Receive a weekly summary and discussion of the top papers of the week by leading researchers in the field.
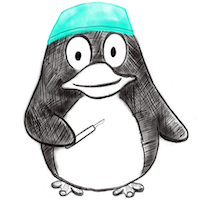
Using a New Deep Learning Method for 3D Cephalometry in Patients With Cleft Lip and Palate.
In The Journal of craniofacial surgery
Xu Meng, Liu Bingyang, Luo Zhaoyang, Ma Hengyuan, Sun Min, Wang Yongqian, Yin Ningbei, Tang Xiaojun, Song Tao
2023-Mar-22
Impact of inactivated COVID-19 vaccines on lung injury in B.1.617.2 (Delta) variant-infected patients.
In Annals of clinical microbiology and antimicrobials
BACKGROUND :
METHODS :
RESULTS :
CONCLUSION :
Lai Miao, Wang Kai, Ding Chengyuan, Yin Yi, Lin Xiaoling, Xu Chuanjun, Hu Zhiliang, Peng Zhihang
2023-Mar-21
Artificial intelligence (AI), COVID-19, COVID-19 vaccines, Chest CT, Lung injury
Compositional Zero-Shot Domain Transfer with Text-to-Text Models
ArXiv Preprint
Fangyu Liu, Qianchu Liu, Shruthi Bannur, Fernando Pérez-García, Naoto Usuyama, Sheng Zhang, Tristan Naumann, Aditya Nori, Hoifung Poon, Javier Alvarez-Valle, Ozan Oktay, Stephanie L. Hyland
2023-03-23
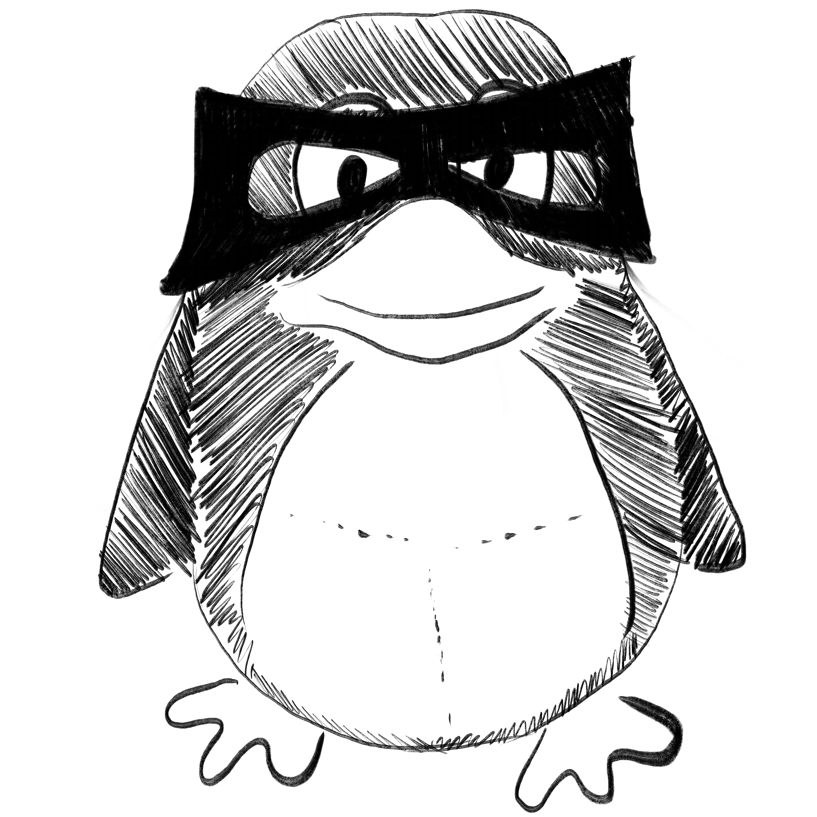
Fault Prognosis of Turbofan Engines: Eventual Failure Prediction and Remaining Useful Life Estimation
ArXiv Preprint
Joseph Cohen, Xun Huan, Jun Ni
2023-03-23
Mesoporous nanodrug delivery system: a powerful tool for a new paradigm of remodeling of the tumor microenvironment.
In Journal of nanobiotechnology
Hang Yinhui, Liu Yanfang, Teng Zhaogang, Cao Xiongfeng, Zhu Haitao
2023-Mar-21
Mesoporous nanodrug delivery systems, Metabolism, Targeted remodeling, Tumor microenvironment, Tumor therapy
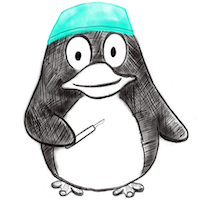
Radiomics nomogram for preoperative differentiation of pulmonary mucinous adenocarcinoma from tuberculoma in solitary pulmonary solid nodules.
In BMC cancer
OBJECTIVE :
METHOD :
RESULTS :
CONCLUSION :
Zhang Junjie, Hao Ligang, Qi MingWei, Xu Qian, Zhang Ning, Feng Hui, Shi Gaofeng
2023-Mar-21
Contrast-enhanced CT, Mucinous adenocarcinoma, Nomogram, Radiomics, Tuberculoma
Weekly Summary
Receive a weekly summary and discussion of the top papers of the week by leading researchers in the field.