Receive a weekly summary and discussion of the top papers of the week by leading researchers in the field.
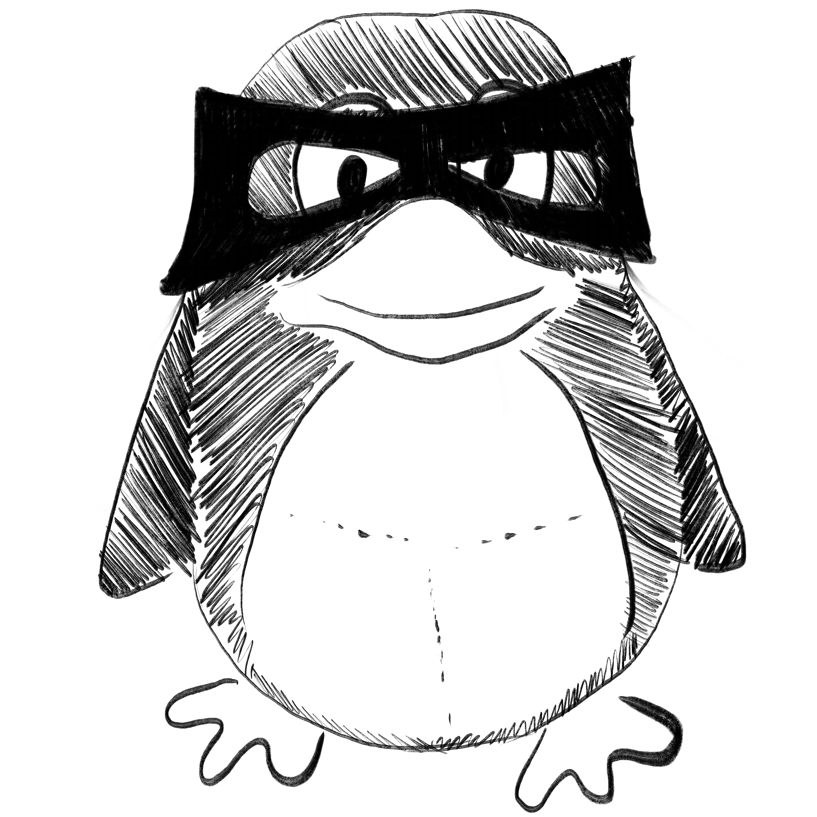
Interpreting Generative Adversarial Networks to Infer Natural Selection from Genetic Data.
In bioRxiv : the preprint server for biology
MOTIVATION :
RESULTS :
AVAILABILITY :
Riley Rebecca, Mathieson Iain, Mathieson Sara
2023-Mar-08
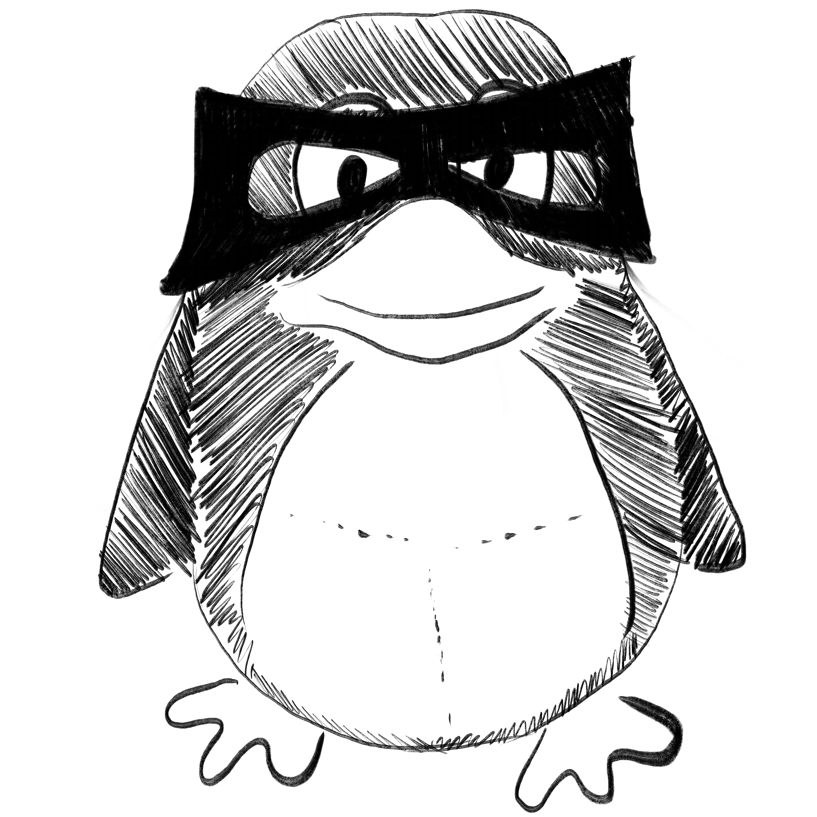
Comparative study of convolutional neural network architectures for gastrointestinal lesions classification.
In PeerJ
Cuevas-Rodriguez Erik O, Galvan-Tejada Carlos E, Maeda-Gutiérrez Valeria, Moreno-Chávez Gamaliel, Galván-Tejada Jorge I, Gamboa-Rosales Hamurabi, Luna-García Huizilopoztli, Moreno-Baez Arturo, Celaya-Padilla José María
2023
Classification, Computer-aided diagnostic, Convolutional neural network, Deep learning, Endoscopy, Gastrointestinal, Gastrointestinal lesions
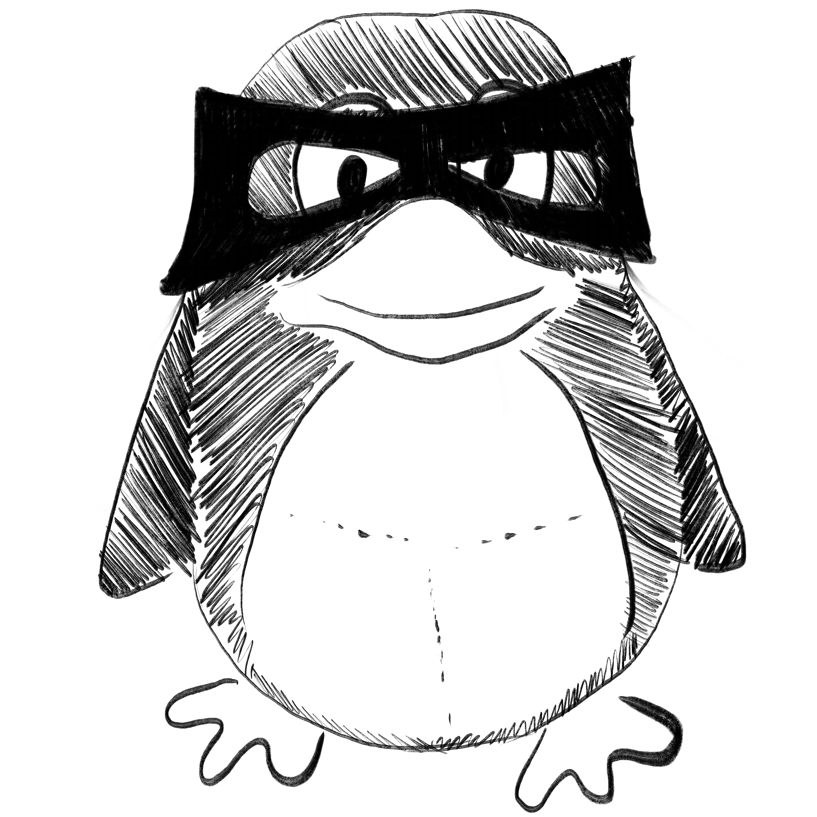
A ventilation early warning system (VEWS) for diaphanous workspaces considering COVID-19 and future pandemics scenarios.
In Heliyon
Costa Gonçal, Arroyo Oriol, Rueda Pablo, Briones Alan
2023-Mar
BIM, Building digital twin, COVID-19, Facilities management, IoT, Simulation, Smart building
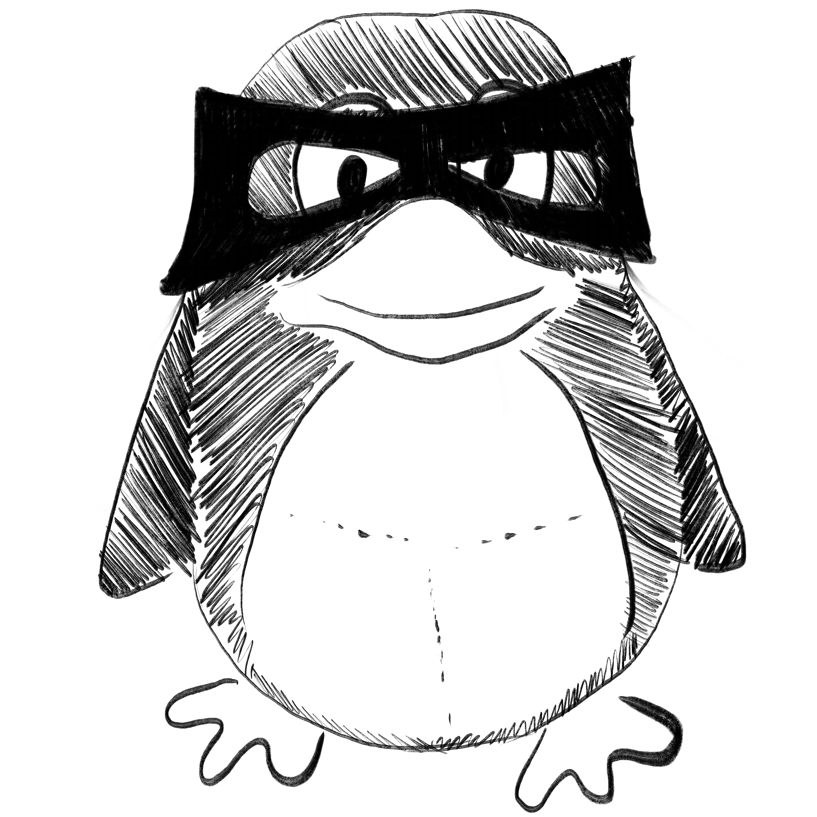
COVID-19 diagnosis: A comprehensive review of pre-trained deep learning models based on feature extraction algorithm.
In Results in engineering
Poola Rahul Gowtham, Pl Lahari, Y Siva Sankar
2023-Jun
Boundary tracing, Covid diagnosis, Deep transfer-learning, Medical imaging, Neural network models and classifiers
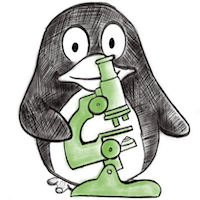
AMLnet, A deep-learning pipeline for the differential diagnosis of acute myeloid leukemia from bone marrow smears.
In Journal of hematology & oncology ; h5-index 60.0
Yu Zebin, Li Jianhu, Wen Xiang, Han Yingli, Jiang Penglei, Zhu Meng, Wang Minmin, Gao Xiangli, Shen Dan, Zhang Ting, Zhao Shuqi, Zhu Yijing, Tong Jixiang, Yuan Shuchong, Zhu HongHu, Huang He, Qian Pengxu
2023-Mar-21
Acute myeloid leukemia, Bone marrow smears, Deep learning, Diagnosis
Serum immuno-oncology markers carry independent prognostic information in patients with newly diagnosed metastatic breast cancer, from a prospective observational study.
In Breast cancer research : BCR
BACKGROUND :
PATIENTS AND METHODS :
RESULTS :
CONCLUSION :
Gunnarsdottir Frida Björk, Bendahl Pär-Ola, Johansson Alexandra, Benfeitas Rui, Rydén Lisa, Bergenfelz Caroline, Larsson Anna-Maria
2023-Mar-21
Immuno-oncology, Marker, Metastatic breast cancer, Serum, Survival
Weekly Summary
Receive a weekly summary and discussion of the top papers of the week by leading researchers in the field.