Receive a weekly summary and discussion of the top papers of the week by leading researchers in the field.
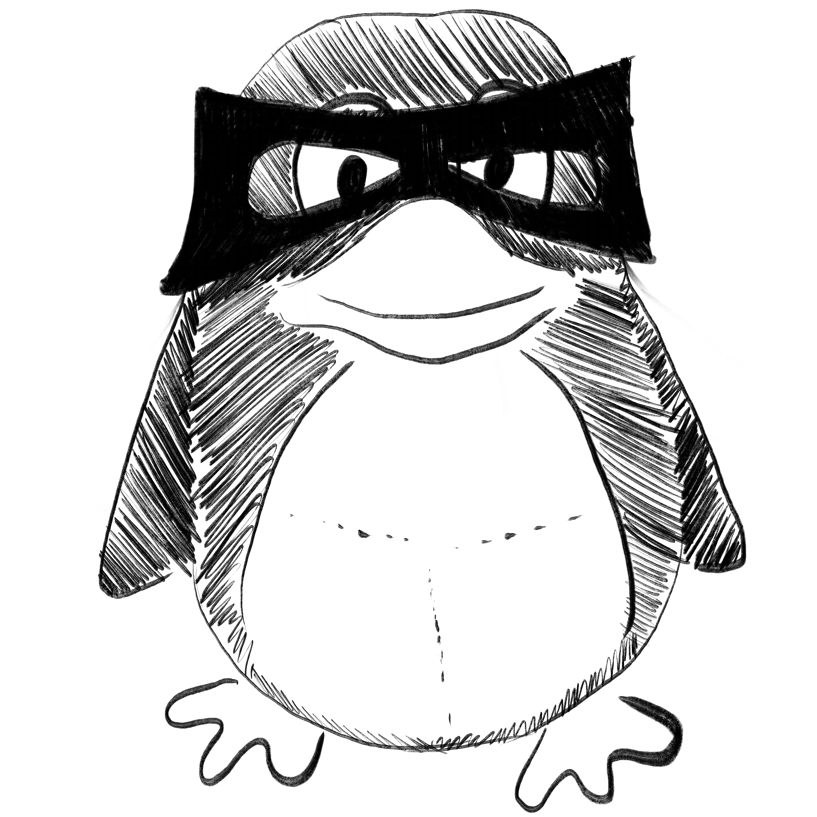
Asynchronous fault detection filter of positive Markov jump systems by dynamic event-triggered mechanism.
In ISA transactions
Yin Kai, Yang Dedong
2023-Mar-15
A hidden Markov model, Dynamic event-triggered mechanism, Non-triviality, Positive Markov jump systems, Positive asynchronous fault detection filter
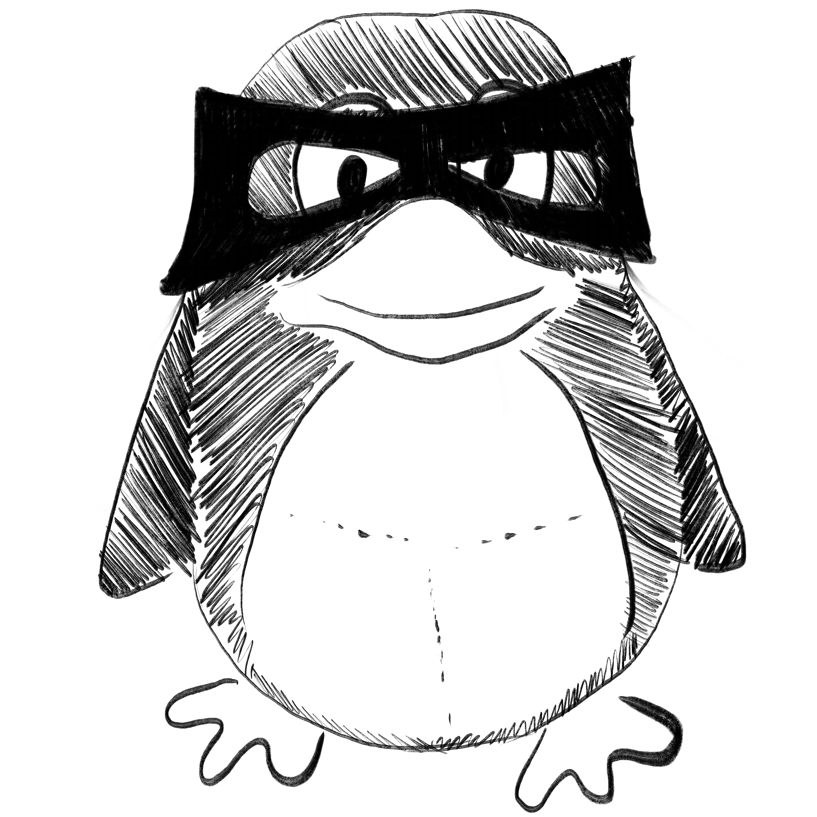
Multivariable time series classification for clinical mastitis detection and prediction in automated milking systems.
In Journal of dairy science
Fan X, Watters R D, Nydam D V, Virkler P D, Wieland M, Reed K F
2023-Mar-17
automated milking system, clinical mastitis, machine learning, time series classification
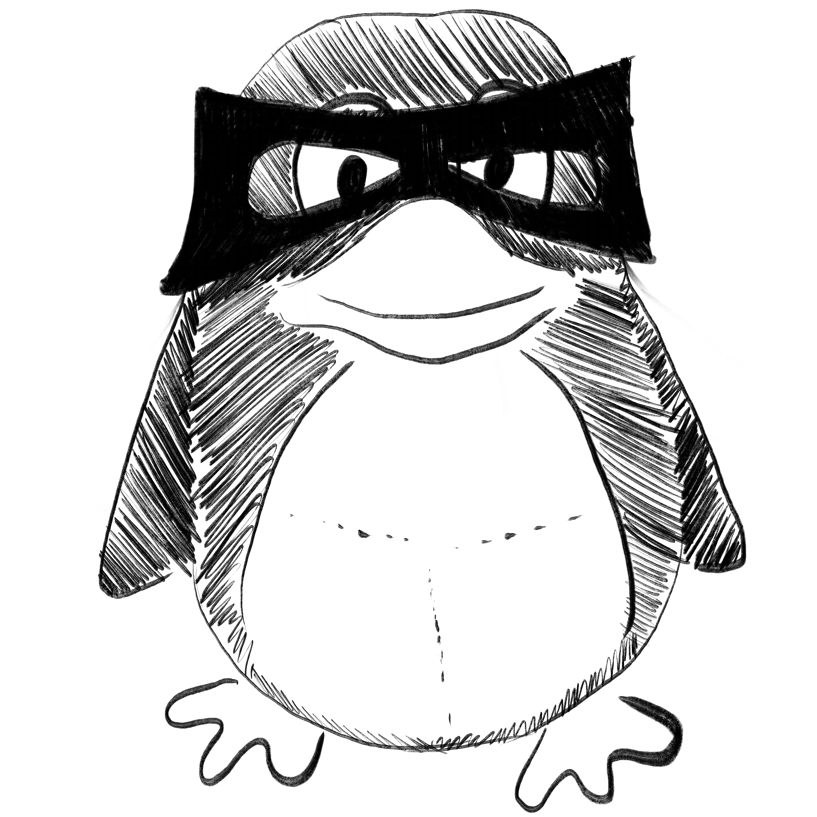
Using machine learning algorithm to predict the risk of post-traumatic stress disorder among firefighters in Changsha.
In Zhong nan da xue xue bao. Yi xue ban = Journal of Central South University. Medical sciences
OBJECTIVES :
METHODS :
RESULTS :
CONCLUSIONS :
Deng Aoqian, Yang Yanyi, Li Yunjing, Huang Mei, Li Liang, Lu Yimei, Chen Wentao, Yuan Rui, Ju Yumeng, Liu Bangshan, Zhang Yan
2023-Jan-28
firefighter, machine learning algorithm, post-traumatic stress disorder, predictor
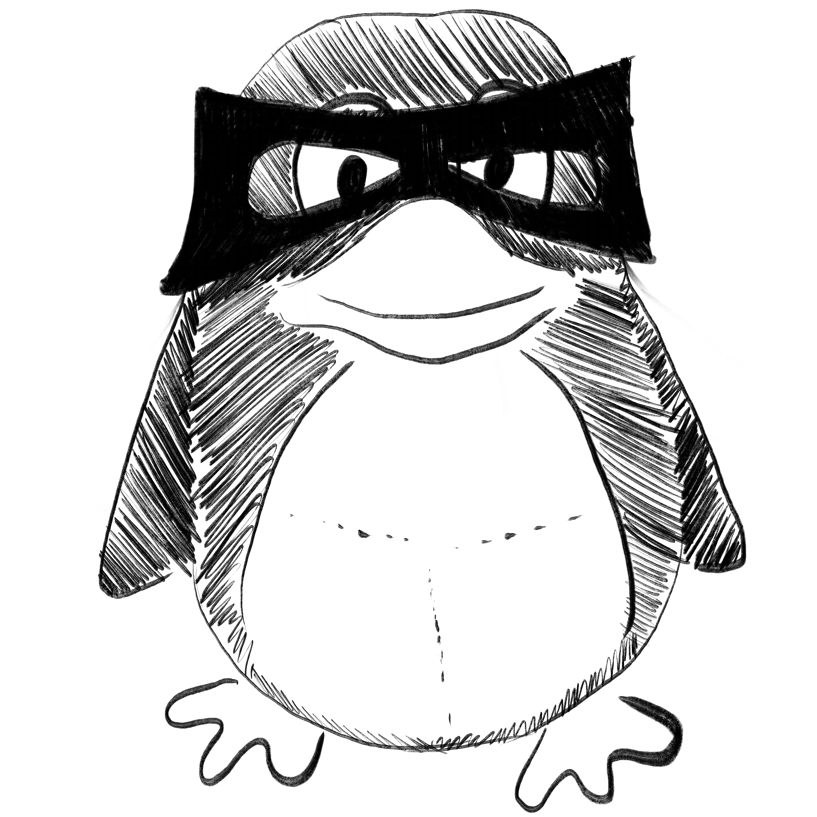
Multiplex single-cell droplet PCR with machine learning for detection of high-risk human papillomaviruses.
In Analytica chimica acta
Huang Yizheng, Sun Linjun, Liu Wenwen, Yang Ling, Song Zhigang, Ning Xin, Li Weijun, Tan Manqing, Yu Yude, Li Zhao
2023-Apr-29
High-risk HPV detection, Machine learning, Multiplex microfluidic chip, Single-cell droplet PCR
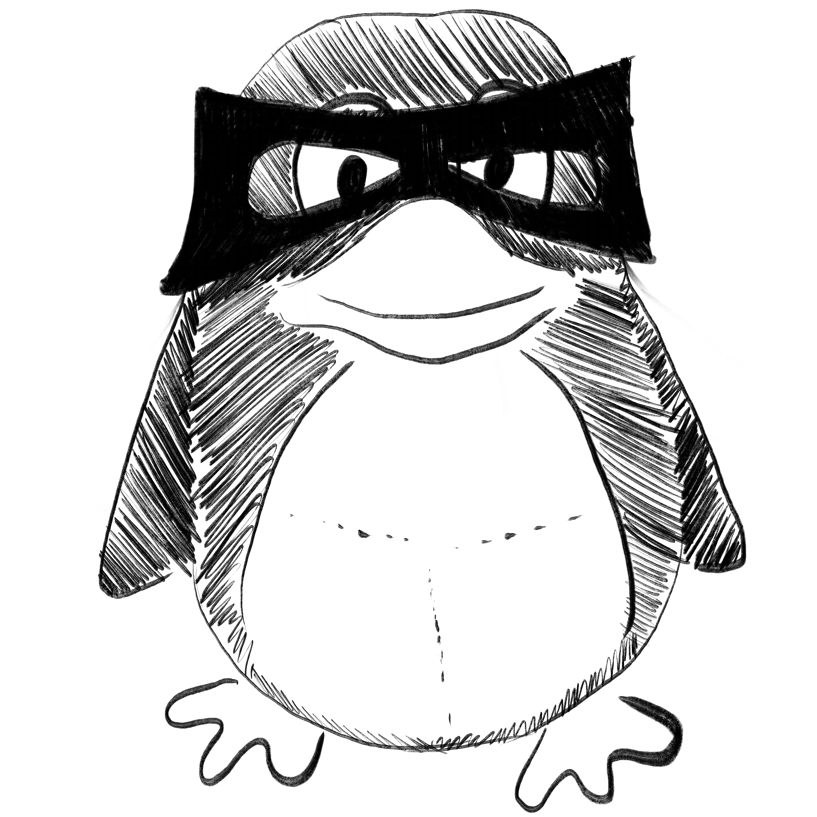
Bioinformatics analysis of rheumatoid arthritis tissues identifies genes and potential drugs that are expressed specifically.
In Scientific reports ; h5-index 158.0
He Qingshan, Ding Hanmeng
2023-Mar-18
Integration of Radiomics and Tumor Biomarkers in Interpretable Machine Learning Models
ArXiv Preprint
Lennart Brocki, Neo Christopher Chung
2023-03-20
Weekly Summary
Receive a weekly summary and discussion of the top papers of the week by leading researchers in the field.