Receive a weekly summary and discussion of the top papers of the week by leading researchers in the field.
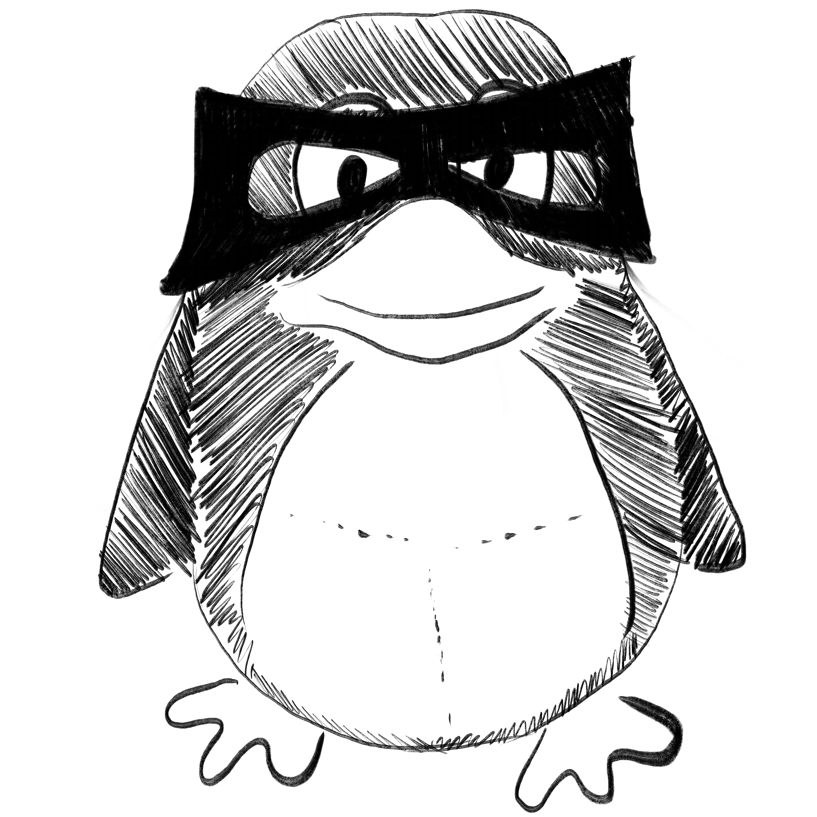
Right Ventricular Sarcomere Contractile Depression and the Role of Thick Filament Activation in Human Heart Failure with Pulmonary Hypertension.
In bioRxiv : the preprint server for biology
RATIONALE :
OBJECTIVE :
METHODS AND RESULTS :
CONCLUSIONS :
Jani Vivek, Aslam M Imran, Fenwick Axel J, Ma Weikang, Gong Henry, Milburn Gregory, Nissen Devin, Salazar Ilton Cubero, Hanselman Olivia, Mukherjee Monica, Halushka Marc K, Margulies Kenneth B, Campbell Kenneth, Irving Thomas C, Kass David A, Hsu Steven
2023-Mar-12
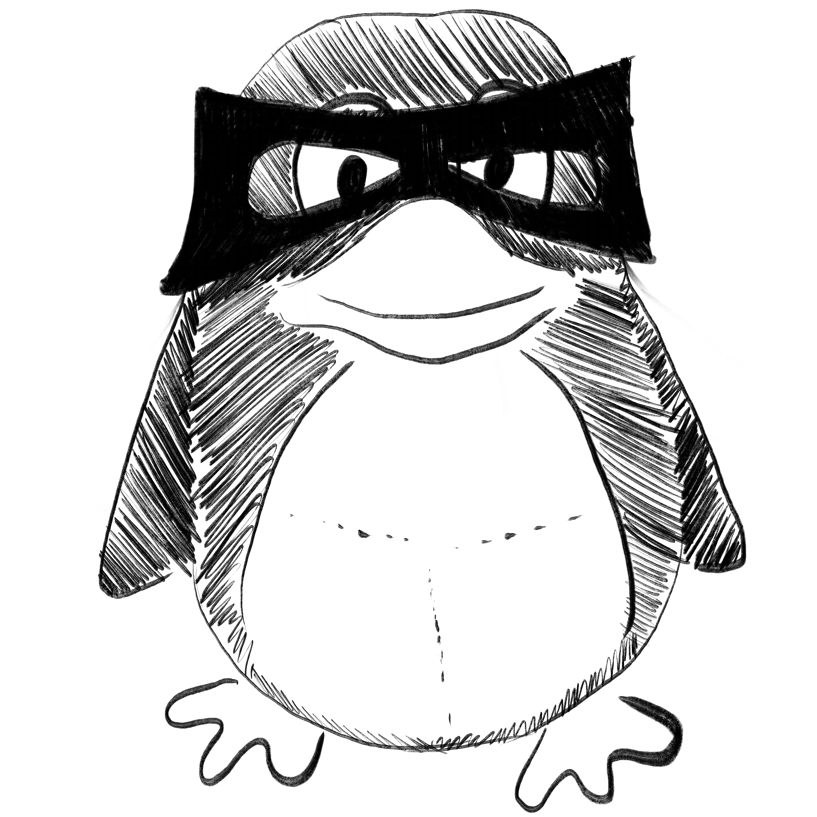
MAHOMES II: A webserver for predicting if a metal binding site is enzymatic.
In bioRxiv : the preprint server for biology
UNLABELLED :
SIGNIFICANCE STATEMENT :
Feehan Ryan, Copeland Matthew, Franklin Meghan W, Slusky Joanna S G
2023-Mar-12
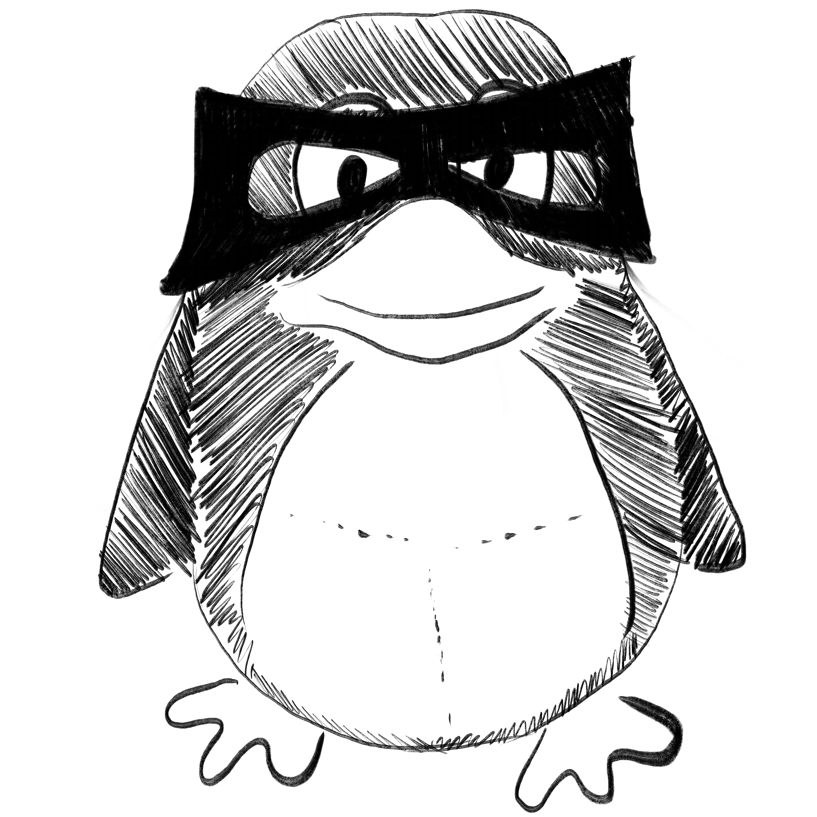
Structure-Kinetics Relationships of Opioids from Metadynamics and Machine Learning.
In bioRxiv : the preprint server for biology
Mahinthichaichan Paween, Liu Ruibin, Vo Quynh N, Ellis Christopher R, Stavitskaya Lidiya, Shen Jana
2023-Mar-07
Clinical and genetic associations of deep learning-derived cardiac magnetic resonance-based left ventricular mass.
In Nature communications ; h5-index 260.0
Khurshid Shaan, Lazarte Julieta, Pirruccello James P, Weng Lu-Chen, Choi Seung Hoan, Hall Amelia W, Wang Xin, Friedman Samuel F, Nauffal Victor, Biddinger Kiran J, Aragam Krishna G, Batra Puneet, Ho Jennifer E, Philippakis Anthony A, Ellinor Patrick T, Lubitz Steven A
2023-Mar-21
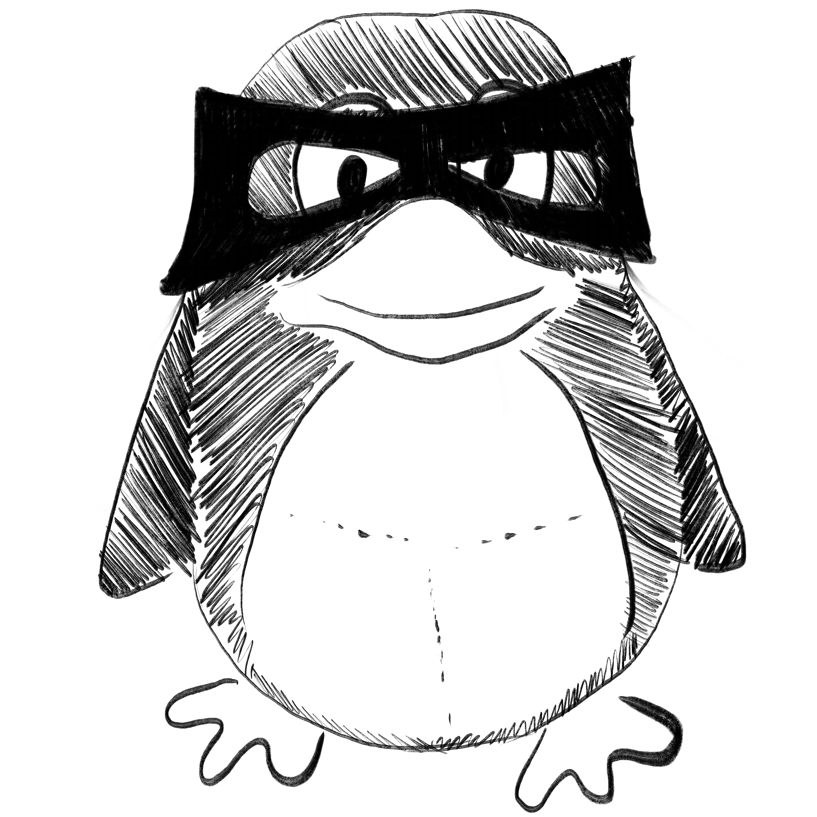
A ventilation early warning system (VEWS) for diaphanous workspaces considering COVID-19 and future pandemics scenarios.
In Heliyon
Costa Gonçal, Arroyo Oriol, Rueda Pablo, Briones Alan
2023-Mar
BIM, Building digital twin, COVID-19, Facilities management, IoT, Simulation, Smart building
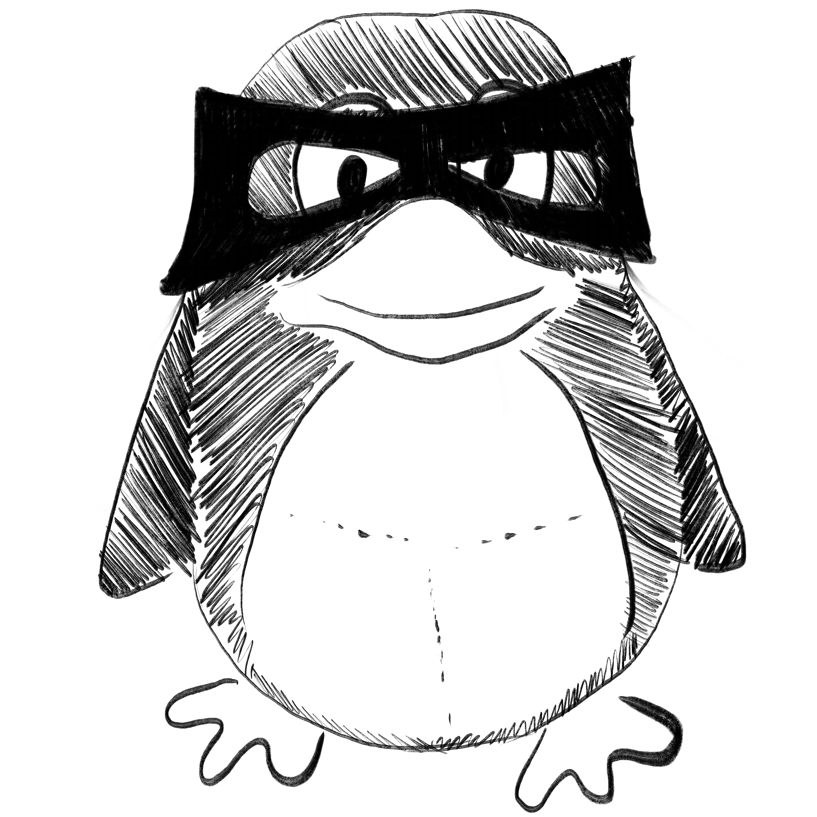
Fault Prognosis of Turbofan Engines: Eventual Failure Prediction and Remaining Useful Life Estimation
ArXiv Preprint
Joseph Cohen, Xun Huan, Jun Ni
2023-03-23
Weekly Summary
Receive a weekly summary and discussion of the top papers of the week by leading researchers in the field.