Receive a weekly summary and discussion of the top papers of the week by leading researchers in the field.
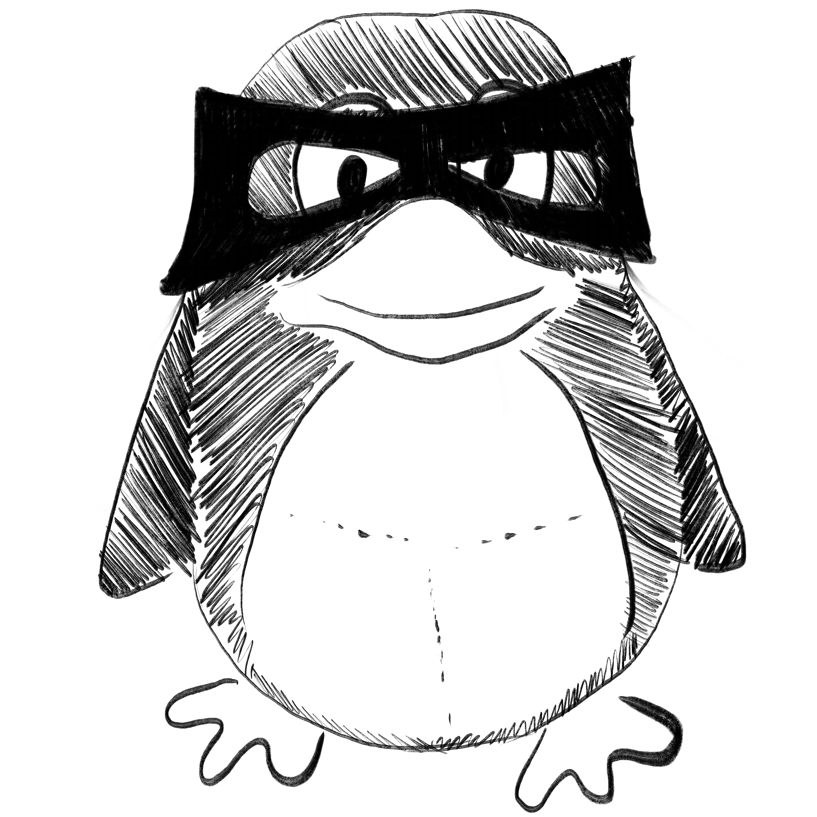
Artificial intelligence for drug discovery: Resources, methods, and applications.
In Molecular therapy. Nucleic acids
Chen Wei, Liu Xuesong, Zhang Sanyin, Chen Shilin
2023-Mar-14
MT: Bioinformatics, artificial intelligence, bioinformatics, data resources, drug discovery and development, molecular descriptors
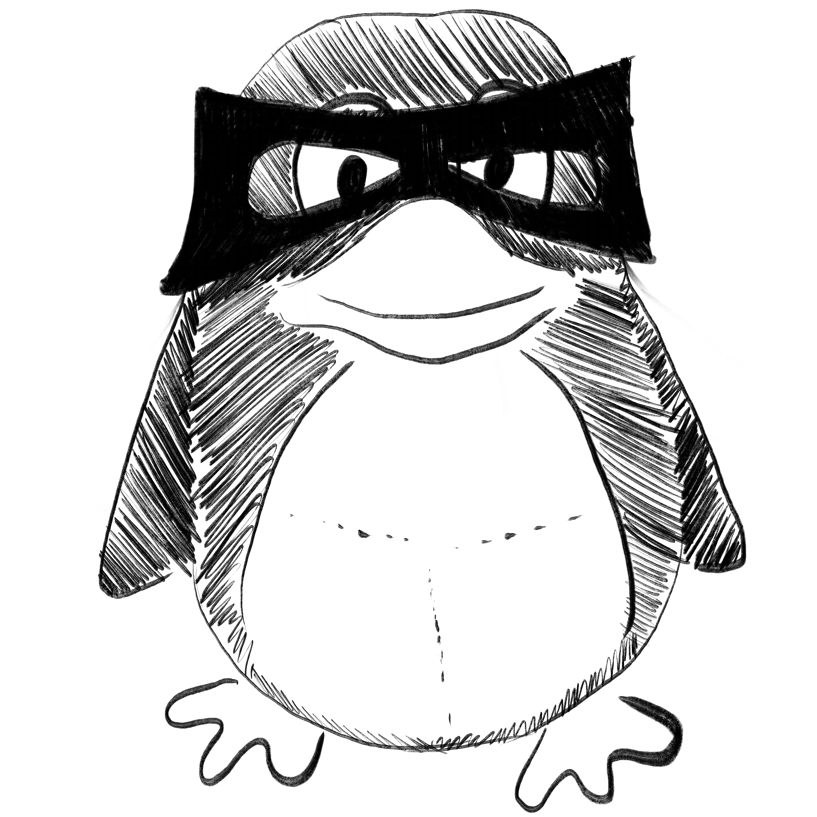
Small target detection with remote sensing images based on an improved YOLOv5 algorithm.
In Frontiers in neurorobotics
INTRODUCTION :
METHODS :
RESULTS AND DISCUSSION :
Pei Wenjing, Shi Zhanhao, Gong Kai
2022
EIoU loss, YOLOv5s, deep learning, remote sensing images, small target detection
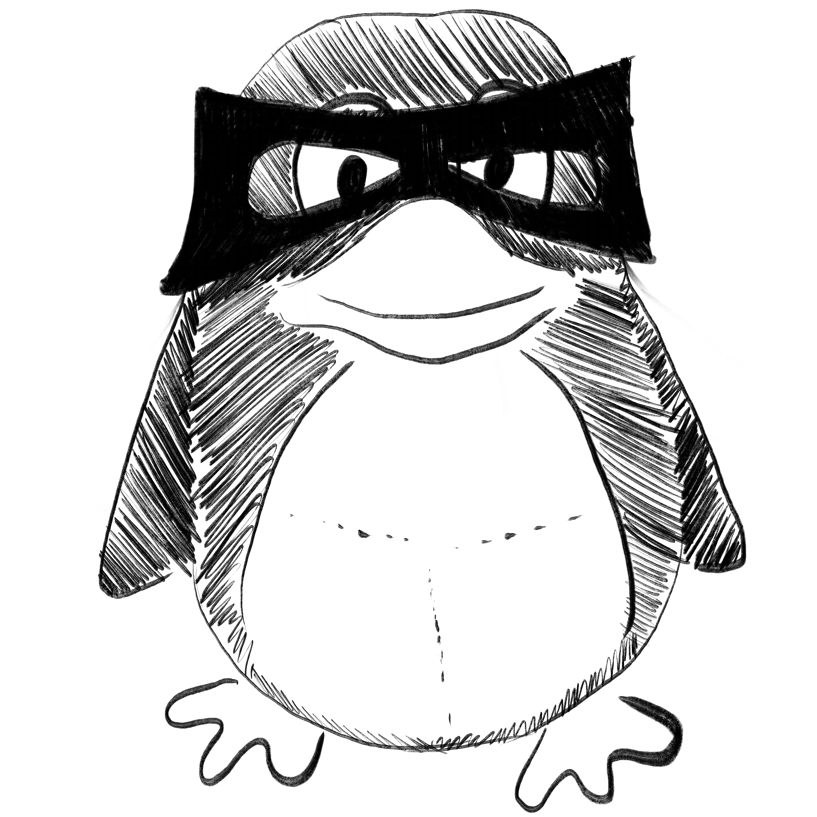
Accelerated Discovery of Macrocyclic CDK2 Inhibitor QR-6401 by Generative Models and Structure-Based Drug Design.
In ACS medicinal chemistry letters ; h5-index 37.0
Yu Yang, Huang Junhong, He Hu, Han Jing, Ye Geyan, Xu Tingyang, Sun Xianqiang, Chen Xiumei, Ren Xiaoming, Li Chunlai, Li Huijuan, Huang Wei, Liu Yangyang, Wang Xinjuan, Gao Yongzhi, Cheng Nianhe, Guo Na, Chen Xibo, Feng Jianxia, Hua Yuxia, Liu Chong, Zhu Guoyun, Xie Zhi, Yao Lili, Zhong Wenge, Chen Xinde, Liu Wei, Li Hailong
2023-Mar-09
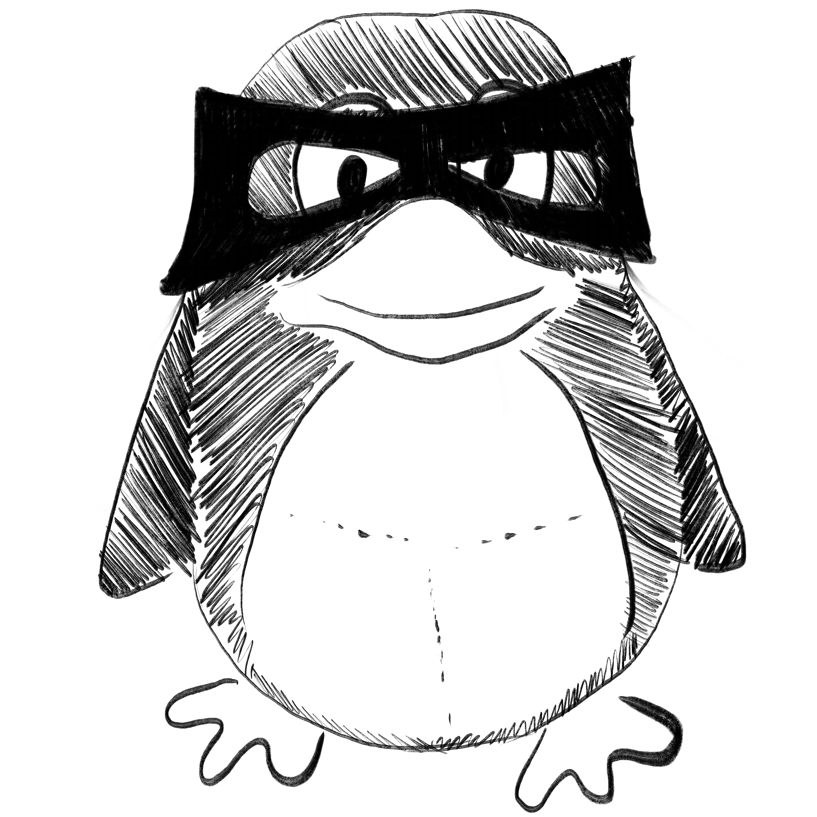
Research on Supply Chain Financial Risk Prevention Based on Machine Learning.
In Computational intelligence and neuroscience
Lei Yang, Qiaoming Hou, Tong Zhao
2023
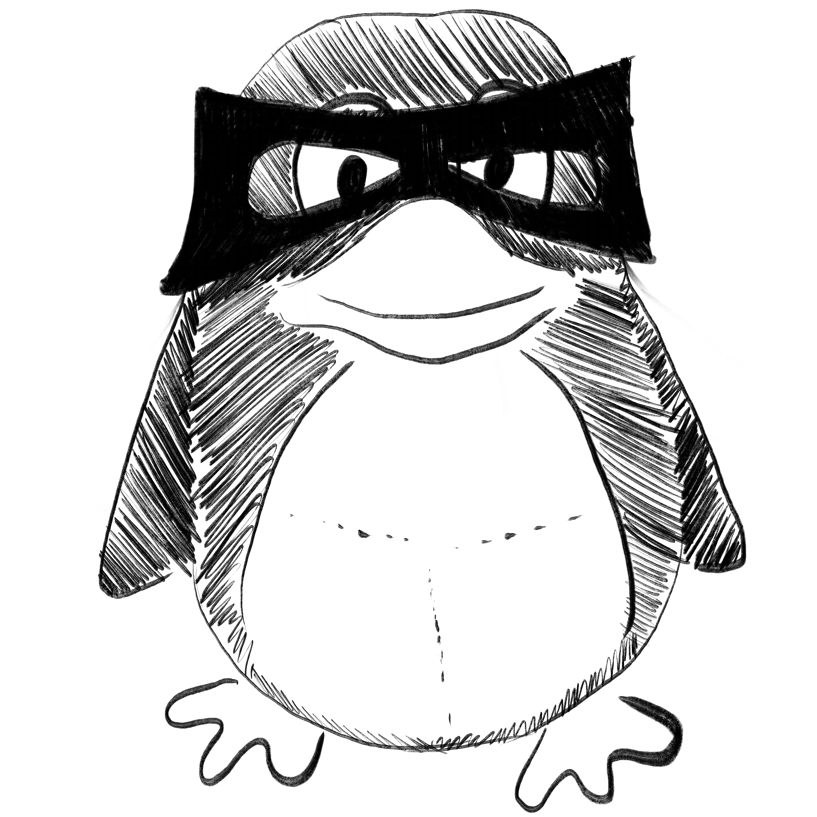
Weibo users and Academia's foci on tourism safety: Implications from institutional differences and digital divide.
In Heliyon
Zeng Liyun, Li Rita Yi Man, Zeng Huiling
2023-Mar
Artificial intelligence, Bibliometrics, Comparative analysis, Digital divide, Information asymmetry, Tourism safety, Web of science, Weibo
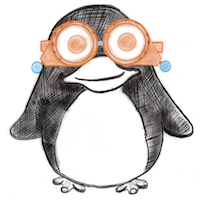
Machine learning radiomics to predict the early recurrence of intrahepatic cholangiocarcinoma after curative resection: A multicentre cohort study.
In European journal of nuclear medicine and molecular imaging ; h5-index 66.0
PURPOSE :
METHODS :
RESULTS :
CONCLUSION :
Bo Zhiyuan, Chen Bo, Yang Yi, Yao Fei, Mao Yicheng, Yao Jiangqiao, Yang Jinhuan, He Qikuan, Zhao Zhengxiao, Shi Xintong, Chen Jicai, Yu Zhengping, Yang Yunjun, Wang Yi, Chen Gang
2023-Mar-16
Contrast-enhanced computed tomography, Early recurrence, Intrahepatic cholangiocarcinoma, Machine learning, Radiomics
Weekly Summary
Receive a weekly summary and discussion of the top papers of the week by leading researchers in the field.