Receive a weekly summary and discussion of the top papers of the week by leading researchers in the field.
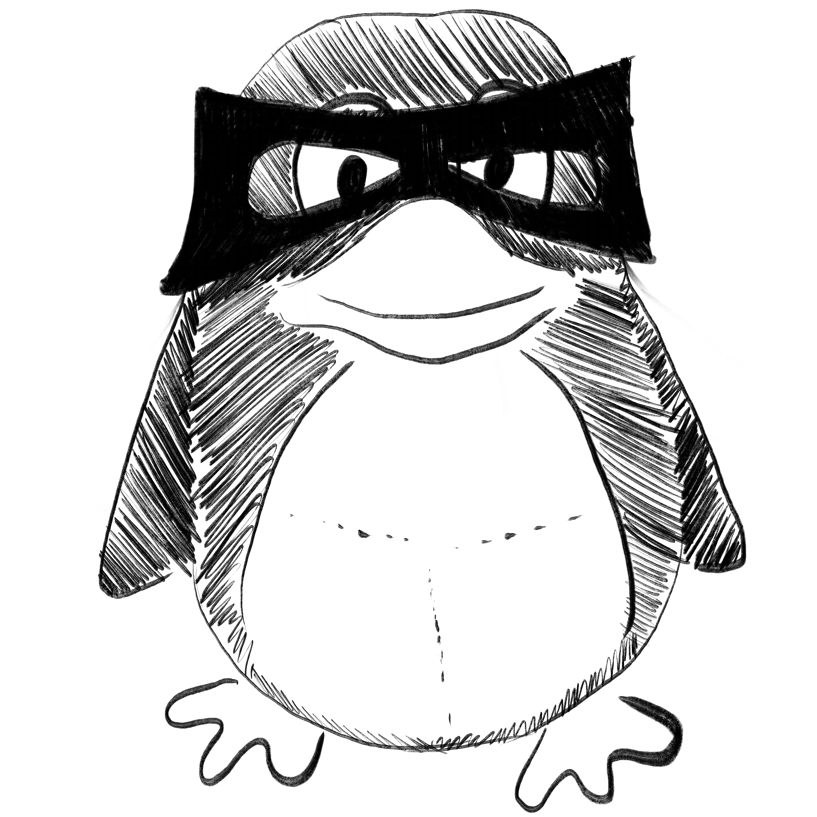
SARS-CoV-2 related adaptation mechanisms of rehabilitation clinics affecting patient-centred care: Qualitative study of online patient reports.
In JMIR rehabilitation and assistive technologies
BACKGROUND :
OBJECTIVE :
METHODS :
RESULTS :
CONCLUSIONS :
CLINICALTRIAL :
Kühn Lukas, Lindert Lara, Kuper Paulina, Choi Kyung-Eun Anna
2023-Mar-05
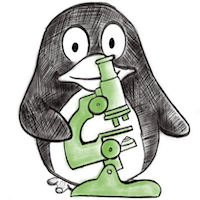
OCELOT: Overlapped Cell on Tissue Dataset for Histopathology
ArXiv Preprint
Jeongun Ryu, Aaron Valero Puche, JaeWoong Shin, Seonwook Park, Biagio Brattoli, Jinhee Lee, Wonkyung Jung, Soo Ick Cho, Kyunghyun Paeng, Chan-Young Ock, Donggeun Yoo, Sérgio Pereira
2023-03-23
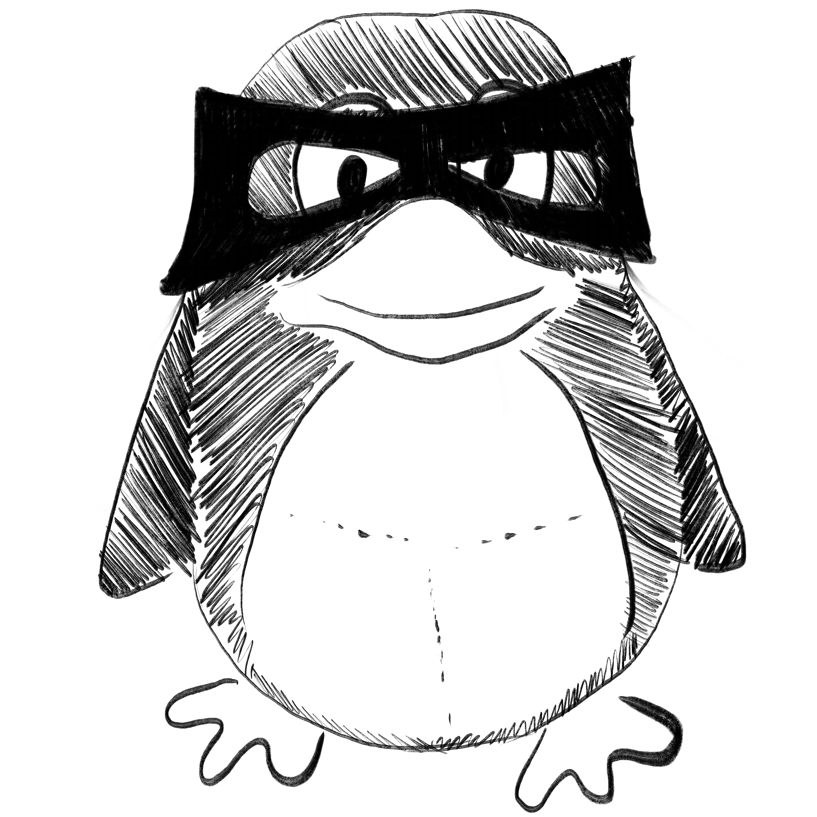
CT-free quantitative SPECT for automatic evaluation of %thyroid uptake based on deep-learning.
In EJNMMI physics
PURPOSE :
METHODS :
RESULTS :
CONCLUSION :
Kwon Kyounghyoun, Hwang Donghwi, Oh Dongkyu, Kim Ji Hye, Yoo Jihyung, Lee Jae Sung, Lee Won Woo
2023-Mar-22
Quantification; Single-photon emission computed tomography; Deep-learning; Attenuation correction; Segmentation
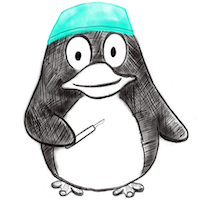
Deep learning-based recognition of key anatomical structures during robot-assisted minimally invasive esophagectomy.
In Surgical endoscopy ; h5-index 65.0
OBJECTIVE :
BACKGROUND :
METHODS :
RESULTS :
CONCLUSION :
den Boer R B, Jaspers T J M, de Jongh C, Pluim J P W, van der Sommen F, Boers T, van Hillegersberg R, Van Eijnatten M A J M, Ruurda J P
2023-Mar-22
Anatomy recognition, Computer vision, Deep learning, Robotics, Surgery
Transplant renal artery stenosis: utilization of machine learning to identify ancillary sonographic and doppler parameters to predict stenosis in patients with graft dysfunction.
In Abdominal radiology (New York)
PURPOSE :
MATERIALS AND METHODS :
RESULTS :
CONCLUSION :
Blain Yamile, Alessandrino Francesco, Scortegagna Eduardo, Balcacer Patricia
2023-Mar-22
Doppler ultrasound, Machine learning, Renal transplant, Transplant renal artery stenosis
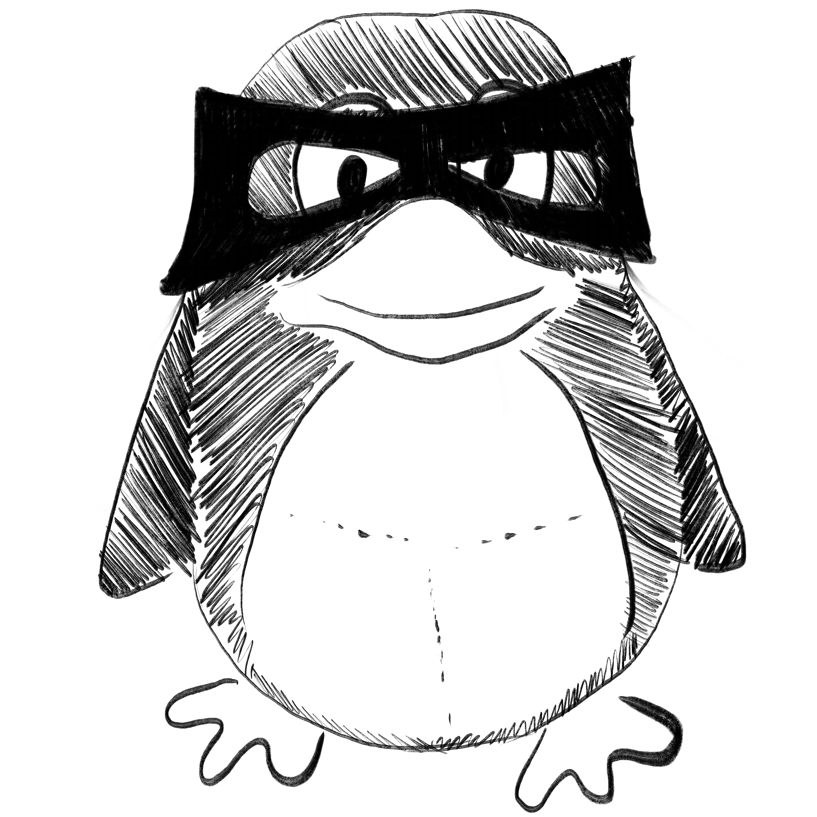
Localizing post-admixture adaptive variants with object detection on ancestry-painted chromosomes.
In Molecular biology and evolution
Hamid Iman, Korunes Katharine L, Schrider Daniel R, Goldberg Amy
2023-Mar-22
Weekly Summary
Receive a weekly summary and discussion of the top papers of the week by leading researchers in the field.