Receive a weekly summary and discussion of the top papers of the week by leading researchers in the field.
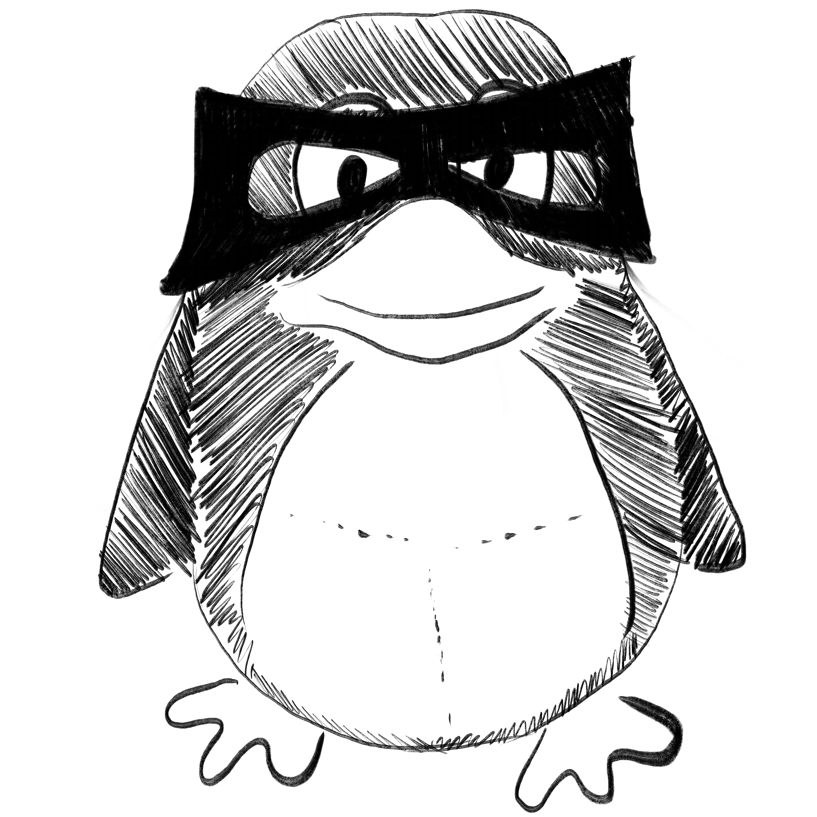
Forecast-Aware Model Driven LSTM
ArXiv Preprint
Sophia Hamer, Jennifer Sleeman, Ivanka Stajner
2023-03-23
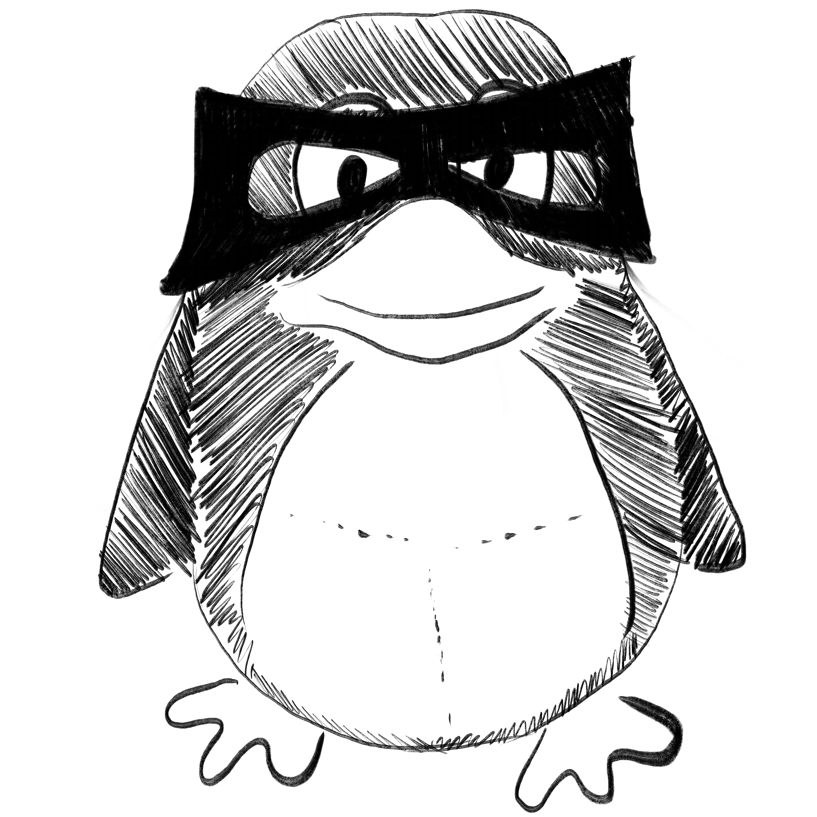
Human Behavior in the Time of COVID-19: Learning from Big Data
ArXiv Preprint
Hanjia Lyu, Arsal Imtiaz, Yufei Zhao, Jiebo Luo
2023-03-23
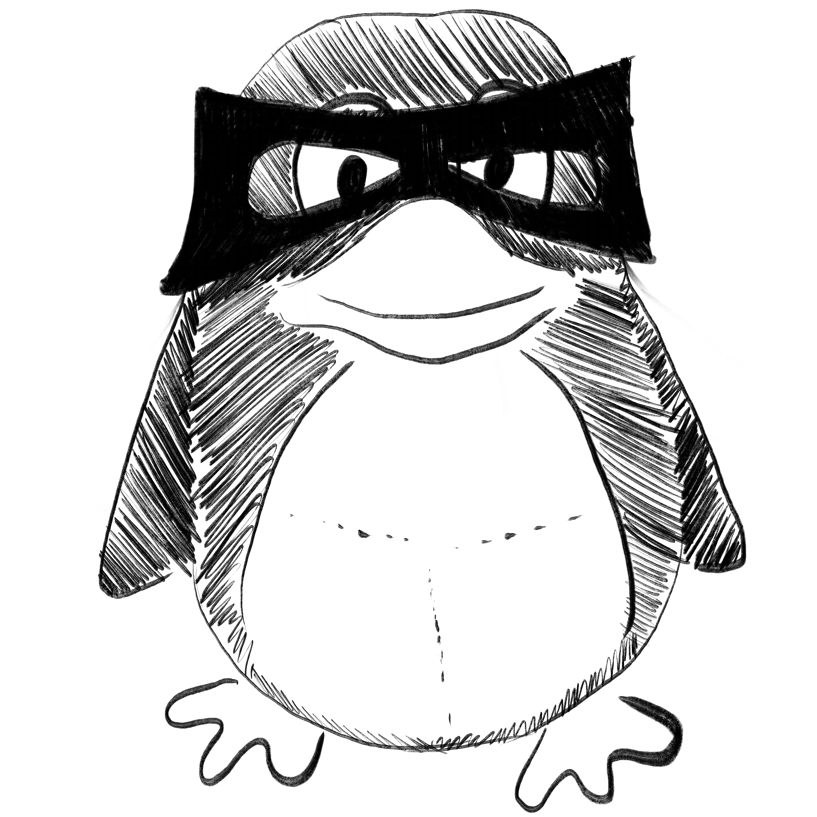
Natural language processing models reveal neural dynamics of human conversation.
In bioRxiv : the preprint server for biology
Cai Jing, Hadjinicolaou Alex E, Paulk Angelique C, Williams Ziv M, Cash Sydney S
2023-Mar-11
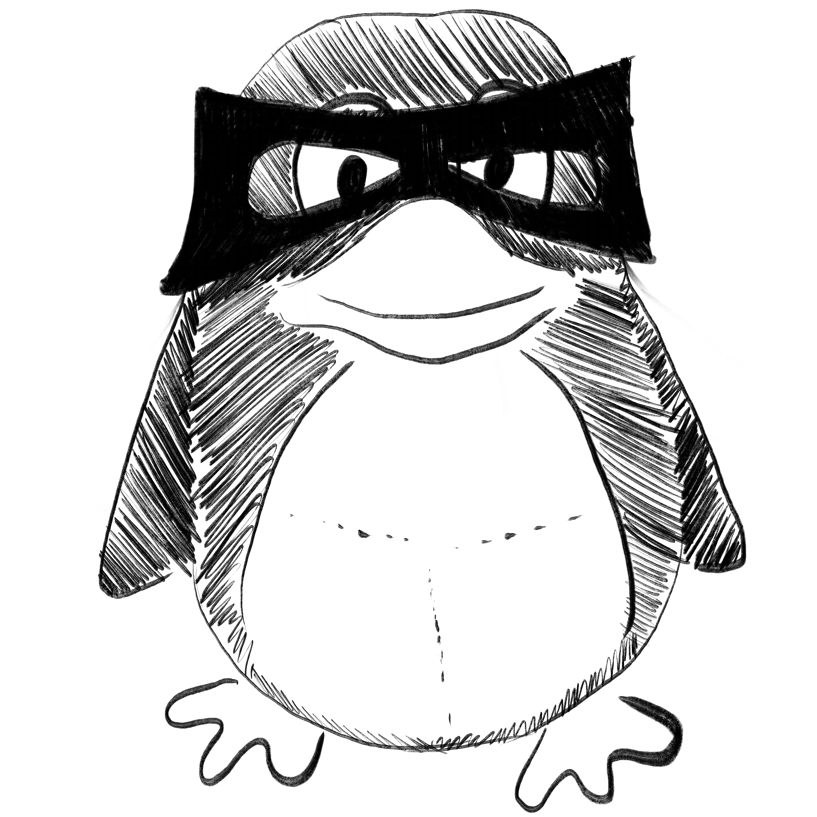
Digitally Diagnosing Multiple Developmental Delays using Crowdsourcing fused with Machine Learning: A Research Protocol.
In medRxiv : the preprint server for health sciences
BACKGROUND :
OBJECTIVE :
METHODS :
CONCLUSIONS :
Washington Peter
2023-Mar-07
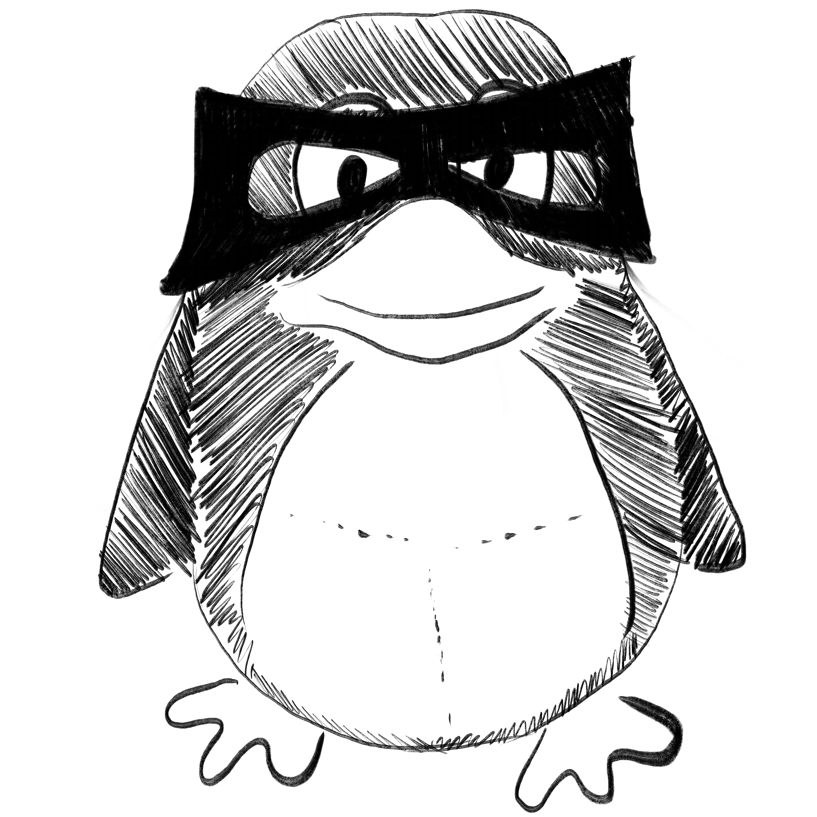
Conserved cysteine residues in Kaposi's sarcoma herpesvirus ORF34 are necessary for viral production and viral pre-initiation complex formation.
In bioRxiv : the preprint server for biology
UNLABELLED :
IMPORTANCE :
Watanabe Tadashi, Narahari Akshara, Bhardwaj Esha, Kuriyama Kazushi, Nishimura Mayu, Izumi Taisuke, Fujimuro Masahiro, Ohno Shinji
2023-Mar-09
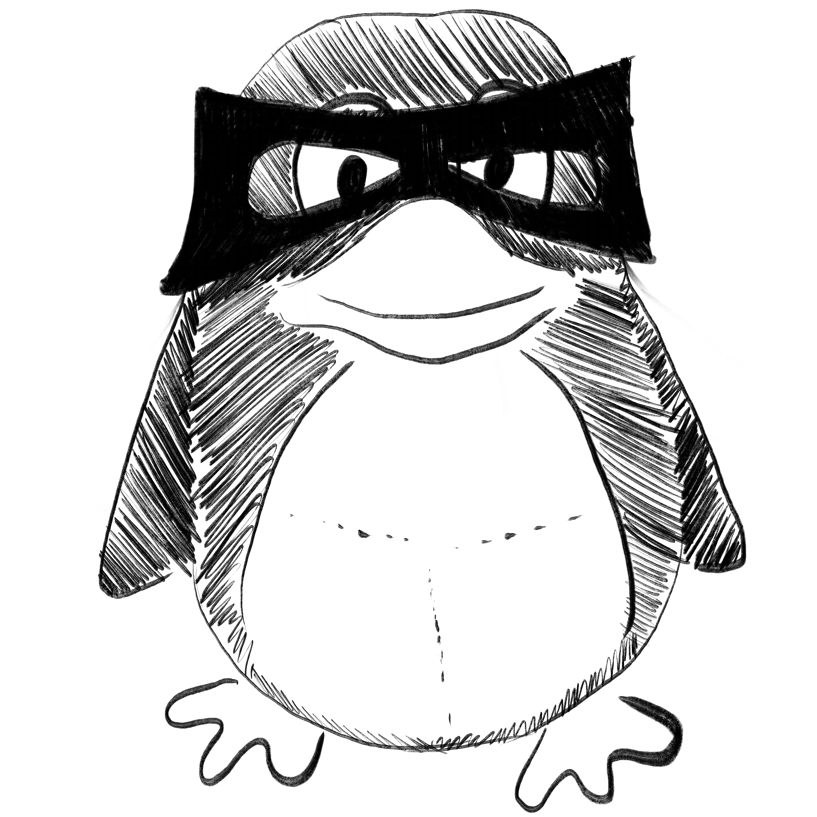
Aberrant phase separation is a common killing strategy of positively charged peptides in biology and human disease.
In bioRxiv : the preprint server for biology
Boeynaems Steven, Ma X Rosa, Yeong Vivian, Ginell Garrett M, Chen Jian-Hua, Blum Jacob A, Nakayama Lisa, Sanyal Anushka, Briner Adam, Van Haver Delphi, Pauwels Jarne, Ekman Axel, Schmidt H Broder, Sundararajan Kousik, Porta Lucas, Lasker Keren, Larabell Carolyn, Hayashi Mirian A F, Kundaje Anshul, Impens Francis, Obermeyer Allie, Holehouse Alex S, Gitler Aaron D
2023-Mar-09
Weekly Summary
Receive a weekly summary and discussion of the top papers of the week by leading researchers in the field.