Receive a weekly summary and discussion of the top papers of the week by leading researchers in the field.
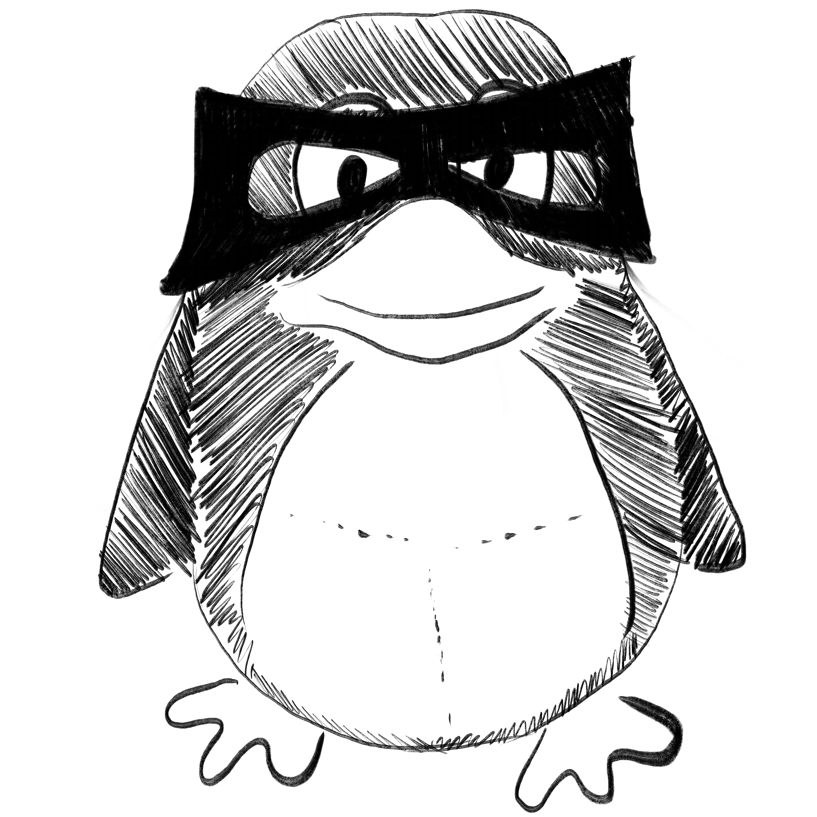
Search, identification, and curation of cell and gene therapy product regulations using augmented intelligent systems.
In Frontiers in medicine
BACKGROUND :
METHODS :
RESULTS :
CONCLUSION :
Schaut William, Shrivastav Akash, Ramakrishnan Srikanth, Bowden Robert
2023
CAR-T, augmented intelligence, automated systematic search, machine learning, regulations, regulatory documents
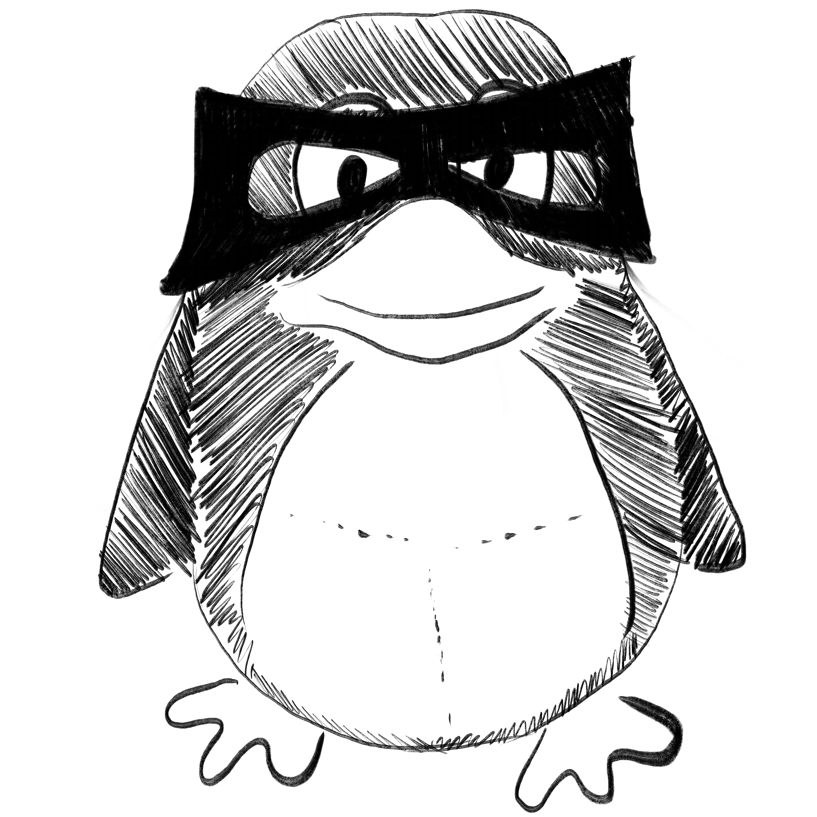
Optimizing MRI-based brain tumor classification and detection using AI: A comparative analysis of neural networks, transfer learning, data augmentation, and the cross-transformer network.
In European journal of radiology open
Anaya-Isaza Andrés, Mera-Jiménez Leonel, Verdugo-Alejo Lucía, Sarasti Luis
2023
Artificial intelligence, Cancer detection, Machine learning, Magnetic resonance imaging, Transformers, Tumors
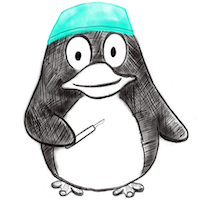
ChatGPT - Reshaping medical education and clinical management.
In Pakistan journal of medical sciences
Khan Rehan Ahmed, Jawaid Masood, Khan Aymen Rehan, Sajjad Madiha
2023
Artificial Intelligence, ChatGPT, Education, NLP, Open AI, clinical management, medical education
Systematic assessment of prognostic molecular features across cancers.
In Cell genomics
Santhanam Balaji, Oikonomou Panos, Tavazoie Saeed
2023-Mar-08
cancer genomics, cancer regulatory networks, precision oncology, prognostic cancer biomarkers
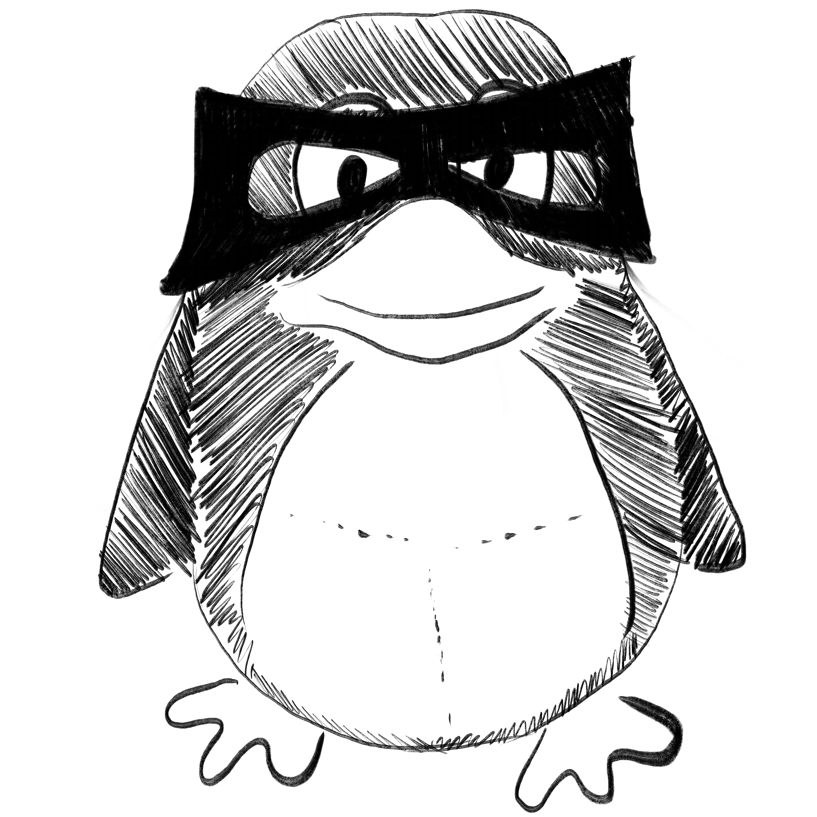
A longan yield estimation approach based on UAV images and deep learning.
In Frontiers in plant science
Li Denghui, Sun Xiaoxuan, Jia Yuhang, Yao Zhongwei, Lin Peiyi, Chen Yingyi, Zhou Haobo, Zhou Zhengqi, Wu Kaixuan, Shi Linlin, Li Jun
2023
UAV image, convolutional neural network, image analysis, regression analysis, yield estimation
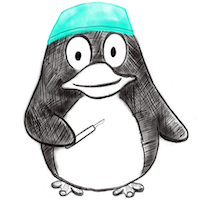
An intracochlear electrocochleography dataset - from raw data to objective analysis using deep learning.
In Scientific data
Schuerch Klaus, Wimmer Wilhelm, Dalbert Adrian, Rummel Christian, Caversaccio Marco, Mantokoudis Georgios, Gawliczek Tom, Weder Stefan
2023-Mar-22
Weekly Summary
Receive a weekly summary and discussion of the top papers of the week by leading researchers in the field.