Receive a weekly summary and discussion of the top papers of the week by leading researchers in the field.
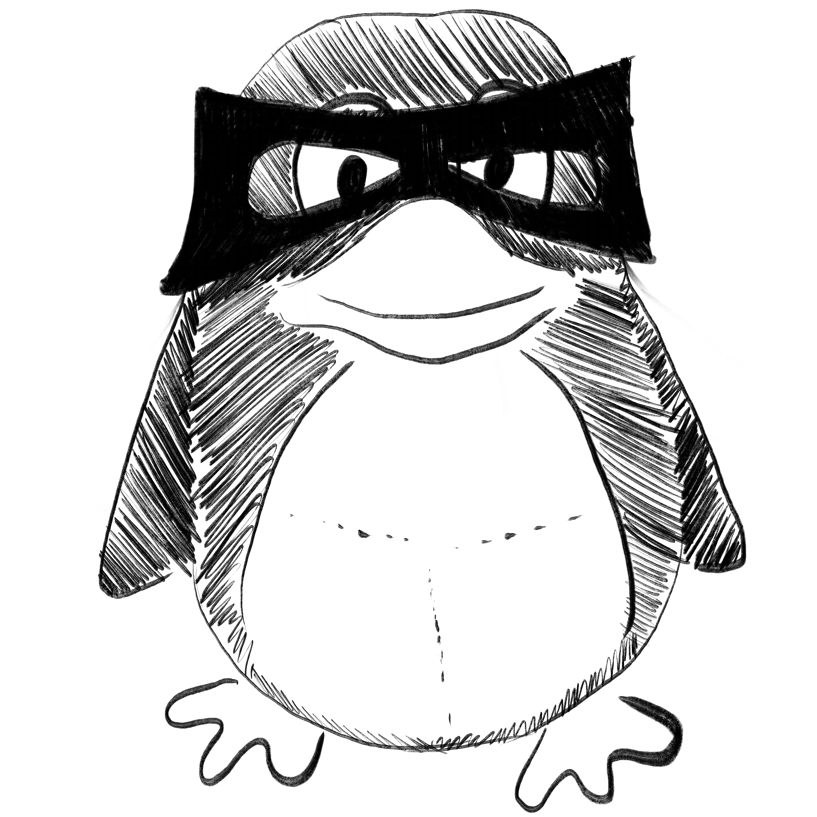
Modeling structure-activity relationships with machine learning to identify GSK3-targeted small molecules as potential COVID-19 therapeutics.
In Frontiers in endocrinology ; h5-index 55.0
Pirzada Rameez Hassan, Ahmad Bilal, Qayyum Naila, Choi Sangdun
2023
GSK3, QSAR, coronaviruses, machine learning, molecular descriptors
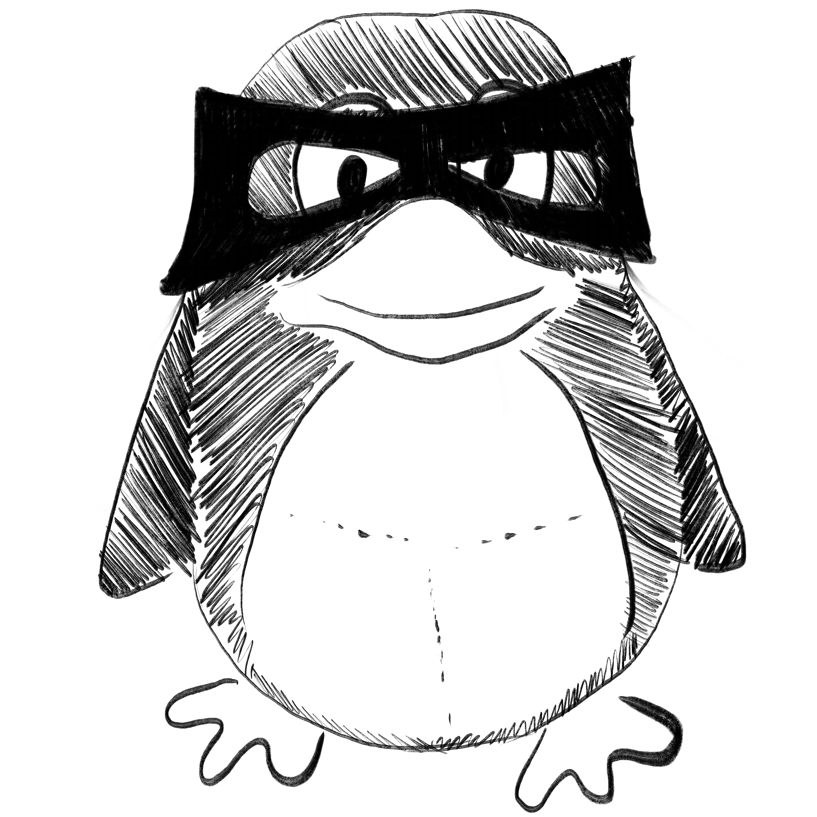
IP4GS: Bringing genomic selection analysis to breeders.
In Frontiers in plant science
Li Tong, Jiang Shan, Fu Ran, Wang Xiangfeng, Cheng Qian, Jiang Shuqin
2023
R shiny, bioinformatics, genomic selection, genotype-to-phenotype prediction, web-based platform
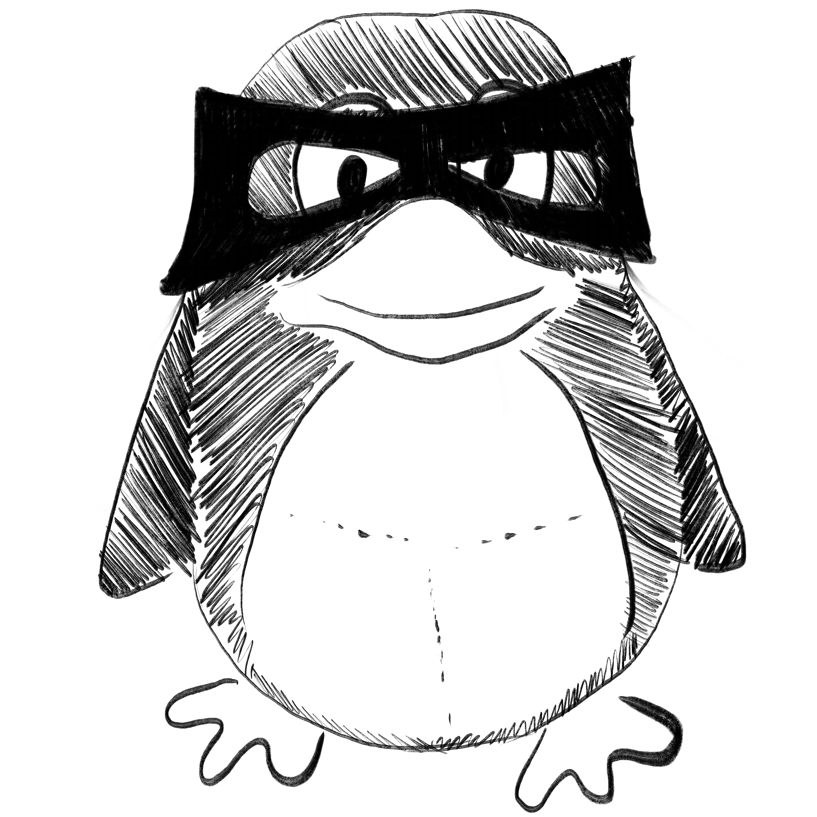
Assessing farmland suitability for agricultural machinery in land consolidation schemes in hilly terrain in China: A machine learning approach.
In Frontiers in plant science
Yang Heng, Ma Wenqiu, Liu Tongxin, Li Wenqing
2023
farmland consolidation suitable for agricultural machinery, hilly terrain, machine learning approach (MLA), potential of farmland productivity, suitability assessment, zoning
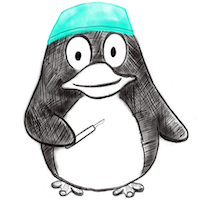
Assessment of shape-based features ability to predict the ascending aortic aneurysm growth.
In Frontiers in physiology
Geronzi Leonardo, Haigron Pascal, Martinez Antonio, Yan Kexin, Rochette Michel, Bel-Brunon Aline, Porterie Jean, Lin Siyu, Marin-Castrillon Diana Marcela, Lalande Alain, Bouchot Olivier, Daniel Morgan, Escrig Pierre, Tomasi Jacques, Valentini Pier Paolo, Biancolini Marco Evangelos
2023
aorta, ascending aorta aneurysm, biomechanical features, cardiovascular diseases, classification, growth rate, machine learning, risk assessment
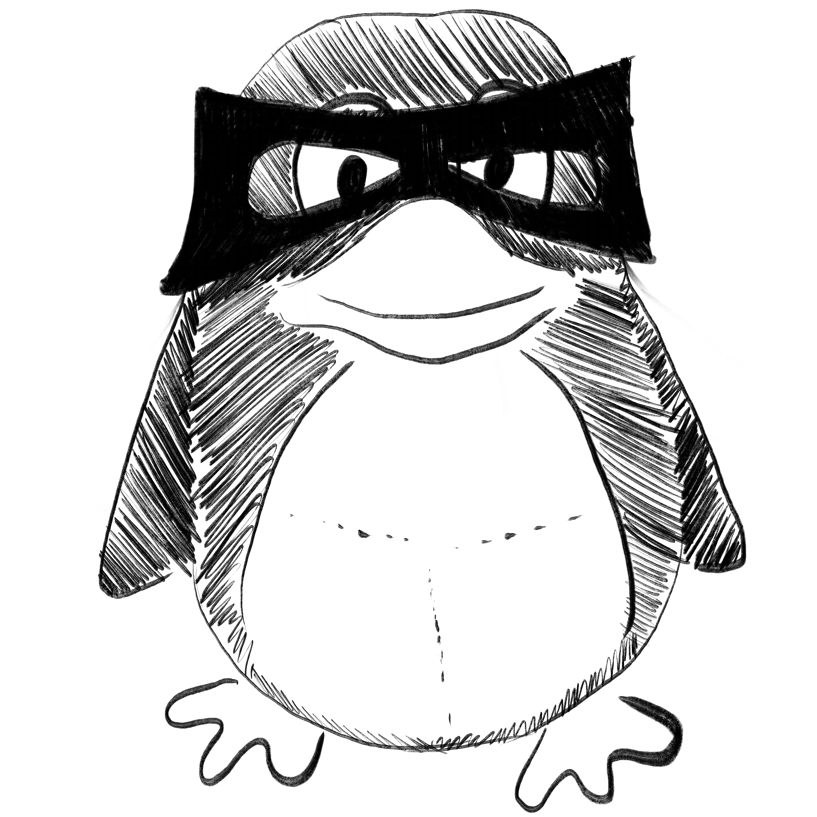
A lightweight fetal distress-assisted diagnosis model based on a cross-channel interactive attention mechanism.
In Frontiers in physiology
Deng Yanjun, Zhang Yefei, Zhou Zhixin, Zhang Xianfei, Jiao Pengfei, Zhao Zhidong
2023
attention mechanism, fetal distress, fetal heart rate, lightweight model, wavelet packet coefficient
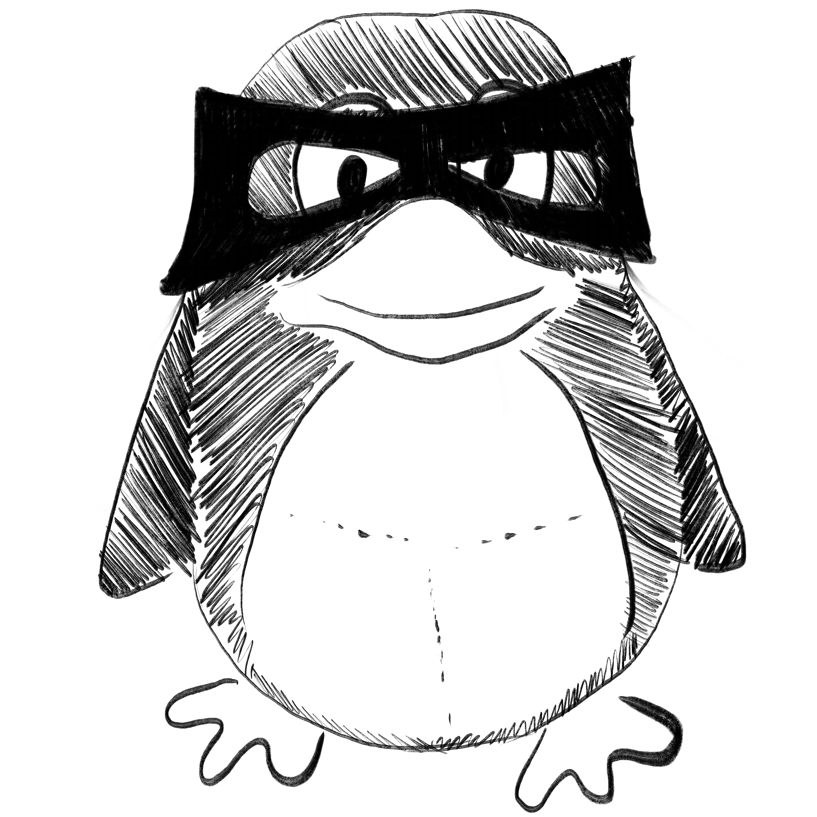
Analysis of the therapeutic interaction provided by a humanoid robot serving stroke survivors as a therapeutic assistant for arm rehabilitation.
In Frontiers in robotics and AI
Platz Thomas, Pedersen Ann Louise, Deutsch Philipp, Umlauft Alexandru-Nicolae, Bader Sebastian
2023
arm, artificial intelligence, interaction, robot, social, stroke, training
Weekly Summary
Receive a weekly summary and discussion of the top papers of the week by leading researchers in the field.