Receive a weekly summary and discussion of the top papers of the week by leading researchers in the field.
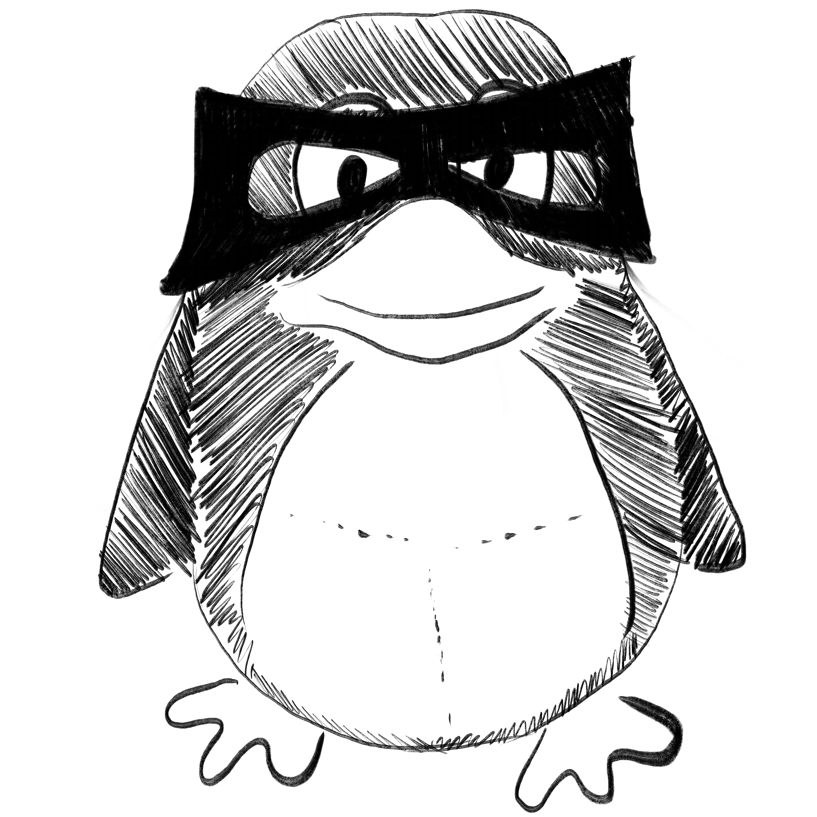
Machine learning models trained on synthetic datasets of multiple sample sizes for the use of predicting blood pressure from clinical data in a national dataset.
In PloS one ; h5-index 176.0
INTRODUCTION :
METHODS :
RESULTS :
DISCUSSION :
Arora Anmol, Arora Ananya
2023
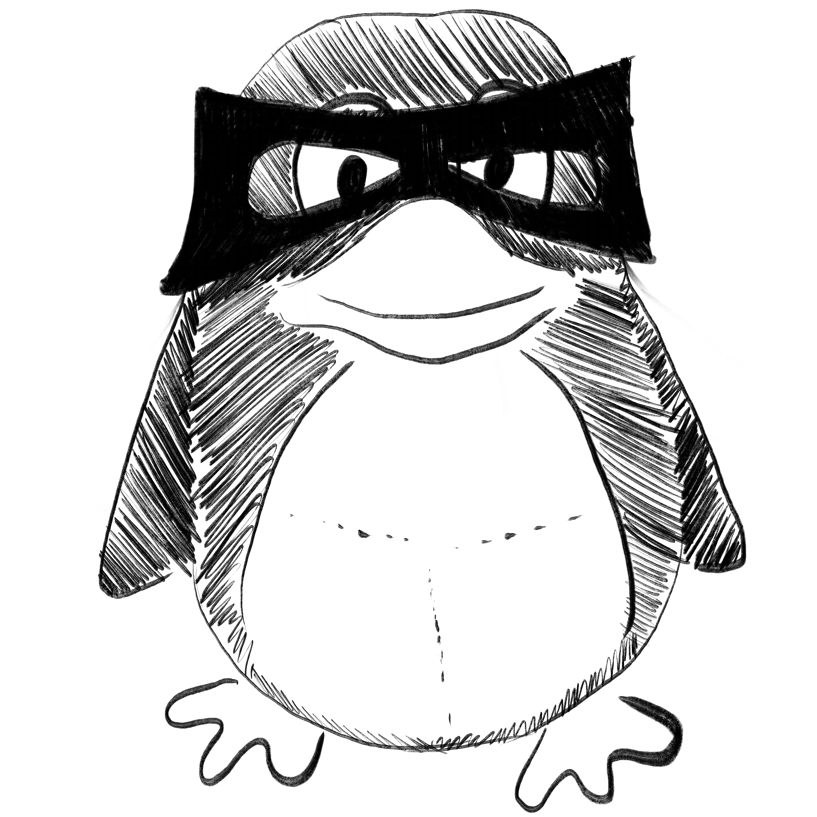
AUNet: a deep learning method for spectral information classification to identify inks.
In Analytical methods : advancing methods and applications
Shi Yan, He Xinyu, Zhang Qinglun, Yin Chongbo, Feng Ninghui, Chen Haoming, Lin Hualing
2023-Mar-17
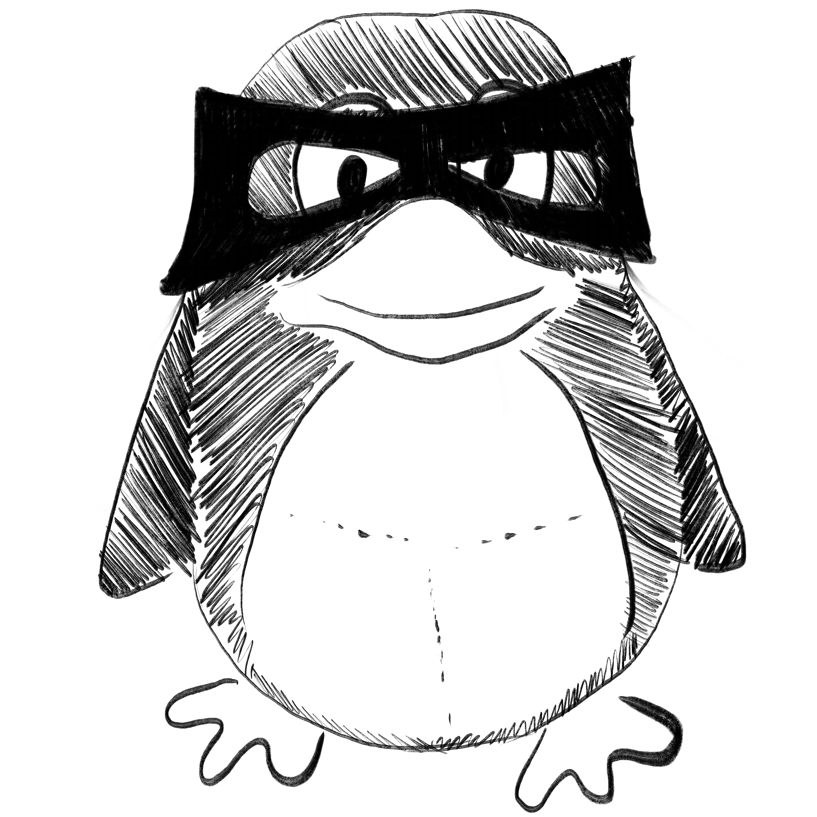
Corrigendum: Small target detection with remote sensing images based on an improved YOLOv5 algorithm.
In Frontiers in neurorobotics
Pei Wenjing, Shi Zhanhao, Gong Kai
2023
EIoU loss, YOLOv5s, deep learning, remote sensing images, small target detection
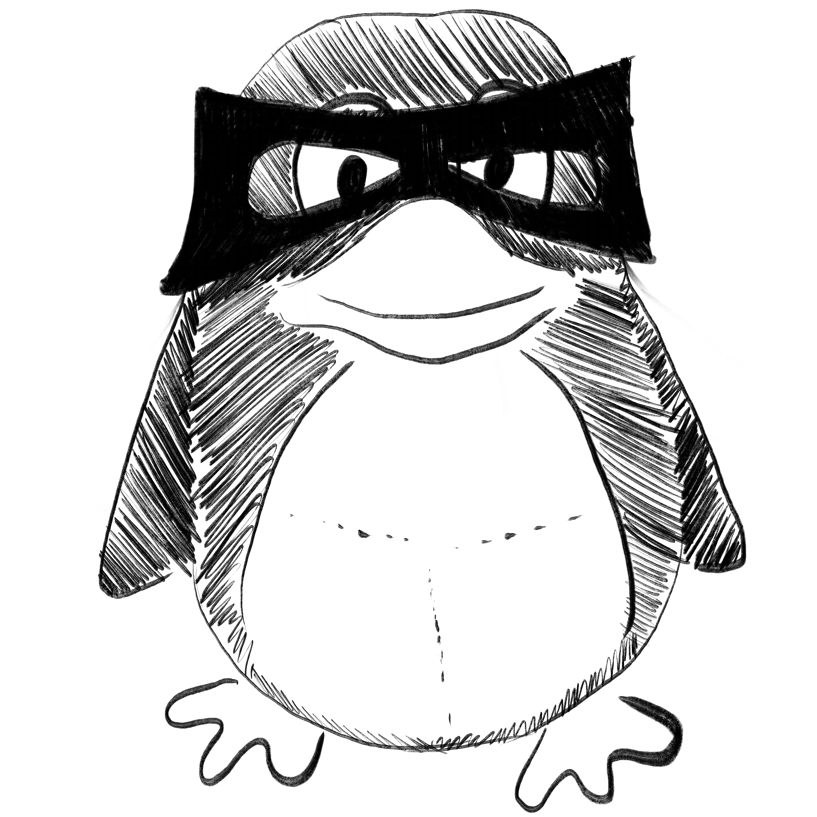
Longitudinal proteomic investigation of COVID-19 vaccination.
In Protein & cell
Wang Yingrui, Zhu Qianru, Sun Rui, Yi Xiao, Huang Lingling, Hu Yifan, Ge Weigang, Gao Huanhuan, Ye Xinfu, Song Yu, Shao Li, Li Yantao, Li Jie, Guo Tiannan, Shi Junping
2023-Feb-06
COVID-19, machine learning, neutralizing antibodies (NAbs), proteomics, vaccination
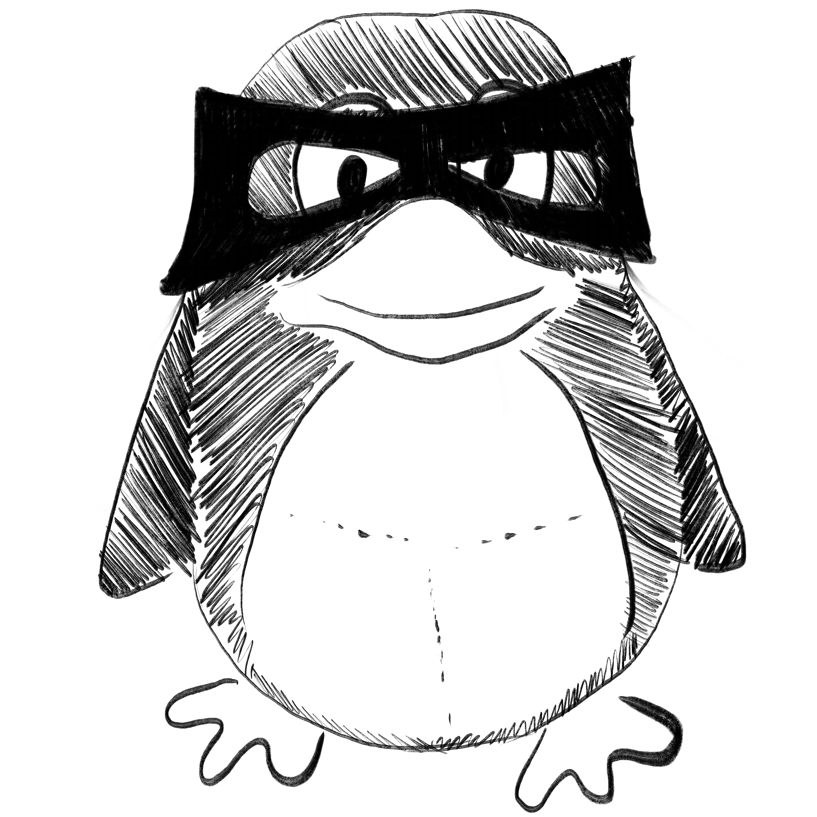
A deep reinforcement learning algorithm for the rectangular strip packing problem.
In PloS one ; h5-index 176.0
Fang Jie, Rao Yunqing, Shi Mingliang
2023
Quantitative bone marrow lesion, meniscus, and synovitis measurement: current status.
In Skeletal radiology
Smith Stacy E, Bahouth Sara M, Duryea Jeffrey
2023-Mar-16
Bone marrow lesions, Effusion synovitis, Hoffa’s, MRI; Quantitative software, Meniscus
Weekly Summary
Receive a weekly summary and discussion of the top papers of the week by leading researchers in the field.