Receive a weekly summary and discussion of the top papers of the week by leading researchers in the field.
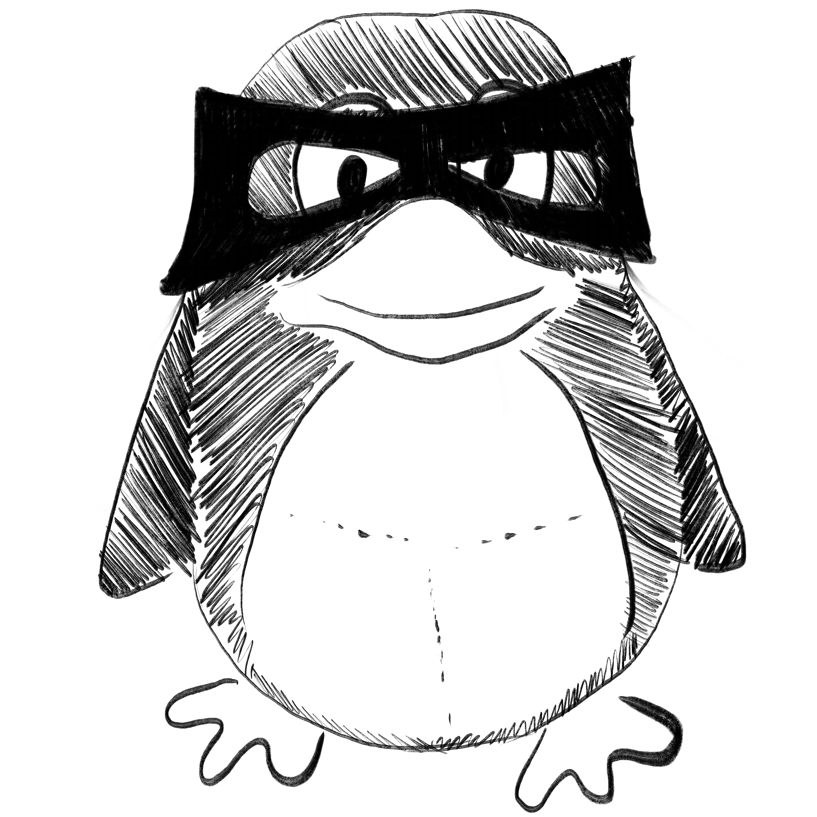
Health Monitoring of Movement Disorder Subject based on Diamond Stacked Sparse Autoencoder Ensemble Model
ArXiv Preprint
Likun Tang, Jie Ma, Yongming Li
2023-03-15
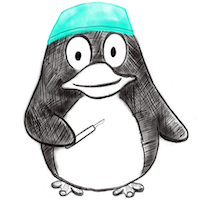
Development and Assessment of Assisted Diagnosis Models Using Machine Learning for Identifying Elderly Patients With Malnutrition: Cohort Study.
In Journal of medical Internet research ; h5-index 88.0
BACKGROUND :
OBJECTIVE :
METHODS :
RESULTS :
CONCLUSIONS :
TRIAL REGISTRATION :
Wang Xue, Yang Fengchun, Zhu Mingwei, Cui Hongyuan, Wei Junmin, Li Jiao, Chen Wei
2023-Mar-14
GLIM, SHAP, Shapley additive explanation, XGBoost, algorithm, diagnose, diagnosis, diagnostic, disease-related malnutrition, elder, global leadership initiative on malnutrition, machine learning, malnutrition, model, nutrition, older adult, older inpatients, risk, visualization
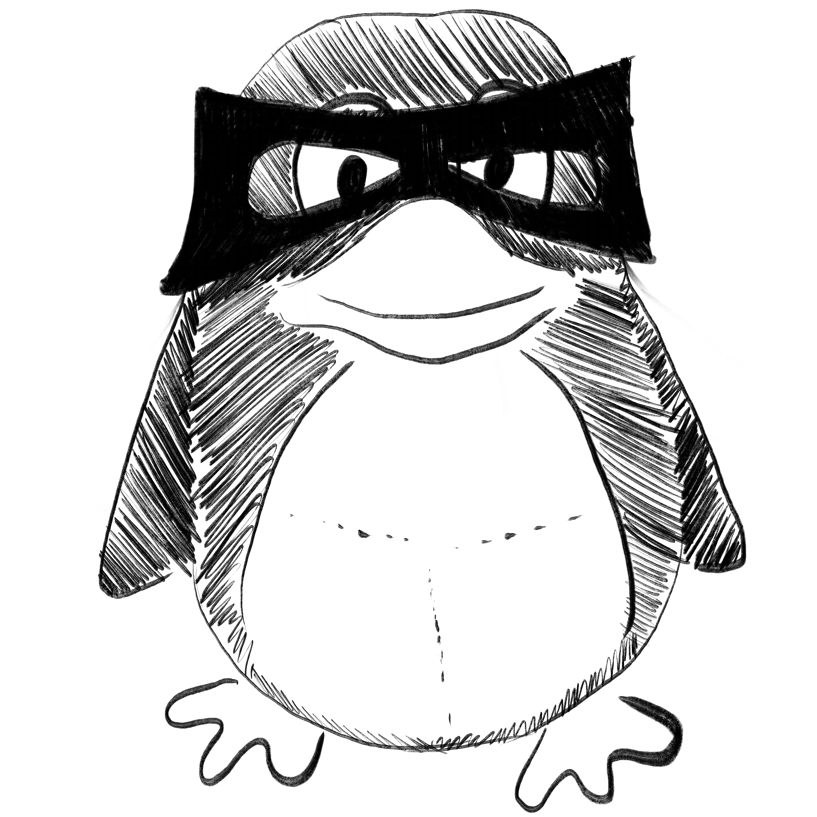
The Effectiveness of Wearable Devices Using Artificial Intelligence for Blood Glucose Level Forecasting or Prediction: Systematic Review.
In Journal of medical Internet research ; h5-index 88.0
BACKGROUND :
OBJECTIVE :
METHODS :
RESULTS :
CONCLUSIONS :
TRIAL REGISTRATION :
Ahmed Arfan, Aziz Sarah, Abd-Alrazaq Alaa, Farooq Faisal, Househ Mowafa, Sheikh Javaid
2023-Mar-14
artificial intelligence, blood glucose, diabetes, forecasting, machine learning, prediction, wearable devices
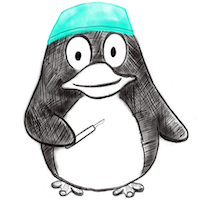
Effectiveness of artificial intelligence-assisted colonoscopy in early diagnosis of colorectal cancer: a systematic review.
In International journal of surgery (London, England)
INTRODUCTION :
METHODS :
RESULTS :
CONCLUSION :
Mehta Aashna, Kumar Harendra, Yazji Katia, Wireko Andrew Awuah, Sivanandan Nagarajan Jai, Ghosh Bikona, Nahas Ahmad, Morales Ojeda Luis, Chandra Ayush, Sharath Medha, Huang Helen, Garg Tulika, Isik Arda
2023-Mar-15
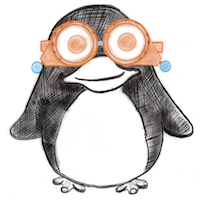
Morphometric Analysis of Retinal Ganglion Cell Axons in Normal and Glaucomatous Brown Norway Rats Optic Nerves.
In Translational vision science & technology
PURPOSE :
METHODS :
RESULTS :
CONCLUSIONS :
TRANSLATIONAL RELEVANCE :
Goyal Vidisha, Read A Thomas, Brown Dillon M, Brawer Luke, Bateh Kaitlyn, Hannon Bailey G, Feola Andrew J, Ethier C Ross
2023-Mar-01
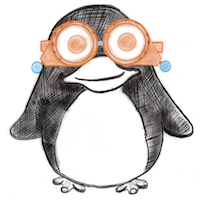
AxoNet 2.0: A Deep Learning-Based Tool for Morphometric Analysis of Retinal Ganglion Cell Axons.
In Translational vision science & technology
PURPOSE :
METHODS :
RESULTS :
CONCLUSIONS :
TRANSLATIONAL RELEVANCE :
Goyal Vidisha, Read A Thomas, Ritch Matthew D, Hannon Bailey G, Rodriguez Gabriela Sanchez, Brown Dillon M, Feola Andrew J, Hedberg-Buenz Adam, Cull Grant A, Reynaud Juan, Garvin Mona K, Anderson Michael G, Burgoyne Claude F, Ethier C Ross
2023-Mar-01
Weekly Summary
Receive a weekly summary and discussion of the top papers of the week by leading researchers in the field.