Receive a weekly summary and discussion of the top papers of the week by leading researchers in the field.
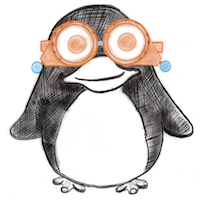
Differentiating malignant and benign eyelid lesions using deep learning.
In Scientific reports ; h5-index 158.0
Lee Min Joung, Yang Min Kyu, Khwarg Sang In, Oh Eun Kyu, Choi Youn Joo, Kim Namju, Choung Hokyung, Seo Chang Won, Ha Yun Jong, Cho Min Ho, Cho Bum-Joo
2023-Mar-13
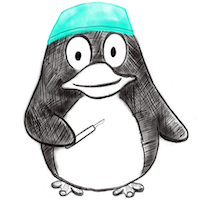
An artificial intelligence approach for predicting death or organ failure after hospitalization for COVID-19: development of a novel risk prediction tool and comparisons with ISARIC-4C, CURB-65, qSOFA, and MEWS scoring systems.
In Respiratory research ; h5-index 45.0
BACKGROUND :
METHODS :
RESULTS :
CONCLUSION :
Kwok Stephen Wai Hang, Wang Guanjin, Sohel Ferdous, Kashani Kianoush B, Zhu Ye, Wang Zhen, Antpack Eduardo, Khandelwal Kanika, Pagali Sandeep R, Nanda Sanjeev, Abdalrhim Ahmed D, Sharma Umesh M, Bhagra Sumit, Dugani Sagar, Takahashi Paul Y, Murad Mohammad H, Yousufuddin Mohammed
2023-Mar-13
COVID-19, Machine learning algorithms, Mortality, Organ failure, Prediction models
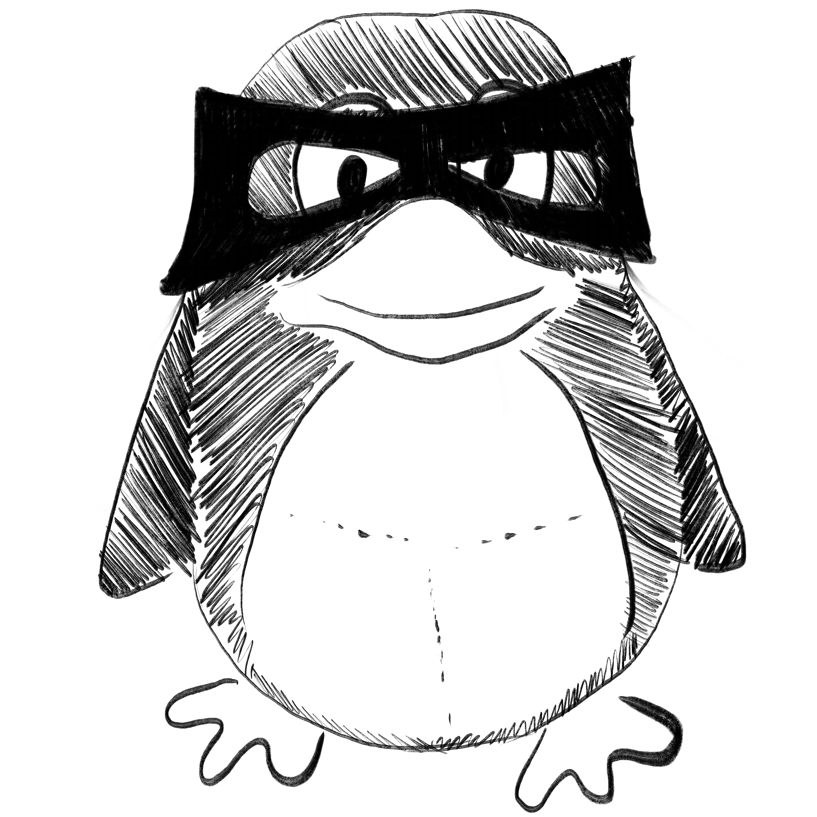
Multi-Modal Facial Expression Recognition with Transformer-Based Fusion Networks and Dynamic Sampling
ArXiv Preprint
Jun-Hwa Kim, Namho Kim, Chee Sun Won
2023-03-15
Development and validation of a prognostic model for HER2-low breast cancer to evaluate neoadjuvant therapy.
In Gland surgery
BACKGROUND :
METHODS :
RESULTS :
CONCLUSIONS :
Li Xiaoping, Lin Zhiquan, Yu Qihe, Qiu Chaoran, Lai Chan, Huang Hui, Zhang Yiwen, Zhang Weibin, Zhu Jintao, Huang Xin, Li Weiwen
2023-Feb-28
Breast cancer (BC), deep learning, human epidermal growth factor receptor 2-low (HER2-low), integral gradient, molecular subtype
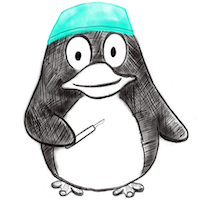
Marmoset monkeys use different avoidance strategies to cope with ambient noise during vocal behavior.
In iScience
Löschner Julia, Pomberger Thomas, Hage Steffen R
2023-Mar-17
Behavioral neuroscience, Biological sciences, Machine learning
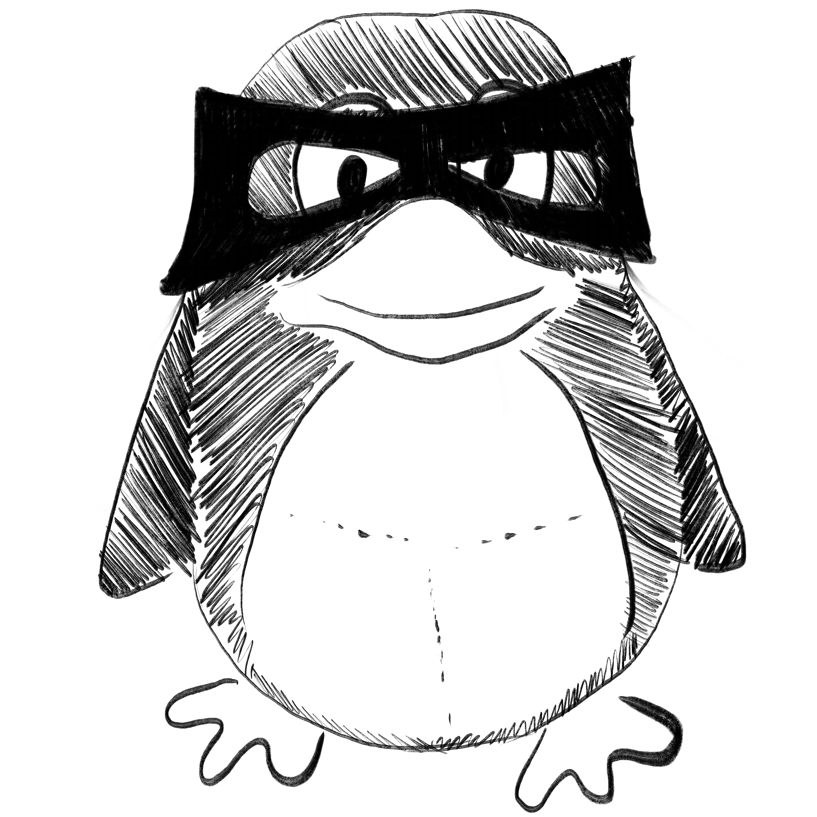
Predicting motor behavior: an efficient EEG signal processing pipeline to detect brain states with potential therapeutic relevance for VR-based neurorehabilitation.
In Virtual reality
McDermott Eric J, Metsomaa Johanna, Belardinelli Paolo, Grosse-Wentrup Moritz, Ziemann Ulf, Zrenner Christoph
2023
Brain-state decoding, Brain–computer interface (BCI), Classification, EEG, EEG-VR, Hand selection, Human-in-the-loop, Machine learning, Motor behavior, Motor intention, Neurorehabilitation, Open source pipeline, Pre-movement, Virtual reality
Weekly Summary
Receive a weekly summary and discussion of the top papers of the week by leading researchers in the field.