Receive a weekly summary and discussion of the top papers of the week by leading researchers in the field.
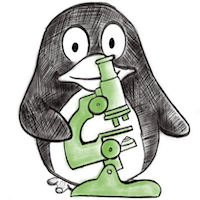
SC-MIL: Supervised Contrastive Multiple Instance Learning for Imbalanced Classification in Pathology
ArXiv Preprint
Dinkar Juyal, Siddhant Shingi, Syed Ashar Javed, Harshith Padigela, Chintan Shah, Anand Sampat, Archit Khosla, John Abel, Amaro Taylor-Weiner
2023-03-23
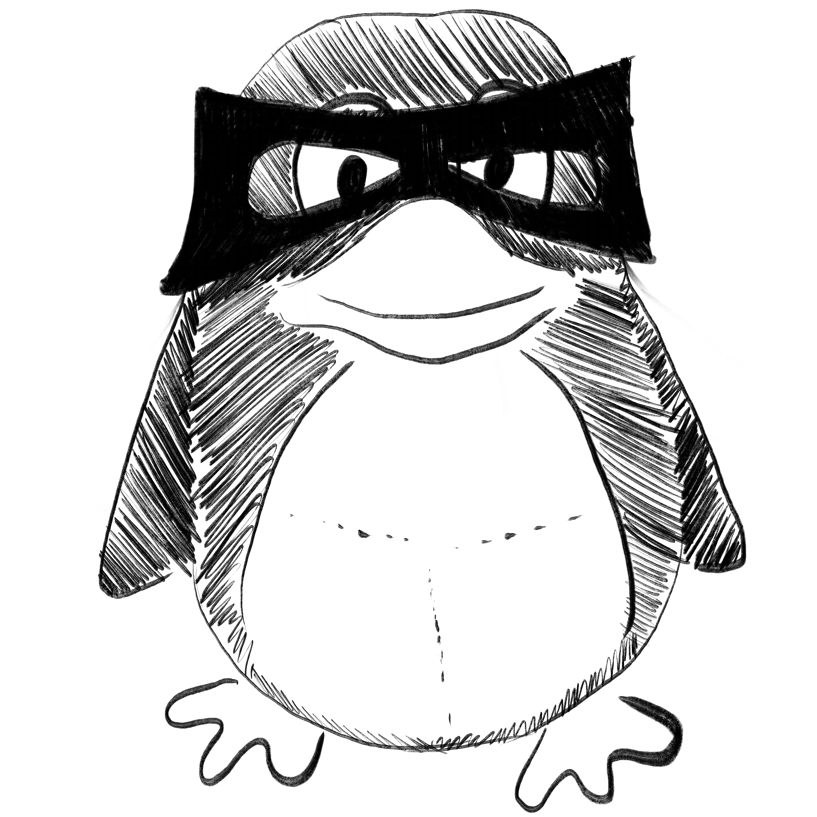
Deep learning for pancreatic diseases based on endoscopic ultrasound: A systematic review.
In International journal of medical informatics ; h5-index 49.0
BACKGROUND AND AIMS :
METHODS :
RESULTS :
CONCLUSIONS :
Yin Minyue, Liu Lu, Gao Jingwen, Lin Jiaxi, Qu Shuting, Xu Wei, Liu Xiaolin, Xu Chunfang, Zhu Jinzhou
2023-Mar-18
Convolutional neural networks, Deep learning, Endoscopic ultrasonography, Pancreatic diseases, Systematic review
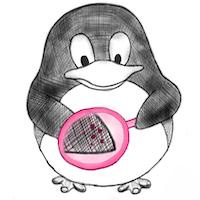
HGM-cNet: Integrating hippocampal gray matter probability map into a cascaded deep learning framework improves hippocampus segmentation.
In European journal of radiology ; h5-index 47.0
Zheng Qiang, Liu Bin, Gao Yan, Bai Lijun, Cheng Yu, Li Honglun
2023-Mar-15
Deep learning, Gray matter volume, Hippocampus, Image segmentation, Multi-atlas segmentation
Application of a developed triple-classification machine learning model for carcinogenic prediction of hazardous organic chemicals to the US, EU, and WHO based on Chinese database.
In Ecotoxicology and environmental safety ; h5-index 67.0
Hao Ning, Sun Peixuan, Zhao Wenjin, Li Xixi
2023-Mar-20
Carcinogenic chemicals, Carcinogenicity classification prediction model, Machine learning, Model evaluation metrics, Molecular structure, Toxicokinetics
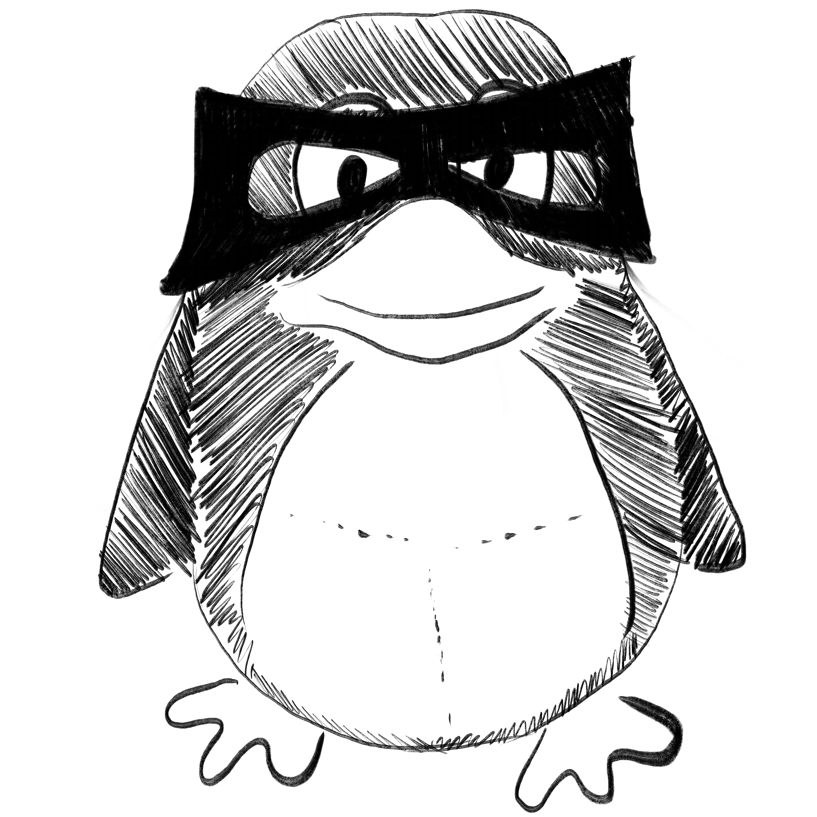
Development of prediction models for one-year brain tumour survival using machine learning: a comparison of accuracy and interpretability.
In Computer methods and programs in biomedicine
BACKGROUND AND OBJECTIVE :
METHODS :
RESULTS :
CONCLUSION :
Charlton Colleen E, Poon Michael T C, Brennan Paul M, Fleuriot Jacques D
2023-Mar-13
Bayesian rule lists, Brain cancer, Explainable boosting machine, Interpretable models, Machine learning, Survival
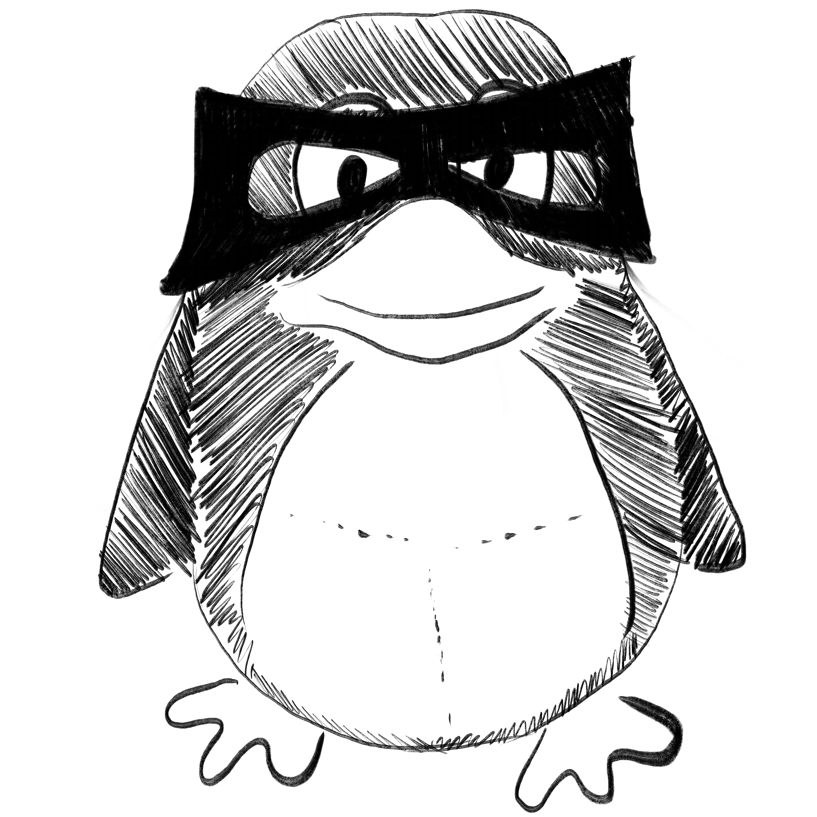
Forecasting and Optimizing Dual Media Filter Performance via Machine Learning.
In Water research
Moradi Sina, Omar Amr, Zhou Zhuoyu, Agostino Anthony, Gandomkar Ziba, Bustamante Heriberto, Power Kaye, Henderson Rita, Leslie Greg
2023-Mar-12
Filtration performance, Hyper-parameter optimisation, Machine learning approach, Unit filter run volume
Weekly Summary
Receive a weekly summary and discussion of the top papers of the week by leading researchers in the field.