Receive a weekly summary and discussion of the top papers of the week by leading researchers in the field.
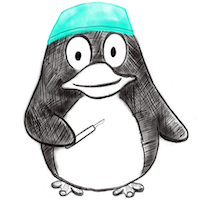
Computer Vision Analysis of Specimen Mammography to Predict Margin Status.
In medRxiv : the preprint server for health sciences
Chen Kevin A, Kirchoff Kathryn E, Butler Logan R, Holloway Alexa D, Kapadia Muneera R, Gallagher Kristalyn K, Gomez Shawn M
2023-Mar-08
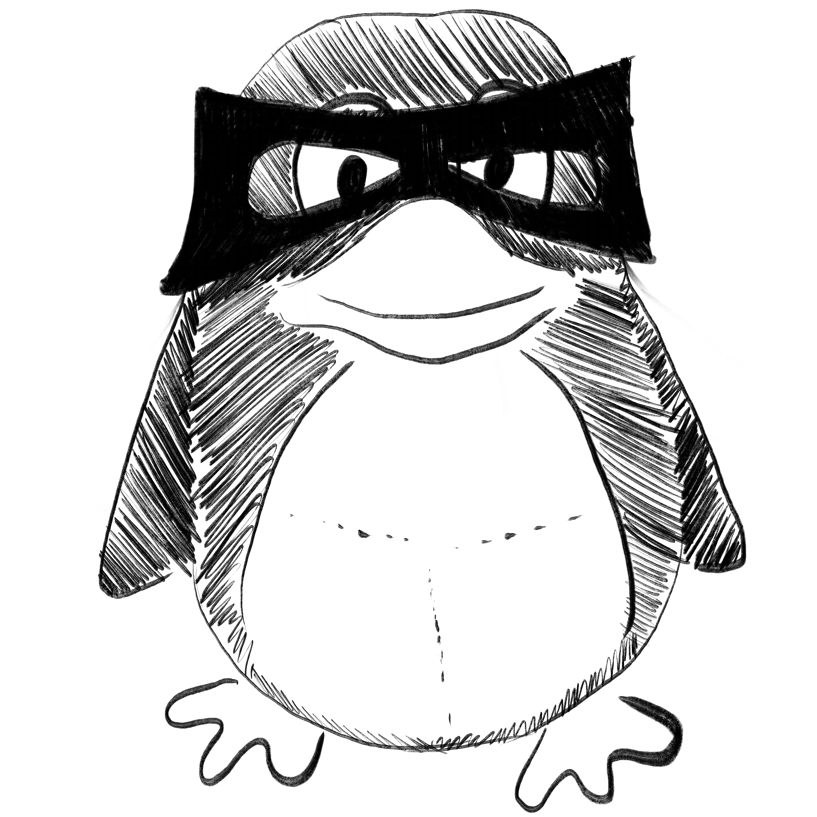
Sequence characteristics and an accurate model of high-occupancy target loci in the human genome.
In bioRxiv : the preprint server for biology
Hudaiberdiev Sanjarbek, Ovcharenko Ivan
2023-Feb-05
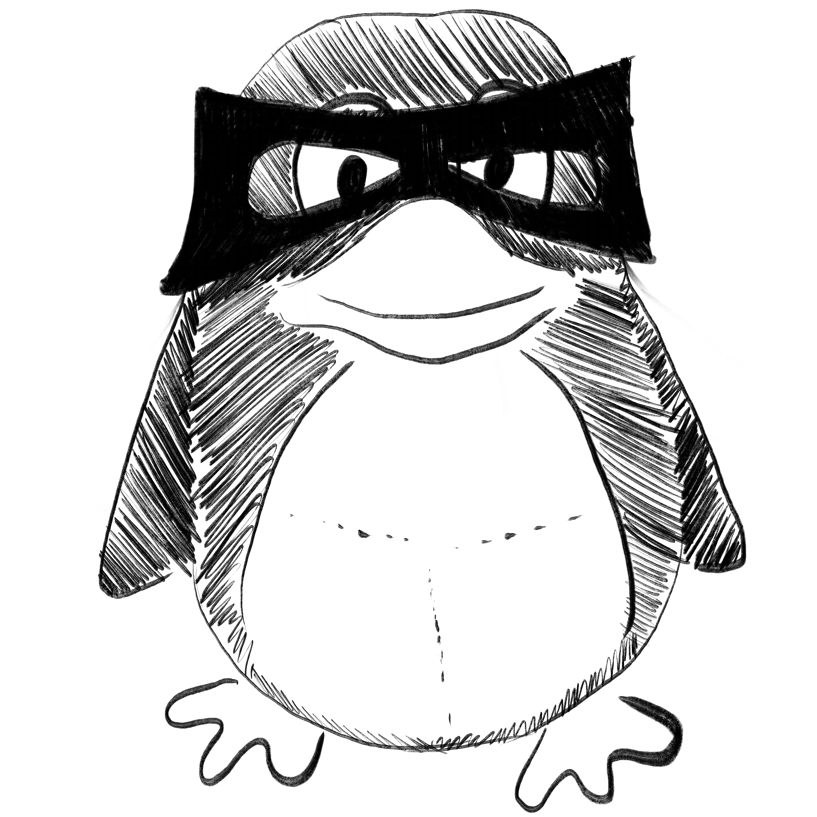
MOVER: Medical Informatics Operating Room Vitals and Events Repository.
In medRxiv : the preprint server for health sciences
Samad Muntaha, Rinehart Joseph, Angel Mirana, Kanomata Yuzo, Baldi Pierre, Cannesson Maxime
2023-Mar-12
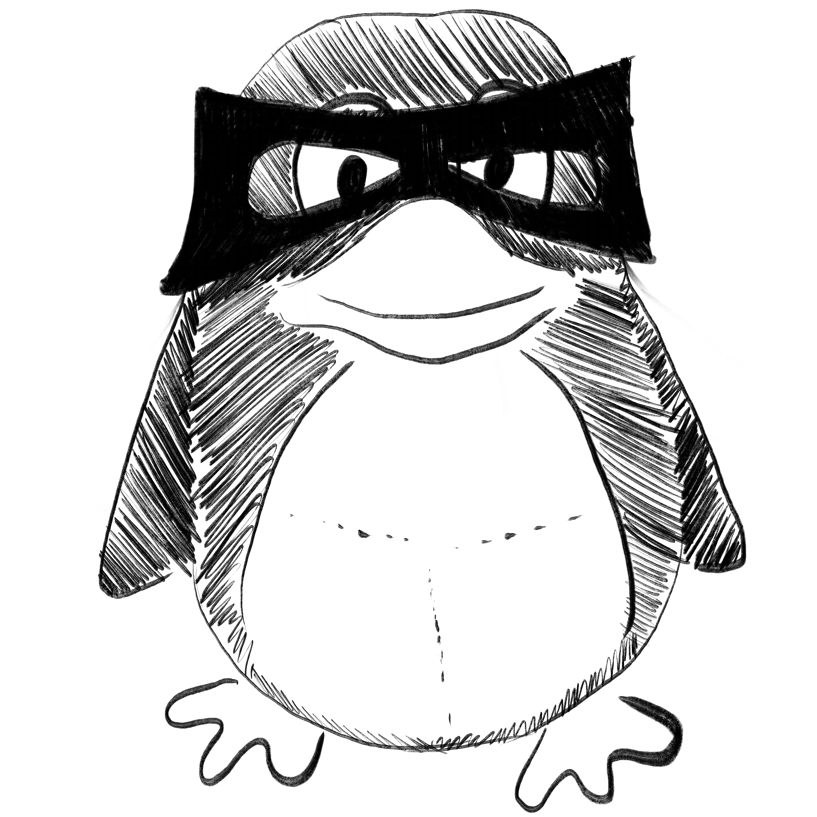
Preterm Preeclampsia Risk Modelling: Examining Hemodynamic, Biochemical, and Biophysical Markers Prior to Pregnancy.
In medRxiv : the preprint server for health sciences
UNLABELLED :
CLINICAL RELEVANCE :
Loftness Bryn C, Bernstein Ira, McBride Carole A, Cheney Nick, McGinnis Ellen W, McGinnis Ryan S
2023-Mar-06
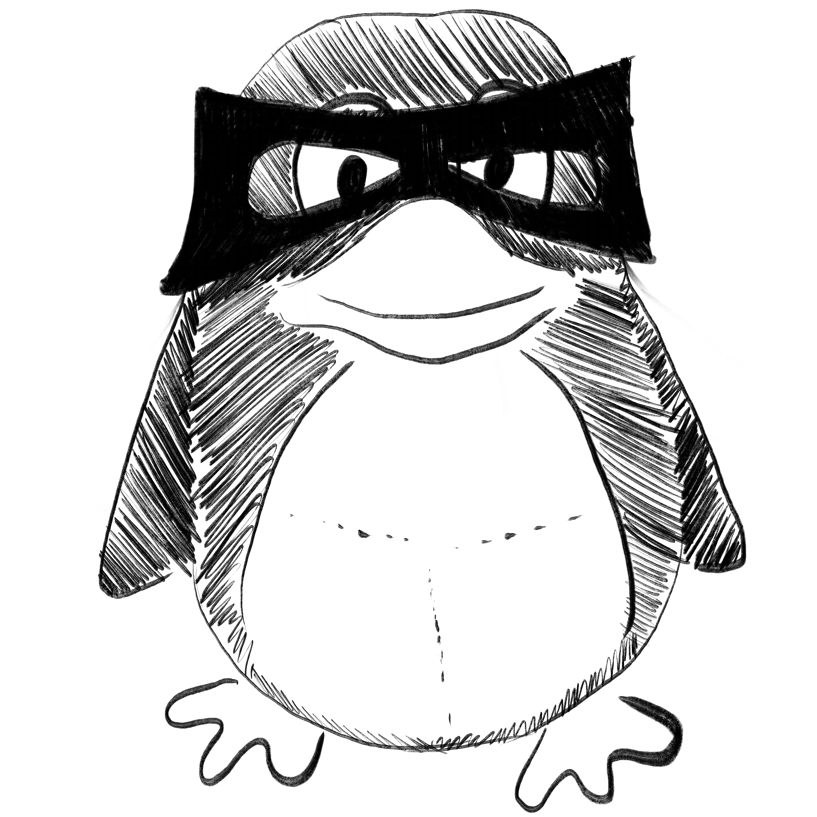
Synthesize Extremely High-dimensional Longitudinal Electronic Health Records via Hierarchical Autoregressive Language Model.
In Research square
Theodorou Brandon, Xiao Cao, Sun Jimeng
2023-Mar-10
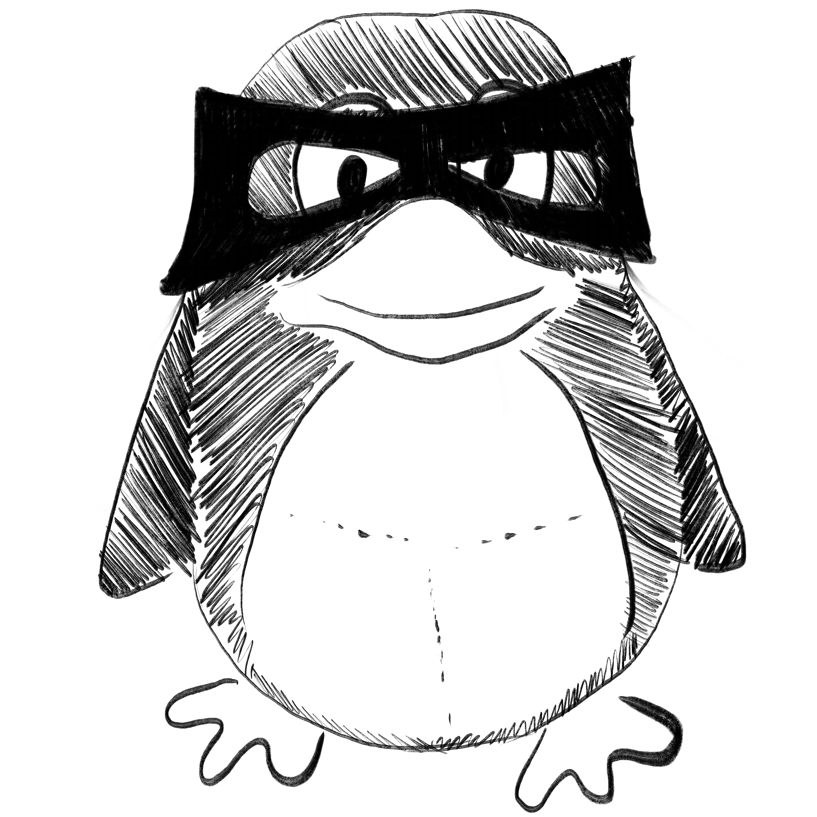
Direct prediction of Homologous Recombination Deficiency from routine histology in ten different tumor types with attention-based Multiple Instance Learning: a development and validation study.
In medRxiv : the preprint server for health sciences
BACKGROUND :
METHODS :
RESULTS :
CONCLUSION :
Lavinia Loeffler Chiara Maria, El Nahhas Omar S M, Muti Hannah Sophie, Seibel Tobias, Cifci Didem, van Treeck Marko, Gustav Marco, Carrero Zunamys I, Gaisa Nadine T, Lehmann Kjong-Van, Leary Alexandra, Selenica Pier, Reis-Filho Jorge S, Bruechle Nadina Ortiz, Kather Jakob Nikolas
2023-Mar-10
Weekly Summary
Receive a weekly summary and discussion of the top papers of the week by leading researchers in the field.