Receive a weekly summary and discussion of the top papers of the week by leading researchers in the field.
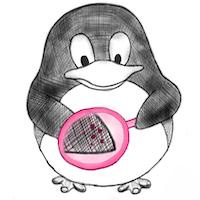
Exploring the potential of artificial intelligence in improving skin lesion diagnosis in primary care.
In Scientific reports ; h5-index 158.0
Escalé-Besa Anna, Yélamos Oriol, Vidal-Alaball Josep, Fuster-Casanovas Aïna, Miró Catalina Queralt, Börve Alexander, Ander-Egg Aguilar Ricardo, Fustà-Novell Xavier, Cubiró Xavier, Rafat Mireia Esquius, López-Sanchez Cristina, Marin-Gomez Francesc X
2023-Mar-15
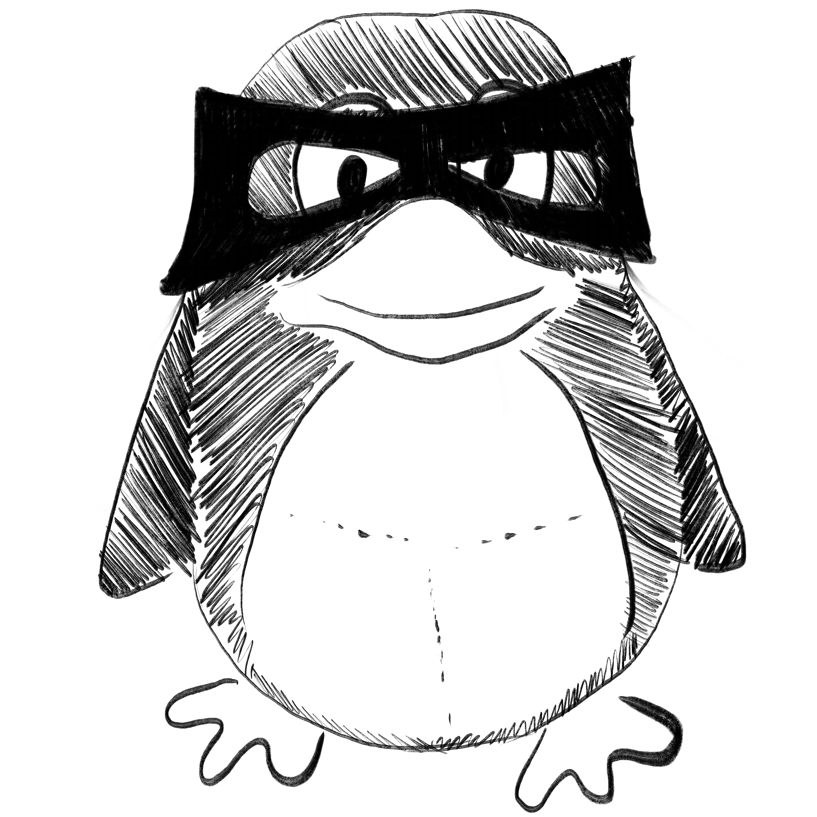
Hybrid Classic-Quantum Computing for Staging of Invasive Ductal Carcinoma of Breast
ArXiv Preprint
Vicente Moret-Bonillo, Eduardo Mosqueira-Rey, Samuel Magaz-Romero, Diego Alvarez-Estevez
2023-03-17
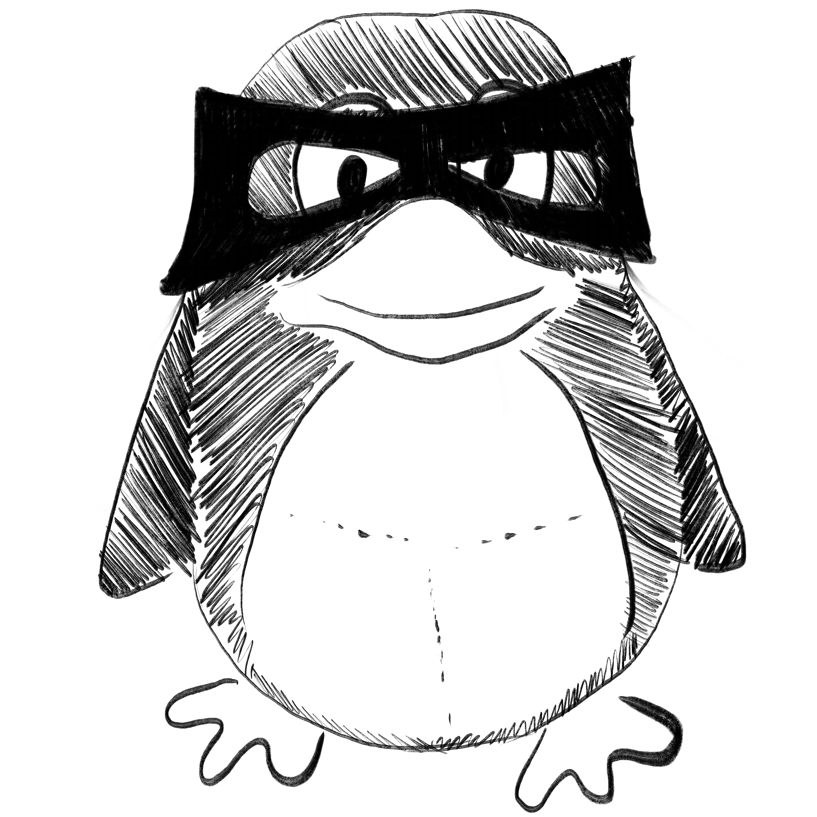
IntelliSleepScorer, a software package with a graphic user interface for automated sleep stage scoring in mice based on a light gradient boosting machine algorithm.
In Scientific reports ; h5-index 158.0
Wang Lei A, Kern Ryan, Yu Eunah, Choi Soonwook, Pan Jen Q
2023-Mar-15
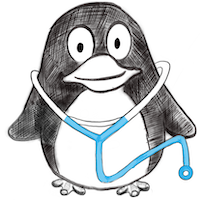
The use of Smart Environments and Robots for Infection Prevention Control: a systematic literature review.
In American journal of infection control ; h5-index 43.0
Piaggio Davide, Zarro Marianna, Pagliara Silvio, Andellini Martina, Almuhini Abdulaziz, Maccaro Alessia, Pecchia Leandro
2023-Mar-14
Infection prevention and control, artificial intelligence, hand hygiene, health 4.0, internet of things, robot
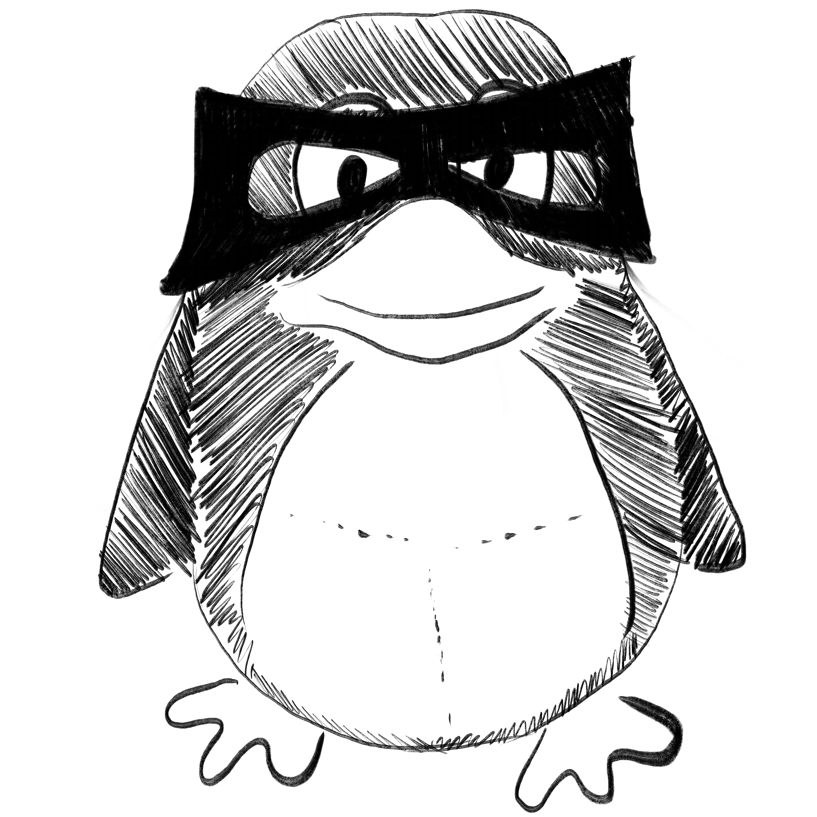
Causal Discovery from Temporal Data: An Overview and New Perspectives
ArXiv Preprint
Chang Gong, Di Yao, Chuzhe Zhang, Wenbin Li, Jingping Bi
2023-03-17
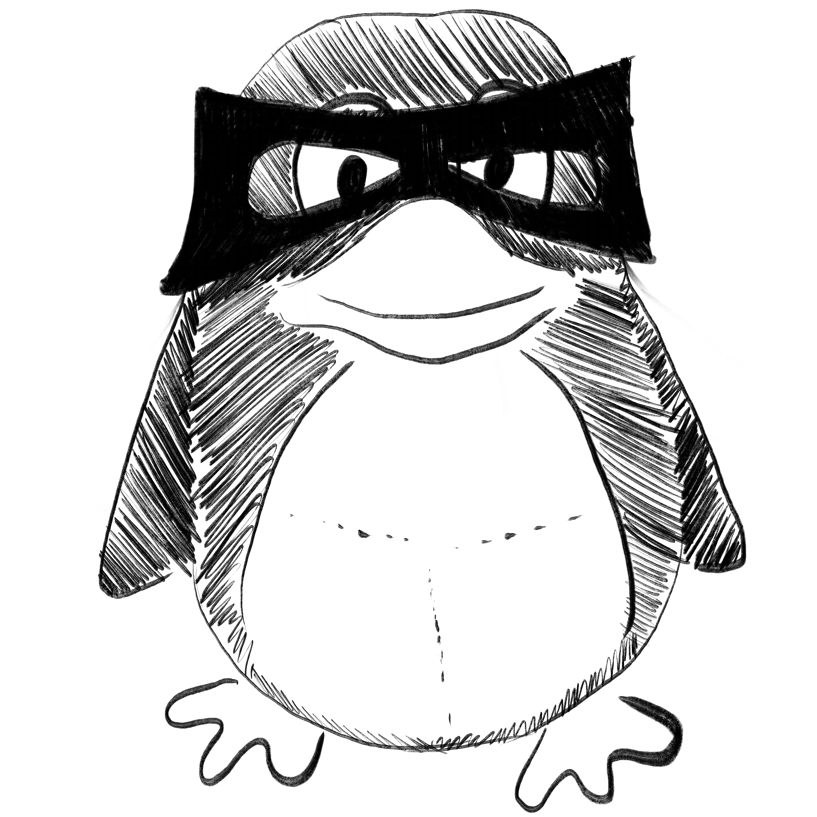
Distributed output formation tracking control of heterogeneous multi-agent systems using reinforcement learning.
In ISA transactions
Shi Yu, Dong Xiwang, Hua Yongzhao, Yu Jianglong, Ren Zhang
2023-Mar-08
Distributed trajectory generator, Heterogeneous system, Output formation tracking, Reinforcement learning
Weekly Summary
Receive a weekly summary and discussion of the top papers of the week by leading researchers in the field.