Receive a weekly summary and discussion of the top papers of the week by leading researchers in the field.
Interoperable slide microscopy viewer and annotation tool for imaging data science and computational pathology.
In Nature communications ; h5-index 260.0
Gorman Chris, Punzo Davide, Octaviano Igor, Pieper Steven, Longabaugh William J R, Clunie David A, Kikinis Ron, Fedorov Andrey Y, Herrmann Markus D
2023-Mar-22
Immune gene patterns and characterization of the tumor immune microenvironment associated with cancer immunotherapy efficacy.
In Heliyon
Lin Lili, Zhang Wenda, Chen Yongjian, Ren Wei, Zhao Jianli, Ouyang Wenhao, He Zifan, Su Weifeng, Yao Herui, Yu Yunfang
2023-Mar
AUC, Area under the curve, CIs, Confidence intervals, CTL, Cytotoxic T-lymphocyte infiltration, Cancer, GEO, Gene Expression Omnibus, GO, Gene Ontology, GSEA, Gene set enrichment analysis, GSVA, Gene set variation analysis, HLAs, Human leukocyte antigens, HRs, Hazard ratios, Immunotherapy, KEGG, Kyoto Encyclopedia of Genes and Genomes, LASSO, Penalized logistic least absolute shrinkage and selector operation, Machine learning, NSCLC, Non-small cell lung cancer, OS, Overall survival, PCA, Principal componentanalysis, PD-L1, Programmed death ligand-1, PFS, Profession-free survival, RNA-seq, Transcriptome RNA sequencing, ROC, receiver operating characteristic curves, TCGA, The Cancer Genome Atlas, TMB, Tumor mutation burden, TME, Tumor immunemicroenvironment, Tumor immune microenvironment, WGCNA, Weighted gene co-expression network analysis, lncRNA, Long non-coding RNA
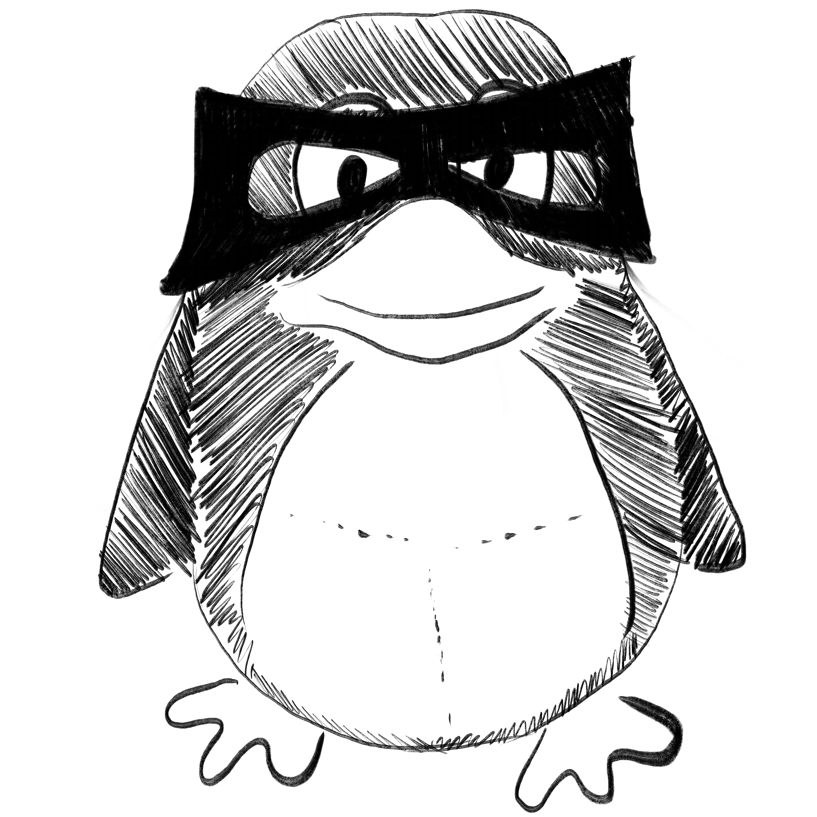
Assessing the influence of industry 4.0 technologies on occupational health and safety.
In Heliyon
Arana-Landín Germán, Laskurain-Iturbe Iker, Iturrate Mikel, Landeta-Manzano Beñat
2023-Mar
ISO 45001, Industry 4.0 technologies, Occupational health and safety, Occupational risks
Amplitude of low-frequency fluctuation-based regional radiomics similarity network: Biomarker for Parkinson's disease.
In Heliyon
Shi Dafa, Ren Zhendong, Zhang Haoran, Wang Guangsong, Guo Qiu, Wang Siyuan, Ding Jie, Yao Xiang, Li Yanfei, Ren Ke
2023-Mar
Amplitude of low-frequency fluctuation (ALFF), Biomarker, Machine learning, Network, Parkinson’s disease
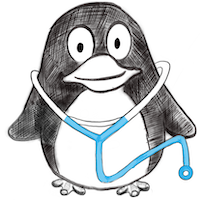
Deep learning-assisted diagnosis of chronic atrophic gastritis in endoscopy.
In Frontiers in oncology
BACKGROUND :
METHODS :
RESULTS :
CONCLUSIONS :
Shi Yanting, Wei Ning, Wang Kunhong, Wu Jingjing, Tao Tao, Li Na, Lv Bing
2023
chronic atrophic gastritis (CAG), deep learning - artificial intelligence, endoscopy, gastric cancer, transfer learning
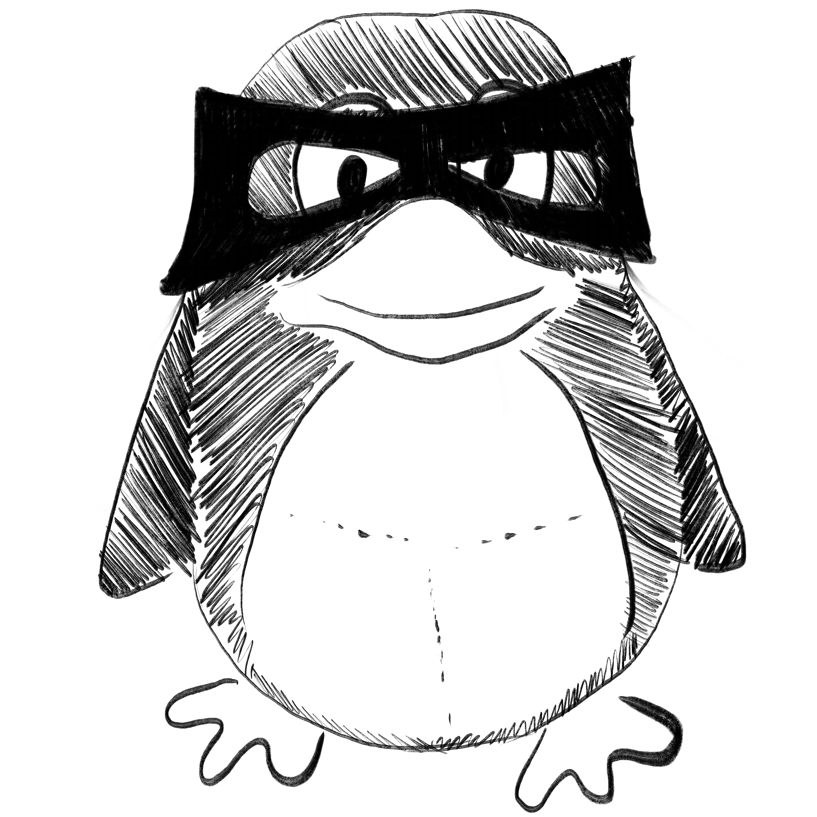
A versatile and fast-sampling rate wearable analog data logger.
In MethodsX
Bouchekioua Youcef, Matsui Hiroshi, Watanabe Shigeru
2023
Accelerometer, Analog, Data logger, Microcontroller, Movement disorders, Sensor, Versatile and Fast-Sampling Rate Wearable Analog Data Logger
Weekly Summary
Receive a weekly summary and discussion of the top papers of the week by leading researchers in the field.