Receive a weekly summary and discussion of the top papers of the week by leading researchers in the field.
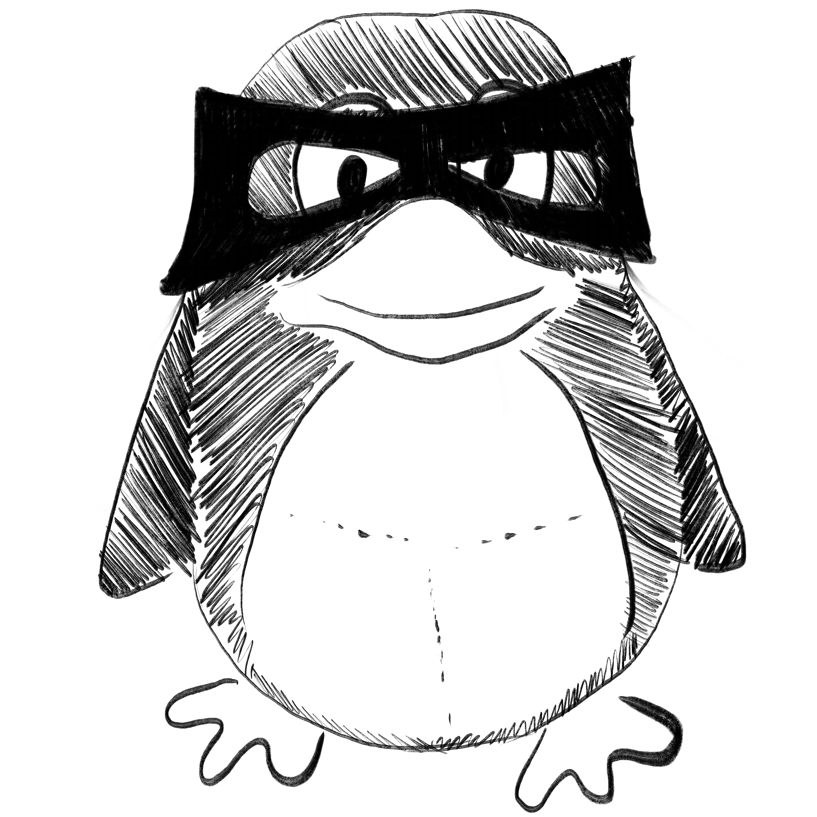
An experimental study of animating-based facial image manipulation in online class environments.
In Scientific reports ; h5-index 158.0
Park Jeong-Ha, Lim Chae-Yun, Kwon Hyuk-Yoon
2023-Mar-22
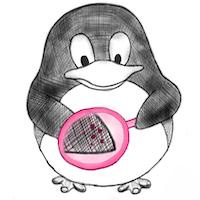
Role of the Microbiome in Immunotherapy of Melanoma.
In Cancer journal (Sudbury, Mass.)
Jiminez Victoria, Yusuf Nabiha
Transformer Performance for Chemical Reactions: Analysis of Different Predictive and Evaluation Scenarios.
In Journal of chemical information and modeling
Jaume-Santero Fernando, Bornet Alban, Valery Alain, Naderi Nona, Vicente Alvarez David, Proios Dimitrios, Yazdani Anthony, Bournez Colin, Fessard Thomas, Teodoro Douglas
2023-Mar-23
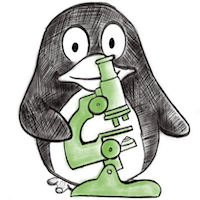
Learning to predict RNA sequence expressions from whole slide images with applications for search and classification.
In Communications biology
Alsaafin Areej, Safarpoor Amir, Sikaroudi Milad, Hipp Jason D, Tizhoosh H R
2023-Mar-22
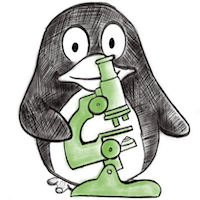
A systematic evaluation of deep learning methods for the prediction of drug synergy in cancer.
In PLoS computational biology
Baptista Delora, Ferreira Pedro G, Rocha Miguel
2023-Mar-23
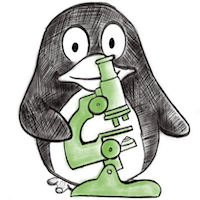
The value of artificial intelligence in the diagnosis of lung cancer: A systematic review and meta-analysis.
In PloS one ; h5-index 176.0
Liu Mingsi, Wu Jinghui, Wang Nian, Zhang Xianqin, Bai Yujiao, Guo Jinlin, Zhang Lin, Liu Shulin, Tao Ke
2023
Weekly Summary
Receive a weekly summary and discussion of the top papers of the week by leading researchers in the field.