Receive a weekly summary and discussion of the top papers of the week by leading researchers in the field.
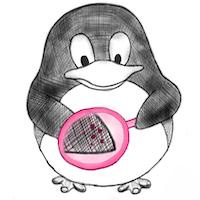
A call for implementing augmented intelligence in pediatric dermatology.
In Pediatric dermatology
Issa Christopher J, Reimer-Taschenbrecker Antonia, Paller Amy S
2023-Mar-23
artificial intelligence, convolutional neural networks, deep learning, health disparities, pediatric dermatology
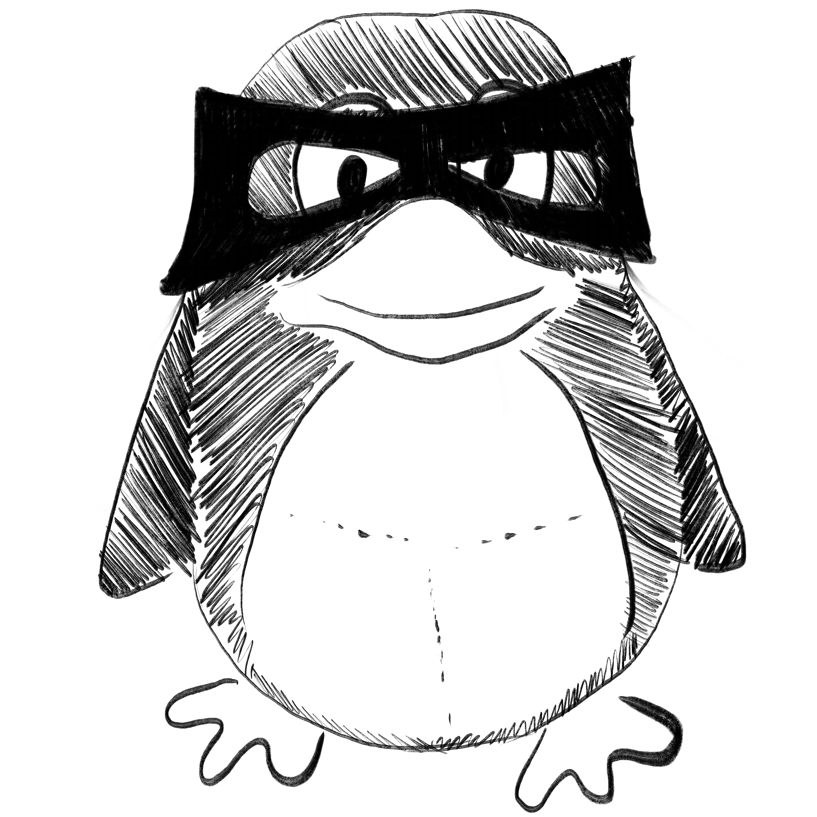
A Long-term Consistent Artificial Intelligence and Remote Sensing-based Soil Moisture Dataset.
In Scientific data
Skulovich Olya, Gentine Pierre
2023-Mar-22
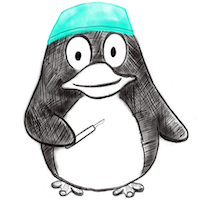
Breast Tumor Classification using Short-ResNet with Pixel-based Tumor Probability Map in Ultrasound Images.
In Ultrasonic imaging
Wang You-Wei, Kuo Tsung-Ter, Chou Yi-Hong, Su Yu, Huang Shing-Hwa, Chen Chii-Jen
2023-Mar-23
breast cancer, convolutional neural network, deep learning, tumor classification, ultrasound
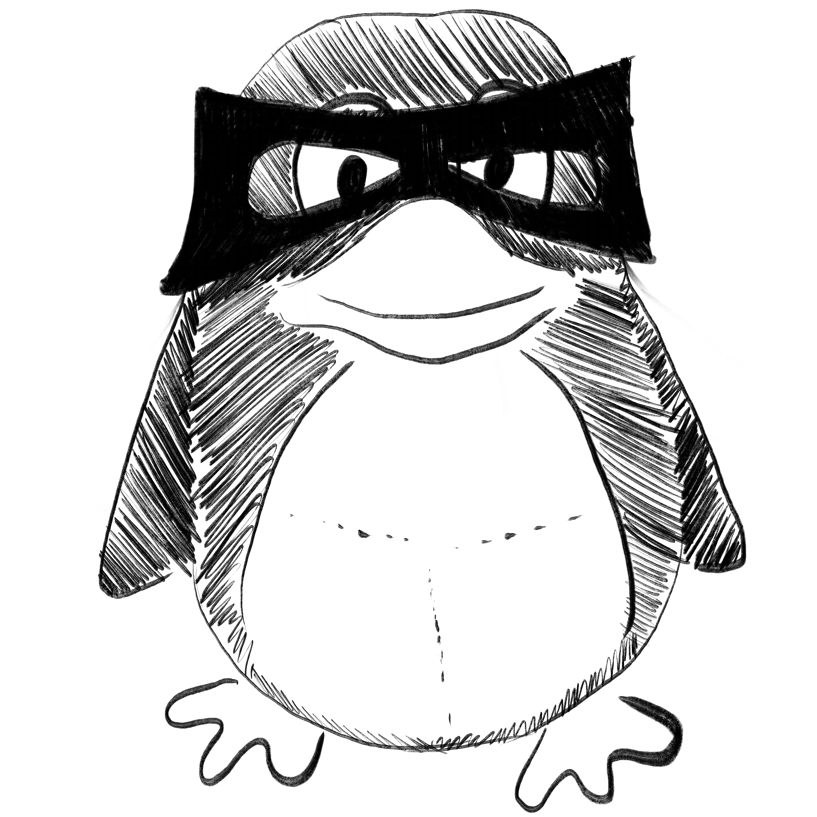
Boundary-oriented Network for Automatic Breast Tumor Segmentation in Ultrasound Images.
In Ultrasonic imaging
Zhang Mengmeng, Huang Aibin, Yang Debiao, Xu Rui
2023-Mar-23
U-Net, breast tumors, deep learning, image segmentation, ultrasound images
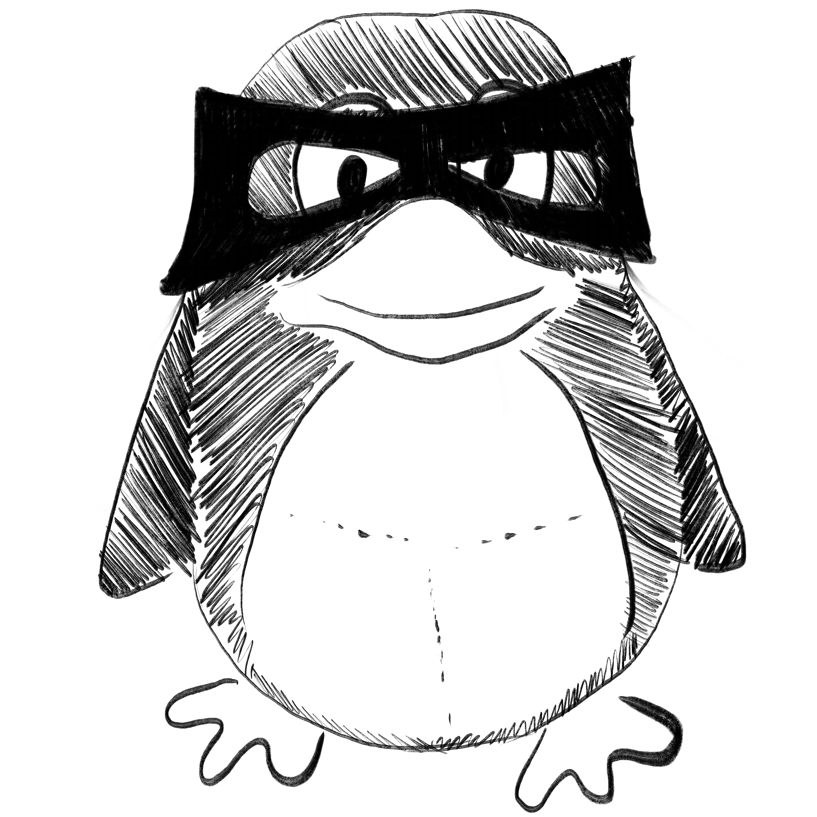
ANPELA: Significantly Enhanced Quantification Tool for Cytometry-Based Single-Cell Proteomics.
In Advanced science (Weinheim, Baden-Wurttemberg, Germany)
Zhang Ying, Sun Huaicheng, Lian Xichen, Tang Jing, Zhu Feng
2023-Mar-22
cell population identification, comprehensive assessment, parallel computing, protein quantification, single-cell proteomics, trajectory inference
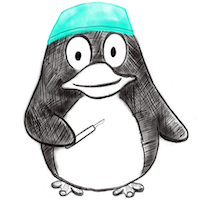
Identification of copper metabolism-related subtypes and establishment of the prognostic model in ovarian cancer.
In Frontiers in endocrinology ; h5-index 55.0
BACKGROUND :
METHODS :
RESULTS :
CONCLUSION :
Zhao Songyun, Zhang Xin, Gao Feng, Chi Hao, Zhang Jinhao, Xia Zhijia, Cheng Chao, Liu Jinhui
2023
OC, Tumor microenvironment, copper metabolism, immunotherapy, machine learning, risk score signature
Weekly Summary
Receive a weekly summary and discussion of the top papers of the week by leading researchers in the field.