Receive a weekly summary and discussion of the top papers of the week by leading researchers in the field.
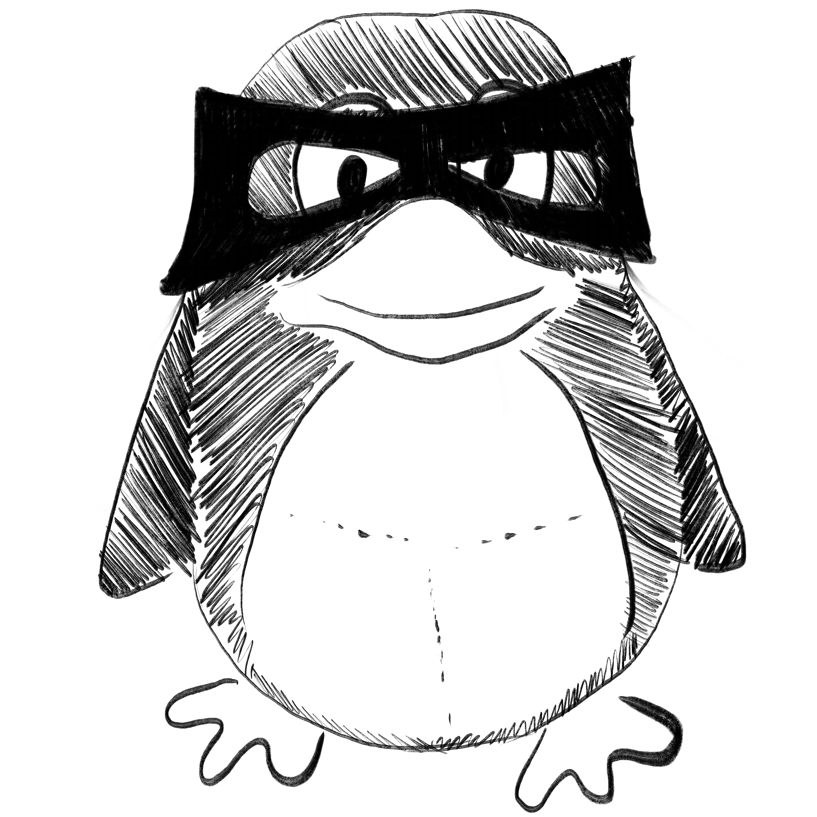
Self-healing bottlebrush polymer networks enabled via a side-chain interlocking design.
In Materials horizons
Xiong Hui, Yue Tongkui, Wu Qi, Zhang Linjun, Xie Zhengtian, Liu Jun, Zhang Liqun, Wu Jinrong
2023-Mar-22
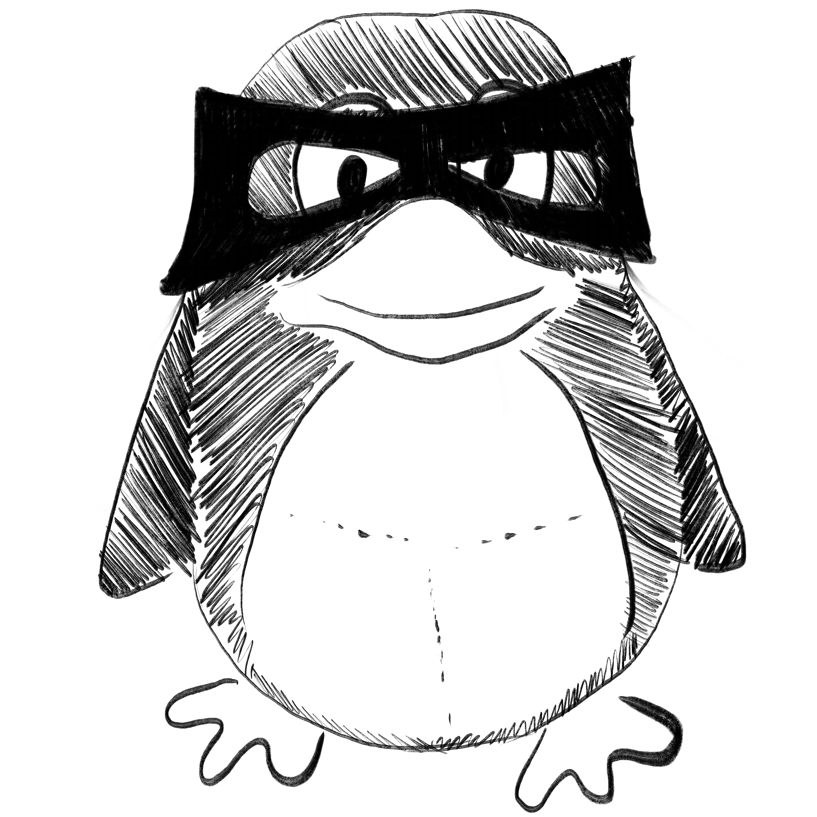
A high-performance deep-learning-based pipeline for whole-brain vasculature segmentation at the capillary resolution.
In Bioinformatics (Oxford, England)
MOTIVATION :
RESULTS :
AVAILABILITY :
SUPPLEMENTARY INFORMATION :
Li Yuxin, Liu Xuhua, Jia Xueyan, Jiang Tao, Wu Jianghao, Zhang Qianlong, Li Junhuai, Li Xiangning, Li Anan
2023-Mar-22
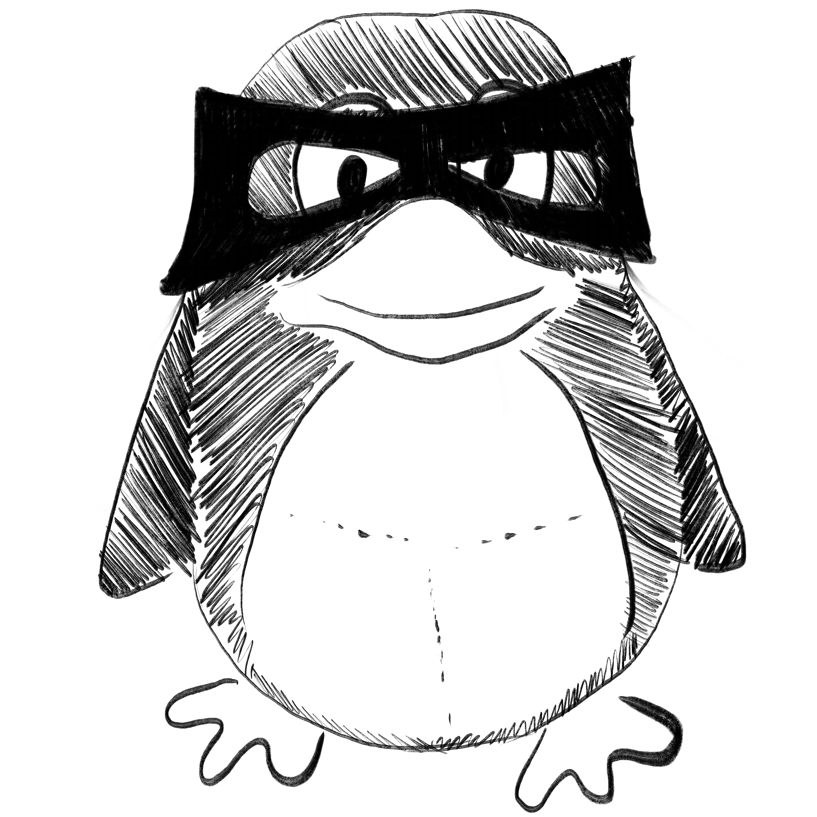
Profiling mechanisms that drive acute oral toxicity in mammals and its prediction via machine learning.
In Toxicological sciences : an official journal of the Society of Toxicology
Wijeyesakere Sanjeeva J, Auernhammer Tyler, Parks Amanda, Wilson Dan
2023-Mar-22
QSAR, acute toxicity, mechanistic toxicology
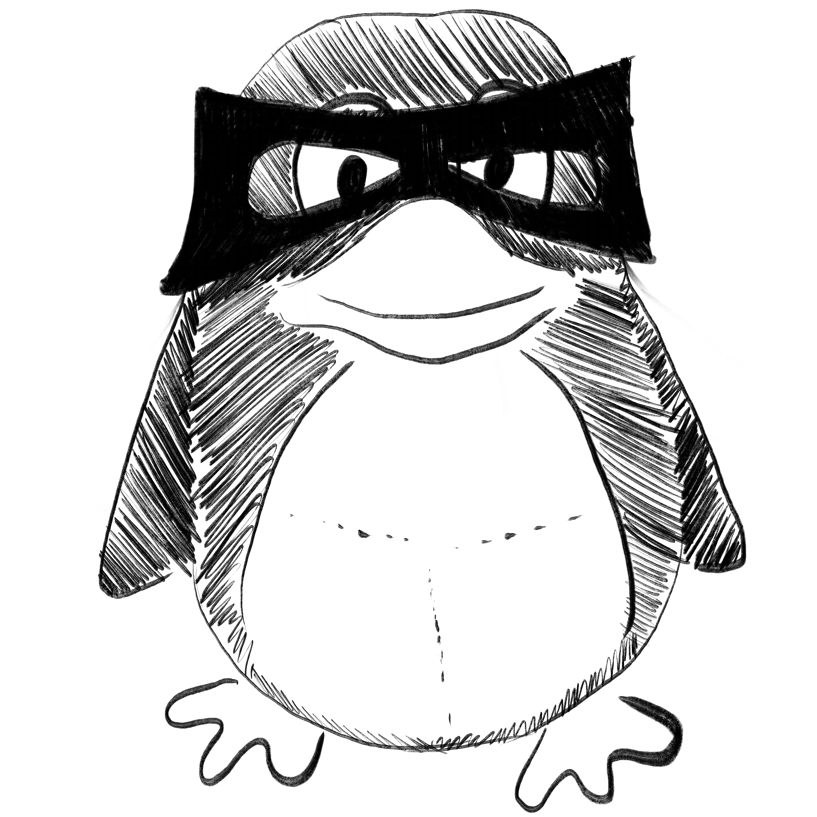
Blood quality evaluation via on-chip classification of cell morphology using a deep learning algorithm.
In Lab on a chip
Yang Yuping, He Hong, Wang Junju, Chen Li, Xu Yi, Ge Chuang, Li Shunbo
2023-Mar-22
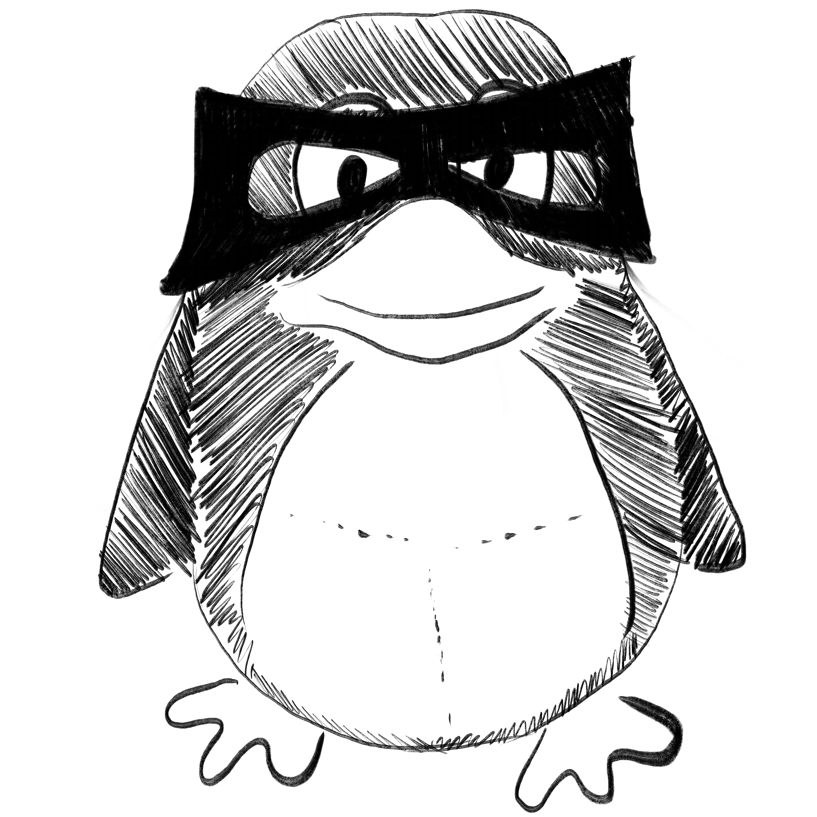
Artificial Intelligence Teaching as part of Medical Education: A Qualitative Analysis of Expert Interviews.
In JMIR medical education
BACKGROUND :
OBJECTIVE :
METHODS :
RESULTS :
CONCLUSIONS :
Weidener Lukas, Fischer Michael
2023-Mar-21
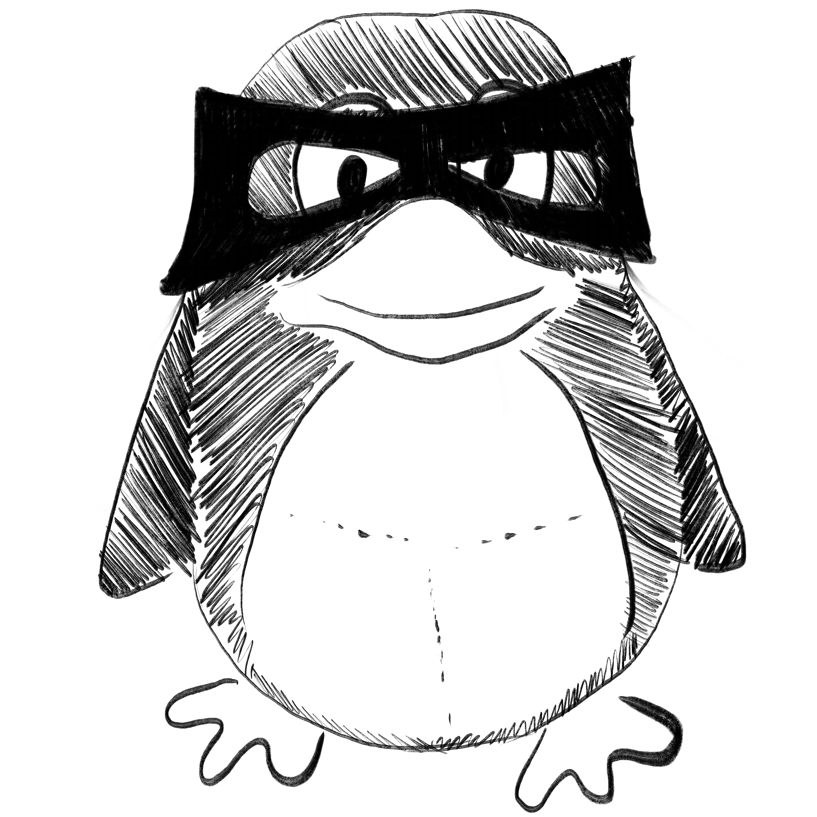
Memristor-based neural networks: a bridge from device to artificial intelligence.
In Nanoscale horizons
Cao Zelin, Sun Bai, Zhou Guangdong, Mao Shuangsuo, Zhu Shouhui, Zhang Jie, Ke Chuan, Zhao Yong, Shao Jinyou
2023-Mar-22
Weekly Summary
Receive a weekly summary and discussion of the top papers of the week by leading researchers in the field.