Receive a weekly summary and discussion of the top papers of the week by leading researchers in the field.
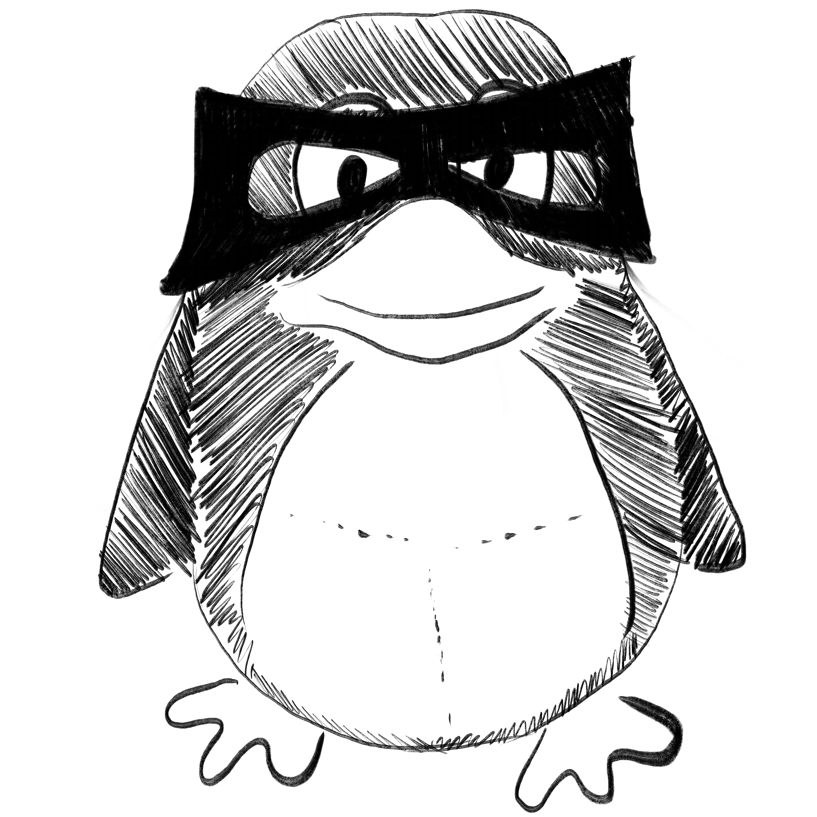
A network-based analysis detects cocaine-induced changes in social interactions in Drosophila melanogaster.
In PloS one ; h5-index 176.0
Petrović Milan, Meštrović Ana, Andretić Waldowski Rozi, Filošević Vujnović Ana
2023
Domain-guided data augmentation for deep learning on medical imaging.
In PloS one ; h5-index 176.0
Athalye Chinmayee, Arnaout Rima
2023
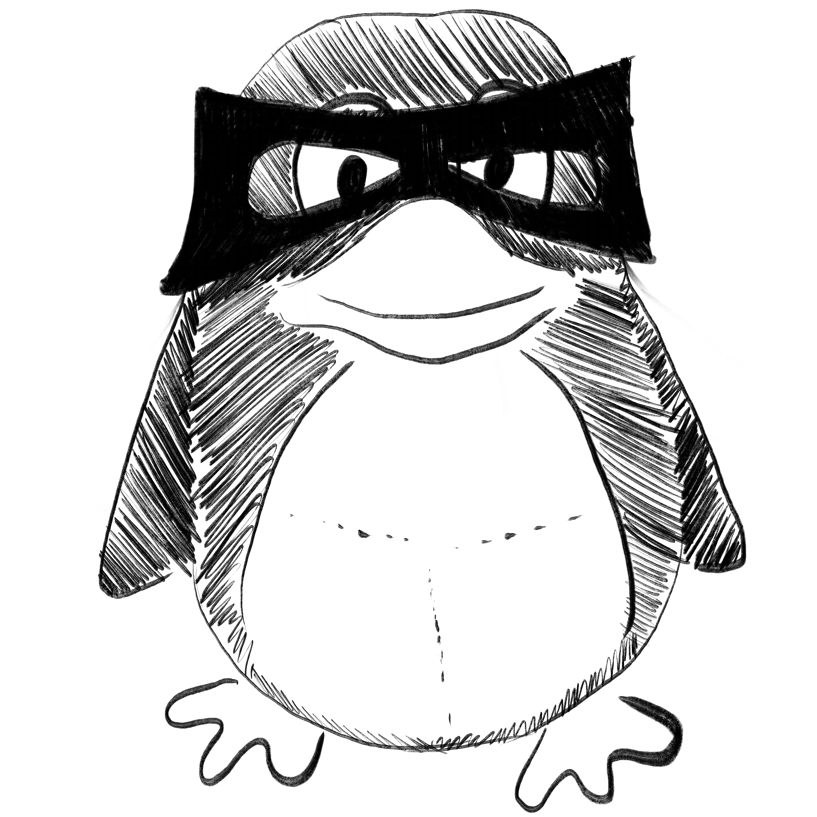
Programming 3D curved mesosurfaces using microlattice designs.
In Science (New York, N.Y.)
Cheng Xu, Fan Zhichao, Yao Shenglian, Jin Tianqi, Lv Zengyao, Lan Yu, Bo Renheng, Chen Yitong, Zhang Fan, Shen Zhangming, Wan Huanhuan, Huang Yonggang, Zhang Yihui
2023-Mar-24
Current and Advanced Applications of Gadoxetic Acid-enhanced MRI in Hepatobiliary Disorders.
In Radiographics : a review publication of the Radiological Society of North America, Inc
Baleato-González Sandra, Vilanova Joan C, Luna Antonio, Menéndez de Llano Rafael, Laguna-Reyes Juan Pablo, Machado-Pereira Diogo M, Bermúdez-Naveira Anaberta, Osorio-Vázquez Iria, Alcalá-Mata Lidia, García-Figueiras Roberto
2023-Apr
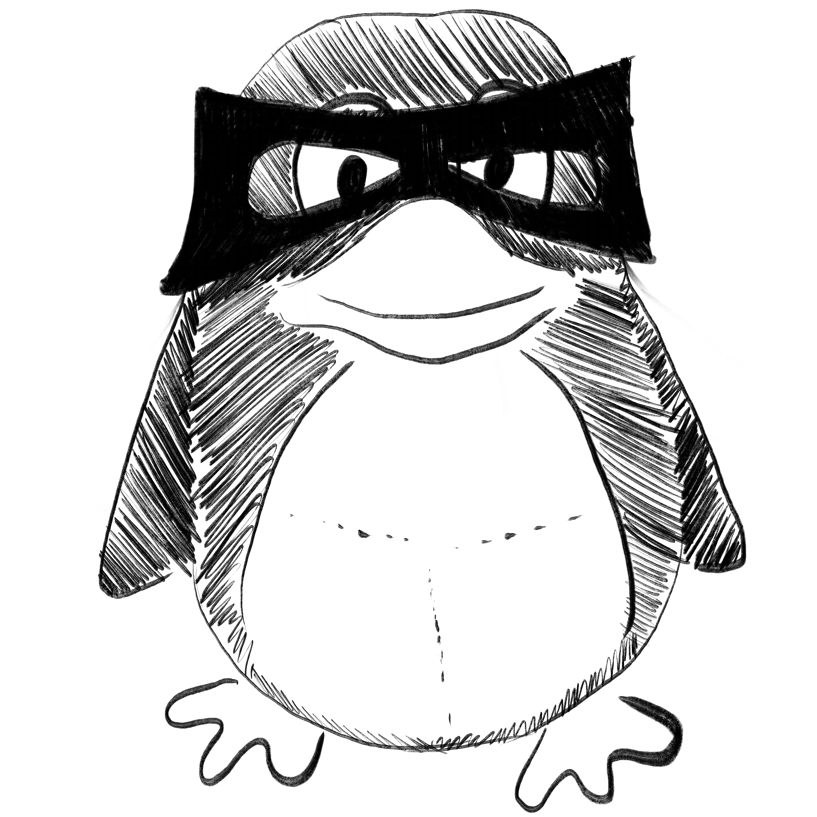
Computational Approaches for Peroxisomal Protein Localization.
In Methods in molecular biology (Clifton, N.J.)
Anteghini Marco, Martins Dos Santos Vitor A P
2023
Cellular compartments, Machine learning, Peroxisome targeting signal, Sub-organelle localization, Subcellular localization
The Application of Artificial Intelligence to Investigate Long-Term Outcomes and Assess Optimal Margin Width in Hepatectomy for Intrahepatic Cholangiocarcinoma.
In Annals of surgical oncology ; h5-index 71.0
BACKGROUND :
METHODS :
RESULTS :
CONCLUSIONS :
Alaimo Laura, Moazzam Zorays, Endo Yutaka, Lima Henrique A, Butey Swatika P, Ruzzenente Andrea, Guglielmi Alfredo, Aldrighetti Luca, Weiss Matthew, Bauer Todd W, Alexandrescu Sorin, Poultsides George A, Maithel Shishir K, Marques Hugo P, Martel Guillaume, Pulitano Carlo, Shen Feng, Cauchy François, Koerkamp Bas Groot, Endo Itaru, Kitago Minoru, Kim Alex, Ejaz Aslam, Beane Joal, Cloyd Jordan, Pawlik Timothy M
2023-Mar-23
Weekly Summary
Receive a weekly summary and discussion of the top papers of the week by leading researchers in the field.