Receive a weekly summary and discussion of the top papers of the week by leading researchers in the field.
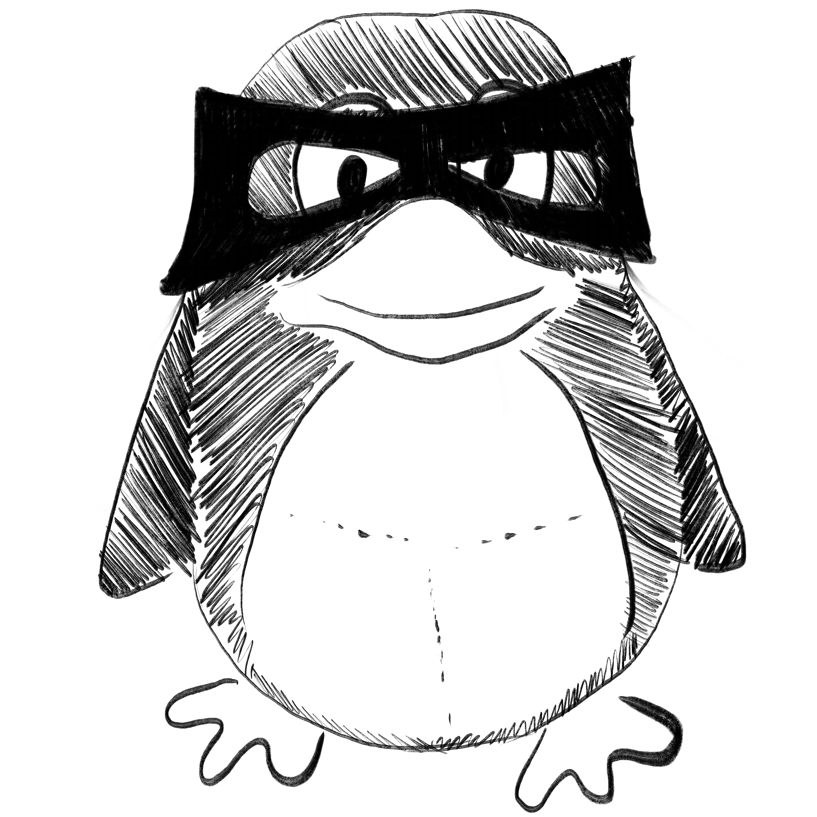
Combining Machine Learning with Physical Knowledge in Thermodynamic Modeling of Fluid Mixtures.
In Annual review of chemical and biomolecular engineering
Jirasek Fabian, Hasse Hans
2023-Mar-21
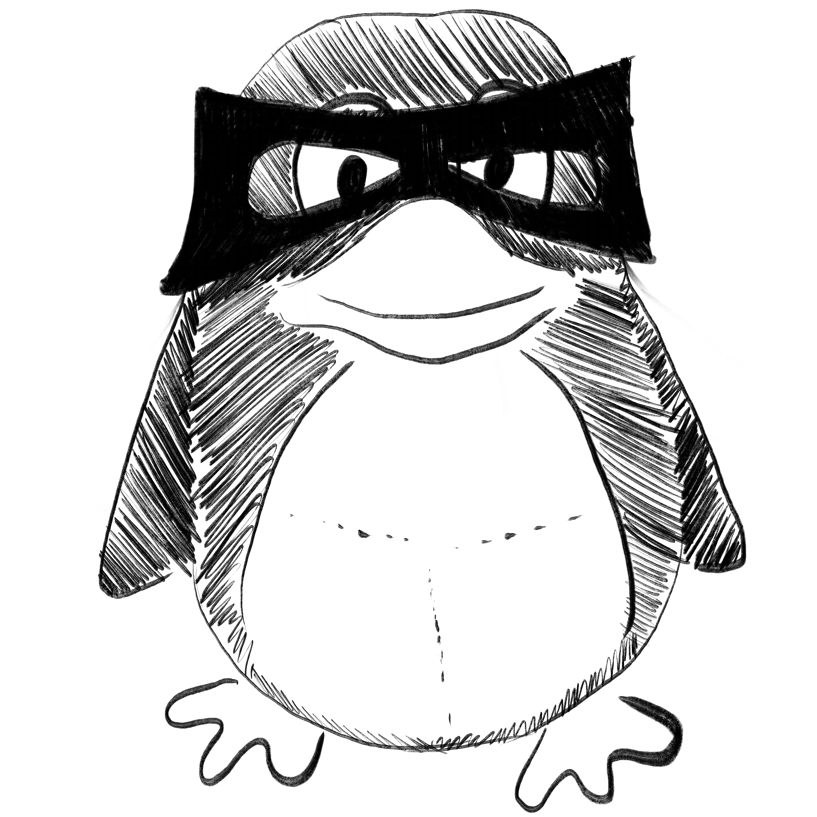
Decoding study-independent mind-wandering from EEG using convolutional neural networks.
In Journal of neural engineering ; h5-index 52.0
Jin Christina Yi, Borst Jelmer P, van Vugt Marieke
2023-Mar-21
EEG, classifier, convolutional neural network, generalizability, machine learning, meta-learner, mind-wandering
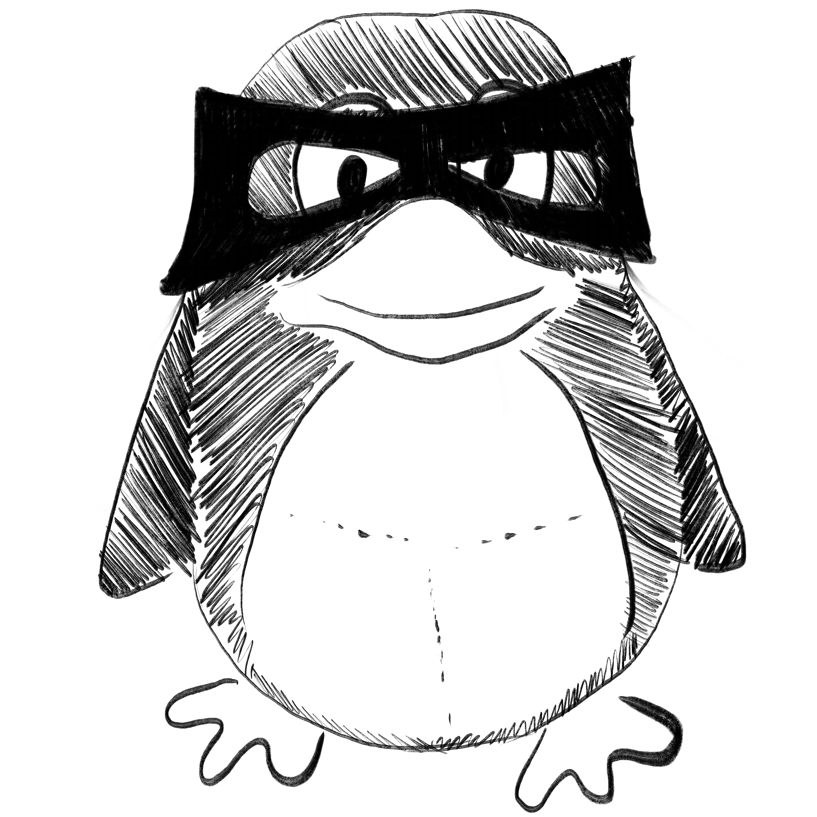
Modern Artificial Neural Networks: Is Evolution Cleverer?
In Neural computation
Bahmer Andreas, Gupta Daya, Effenberger Felix
2023-Mar-16
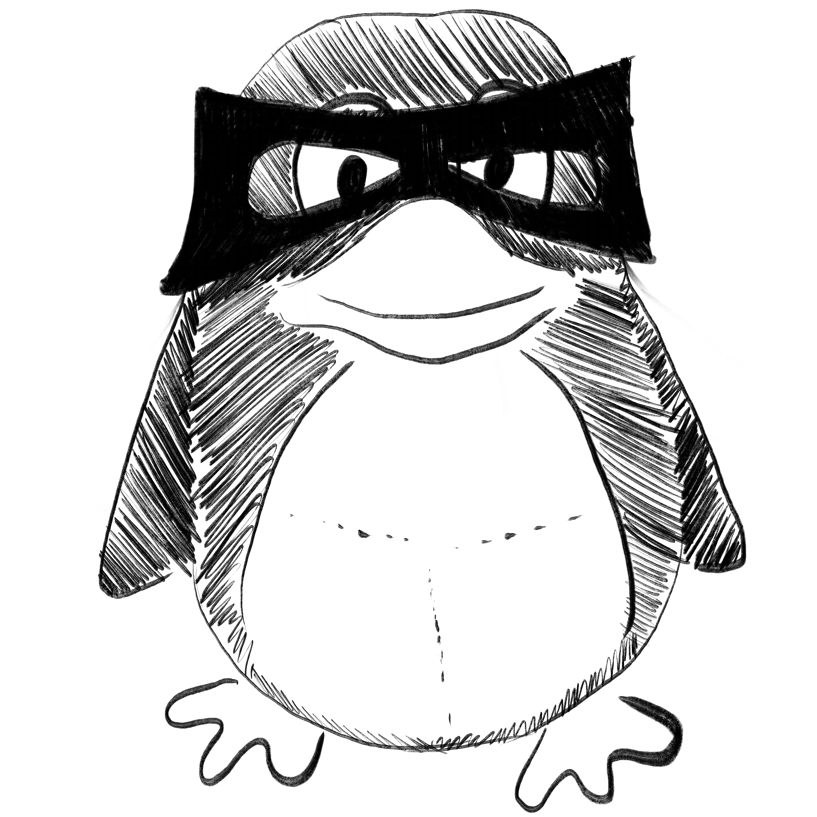
Classification of Parkinson's disease with dementia using phase locking factor of event-related oscillations to visual and auditory stimuli.
In Journal of neural engineering ; h5-index 52.0
Tülay Emine Elif, Yıldırım Ebru, Aktürk Tuba, Güntekin Bahar
2023-Mar-21
Classification, Delta, Theta, Inter-trial phase coherence, Linear Discriminant Analysis, “Parkinsons Disease with dementia”
Combination Use of Compressed Sensing and Deep Learning for Shoulder Magnetic Resonance Imaging With Various Sequences.
In Journal of computer assisted tomography
OBJECTIVE :
METHODS :
RESULTS :
CONCLUSIONS :
Shiraishi Kaori, Nakaura Takeshi, Uetani Hiroyuki, Nagayama Yasunori, Kidoh Masafumi, Kobayashi Naoki, Morita Kosuke, Yamahita Yuichi, Miyamoto Takeshi, Hirai Toshinori
2023-Mar-09
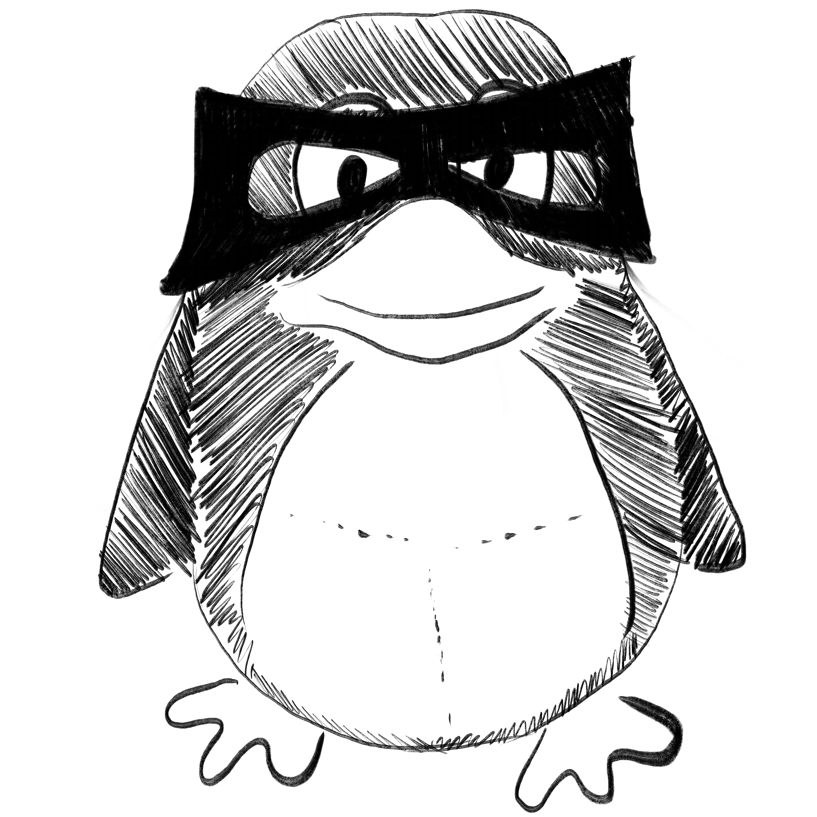
Using artificial intelligence to support rapid, mixed-methods analysis: Developing an automated qualitative assistant (AQUA).
In Annals of family medicine
Lennon Robert, Calo William, Miller Erin, Zgierska Aleksandra, Van Scoy Lauren, Fraleigh Robert
2022-Apr-01
Weekly Summary
Receive a weekly summary and discussion of the top papers of the week by leading researchers in the field.