Receive a weekly summary and discussion of the top papers of the week by leading researchers in the field.
Blood Inflammatory Biomarkers Differentiate Inpatient and Outpatient Coronavirus Disease 2019 From Influenza.
In Open forum infectious diseases
BACKGROUND :
METHODS :
RESULTS :
CONCLUSIONS :
Luciani Lauren L, Miller Leigh M, Zhai Bo, Clarke Karen, Hughes Kramer Kailey, Schratz Lucas J, Balasubramani G K, Dauer Klancie, Nowalk M Patricia, Zimmerman Richard K, Shoemaker Jason E, Alcorn John F
2023-Mar
SARS-CoV-2, cytokine, human, machine learning, pneumonia
Predictive factors for degenerative lumbar spinal stenosis: a model obtained from a machine learning algorithm technique.
In BMC musculoskeletal disorders ; h5-index 46.0
BACKGROUND :
METHODS :
RESULTS :
CONCLUSIONS :
Abbas Janan, Yousef Malik, Peled Natan, Hershkovitz Israel, Hamoud Kamal
2023-Mar-23
Computer Tomography, Degenerative lumbar spinal stenosis, Machine learning, Spine dimensions
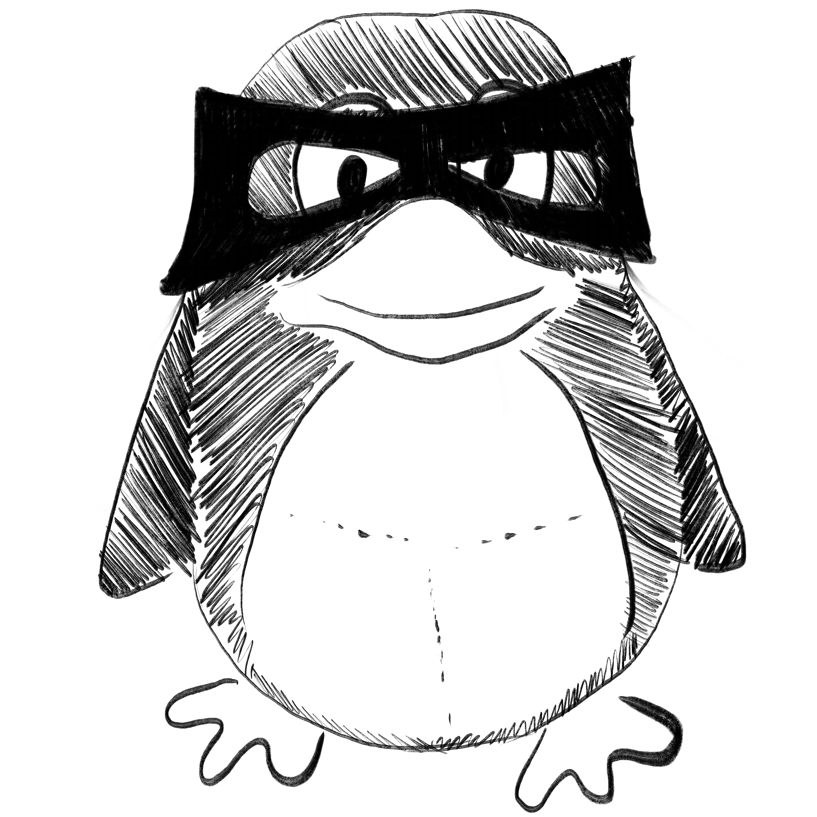
A study on predicting the length of hospital stay for Chinese patients with ischemic stroke based on the XGBoost algorithm.
In BMC medical informatics and decision making ; h5-index 38.0
BACKGROUND :
METHODS :
RESULTS :
CONCLUSIONS :
Chen Rui, Zhang Shengfa, Li Jie, Guo Dongwei, Zhang Weijun, Wang Xiaoying, Tian Donghua, Qu Zhiyong, Wang Xiaohua
2023-Mar-22
Ischemic stroke, Length of hospital stay (LOS), Machine learning (ML) model, XGBoost algorithm
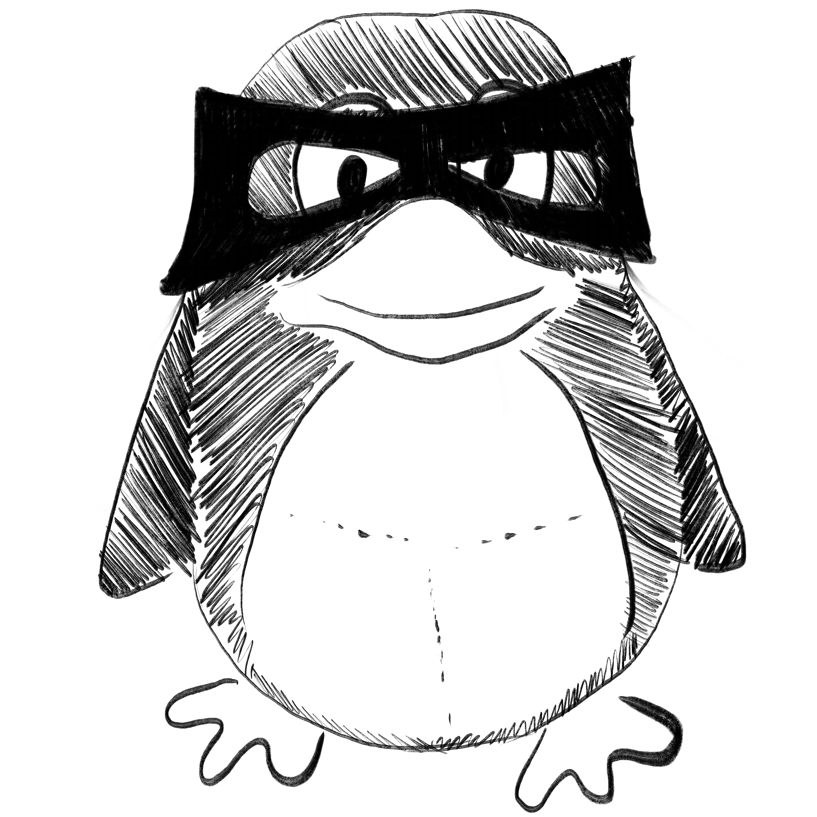
Automated Adolescence Scoliosis Detection Using Augmented U-Net With Non-square Kernels.
In Canadian Association of Radiologists journal = Journal l'Association canadienne des radiologistes
Wu Yujie, Namdar Khashayar, Chen Chaojun, Hosseinpour Shahob, Shroff Manohar, Doria Andrea S, Khalvati Farzad
2023-Mar-22
Cobb angle, convolutional neural network, scoliosis, vertebra landmark
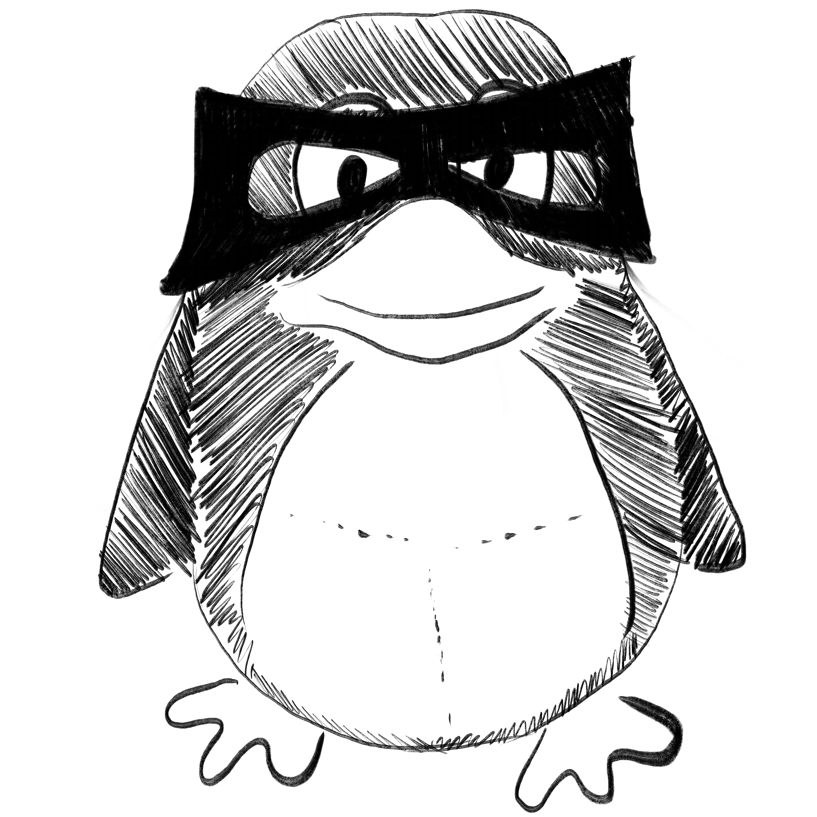
MSLP: mRNA subcellular localization predictor based on machine learning techniques.
In BMC bioinformatics
BACKGROUND :
METHODS :
RESULTS :
AVAILABILITY :
Musleh Saleh, Islam Mohammad Tariqul, Qureshi Rizwan, Alajez Nihad, Alam Tanvir
2023-Mar-22
Localization prediction, Machine learning, RNA, Sequence analysis, Subcellular localization, mRNA
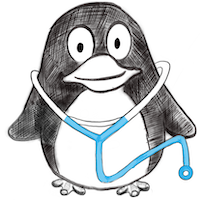
Anaemia in the first week may be associated with long-term mortality among critically ill patients: propensity score-based analyses.
In BMC emergency medicine
BACKGROUND :
METHODS :
RESULTS :
CONCLUSIONS :
Lin I-Hung, Liao Pei-Ya, Wong Li-Ting, Chan Ming-Cheng, Wu Chieh-Liang, Chao Wen-Cheng
2023-Mar-22
Anaemia, Critical illness, Long-term outcome, Propensity score
Weekly Summary
Receive a weekly summary and discussion of the top papers of the week by leading researchers in the field.