Receive a weekly summary and discussion of the top papers of the week by leading researchers in the field.
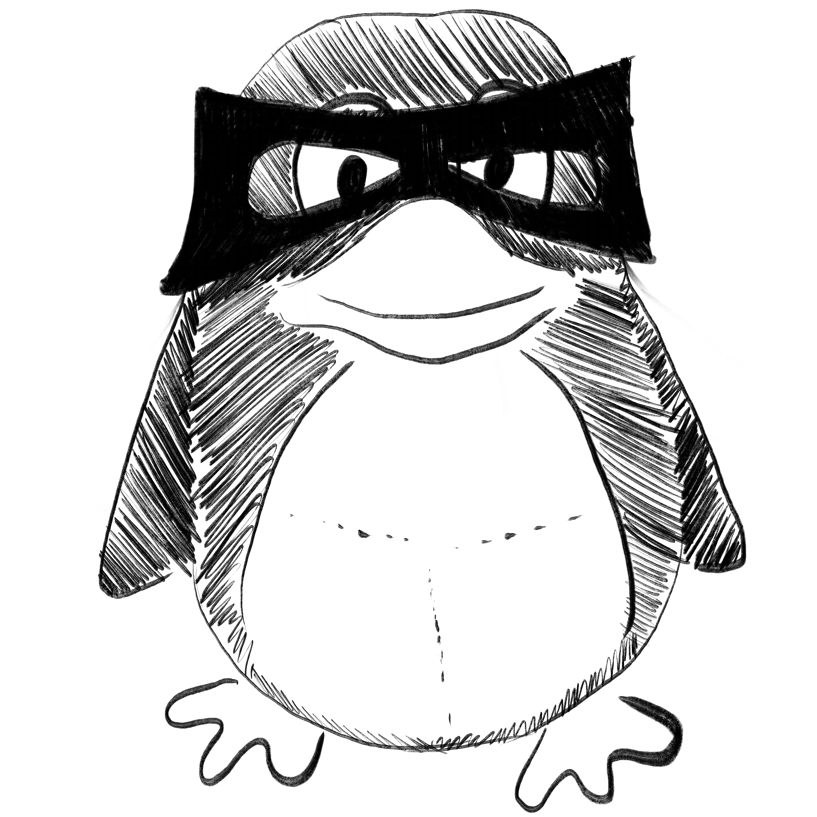
NeuroSuites: An online platform for running neuroscience, statistical, and machine learning tools.
In Frontiers in neuroinformatics
Moreno-Rodríguez José Luis, Larrañaga Pedro, Bielza Concha
2023
Bayesian networks, machine learning, neuroscience, statistical analysis, supervised classification, unsupervised classification, web application
Deep-learning based quantification model for hip bone marrow edema and synovitis in patients with spondyloarthritis based on magnetic resonance images.
In Frontiers in physiology
Zheng Yan, Bai Chao, Zhang Kui, Han Qing, Guan Qingbiao, Liu Ying, Zheng Zhaohui, Xia Yong, Zhu Ping
2023
deep learning, hip, magnetic resonance imaging, spondyloarthritis, synovitis
Computerization of the Work of General Practitioners: Mixed Methods Survey of Final-Year Medical Students in Ireland.
In JMIR medical education
BACKGROUND :
OBJECTIVE :
METHODS :
RESULTS :
CONCLUSIONS :
Blease Charlotte, Kharko Anna, Bernstein Michael, Bradley Colin, Houston Muiris, Walsh Ian, D Mandl Kenneth
2023-Mar-20
COVID-19, artificial intelligence, biomedical, design, digital health, general practitioners, machine learning, medical education, medical professional, medical students, survey, technology, tool
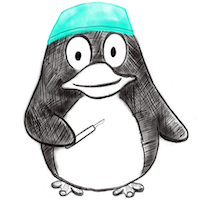
Improving Resource Utilization for Arthroplasty Care by Leveraging Machine Learning and Optimization: A Systematic Review.
In Arthroplasty today
BACKGROUND :
METHODS :
RESULTS :
CONCLUSIONS :
Entezari Bahar, Koucheki Robert, Abbas Aazad, Toor Jay, Wolfstadt Jesse I, Ravi Bheeshma, Whyne Cari, Lex Johnathan R
2023-Apr
Artificial intelligence, Optimization, Predictive modeling, Surgical scheduling, Total hip arthroplasty, Total knee arthroplasty
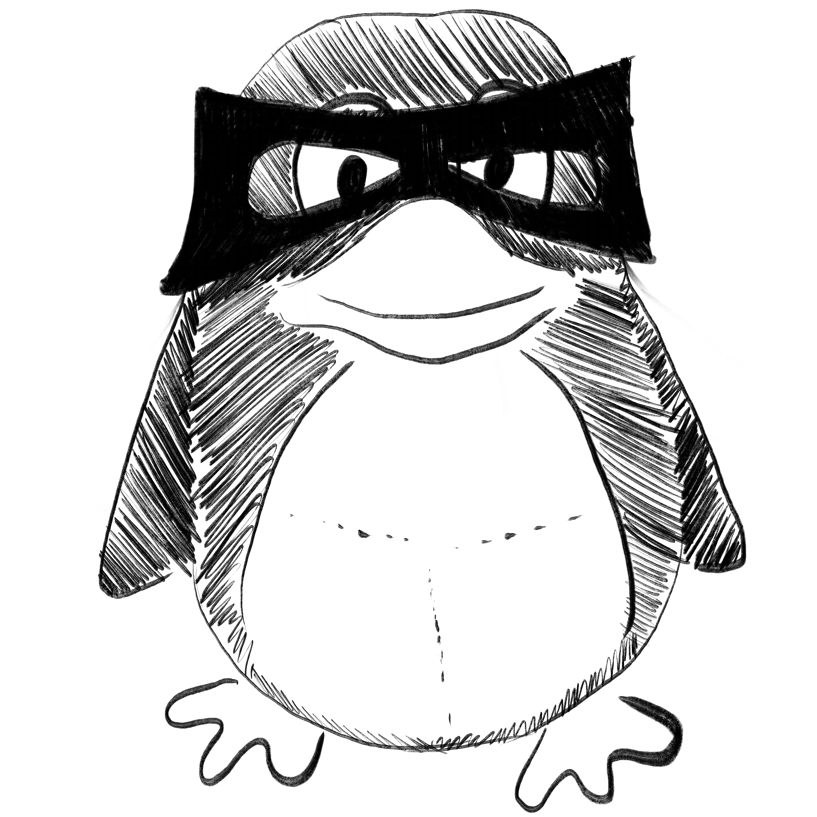
Interpretation of Depression Detection Models via Feature Selection Methods.
In IEEE transactions on affective computing
Alghowinem Sharifa, Gedeon Tom, Goecke Roland, Cohn Jeffrey F, Parker Gordon
2023
datasets generalisation, depression detection, feature selection, multimodal analysis
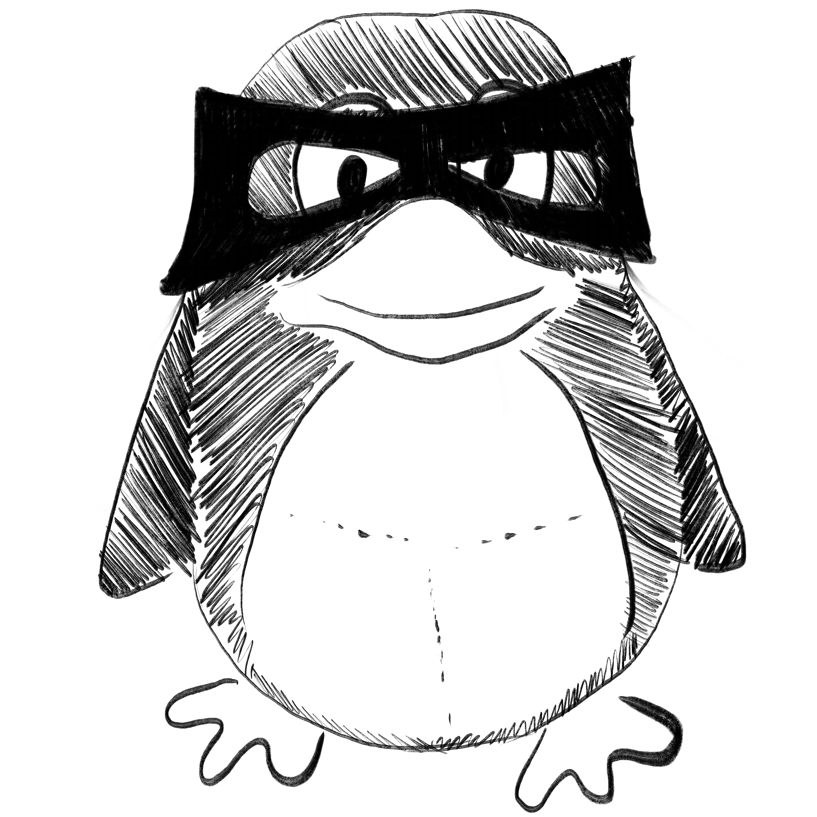
Precision recruitment for high-risk participants in a COVID-19 cohort study.
In Contemporary clinical trials communications
BACKGROUND :
METHODS :
RESULTS :
CONCLUSION :
Mezlini Aziz M, Caddigan Eamon, Shapiro Allison, Ramirez Ernesto, Kondow-McConaghy Helena M, Yang Justin, DeMarco Kerry, Naraghi-Arani Pejman, Foschini Luca
2023-Jun
CDC, Centers for Disease Control and Prevention, COVID-19, Clinical trials, GAMs, generalized additive models, Risk modeling
Weekly Summary
Receive a weekly summary and discussion of the top papers of the week by leading researchers in the field.