Receive a weekly summary and discussion of the top papers of the week by leading researchers in the field.
Can automated CT body composition analysis predict high-grade Clavien-Dindo complications in patients with RCC undergoing partial and radical nephrectomy?
In Scottish medical journal
INTRODUCTION :
METHODS :
RESULTS :
CONCLUSION :
Demirel Emin, Dilek Okan
2023-Mar-22
Clavien–Dindo classification, Nephrectomy, adipose, muscle, renal cell cancer, surgical complication
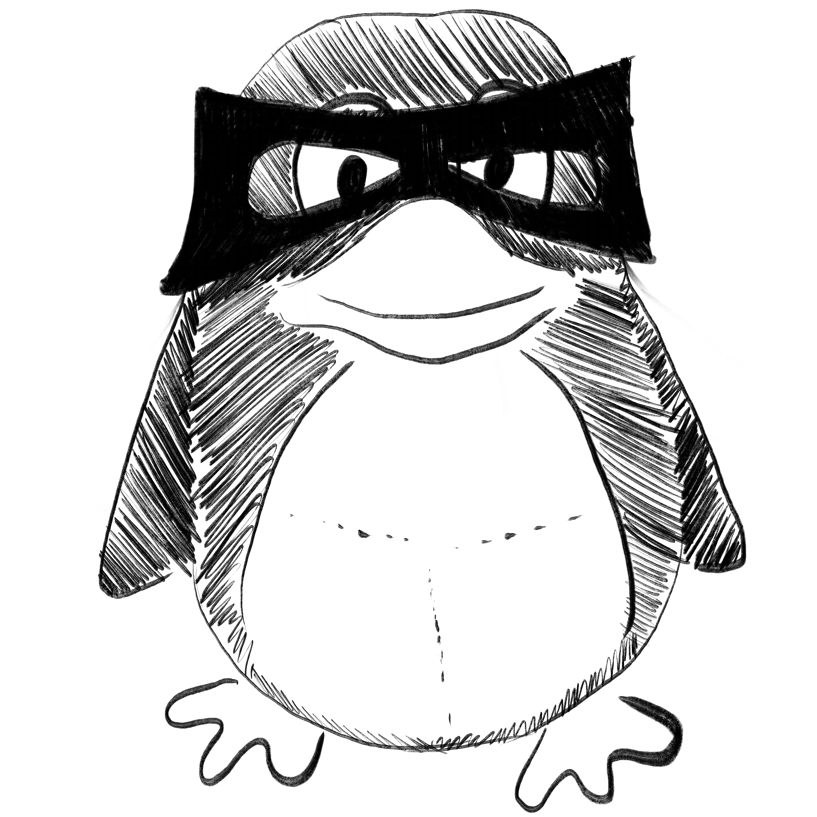
[Construction and evaluation of an artificial intelligence-based risk prediction model for death in patients with nasopharyngeal cancer].
In Nan fang yi ke da xue xue bao = Journal of Southern Medical University
OBJECTIVE :
METHODS :
RESULTS :
CONCLUSIONS :
Zhang H, Lu J, Jiang C, Fang M
2023-Feb-20
artificial intelligence, nasopharyngeal carcinoma, nomogram, predictive model, risk factors
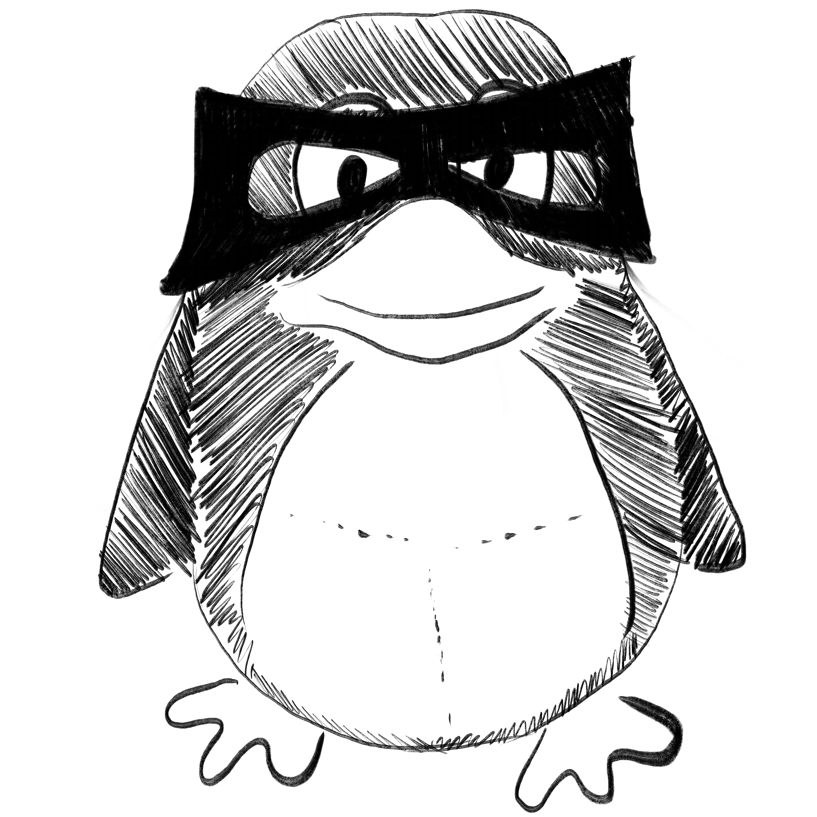
Accelerating network layouts using graph neural networks.
In Nature communications ; h5-index 260.0
Both Csaba, Dehmamy Nima, Yu Rose, Barabási Albert-László
2023-Mar-21
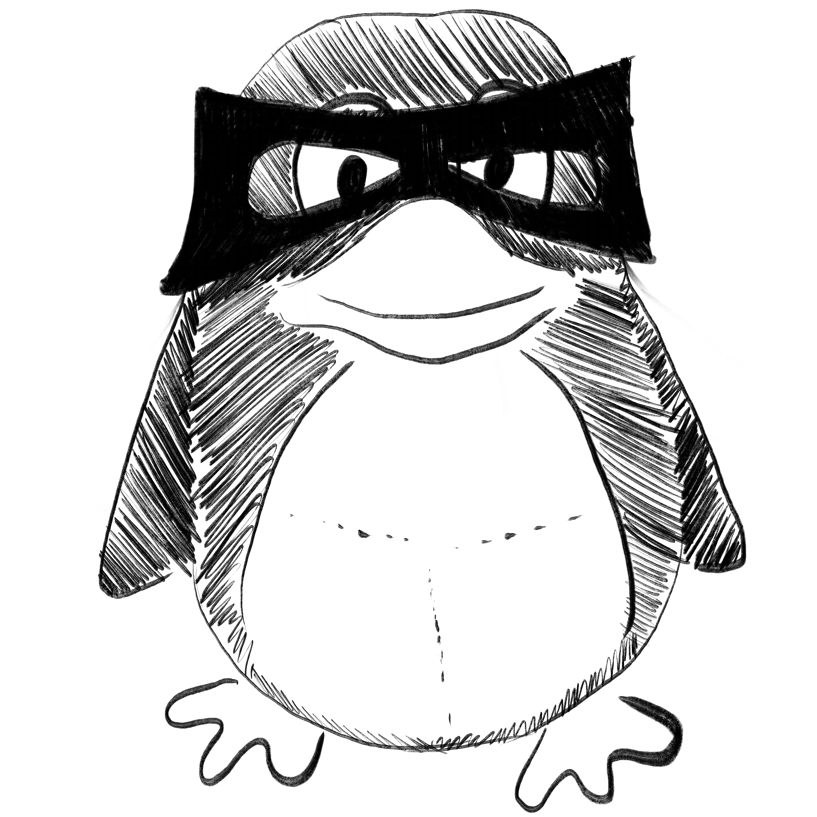
Risk Factors and Predictive Modeling for Post-Acute Sequelae of SARS-CoV-2 Infection: Findings from EHR Cohorts of the RECOVER Initiative.
In Research square
Zang Chengxi, Hou Yu, Schenck Edward, Xu Zhenxing, Zhang Yongkang, Xu Jie, Bian Jiang, Morozyuk Dmitry, Khullar Dhruv, Nordvig Anna, Shenkman Elizabeth, Rothman Russel, Block Jason, Lyman Kristin, Zhang Yiye, Varma Jay, Weiner Mark, Carton Thomas, Wang Fei, Kaushal Rainu, Consortium The Recover
2023-Mar-08
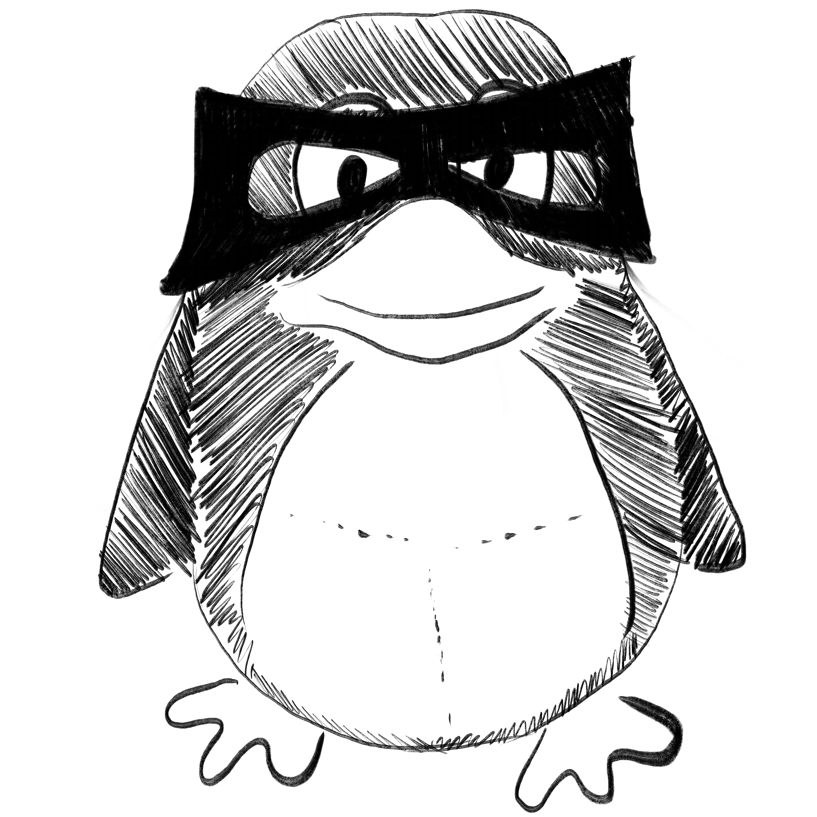
SPeC: A Soft Prompt-Based Calibration on Mitigating Performance Variability in Clinical Notes Summarization
ArXiv Preprint
Yu-Neng Chuang, Ruixiang Tang, Xiaoqian Jiang, Xia Hu
2023-03-23
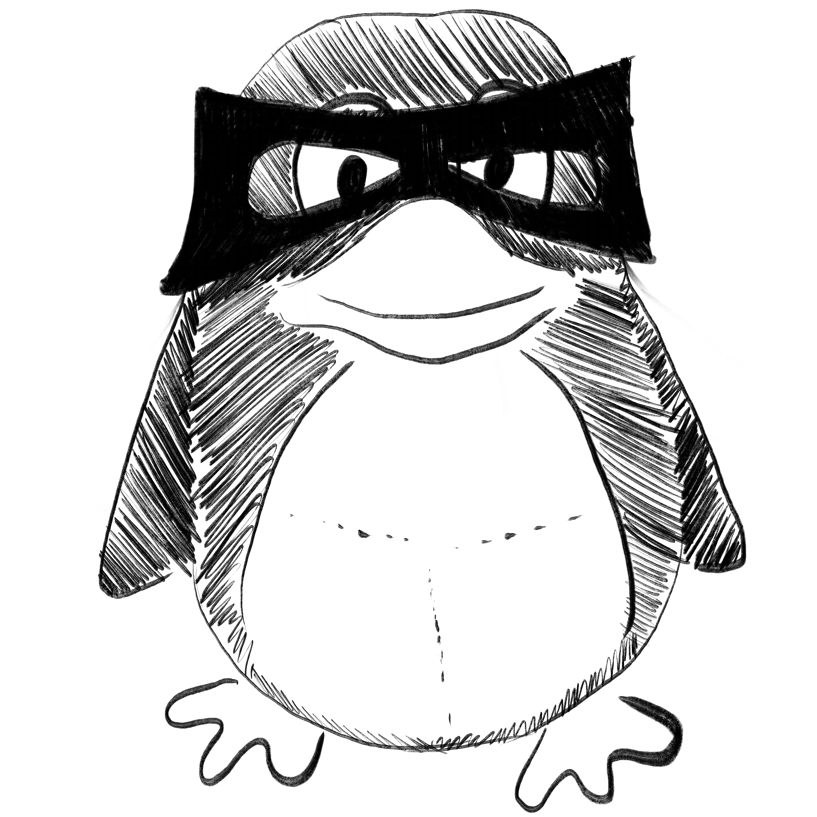
Assessing the performance of ChatGPT in answering questions regarding cirrhosis and hepatocellular carcinoma.
In Clinical and molecular hepatology
BACKGROUND & AIMS :
METHODS :
RESULTS :
CONCLUSIONS :
Yeo Yee Hui, Samaan Jamil S, Ng Wee Han, Ting Peng-Sheng, Trivedi Hirsh, Vipani Aarshi, Ayoub Walid, Yang Ju Dong, Liran Omer, Spiegel Brennan, Kuo Alexander
2023-Mar-22
accuracy, artificial intelligence, health literacy, patient knowledge, reproducibility
Weekly Summary
Receive a weekly summary and discussion of the top papers of the week by leading researchers in the field.