Receive a weekly summary and discussion of the top papers of the week by leading researchers in the field.
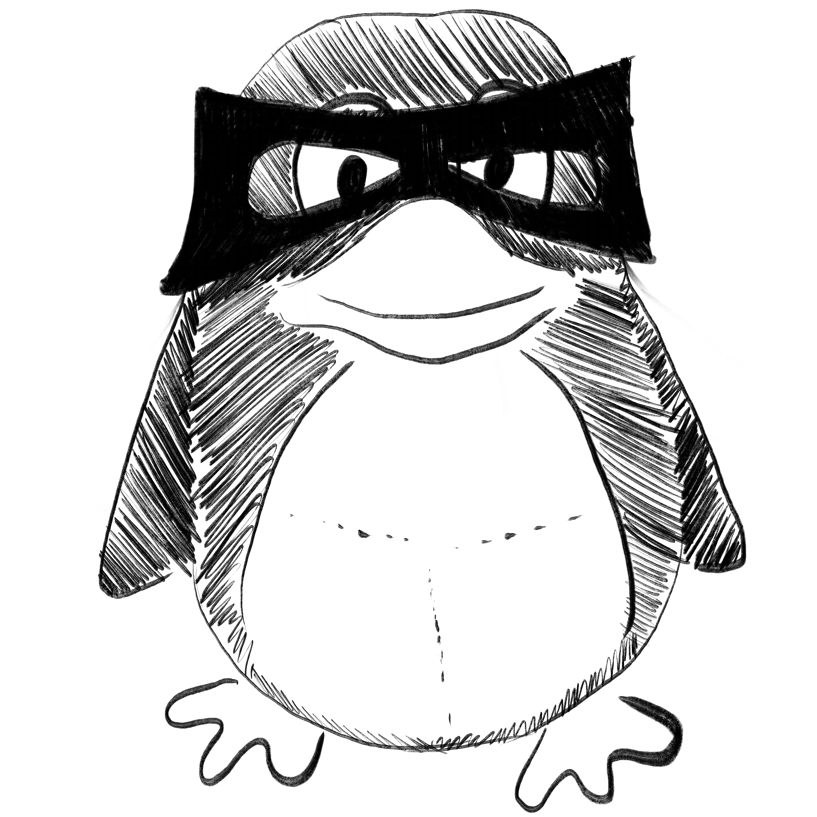
Smartphone-Tracked Digital Markers of Momentary Subjective Stress in College Students: Idiographic Machine Learning Analysis.
In JMIR mHealth and uHealth
BACKGROUND :
OBJECTIVE :
METHODS :
RESULTS :
CONCLUSIONS :
Aalbers George, Hendrickson Andrew T, Vanden Abeele Mariek Mp, Keijsers Loes
2023-Mar-23
digital biomarker, digital phenotype, machine learning, mobile health, mobile phone, personalized models
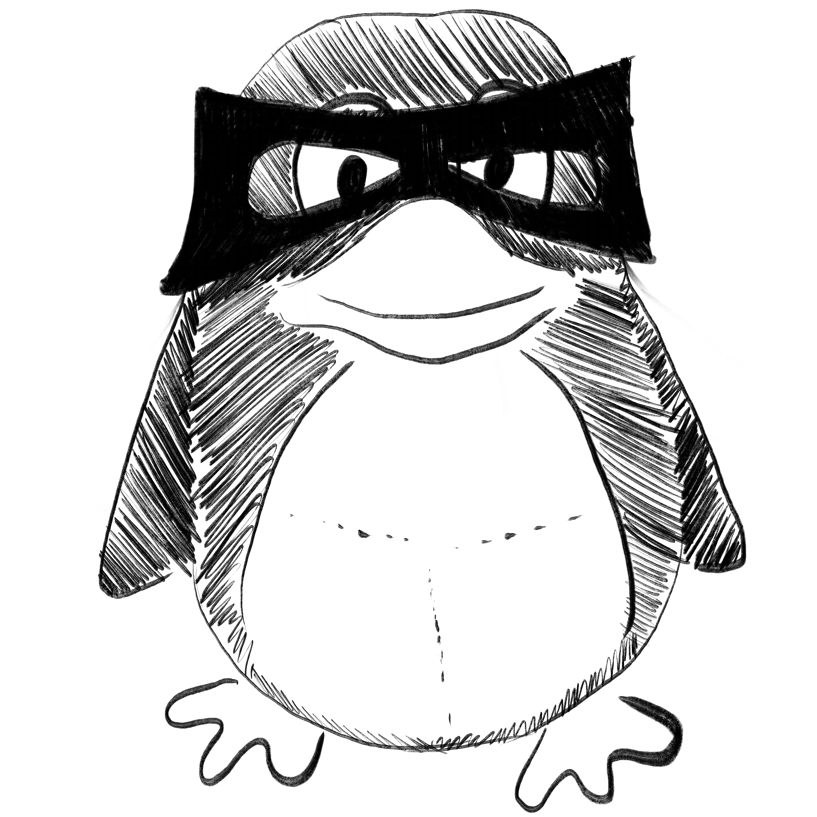
Acoustic Analysis of Speech for Screening for Suicide Risk: Machine Learning Classifiers for Between- and Within-Person Evaluation of Suicidality.
In Journal of medical Internet research ; h5-index 88.0
BACKGROUND :
OBJECTIVE :
METHODS :
RESULTS :
CONCLUSIONS :
Min Sooyeon, Shin Daun, Rhee Sang Jin, Park C Hyung Keun, Yang Jeong Hun, Song Yoojin, Kim Min Ji, Kim Kyungdo, Cho Won Ik, Kwon Oh Chul, Ahn Yong Min, Lee Hyunju
2023-Mar-23
artificial intelligence, digital health tool, mood disorder, prediction, screening, suicide, voice analysis
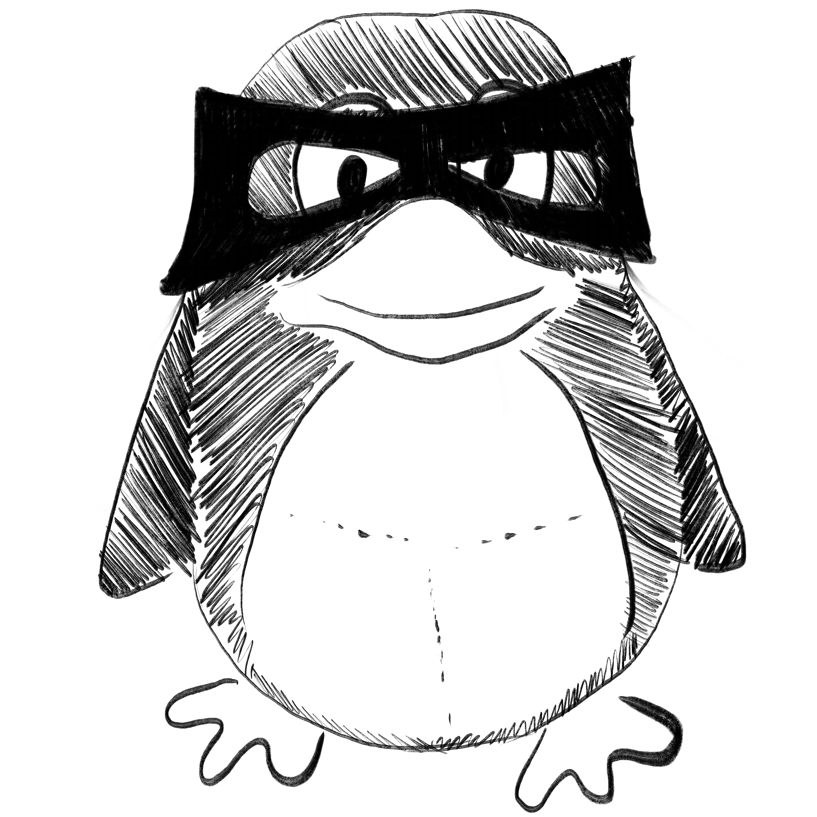
Modular Soft Robot with Origami Skin for Versatile Applications.
In Soft robotics
Jin Tao, Wang Tianhong, Xiong Quan, Tian Yingzhong, Li Long, Zhang Quan, Yeow Chen-Hua
2023-Mar-23
modular actuation, modular soft robot, origami, soft pneumatic actuators
Multidelay MR Arterial Spin Labeling Perfusion Map for the Prediction of Cerebral Hyperperfusion After Carotid Endarterectomy.
In Journal of magnetic resonance imaging : JMRI
BACKGROUND :
PURPOSE :
STUDY TYPE :
SUBJECTS :
FIELD STRENGTH/SEQUENCE :
ASSESSMENT :
STATISTICAL TESTS :
RESULTS :
DATA CONCLUSIONS :
EVIDENCE LEVEL :
Fan Xiaoyuan, Lai Zhichao, Lin Tianye, Li Kang, Hou Bo, You Hui, Wei Juan, Qu Jianxun, Liu Bao, Zuo Zhentao, Feng Feng
2023-Mar-23
arterial spin labeling, carotid endarterectomy, carotid stenosis, cerebral hyperperfusion syndrome
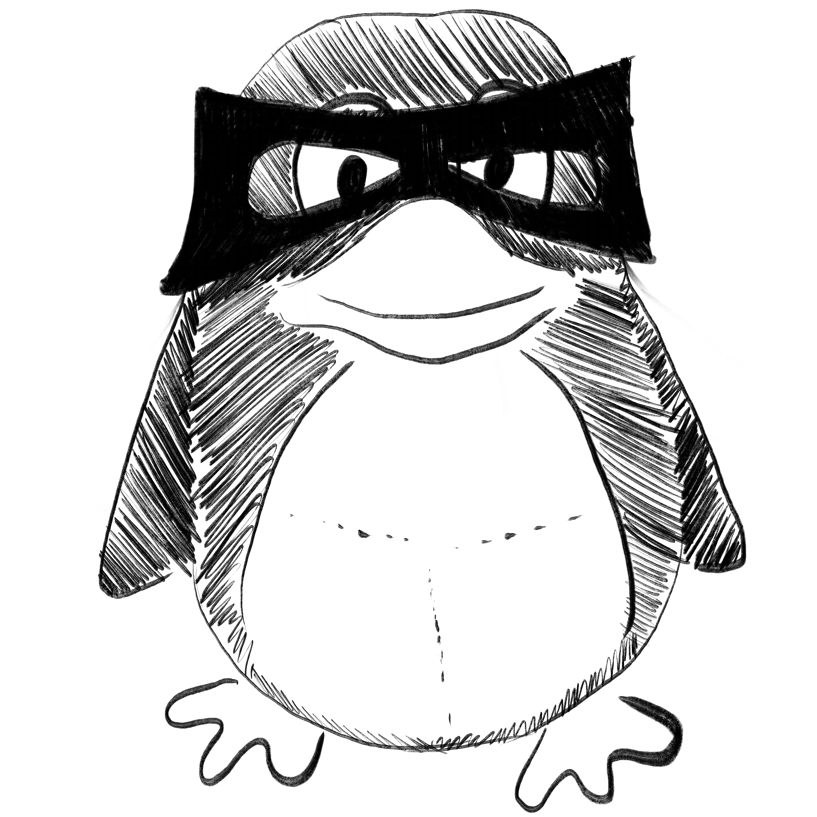
EBi-LSTM: an enhanced bi-directional LSTM for time-series data classification by heuristic development of optimal feature integration in brain computer interface.
In Computer methods in biomechanics and biomedical engineering
Saraswat Mala, Dubey Anil Kumar
2023-Mar-23
Time-series data classification, brain–computer interface, electroencephalography signal, enhanced bi-directional long short-term memory, ensemble feature extraction, probability ratio-based reptile search algorithm
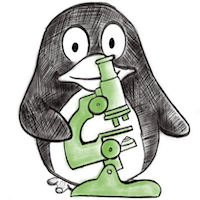
Whole Slide Images in Artificial Intelligence Applications in Digital Pathology: Challenges and Pitfalls.
In Turk patoloji dergisi
Basak Kayhan, Ozyoruk Kutsev Bengisu, Demir Derya
2023-Mar-23
Weekly Summary
Receive a weekly summary and discussion of the top papers of the week by leading researchers in the field.