Receive a weekly summary and discussion of the top papers of the week by leading researchers in the field.
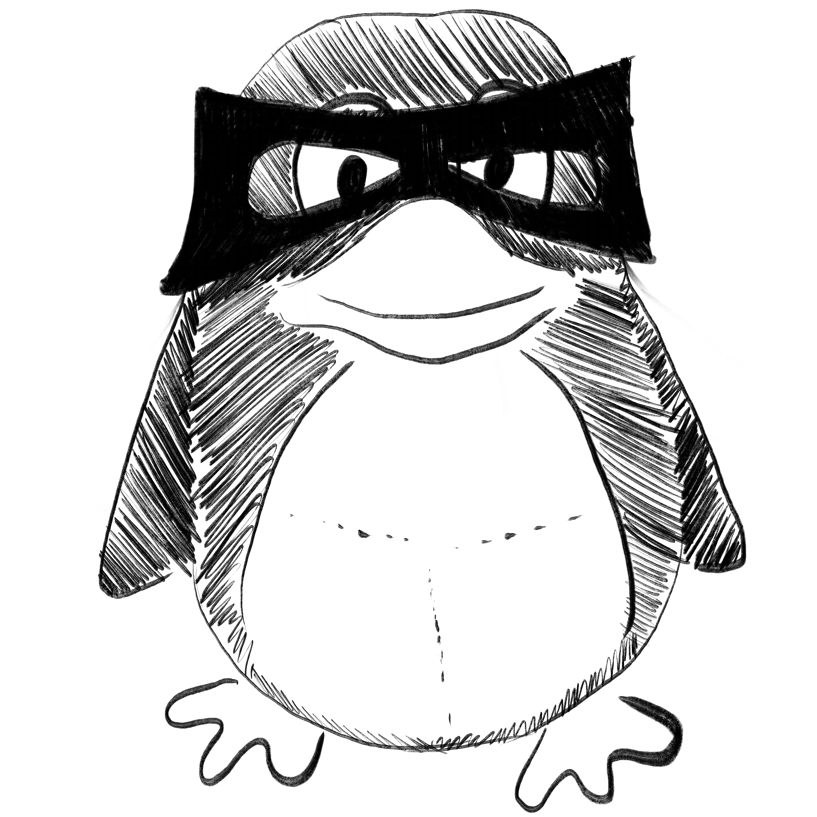
Modeling structure-activity relationships with machine learning to identify GSK3-targeted small molecules as potential COVID-19 therapeutics.
In Frontiers in endocrinology ; h5-index 55.0
Pirzada Rameez Hassan, Ahmad Bilal, Qayyum Naila, Choi Sangdun
2023
GSK3, QSAR, coronaviruses, machine learning, molecular descriptors
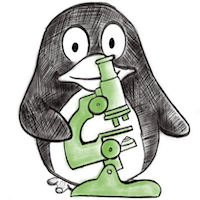
A pathomic approach for tumor-infiltrating lymphocytes classification on breast cancer digital pathology images.
In Heliyon
BACKGROUND AND OBJECTIVES :
METHODS :
RESULTS :
CONCLUSIONS :
Verdicchio Mario, Brancato Valentina, Cavaliere Carlo, Isgrò Francesco, Salvatore Marco, Aiello Marco
2023-Mar
Breast cancer, Digital pathology, Machine learning, Pathomics, Tumor infiltrating lymphocytes
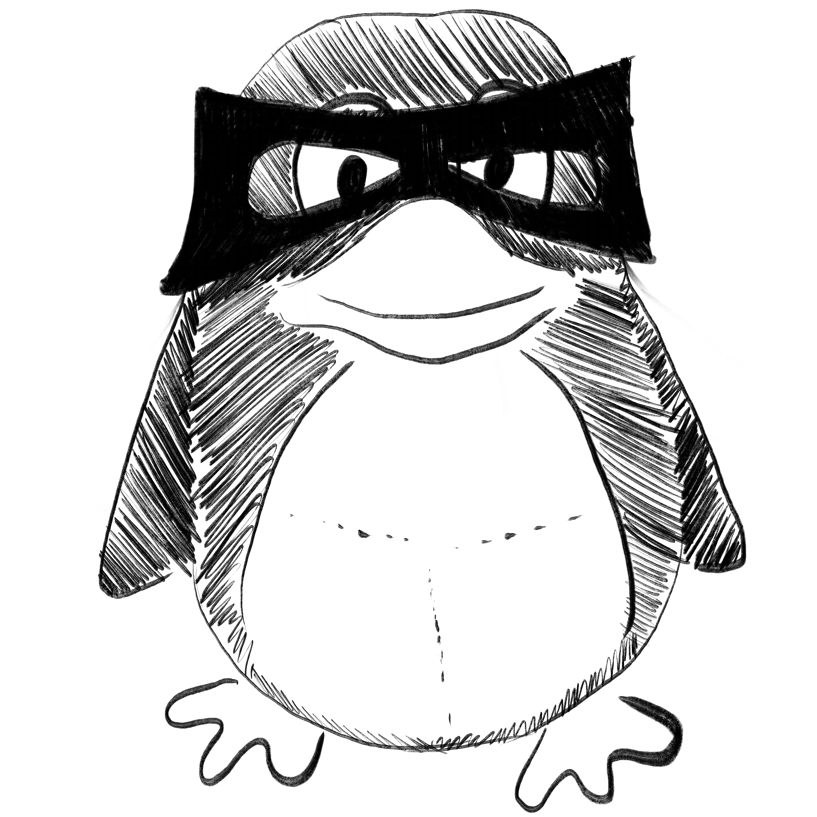
Does managerial short-termism always matter in a firm's corporate social responsibility performance? Evidence from China.
In Heliyon
Xu Xiaohui, Yang Jun
2023-Mar
CSR performance, Managerial short-termism, Random forest regression
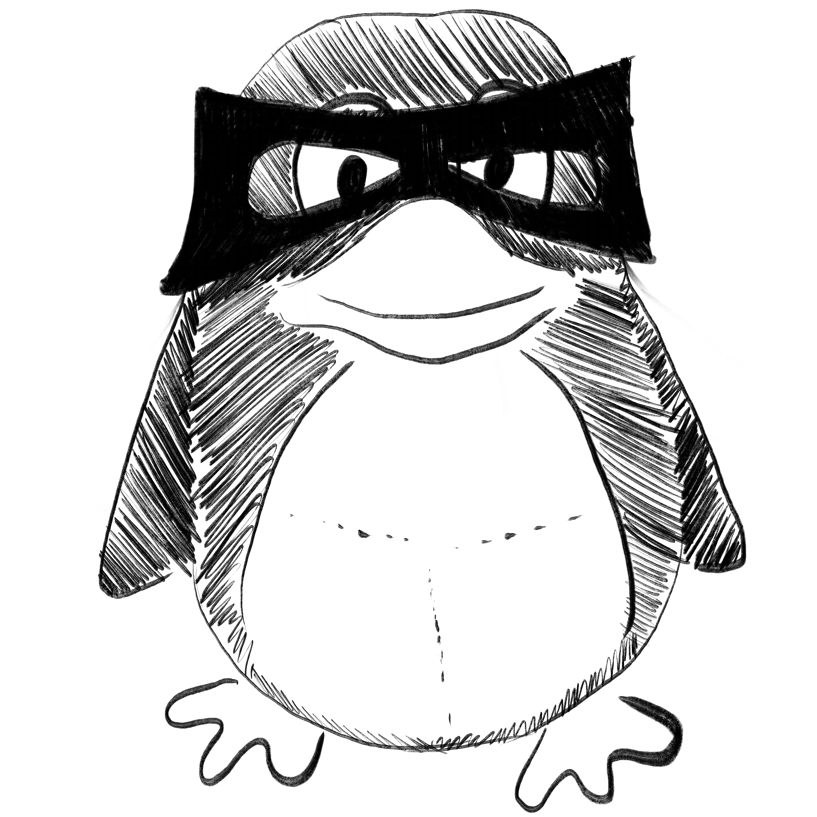
Techniques of power system static security assessment and improvement: A literature survey.
In Heliyon
Hailu Engidaw Abel, Nyakoe George Nyauma, Muriithi Christopher Maina
2023-Mar
FACTS device, Machine learning, Optimal allocation, Performance index, Power system uncertainties, Static security assessment, Static security improvement
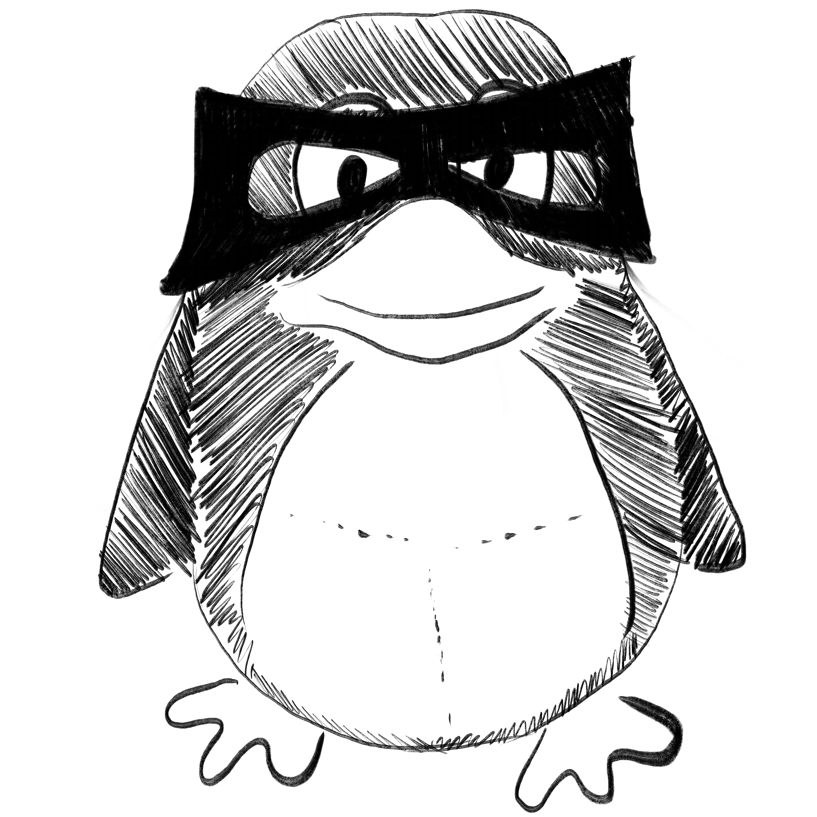
Scientometric and multidimensional contents analysis of PM2.5 concentration prediction.
In Heliyon
Gong Jintao, Ding Lei, Lu Yingyu, Qiong Zhang Yun Li
2023-Mar
Contents analysis, Forecasting methods, Forecasting scale, PM2.5 concentration prediction, Scientometric
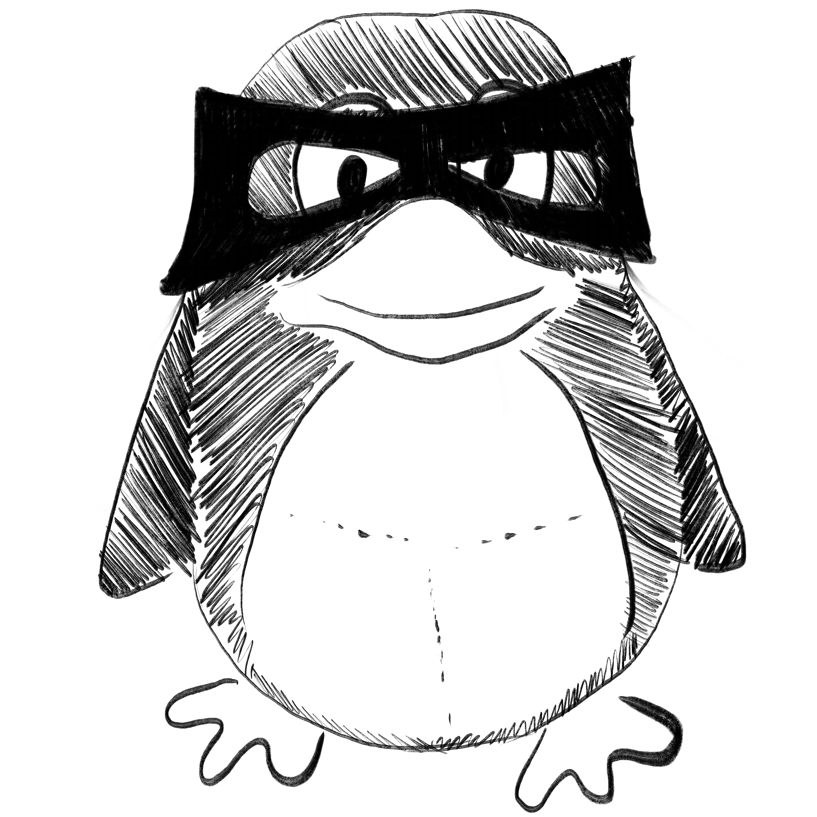
Optimal sizing of residential photovoltaic and battery system connected to the power grid based on the cost of energy and peak load.
In Heliyon
Vahabi Khah Mohammad, Zahedi Rahim, Eskandarpanah Reza, Mirzaei Amir Mohammad, Farahani Omid Noudeh, Malek Iman, Rezaei Nima
2023-Mar
PV battery System, Renewable energy, Sizing, Solar energy
Weekly Summary
Receive a weekly summary and discussion of the top papers of the week by leading researchers in the field.