Receive a weekly summary and discussion of the top papers of the week by leading researchers in the field.
Examining thematic and emotional differences across Twitter, Reddit, and YouTube: The case of COVID-19 vaccine side effects.
In Computers in human behavior ; h5-index 125.0
Kwon Soyeon, Park Albert
2023-Jul
Consumer health information, Schema theory, Social media, Social network analysis, Unsupervised machine learning
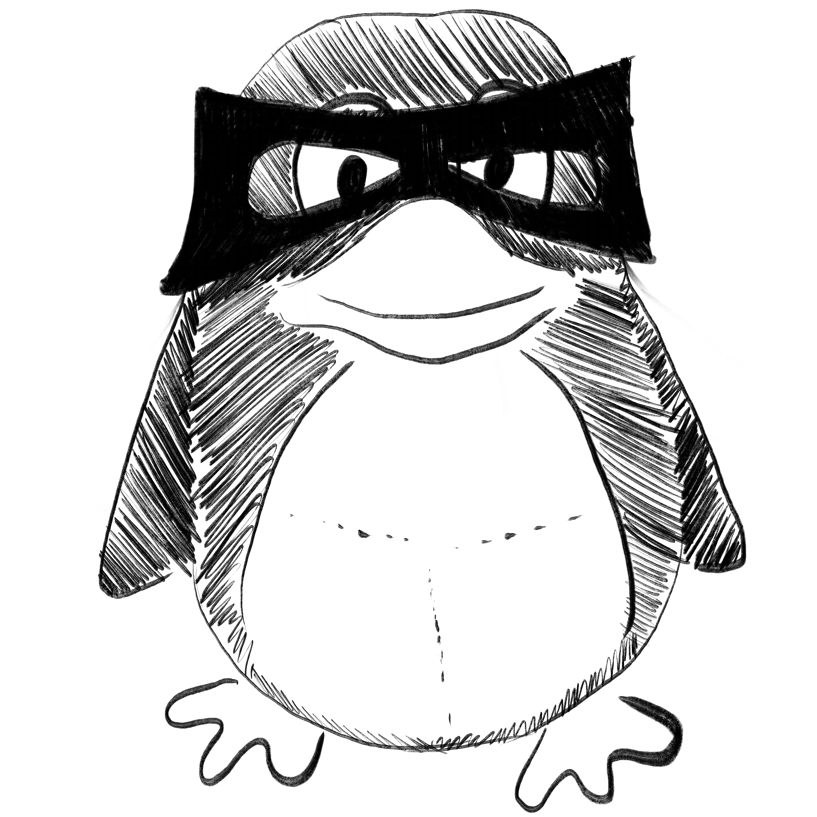
A study on Shine-Muscat grape detection at maturity based on deep learning.
In Scientific reports ; h5-index 158.0
Wei Xinjie, Xie Fuxiang, Wang Kai, Song Jian, Bai Yang
2023-Mar-20
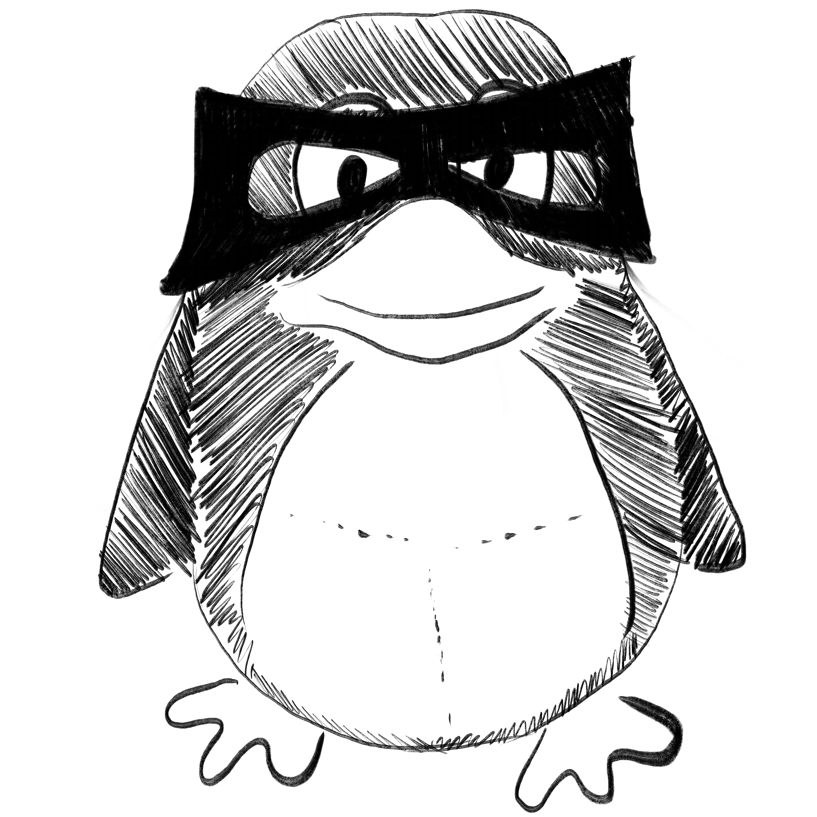
CBCovid19EC: A dataset complete blood count and PCR test for COVID-19 detection in Ecuadorian population.
In Data in brief
Ordoñez-Avila R, Parraga-Alava J, Hormaza J Meza, Vaca-Cárdenas L, Portmann E, Terán L, Dorn M
2023-Apr
Ecuador, Hematological data, Machine learning, SARS-Cov-2
MEDIMP: Medical Images and Prompts for renal transplant representation learning
ArXiv Preprint
Leo Milecki, Vicky Kalogeiton, Sylvain Bodard, Dany Anglicheau, Jean-Michel Correas, Marc-Olivier Timsit, Maria Vakalopoulou
2023-03-22
Splicing signature database development to delineate cancer pathways using literature mining and transcriptome machine learning.
In Computational and structural biotechnology journal
Lee Kyubin, Hyung Daejin, Cho Soo Young, Yu Namhee, Hong Sewha, Kim Jihyun, Kim Sunshin, Han Ji-Youn, Park Charny
2023
AS, Alternative splicing, AUCPR, the area under the precision-recall curve, AUROC, the area under the receiver operating characteristic, Alternative splicing, DAS, differential alternative splicing, Database, EMT, epithelial mesenchymal transition, Gene signature, ML, machine learning, Machine-learning, NER, named entity recognition, NLP, natural language process, PCA, principal component analysis, PSI, percent spliced in index, RF, random-forest, SF, splicing factor, TCGA, The Cancer Genome Atlas, Text-mining, Tumor transcriptome
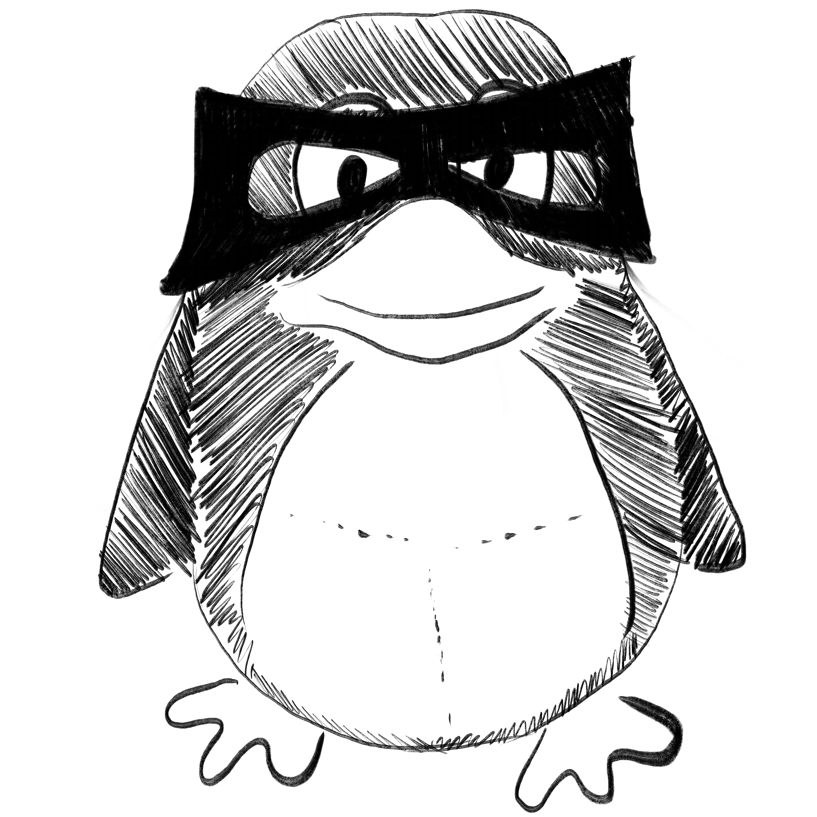
A dataset on the physiological state and behavior of drivers in conditionally automated driving.
In Data in brief
Meteier Quentin, Capallera Marine, de Salis Emmanuel, Angelini Leonardo, Carrino Stefano, Widmer Marino, Abou Khaled Omar, Mugellini Elena, Sonderegger Andreas
2023-Apr
Conditionally automated driving, Driver state, Electrocardiogram (ECG), Electrodermal activity (EDA), Physiology, Respiration, Situation awareness (SA), Takeover quality
Weekly Summary
Receive a weekly summary and discussion of the top papers of the week by leading researchers in the field.