-Oct-08
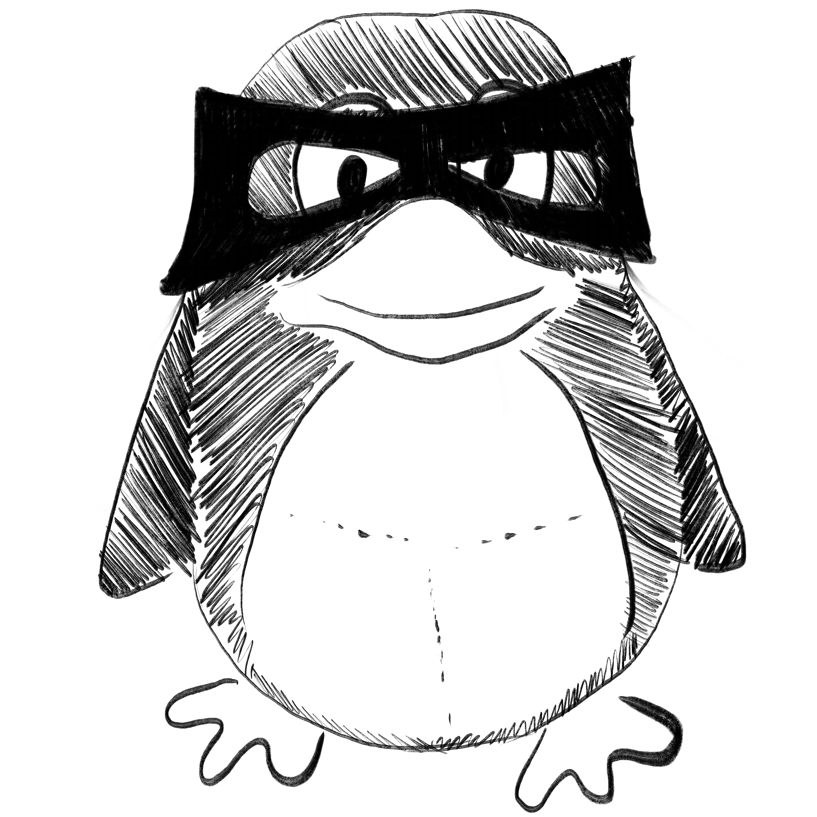
The COVIDTW study: Clinical predictors of COVID-19 mortality and a novel AI prognostic model using chest X-ray.
In Journal of the Formosan Medical Association = Taiwan yi zhi
BACKGROUND :
METHOD :
RESULT :
CONCLUSION :
Wu Chih-Wei, Pham Bach-Tung, Wang Jia-Ching, Wu Yao-Kuang, Kuo Chan-Yen, Hsu Yi-Chiung
2022-Sep-26
Artificial intelligence, COVID-19, Chest X-rays, Intensive care unit, Mortality, Prognosis
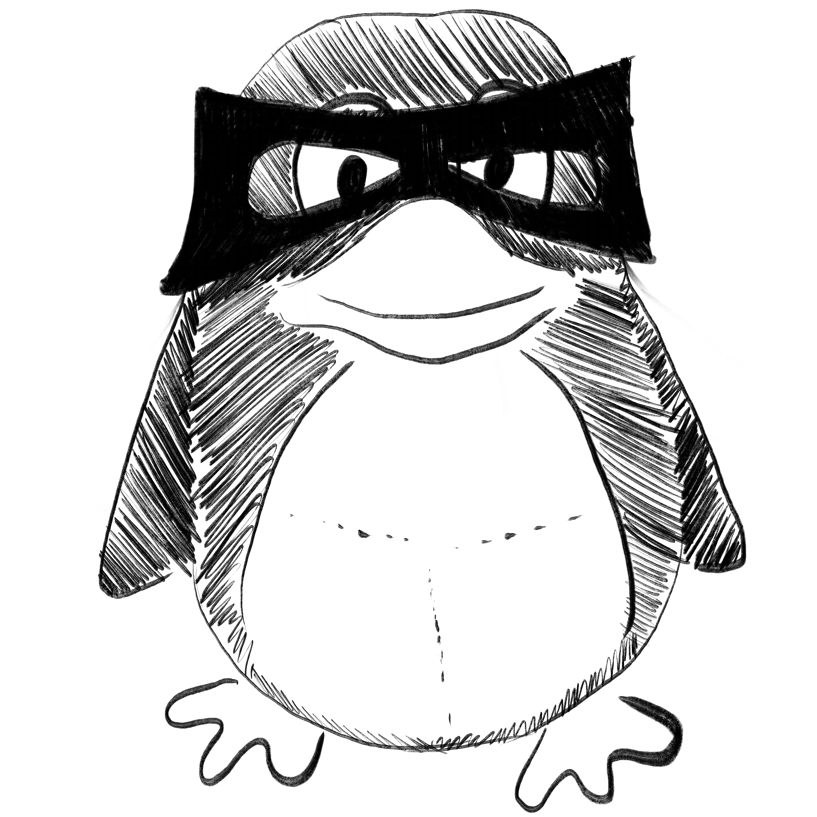
Impact of Covid-19 on research and training in Parkinson's disease.
In International review of neurobiology
Wan Yi-Min, van Wamelen Daniel J, Lau Yue Hui, Rota Silvia, Tan Eng-King
2022
Covid-19, Impact, Movement disorders, “Parkinsons disease”, Research, Training
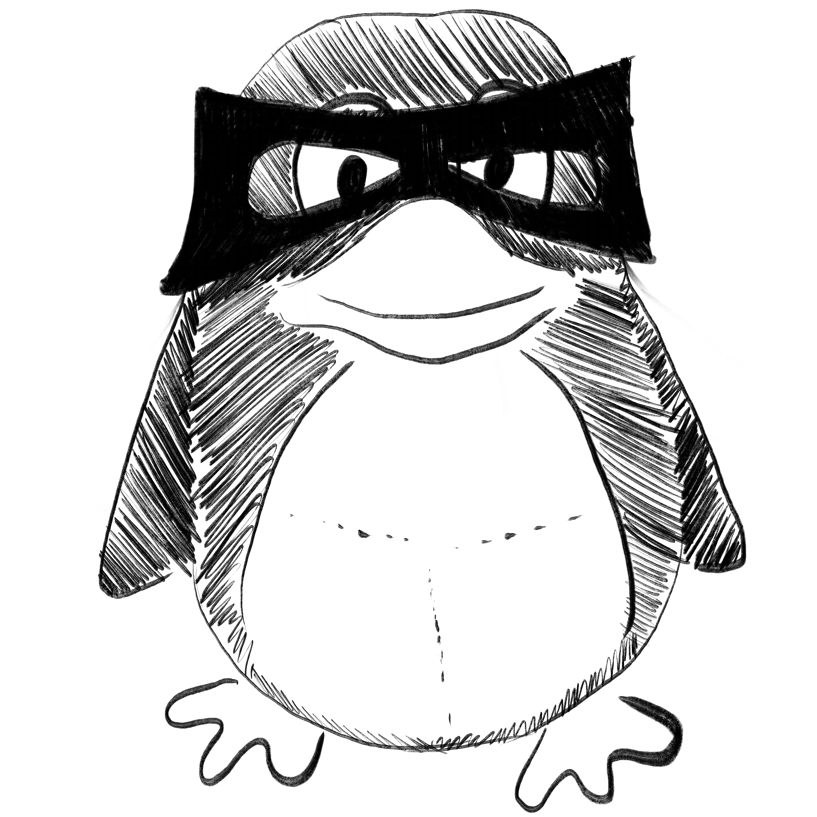
Mathematical modeling and AI based decision making for COVID-19 suspects backed by novel distance and similarity measures on plithogenic hypersoft sets.
In Artificial intelligence in medicine ; h5-index 34.0
Ahmad Muhammad Rayees, Afzal Usman
2022-Oct
COVID-19, Multi-criteria decision making (MCDM), Plithogenic distance measure (PDM), Plithogenic hypersoft set (PHSS), Plithogenic similarity measure (PSM)
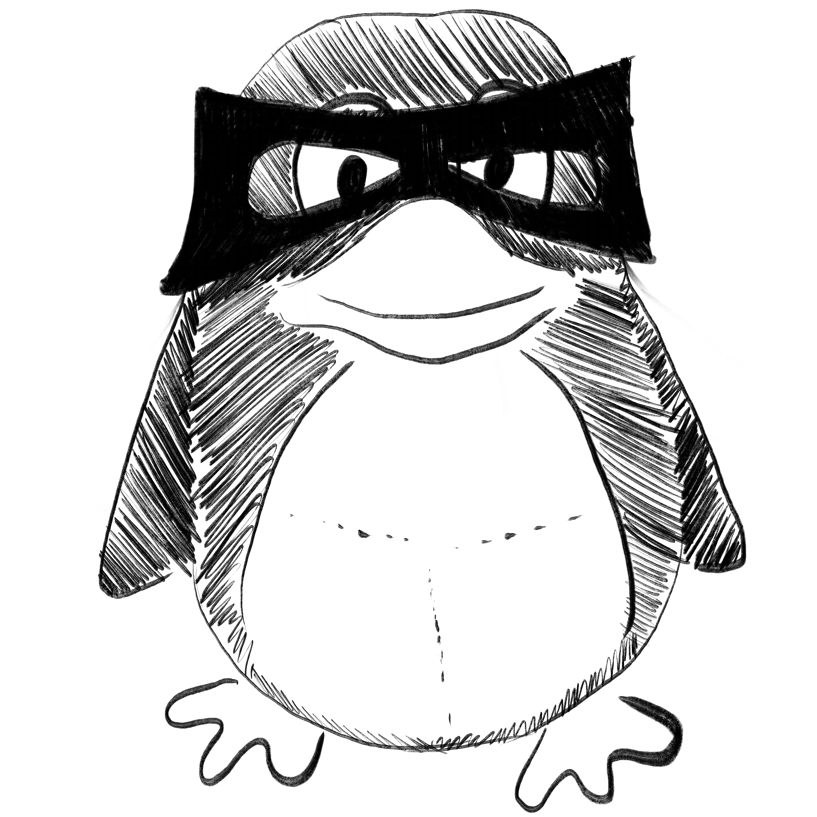
Defining factors in hospital admissions during COVID-19 using LSTM-FCA explainable model.
In Artificial intelligence in medicine ; h5-index 34.0
Md Saleh Nurul Izrin, Ab Ghani Hadhrami, Jilani Zairul
2022-Oct
COVID-19, Formal Concept Analysis (FCA), Hospital admissions, Long Short-Term Memory (LSTM)
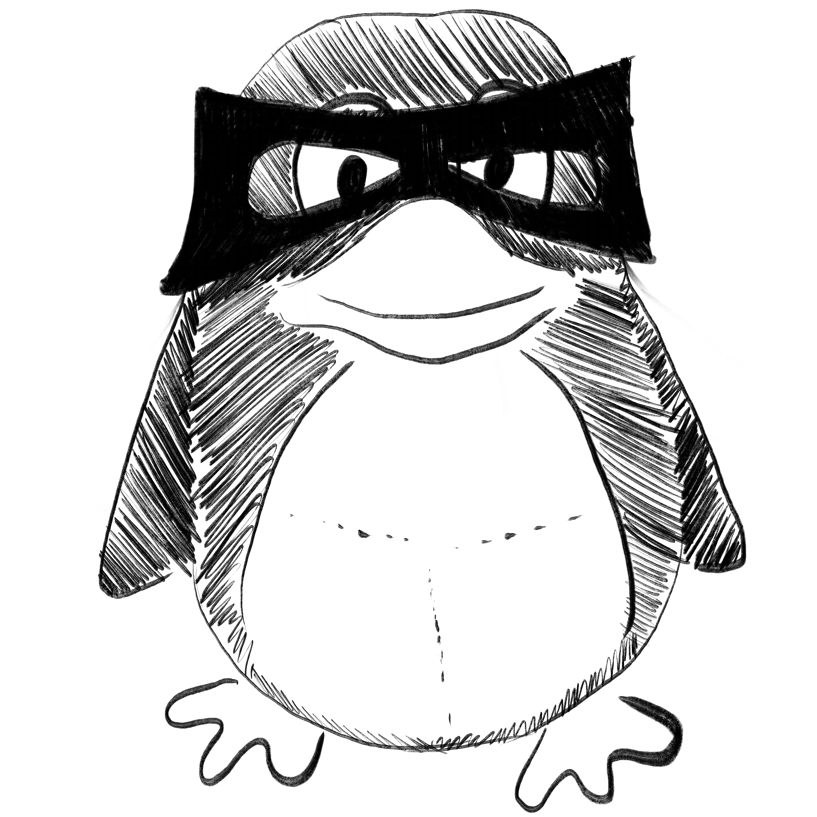
A self-supervised COVID-19 CT recognition system with multiple regularizations.
In Computers in biology and medicine
Lu Han, Dai Qun
2022-Sep-29
COVID-19 CT Diagnosis, Contrastive learning, Deep neural network, Ensemble learning, Loss regularization
RadImageNet: An Open Radiologic Deep Learning Research Dataset for Effective Transfer Learning.
In Radiology. Artificial intelligence
Purpose :
Materials and Methods :
Results :
Conclusion :
Mei Xueyan, Liu Zelong, Robson Philip M, Marinelli Brett, Huang Mingqian, Doshi Amish, Jacobi Adam, Cao Chendi, Link Katherine E, Yang Thomas, Wang Ying, Greenspan Hayit, Deyer Timothy, Fayad Zahi A, Yang Yang
2022-Sep
Brain/Brain Stem, CT, Computer Applications–General (Informatics), Evidence-based Medicine, Head/Neck, MR Imaging, Thorax, US
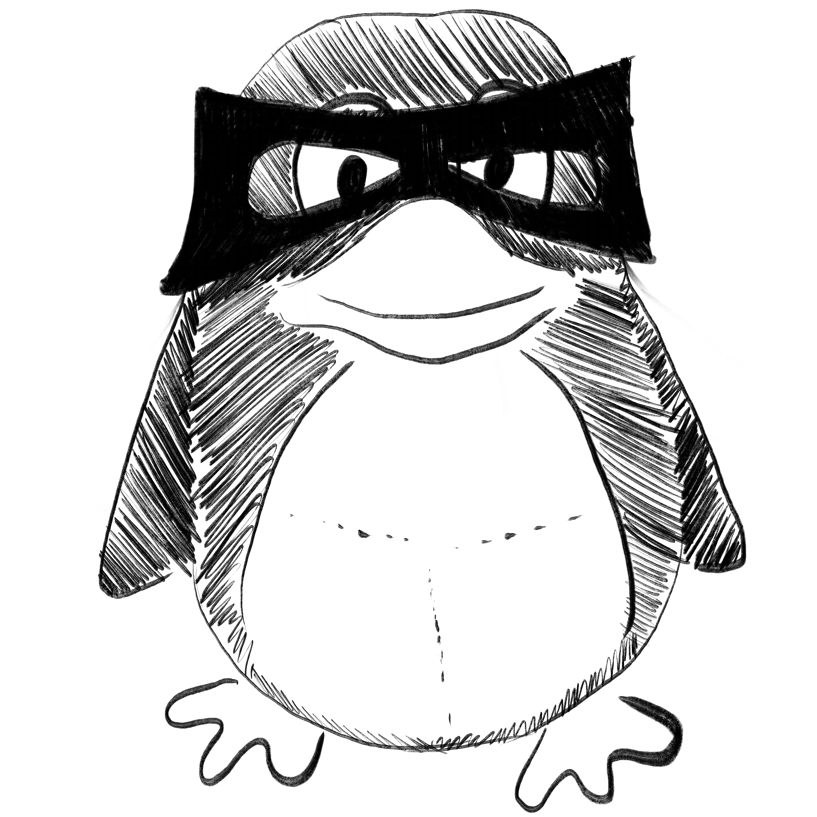
Difference in mortality rates in hospitalized COVID-19 patients identified by cytokine profile clustering using a machine learning approach: An outcome prediction alternative.
In Frontiers in medicine
Castro-Castro Ana Cristina, Figueroa-Protti Lucia, Molina-Mora Jose Arturo, Rojas-Salas María Paula, Villafuerte-Mena Danae, Suarez-Sánchez María José, Sanabría-Castro Alfredo, Boza-Calvo Carolina, Calvo-Flores Leonardo, Solano-Vargas Mariela, Madrigal-Sánchez Juan José, Sibaja-Campos Mario, Silesky-Jiménez Juan Ignacio, Chaverri-Fernández José Miguel, Soto-Rodríguez Andrés, Echeverri-McCandless Ann, Rojas-Chaves Sebastián, Landaverde-Recinos Denis, Weigert Andreas, Mora Javier
2022
COVID-19, CXCL10, IL-38, SARS-CoV-2, cytokine profile
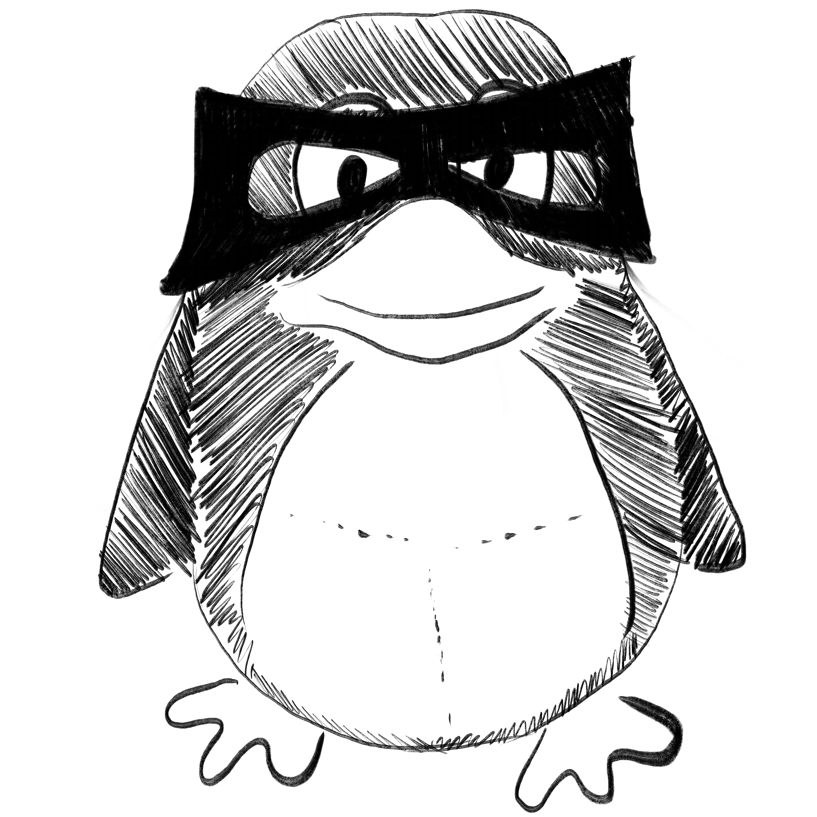
Blood gene expression predicts intensive care unit admission in hospitalised patients with COVID-19.
In Frontiers in immunology ; h5-index 100.0
Background :
Methods :
Results :
Conclusions :
Penrice-Randal Rebekah, Dong Xiaofeng, Shapanis Andrew George, Gardner Aaron, Harding Nicholas, Legebeke Jelmer, Lord Jenny, Vallejo Andres F, Poole Stephen, Brendish Nathan J, Hartley Catherine, Williams Anthony P, Wheway Gabrielle, Polak Marta E, Strazzeri Fabio, Schofield James P R, Skipp Paul J, Hiscox Julian A, Clark Tristan W, Baralle Diana
2022
COVID-19, Critical Care, RNA-seq - RNA sequencing, biomarkers, prognosis, topology, transcriptome
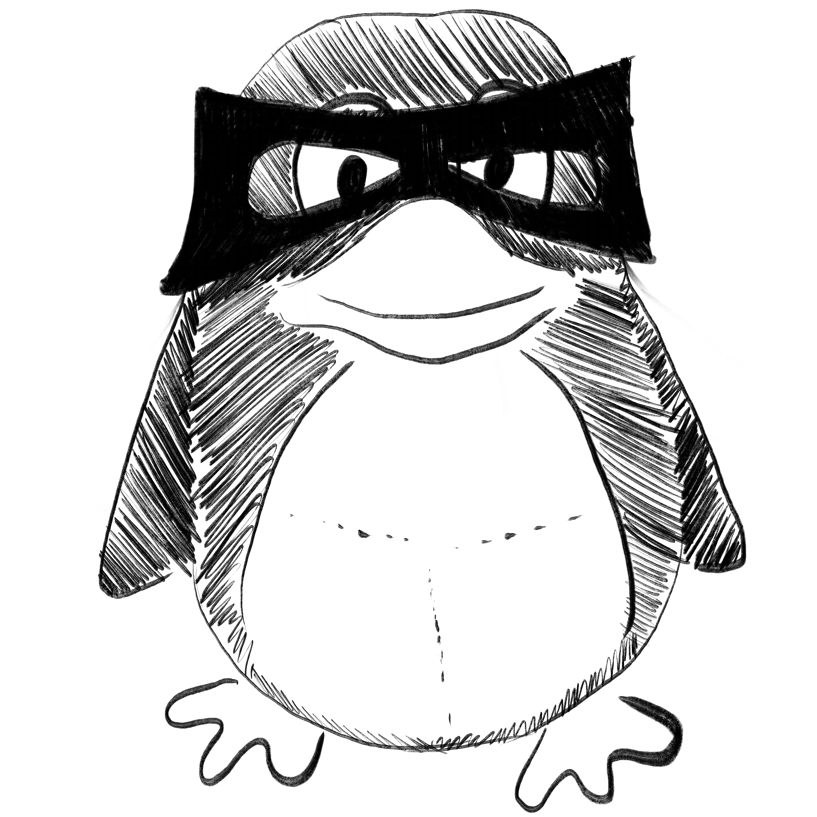
Hospital trajectories and early predictors of clinical outcomes differ between SARS-CoV-2 and influenza pneumonia.
In EBioMedicine
BACKGROUND :
METHODS :
FINDINGS :
INTERPRETATION :
FUNDING :
Lyons Patrick G, Bhavani Sivasubramanium V, Mody Aaloke, Bewley Alice, Dittman Katherine, Doyle Aisling, Windham Samuel L, Patel Tej M, Raju Bharat Neelam, Keller Matthew, Churpek Matthew M, Calfee Carolyn S, Michelson Andrew P, Kannampallil Thomas, Geng Elvin H, Sinha Pratik
2022-Oct-03
Hospital outcomes, Influenza, SARS-CoV-2, Statistical modelling, Viral pneumonia
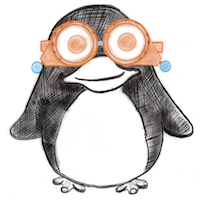
Deep learning models for COVID-19 chest x-ray classification: Preventing shortcut learning using feature disentanglement.
In PloS one ; h5-index 176.0
Trivedi Anusua, Robinson Caleb, Blazes Marian, Ortiz Anthony, Desbiens Jocelyn, Gupta Sunil, Dodhia Rahul, Bhatraju Pavan K, Liles W Conrad, Kalpathy-Cramer Jayashree, Lee Aaron Y, Lavista Ferres Juan M
2022
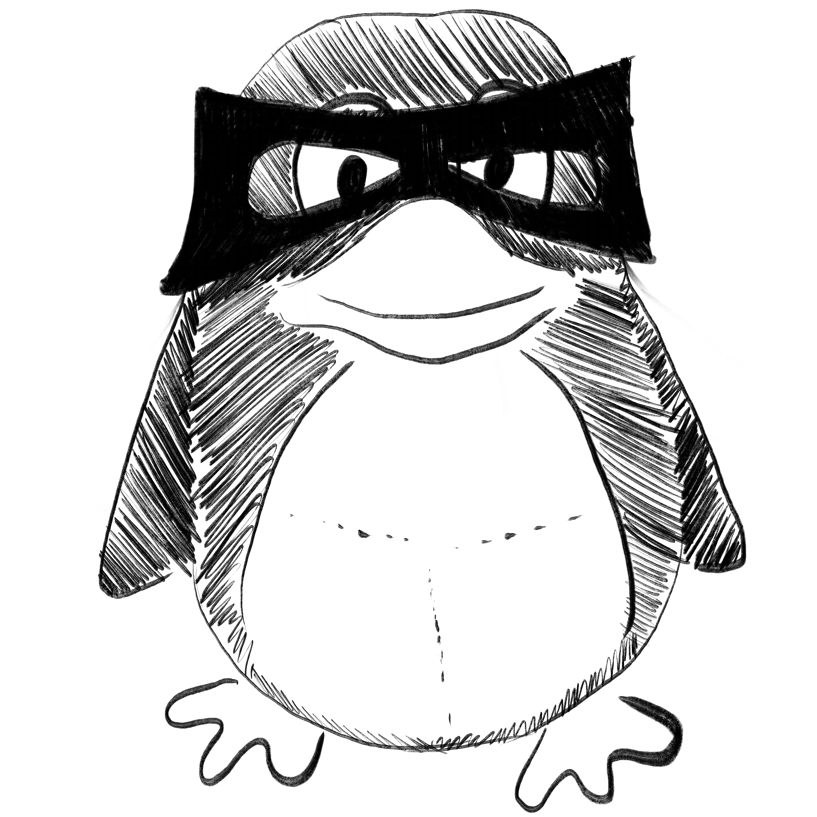
Neural-Symbolic Ensemble Learning for early-stage prediction of critical state of Covid-19 patients.
In Medical & biological engineering & computing ; h5-index 32.0
Fadja Arnaud Nguembang, Fraccaroli Michele, Bizzarri Alice, Mazzuchelli Giulia, Lamma Evelina
2022-Oct-06
Covid-19, Decision Trees, Deep Learning, Hierarchical Probabilistic Logic Program, Severity
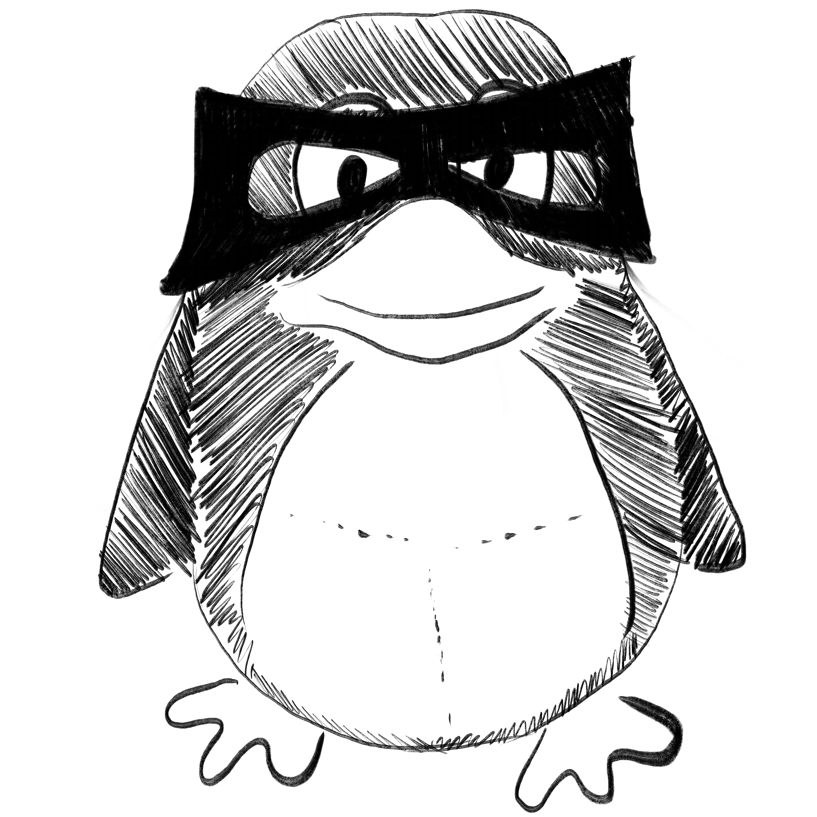
Monkeypox Virus Detection Using Pre-trained Deep Learning-based Approaches.
In Journal of medical systems ; h5-index 48.0
Sitaula Chiranjibi, Shahi Tej Bahadur
2022-Oct-06
Classification, Deep learning, Detection, Monkeypox, Pandemic, SARS-Cov2
Exploration of the Potential Link, Hub Genes, and Potential Drugs for Coronavirus Disease 2019 and Lung Cancer Based on Bioinformatics Analysis.
In Journal of oncology
Wang Ye, Li Qing, Zhang Jianfang, Xie Hui
2022
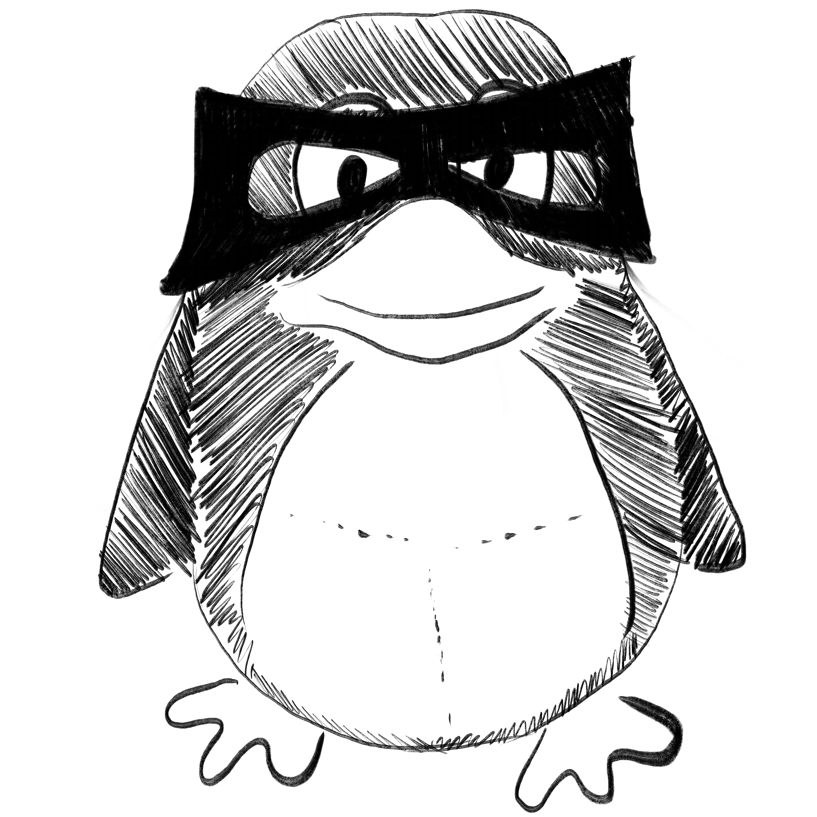
An integrated and automated testing approach on Inception Restnet-V3 based on convolutional neural network for leukocytes image classification.
In Biomedizinische Technik. Biomedical engineering
OBJECTIVES :
METHODS :
RESULTS :
CONCLUSIONS :
Palanivel Silambarasi, Nallasamy Viswanathan
2022-Oct-05
deep learning, image classification, inception V3, leukocyte, residual network
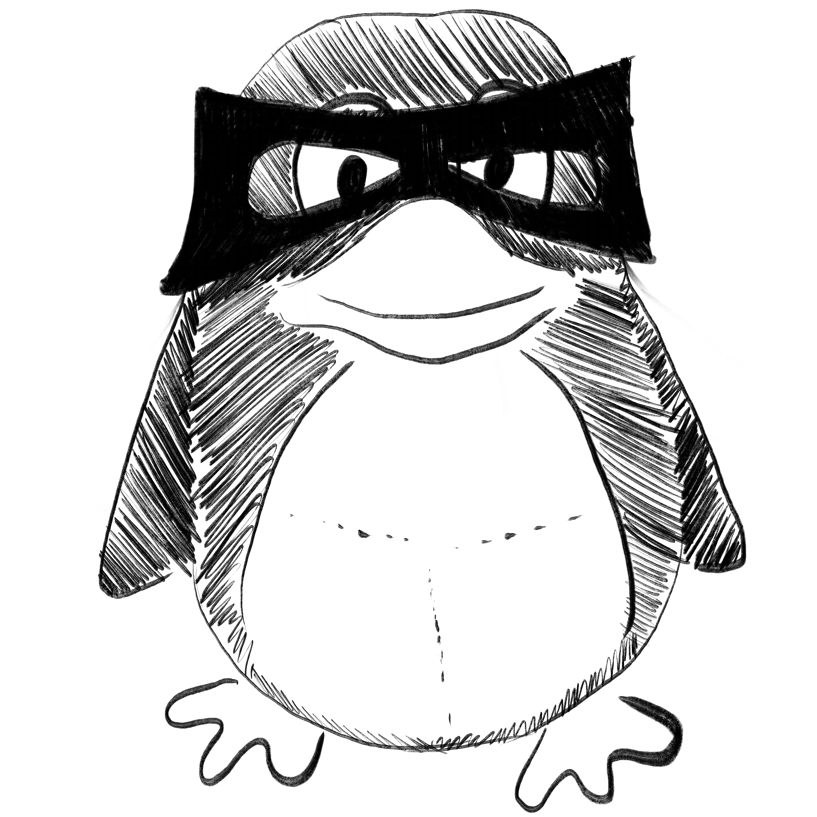
Enhancing the feasibility of cognitive load recognition in remote learning using physiological measures and an adaptive feature recalibration convolutional neural network.
In Medical & biological engineering & computing ; h5-index 32.0
Wu Chennan, Liu Yang, Guo Xiang, Zhu Tianshui, Bao Zongliang
2022-Oct-05
Cognitive load, Deep learning, EEG, Eye-tracking, Multimodal, Remote learning
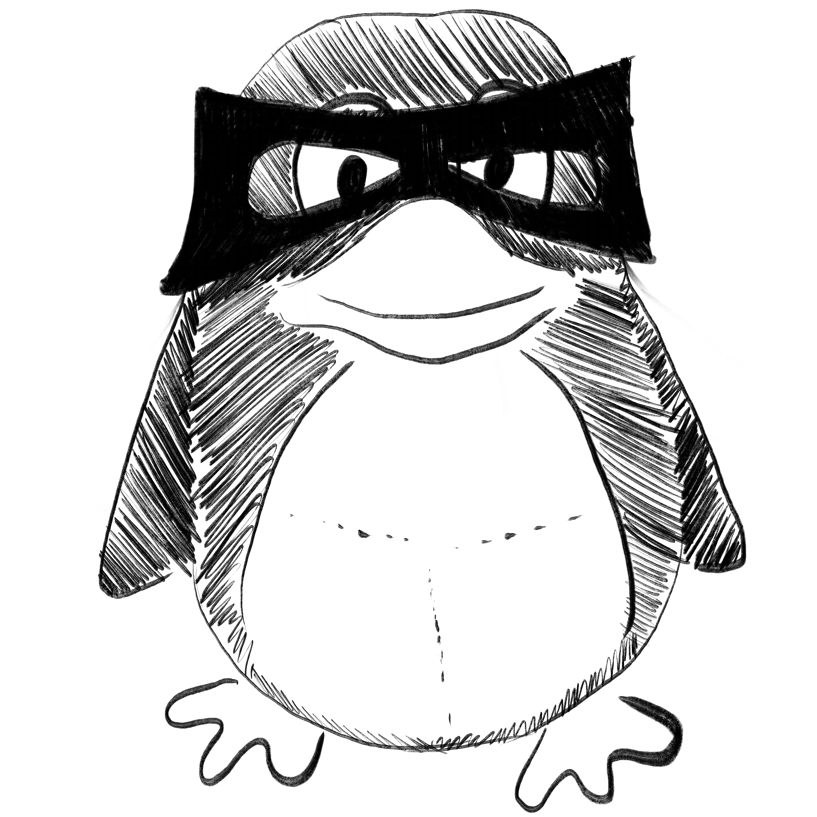
Face mask detection and social distance monitoring system for COVID-19 pandemic.
In Multimedia tools and applications
Javed Iram, Butt Muhammad Atif, Khalid Samina, Shehryar Tehmina, Amin Rashid, Syed Adeel Muzaffar, Sadiq Marium
2022-Sep-30
Coronavirus, Face mask detection, Single and multi-stage detectors, Social distance measurement
Using machine learning in prediction of ICU admission, mortality, and length of stay in the early stage of admission of COVID-19 patients.
In Annals of operations research
Saadatmand Sara, Salimifard Khodakaram, Mohammadi Reza, Kuiper Alex, Marzban Maryam, Farhadi Akram
2022-Sep-29
COVID-19 pandemic, Ensemble modeling, ML in health systems, Supervised learning
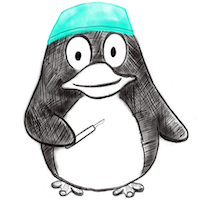
SARS-CoV-2: Has artificial intelligence stood the test of time.
In Chinese medical journal
Sajid Mir Ibrahim, Ahmed Shaheer, Waqar Usama, Tariq Javeria, Chundrigarh Mohsin, Balouch Samira Shabbir, Abaidullah Sajid
2022-Aug-05
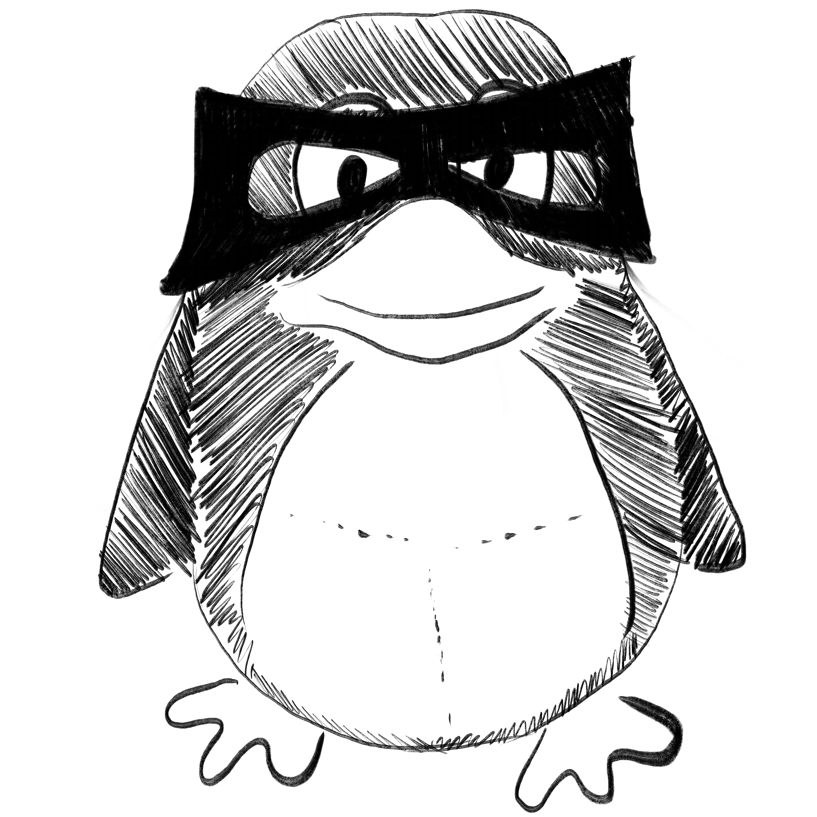
Analyzing historical diagnosis code data from NIH N3C and RECOVER Programs using deep learning to determine risk factors for Long Covid
ArXiv Preprint
Saurav Sengupta, Johanna Loomba, Suchetha Sharma, Donald E. Brown, Lorna Thorpe, Melissa A Haendel, Christopher G Chute, Stephanie Hong
2022-10-05
Artificial intelligence-based model for COVID-19 prognosis incorporating chest radiographs and clinical data; a retrospective model development and validation study.
In The British journal of radiology
OBJECTIVES :
METHODS :
RESULTS :
CONCLUSIONS :
ADVANCES IN KNOWLEDGE :
Walston Shannon L, Matsumoto Toshimasa, Miki Yukio, Ueda Daiju
2022-Oct-04
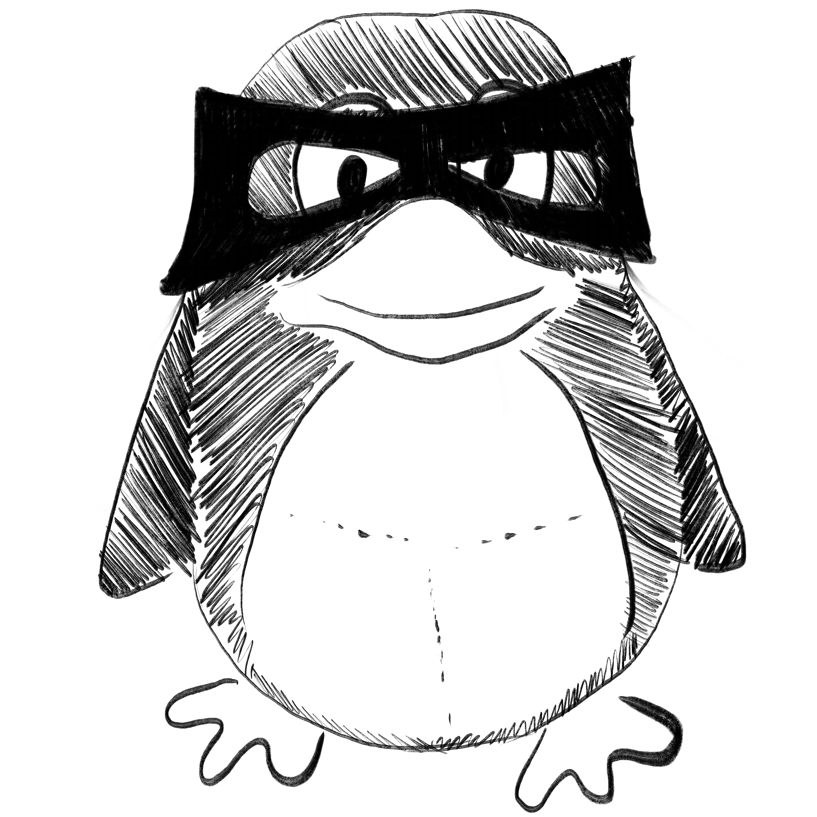
HeartSpot: Privatized and Explainable Data Compression for Cardiomegaly Detection
ArXiv Preprint
Elvin Johnson, Shreshta Mohan, Alex Gaudio, Asim Smailagic, Christos Faloutsos, Aurélio Campilho
2022-10-05
Data Exploration and Classification of News Article Reliability: Deep Learning Study.
In JMIR infodemiology
Background :
Objective :
Methods :
Results :
Conclusions :
Zhan Kevin, Li Yutong, Osmani Rafay, Wang Xiaoyu, Cao Bo
COVID-19, deep learning, ensemble model, false information, infodemic, news article reliability
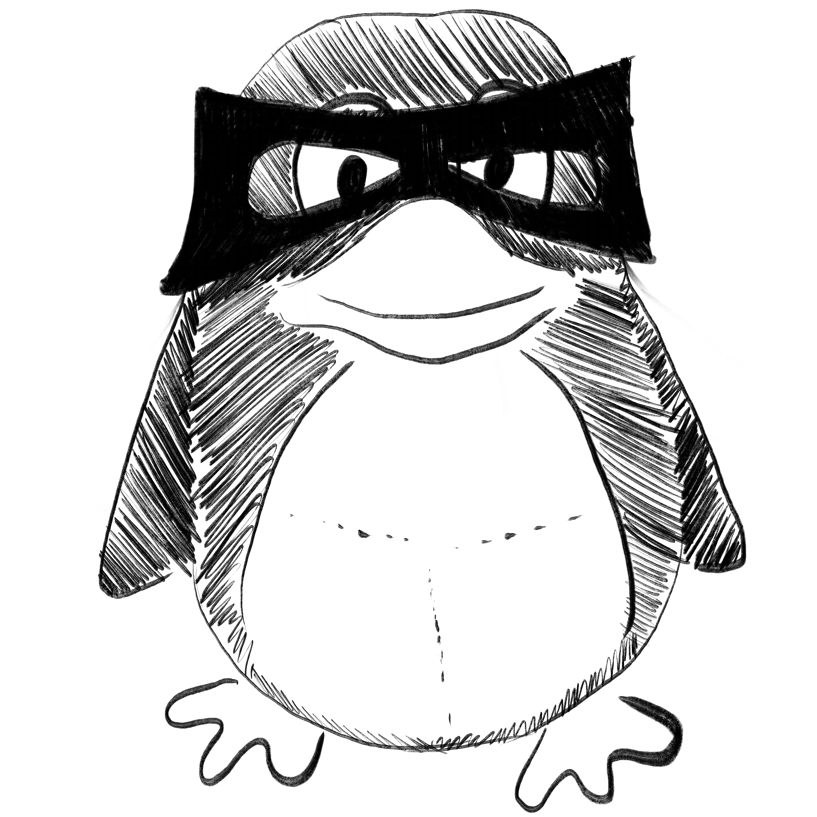
Learning to Act: Novel Integration of Algorithms and Models for Epidemic Preparedness
ArXiv Preprint
Sekou L. Remy, Oliver E. Bent
2022-10-05
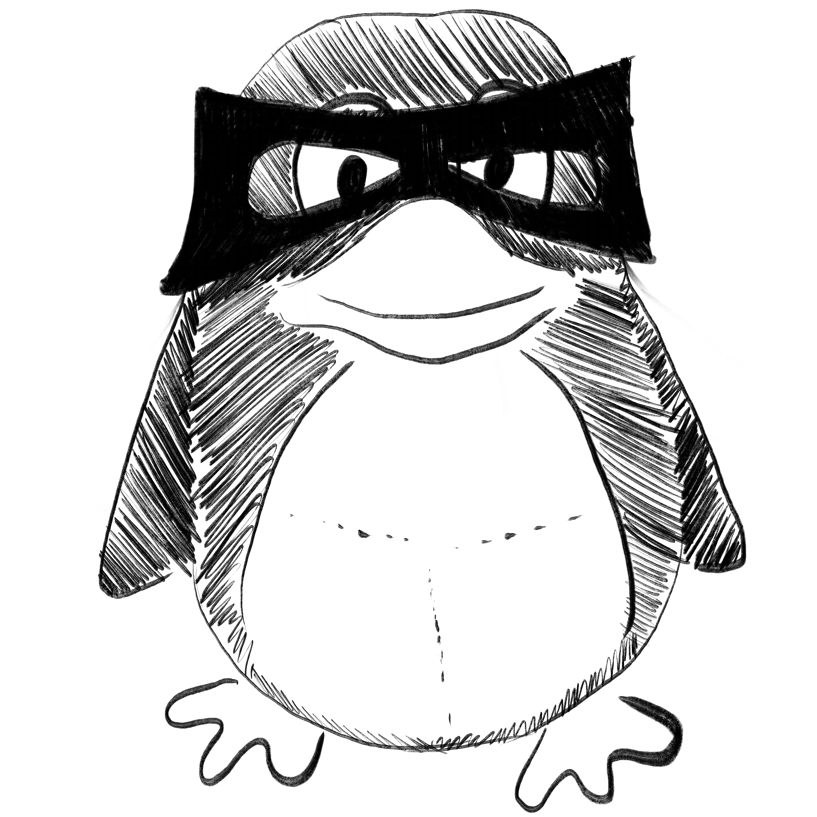
Real-Time Social Distance Measurement and Face Masks Detection in Public Transportation Systems during the COVID-19 Pandemic and Post-pandemic Era: Theoretical Approach and Case Study in Italy.
In Transportation research interdisciplinary perspectives
Guerrieri Marco, Parla Giuseppe
2022-Sep-28
Covid-19, Deep learning, Pandemic and post-pandemic era, Social distancing, YOLOv3, face mask detection
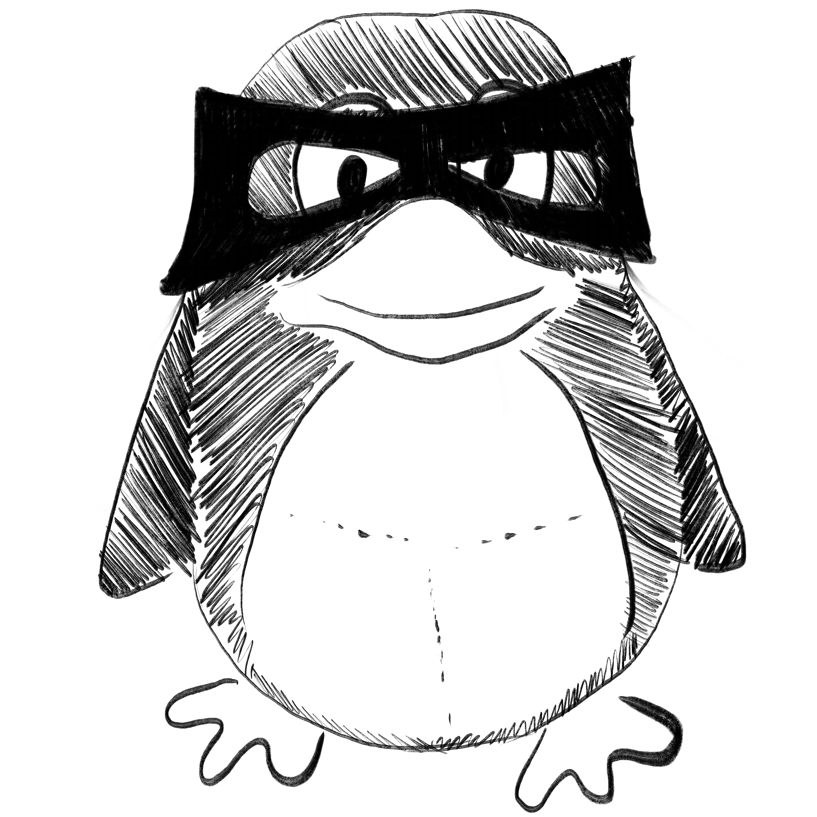
COVID-19 Identification in Chest X-Ray Images Using Intelligent Multi-Level Classification Scenario.
In Computers & electrical engineering : an international journal
Babukarthik R G, Chandramohan D, Tripathi Diwakar, Kumar Manish, Sambasivam G
2022-Sep-26
COVID-19, Genetic Algorithm, Genetic Deep Learning Convolutional Neural Network, Huddle Particle Swarm, Optimization, Pneumonia
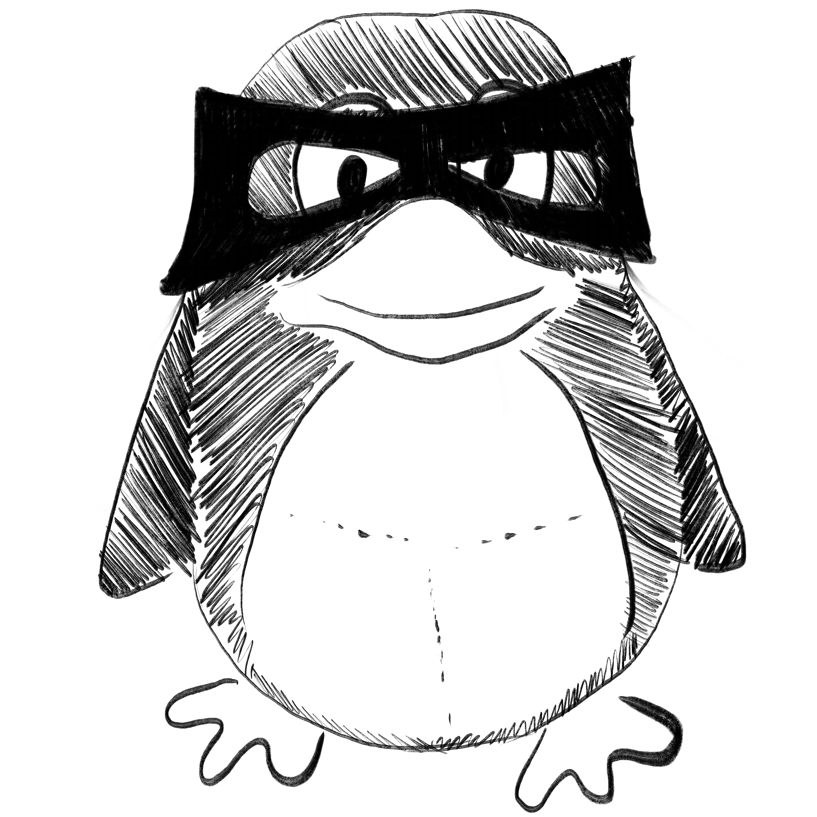
Towards edge devices implementation: deep learning model with visualization for COVID-19 prediction from chest X-ray.
In Advances in computational intelligence
Koh Shaline Jia Thean, Nafea Marwan, Nugroho Hermawan
2022
Chest x-ray, Deep learning, Edge computing, Visualization
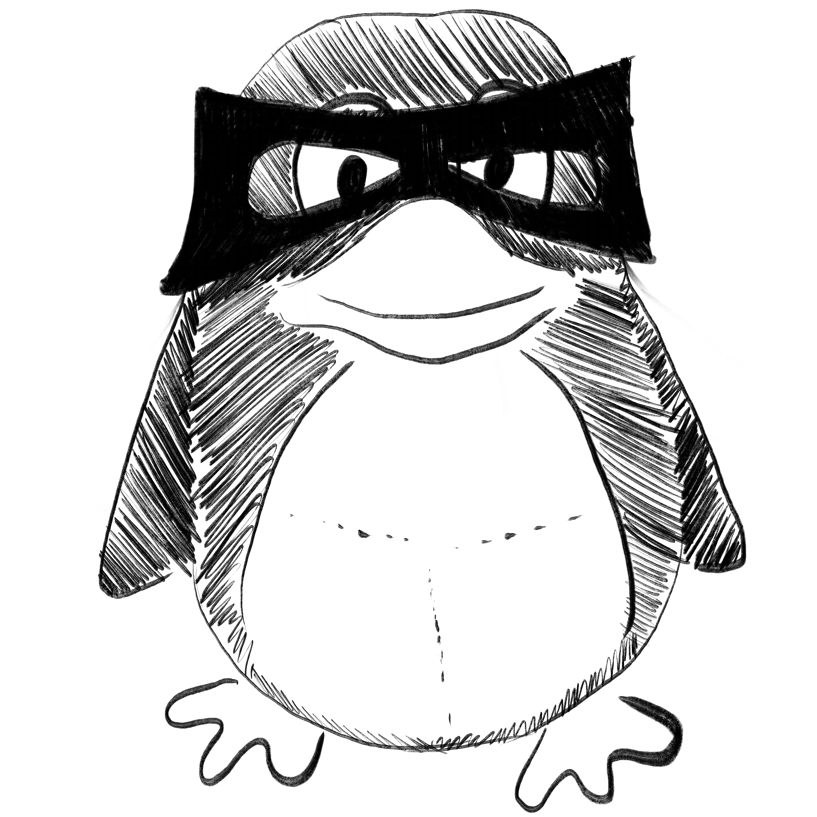
Predicting the sentiment of South Korean Twitter users toward vaccination after the emergence of COVID-19 Omicron variant using deep learning-based natural language processing.
In Frontiers in medicine
Eom Gayeong, Yun Sanghyun, Byeon Haewon
2022
BERT, COVID-19 Omicron variant, NLP, deep learning, sentiment analysis
Augmentation of literature review of COVID-19 radiology.
In World journal of radiology
Merchant Suleman Adam, Nadkarni Prakash, Shaikh Mohd Javed Saifullah
2022-Sep-28
Artificial intelligence in COVID-19, COVID-19 imaging, COVID-19 radiological findings, COVID-19-associated coagulopathy, Cardiac magnetic resonance imaging, Chest radiographs, Computed tomography, Hamptons hump, Westermark sign
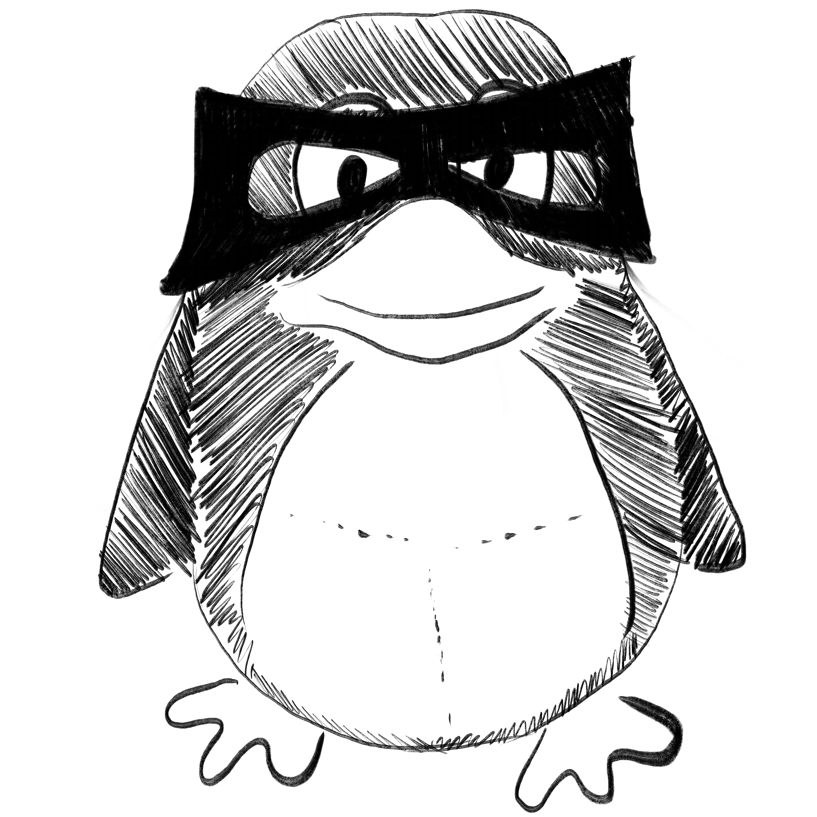
Forecasting adversities of COVID-19 waves in India using intelligent computing.
In Innovations in systems and software engineering
Chakraborty Arijit, Das Dipankar, Mitra Sajal, De Debashis, Pal Anindya J
2022-Sep-26
COVID-19, Extreme learning, MAPE, Machine learning, RMSE
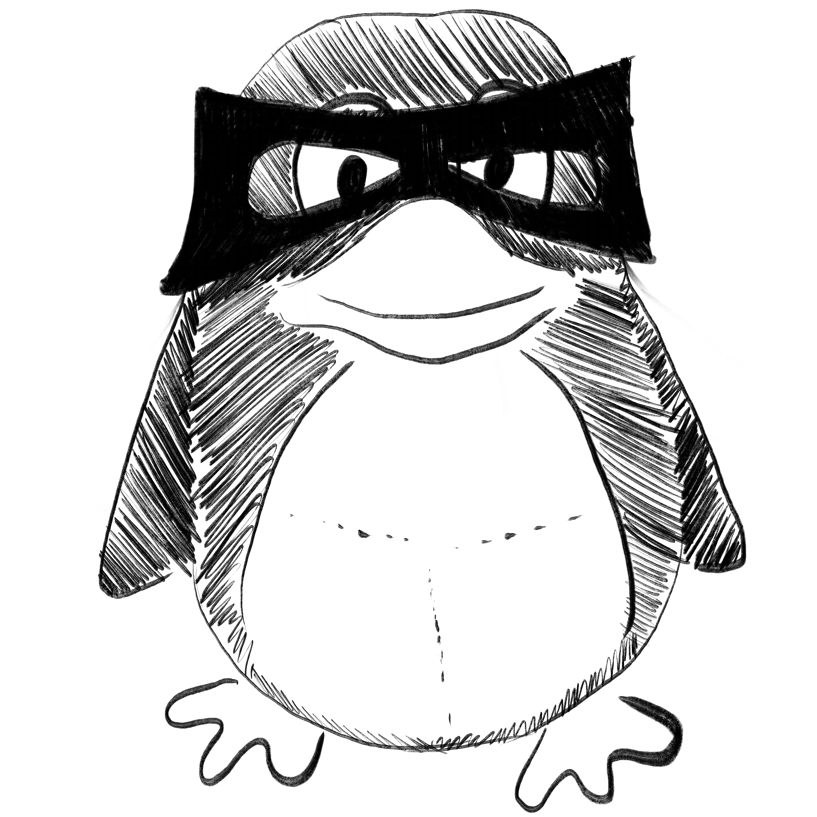
A robust defect detection method for syringe scale without positive samples.
In The Visual computer
Wang Xiaodong, Xu Xianwei, Wang Yanli, Wu Pengtao, Yan Fei, Zeng Zhiqiang
2022-Sep-27
Deep learning, Defect detection, Image processing, Image segmentation
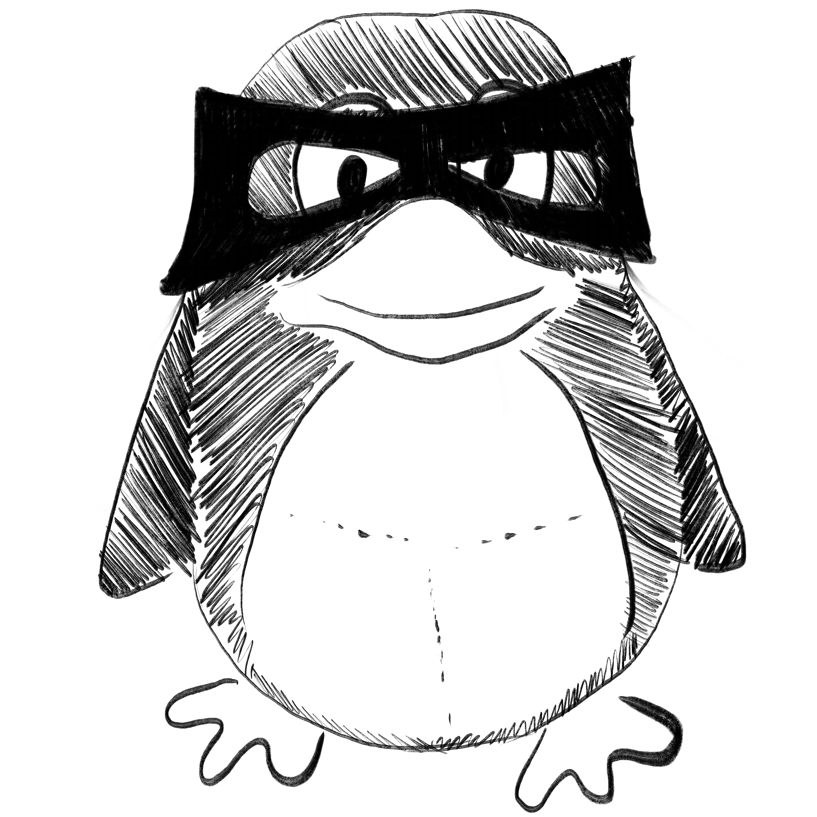
A COVID-19 X-ray image classification model based on an enhanced convolutional neural network and hill climbing algorithms.
In Multimedia tools and applications
Pradhan Ashwini Kumar, Mishra Debahuti, Das Kaberi, Obaidat Mohammad S, Kumar Manoj
2022-Sep-27
COVID 19, Hill climbing algorithms, Image classification, Tailored convolutional neural network, X-Ray images
Understanding the influence of online information, misinformation, disinformation and reinformation on COVID-19 vaccine acceptance: Protocol for a multicomponent study.
In JMIR research protocols ; h5-index 26.0
BACKGROUND :
OBJECTIVE :
METHODS :
RESULTS :
CONCLUSIONS :
INTERNATIONAL REGISTERED REPORT :
Dube Eve, MacDonald Shannon E, Manca Terra, Bettinger Julie A, Driedger S Michelle, Graham Janice, Greyson Devon, MacDonald Noni E, Meyer Samantha, Roch Geneviève, Vivion Maryline, Aylsworth Laura, Witteman Holly, Gélinas-Gascon Félix, Marques Sathler Guimaraes Lucas, Hakim Hina, Gagnon Dominique, Béchard Benoît, Gramaccia Julie A, Khoury Richard, Tremblay Sébastien
2022-Sep-08
Wavelet transformation can enhance computed tomography texture features: a multicenter radiomics study for grade assessment of COVID-19 pulmonary lesions.
In Quantitative imaging in medicine and surgery
Background :
Methods :
Results :
Conclusions :
Jiang Zekun, Yin Jin, Han Peilun, Chen Nan, Kang Qingbo, Qiu Yue, Li Yiyue, Lao Qicheng, Sun Miao, Yang Dan, Huang Shan, Qiu Jiajun, Li Kang
2022-Oct
COVID-19, computed tomography (CT), machine learning, quantitative image analysis, radiomics
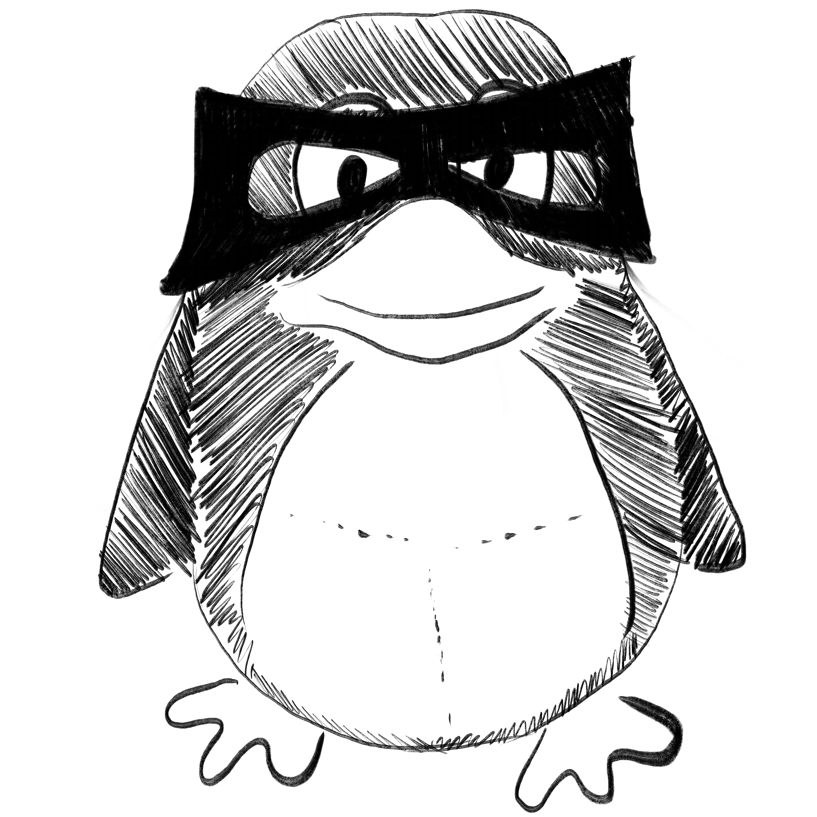
Automated Medical Device Display Reading Using Deep Learning Object Detection
ArXiv Preprint
Lucas P. Moreira
2022-10-04
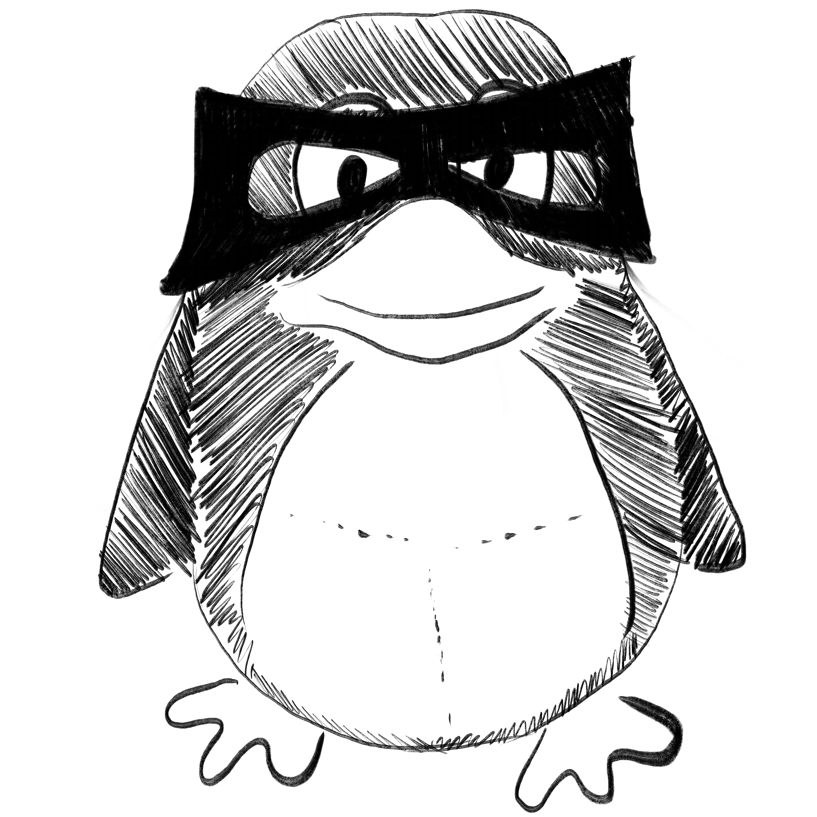
Algorithmic harms and digital ageism in the use of surveillance technologies in nursing homes.
In Frontiers in sociology
Berridge Clara, Grigorovich Alisa
2022
artificial intelligence, big data, dementia, long-term care, machine learning, older adults, privacy, technology
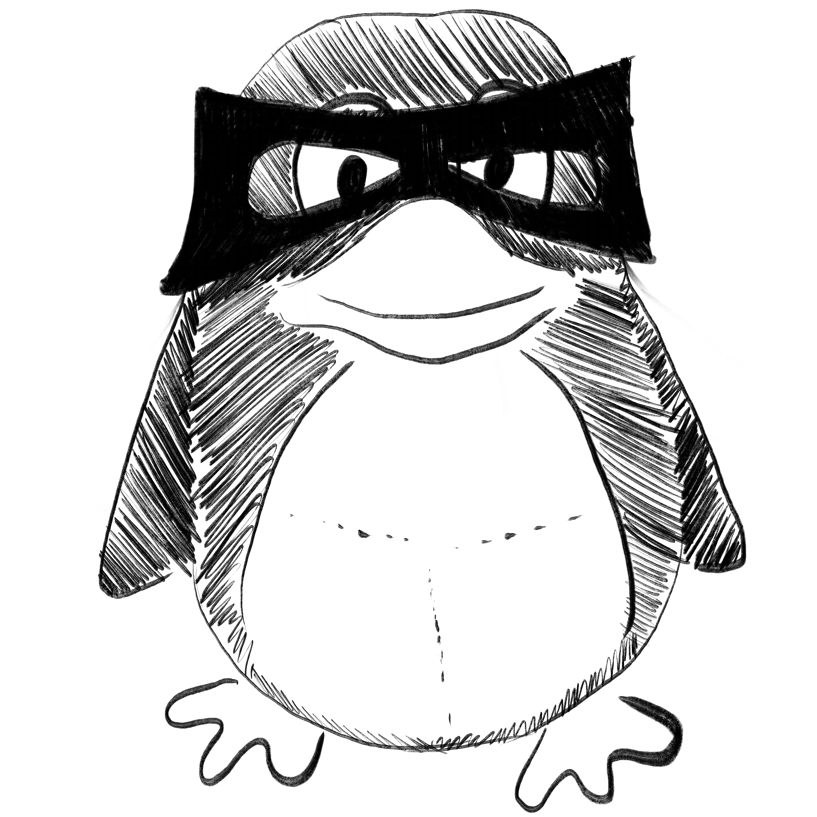
Artificial Intelligence Techniques to Predict the Airway Disorders Illness: A Systematic Review.
In Archives of computational methods in engineering : state of the art reviews
Koul Apeksha, Bawa Rajesh K, Kumar Yogesh
2022-Sep-28
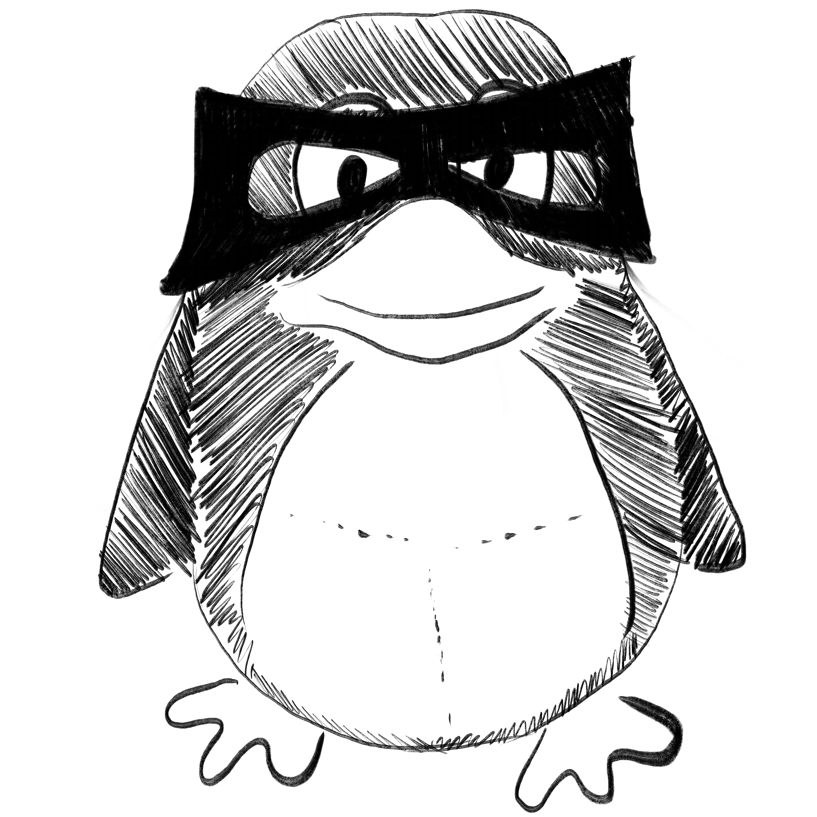
SEMA: Antigen B-cell conformational epitope prediction using deep transfer learning.
In Frontiers in immunology ; h5-index 100.0
Shashkova Tatiana I, Umerenkov Dmitriy, Salnikov Mikhail, Strashnov Pavel V, Konstantinova Alina V, Lebed Ivan, Shcherbinin Dmitriy N, Asatryan Marina N, Kardymon Olga L, Ivanisenko Nikita V
2022
GVP, antibody - antigen complex, conformational B-cell epitopes, epitopes, protein language model, transfer learning, transformer
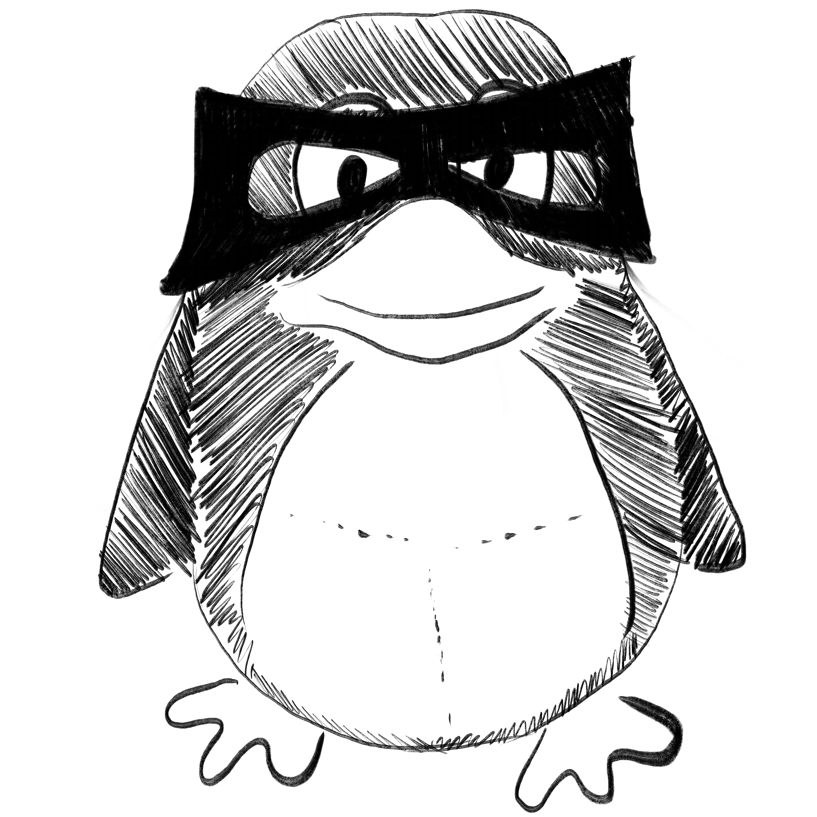
Automatic Detection of Cases of COVID-19 Pneumonia from Chest X-ray Images and Deep Learning Approaches.
In Computational intelligence and neuroscience
Hajjej Fahima, Ayouni Sarra, Hasan Malek, Abir Tanvir
2022
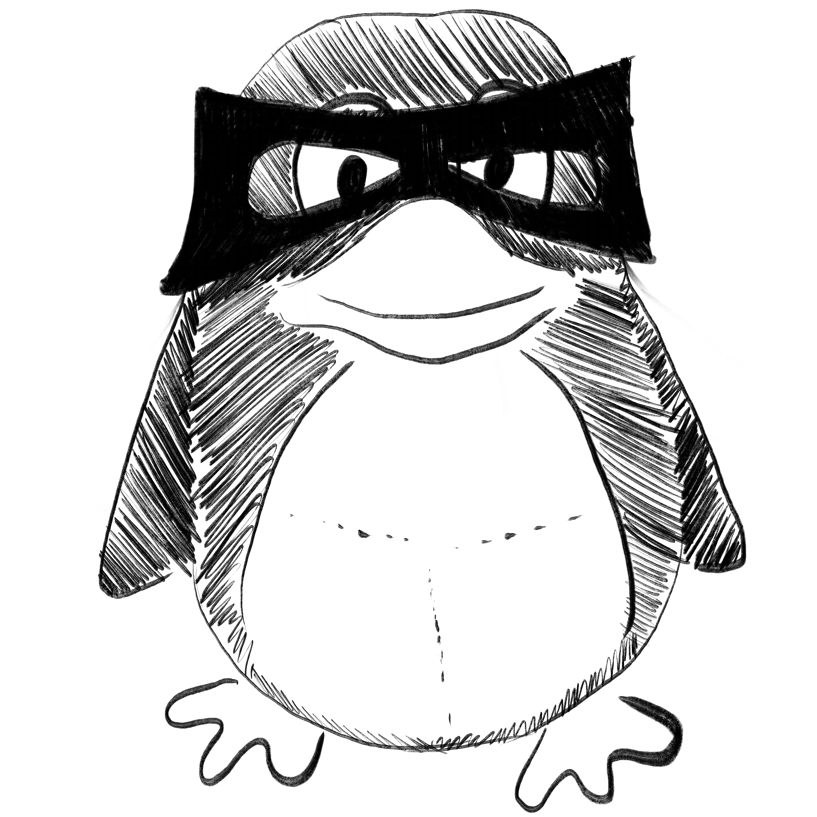
Detecting time-evolving phenotypic components of adverse reactions against BNT162b2 mRNA SARS-CoV-2 vaccine via non-negative tensor factorization.
In iScience
Ikeda Kei, Nakada Taka-Aki, Kageyama Takahiro, Tanaka Shigeru, Yoshida Naoki, Ishikawa Tetsuo, Goshima Yuki, Otaki Natsuko, Iwami Shingo, Shimamura Teppei, Taniguchi Toshibumi, Igari Hidetoshi, Hanaoka Hideki, Yokote Koutaro, Tsuyuzaki Koki, Nakajima Hiroshi, Kawakami Eiryo
2022-Sep-28
Adverse reaction, COVID19, Computational phenotyping, Tensor factorization, mRNA vaccine
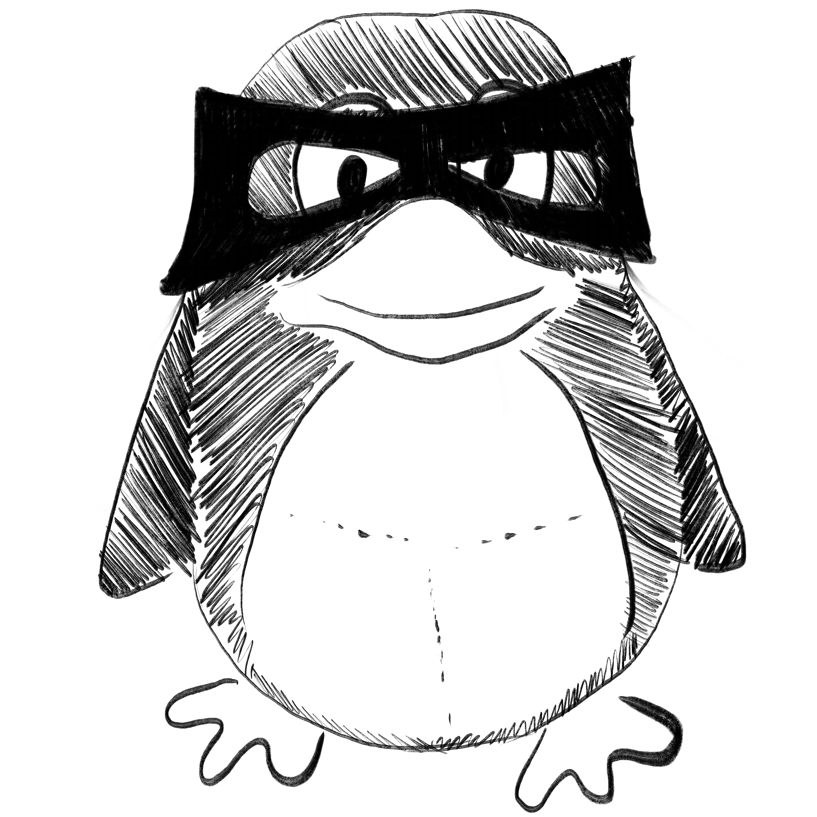
A teacher-student framework with Fourier Transform augmentation for COVID-19 infection segmentation in CT images.
In Biomedical signal processing and control
Chen Han, Jiang Yifan, Ko Hanseok, Loew Murray
2023-Jan
COVID-19, Computed tomography, Fourier Transform, Infection segmentation, Teacher–student network
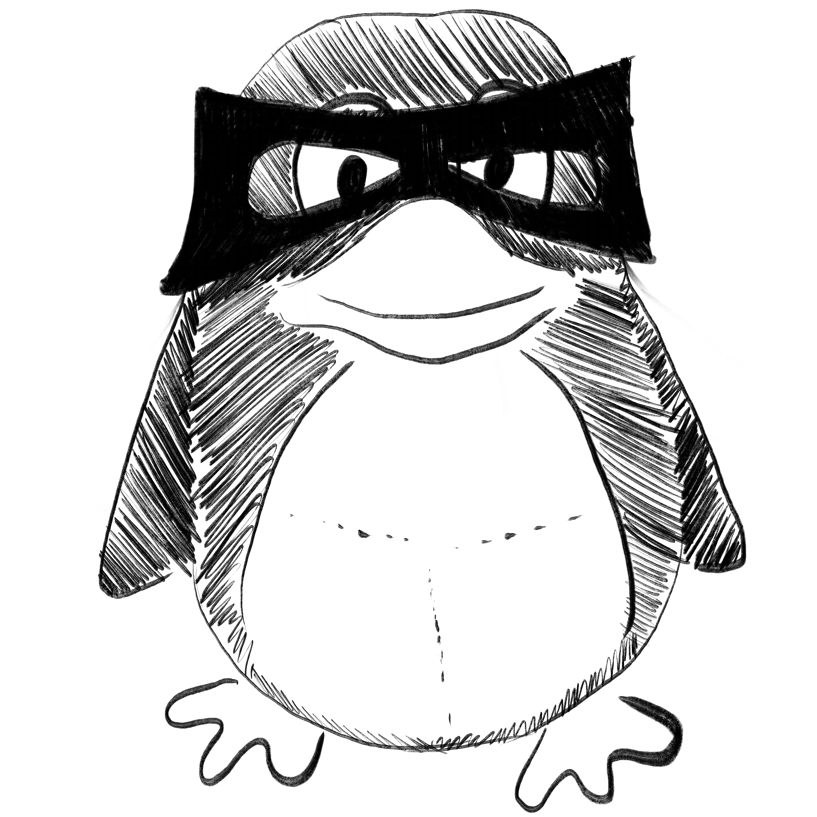
The role of diversity and ensemble learning in credit card fraud detection.
In Advances in data analysis and classification
Paldino Gian Marco, Lebichot Bertrand, Le Borgne Yann-Aël, Siblini Wissam, Oblé Frédéric, Boracchi Giacomo, Bontempi Gianluca
2022-Sep-28
Concept drift, Diversity, Ensemble learning, Finance, Fraud detection
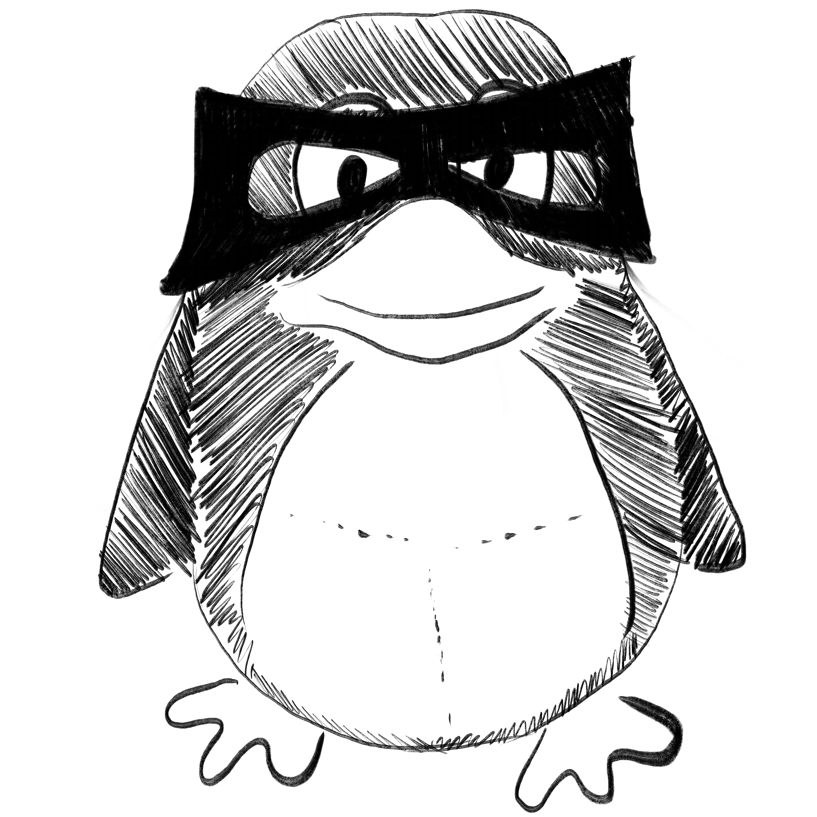
Customer satisfaction with Restaurants Service Quality during COVID-19 outbreak: A two-stage methodology.
In Technology in society
Zibarzani Masoumeh, Abumalloh Rabab Ali, Nilashi Mehrbakhsh, Samad Sarminah, Alghamdi O A, Nayer Fatima Khan, Ismail Muhammed Yousoof, Mohd Saidatulakmal, Mohammed Akib Noor Adelyna
2022-Aug
Customer satisfaction, Machine learning, Segmentation, Social data analysis, Text mining
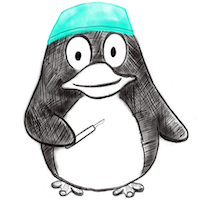
Research progress and hotspot of the artificial intelligence application in the ultrasound during 2011-2021: A bibliometric analysis.
In Frontiers in public health
Xia Demeng, Chen Gaoqi, Wu Kaiwen, Yu Mengxin, Zhang Zhentao, Lu Yixian, Xu Lisha, Wang Yin
2022
CNN, COVID-19, artificial intelligence, bibliometrics, ultrasound
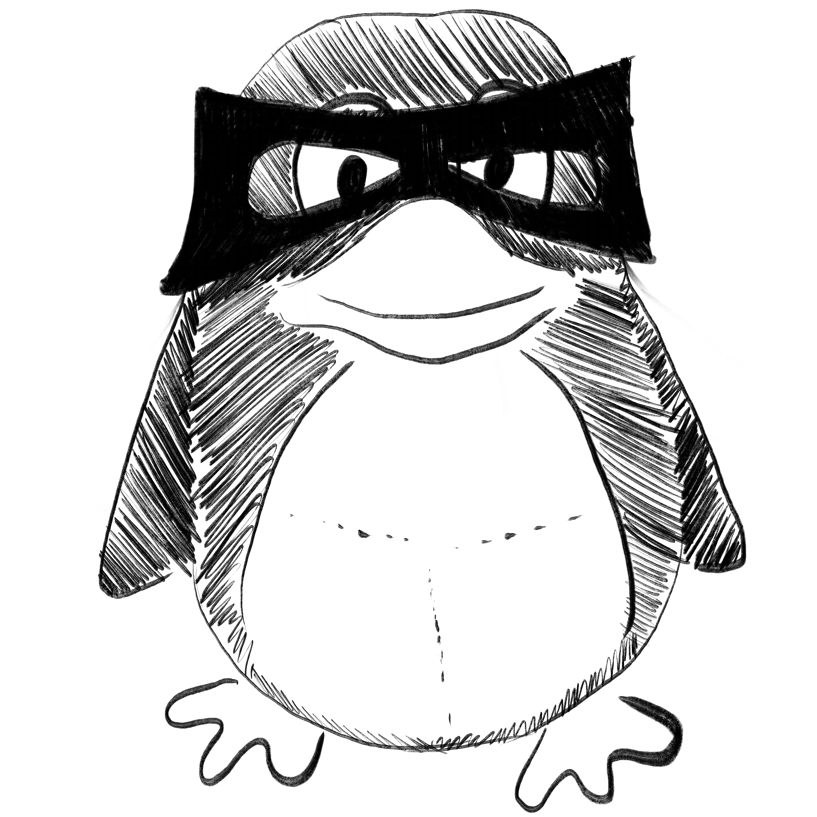
The impact factors of social media users' forwarding behavior of COVID-19 vaccine topic: Based on empirical analysis of Chinese Weibo users.
In Frontiers in public health
Introduction :
Methods :
Results :
Conclusion :
Sun Kun, Wang Han, Zhang Jinsheng
2022
COVID-19 vaccine, emotion, forwarding behavior, social media, social network structure
Characteristics and outcomes of SARS-COV 2 critically ill patients after emergence of the variant of concern 20H/501Y.V2: A comparative cohort study.
In Medicine
Aries Philippe, Huet Olivier, Balicchi Julien, Mathais Quentin, Estagnasie Camille, Martin-Lecamp Gonzague, Simon Olivier, Morvan Anne-Cécile, Puech Bérénice, Subiros Marion, Blonde Renaud, Boue Yvonnick
2022-Sep-30
Baseline host determinants of robust human HIV-1 vaccine-induced immune responses: A meta-analysis of 26 vaccine regimens.
In EBioMedicine
BACKGROUND :
METHODS :
FINDINGS :
INTERPRETATION :
FUNDING :
Huang Yunda, Zhang Yuanyuan, Seaton Kelly E, De Rosa Stephen, Heptinstall Jack, Carpp Lindsay N, Randhawa April Kaur, McKinnon Lyle R, McLaren Paul, Viegas Edna, Gray Glenda E, Churchyard Gavin, Buchbinder Susan P, Edupuganti Srilatha, Bekker Linda-Gail, Keefer Michael C, Hosseinipour Mina C, Goepfert Paul A, Cohen Kristen W, Williamson Brian D, McElrath M Juliana, Tomaras Georgia D, Thakar Juilee, Kobie James J
2022-Sep-27
Antibody, Baseline characteristics, CD4+ T cell, SuperLearner, Vaccine response heterogeneity, Variable importance measurements
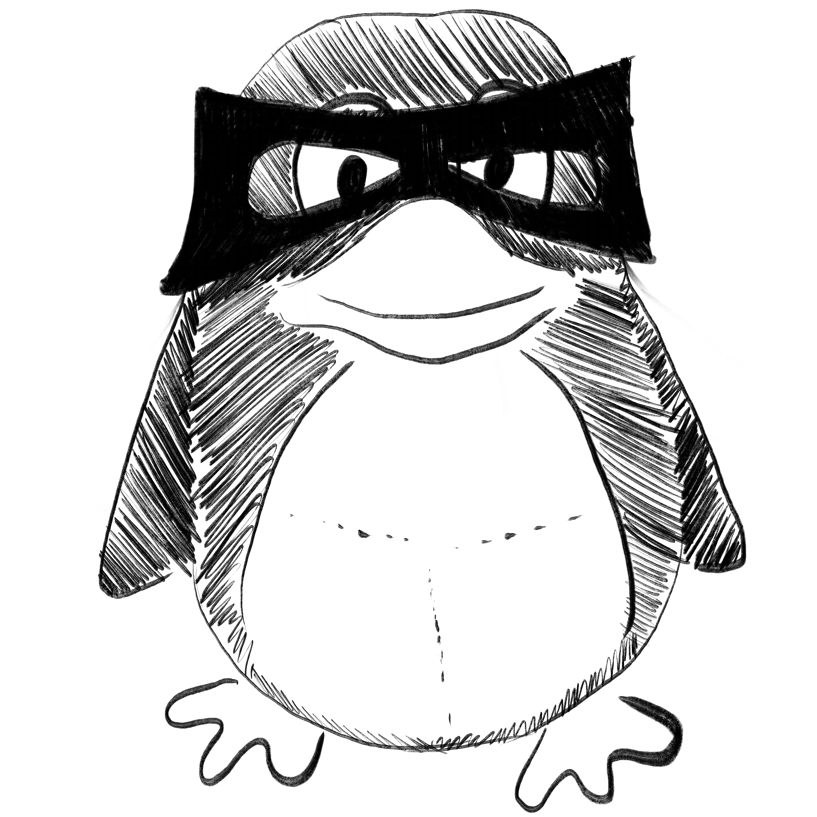
Rethinking adversarial domain adaptation: Orthogonal decomposition for unsupervised domain adaptation in medical image segmentation.
In Medical image analysis
Sun Yongheng, Dai Duwei, Xu Songhua
2022-Sep-21
Medical image segmentation, Orthogonal decomposition, Unsupervised domain adaptation
Reproduction numbers of SARS-CoV-2 Omicron subvariants.
In Journal of travel medicine
Wang Shuqi, Zhang Fengdi, Wang Zhen, Du Zhanwei, Gao Chao
2022-Sep-30
Quantifying Mutational Response to Track the Evolution of SARS-CoV-2 Spike Variants: Introducing a Statistical-Mechanics-Guided Machine Learning Method.
In The journal of physical chemistry. B
Sangeet Satyam, Sarkar Raju, Mohanty Saswat K, Roy Susmita
2022-Sep-30
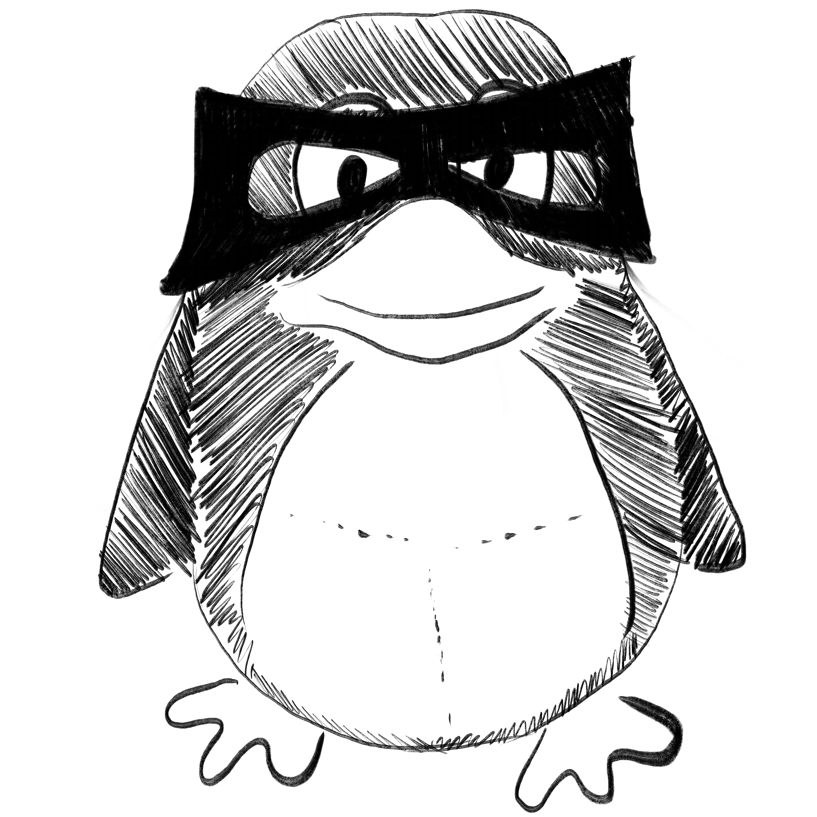
Development of a deep learning-based quantitative structure-activity relationship model to identify potential inhibitors against the 3C-like protease of SARS-CoV-2.
In Future medicinal chemistry ; h5-index 38.0
Kumari Madhulata, Subbarao Naidu
2022-Sep-30
3CLpro, COVID-19, QSAR, SARS-CoV, SARS-CoV-2, convolutional neural network, deep learning, dynamic cross-correlation matrices, free energy landscape, principal component analysis
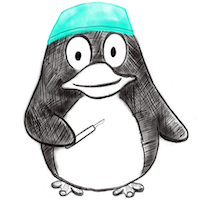
AI in Health science: A Perspective.
In Current pharmaceutical biotechnology
Mishra Raghav, Chaudhary Kajal, Mishra Isha
2022-Sep-29
Applications, Artificial Intelligence, COVID-19, Cancer, Deep Learning, Healthcare, Machine Learning
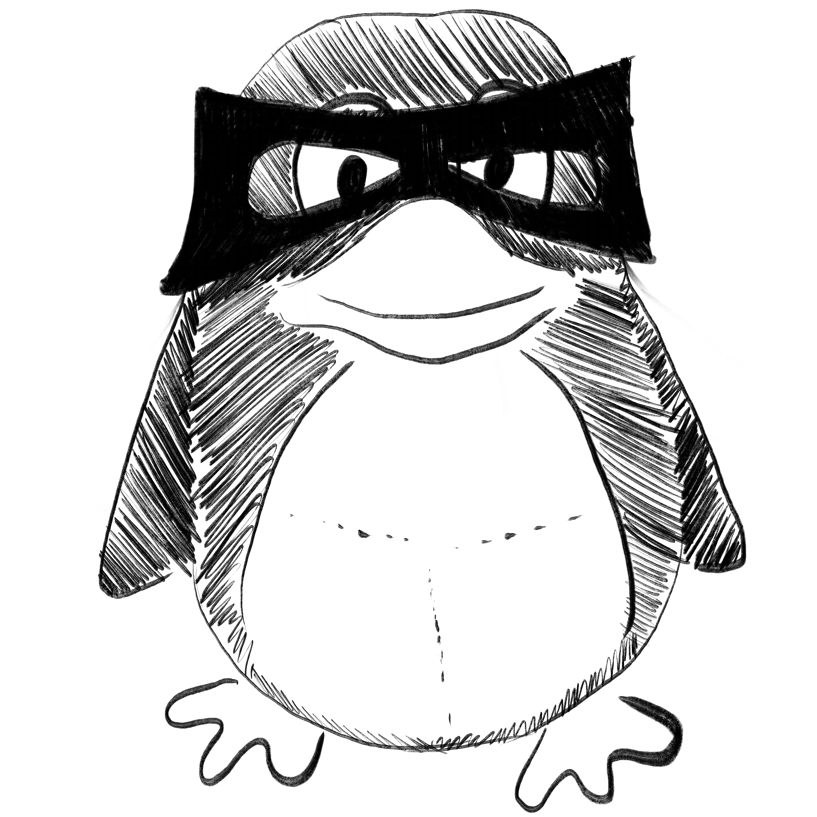
COVID-19 Semantic Pneumonia Segmentation and Classification Using Artificial Intelligence.
In Contrast media & molecular imaging
Abdulaal Mohammed J, Mehedi Ibrahim M, Abusorrah Abdullah M, Aljohani Abdulah Jeza, Milyani Ahmad H, Rana Md Masud, Mahmoud Mohamed
2022
Weekly Summary
Receive a weekly summary and discussion of the top papers of the week by leading researchers in the field.