Receive a weekly summary and discussion of the top papers of the week by leading researchers in the field.
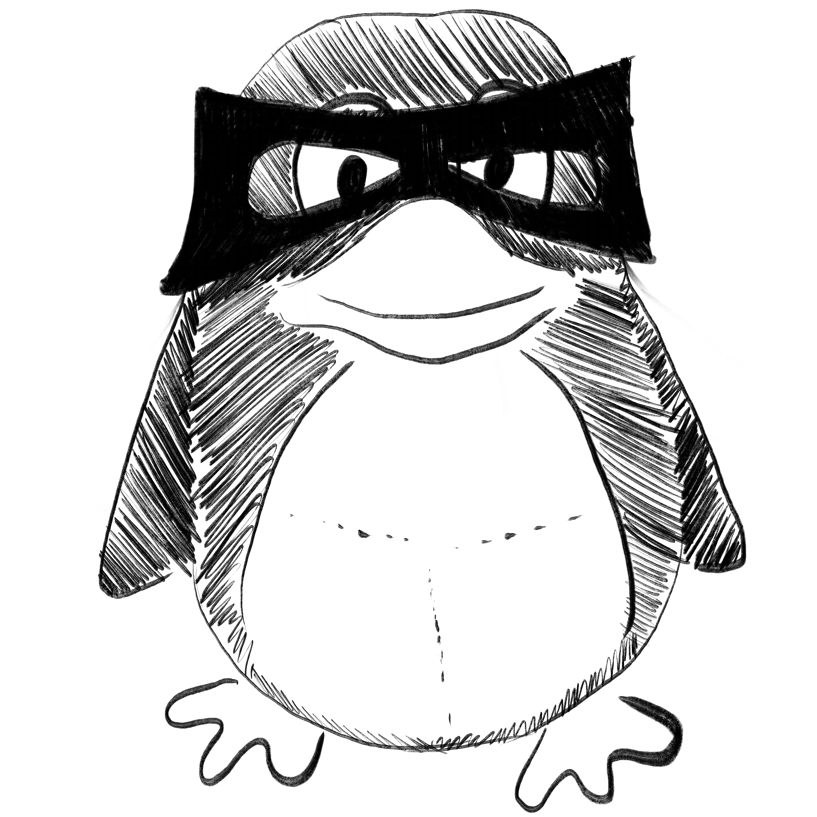
AI Moral Enhancement: Upgrading the Socio-Technical System of Moral Engagement.
In Science and engineering ethics
Volkman Richard, Gabriels Katleen
2023-Mar-23
AI socratic interlocutor, Artificial intelligence, Artificial moral agent, Moral enhancement
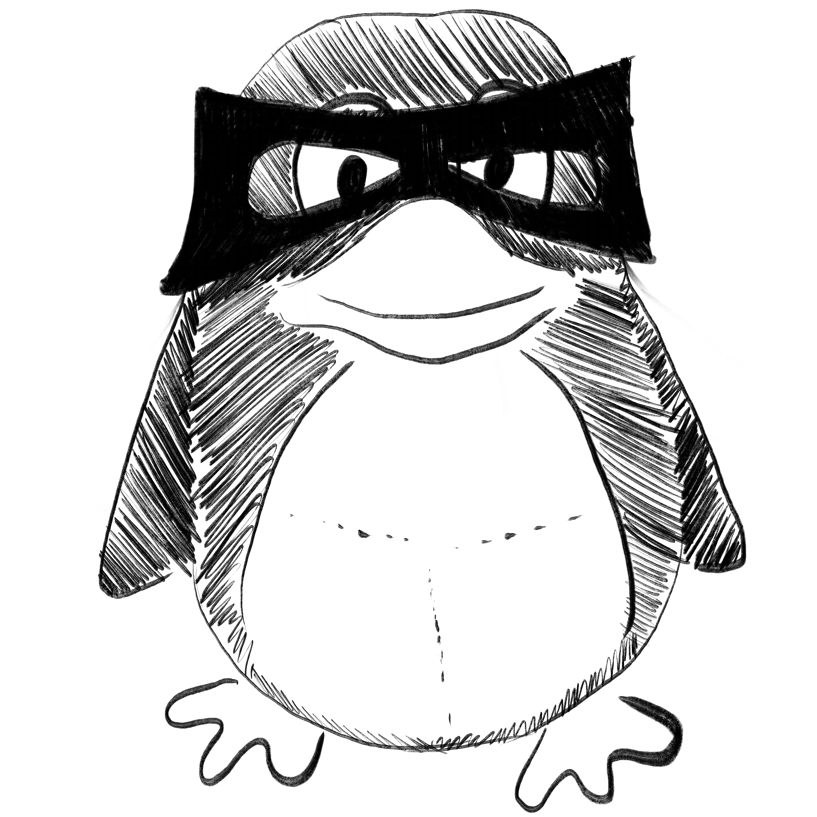
Predicting epileptic seizures based on EEG signals using spatial depth features of a 3D-2D hybrid CNN.
In Medical & biological engineering & computing ; h5-index 32.0
Qi Nan, Piao Yan, Yu Peng, Tan Baolin
2023-Mar-23
3D-2D hybrid CNN, EEG, Epilepsy, Seizure prediction
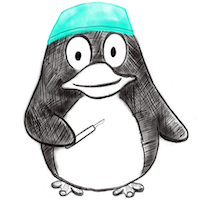
Effectiveness of high-flow nasal cannulae compared with noninvasive positive-pressure ventilation in preventing reintubation in patients receiving prolonged mechanical ventilation.
In Scientific reports ; h5-index 158.0
Tseng Chi-Wei, Chao Ke-Yun, Wu Hsiu-Li, Lin Chen-Chun, Hsu Han-Shui
2023-Mar-22
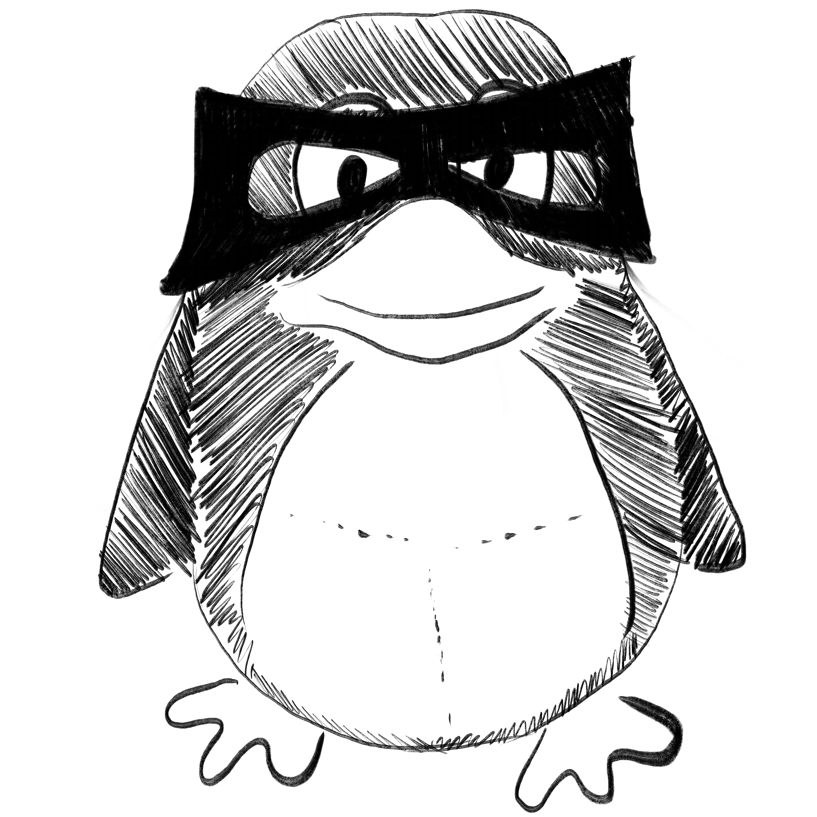
Machine Ethics: Do Androids Dream of Being Good People?
In Science and engineering ethics
Génova Gonzalo, Moreno Valentín, González M Rosario
2023-Mar-23
Artificial intelligence, Computability, Intentional action, Machine ethics, Moral codes of conduct, Rationality of ethics
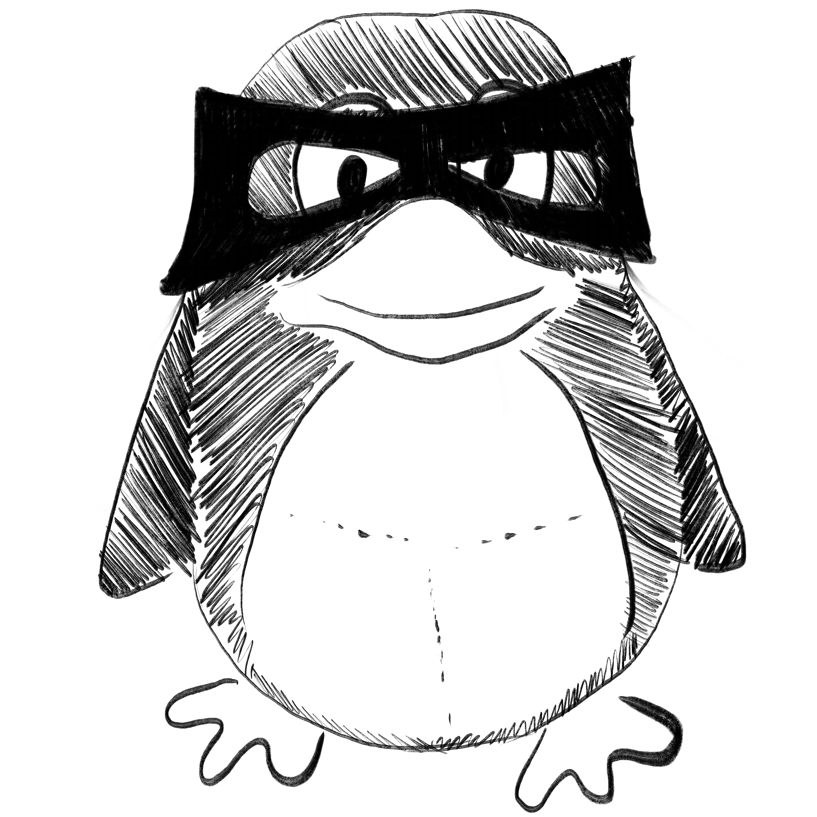
A device-independent method for the colorimetric quantification on microfluidic sensors using a color adaptation algorithm.
In Mikrochimica acta
Feng Junjie, Jiang Huiyun, Jin Yan, Rong Shenghui, Wang Shiqiang, Wang Haozhi, Wang Lin, Xu Wei, Sun Bing
2023-Mar-23
Colorimetry, Image processing, Microfluidic sensors, Paper-based analytical devices, Smartphone
Understanding Public Attitudes and Willingness to Share Commercial Data for Health Research: Survey Study in the United Kingdom.
In JMIR public health and surveillance
BACKGROUND :
OBJECTIVE :
METHODS :
RESULTS :
CONCLUSIONS :
Hirst Yasemin, Stoffel Sandro T, Brewer Hannah R, Timotijevic Lada, Raats Monique M, Flanagan James M
2023-Mar-23
acceptability, commercial data, data, data donation, data sharing, digital, health, loyalty cards, mobile phone, participant recruitment, public, sociodemographic factors
Weekly Summary
Receive a weekly summary and discussion of the top papers of the week by leading researchers in the field.