Receive a weekly summary and discussion of the top papers of the week by leading researchers in the field.
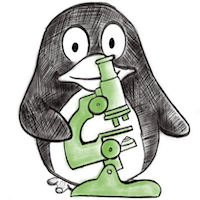
A systematic evaluation of deep learning methods for the prediction of drug synergy in cancer.
In PLoS computational biology
Baptista Delora, Ferreira Pedro G, Rocha Miguel
2023-Mar-23
Interoperable slide microscopy viewer and annotation tool for imaging data science and computational pathology.
In Nature communications ; h5-index 260.0
Gorman Chris, Punzo Davide, Octaviano Igor, Pieper Steven, Longabaugh William J R, Clunie David A, Kikinis Ron, Fedorov Andrey Y, Herrmann Markus D
2023-Mar-22
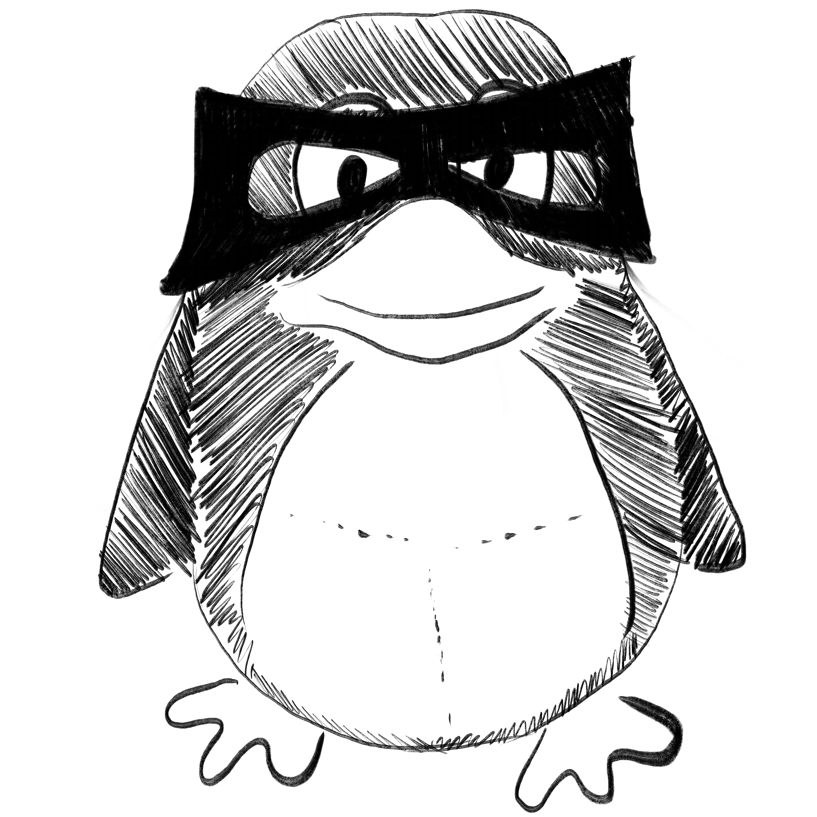
Dense reinforcement learning for safety validation of autonomous vehicles.
In Nature ; h5-index 368.0
Feng Shuo, Sun Haowei, Yan Xintao, Zhu Haojie, Zou Zhengxia, Shen Shengyin, Liu Henry X
2023-Mar
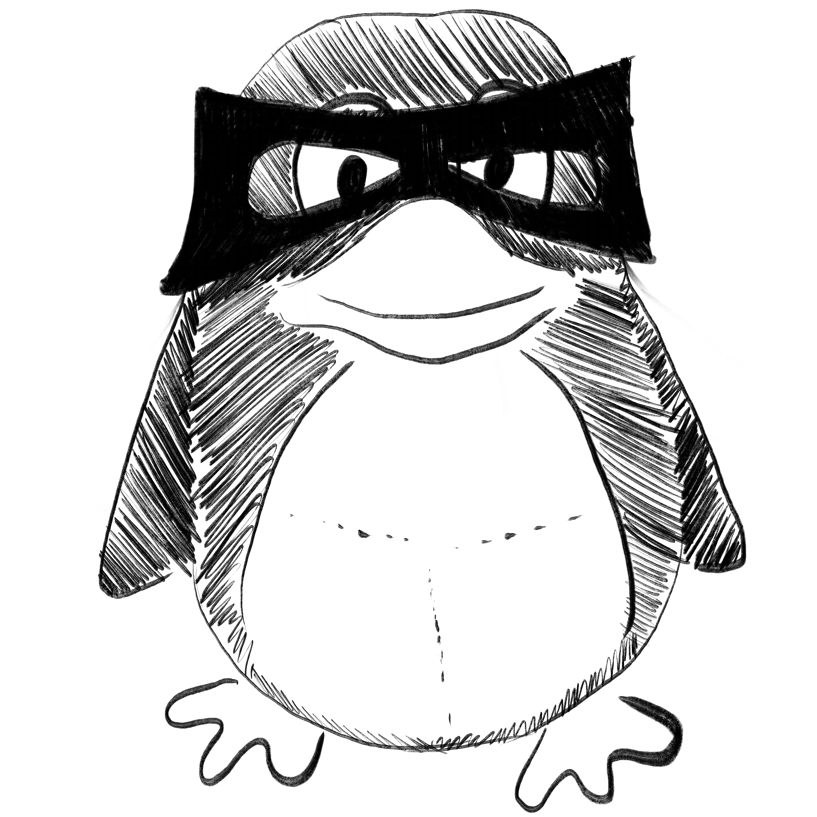
Characterization of RNA polymerase II trigger loop mutations using molecular dynamics simulations and machine learning.
In PLoS computational biology
Dutagaci Bercem, Duan Bingbing, Qiu Chenxi, Kaplan Craig D, Feig Michael
2023-Mar-22
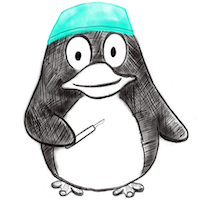
Comparison of Online-Onboard Adaptive Intensity-Modulated Radiation Therapy or Volumetric-Modulated Arc Radiotherapy With Image-Guided Radiotherapy for Patients With Gynecologic Tumors in Dependence on Fractionation and the Planning Target Volume Margin.
In JAMA network open
IMPORTANCE :
OBJECTIVE :
DESIGN, SETTING, AND PARTICIPANTS :
EXPOSURE :
MAIN OUTCOMES AND MEASURES :
RESULTS :
CONCLUSIONS AND RELEVANCE :
Guberina Maja, Santiago Garcia Alina, Khouya Aymane, Pöttgen Christoph, Holubyev Kostyantyn, Ringbaek Toke Printz, Lachmuth Manfred, Alberti Yasemin, Hoffmann Christian, Hlouschek Julian, Gauler Thomas, Lübcke Wolfgang, Indenkämpen Frank, Stuschke Martin, Guberina Nika
2023-Mar-01
An Unsupervised Machine Learning Approach to Evaluating the Association of Symptom Clusters With Adverse Outcomes Among Older Adults With Advanced Cancer: A Secondary Analysis of a Randomized Clinical Trial.
In JAMA network open
IMPORTANCE :
OBJECTIVE :
DESIGN, SETTING, AND PARTICIPANTS :
EXPOSURES :
MAIN OUTCOMES AND MEASURES :
RESULTS :
CONCLUSIONS AND RELEVANCE :
TRIAL REGISTRATION :
Xu Huiwen, Mohamed Mostafa, Flannery Marie, Peppone Luke, Ramsdale Erika, Loh Kah Poh, Wells Megan, Jamieson Leah, Vogel Victor G, Hall Bianca Alexandra, Mustian Karen, Mohile Supriya, Culakova Eva
2023-Mar-01
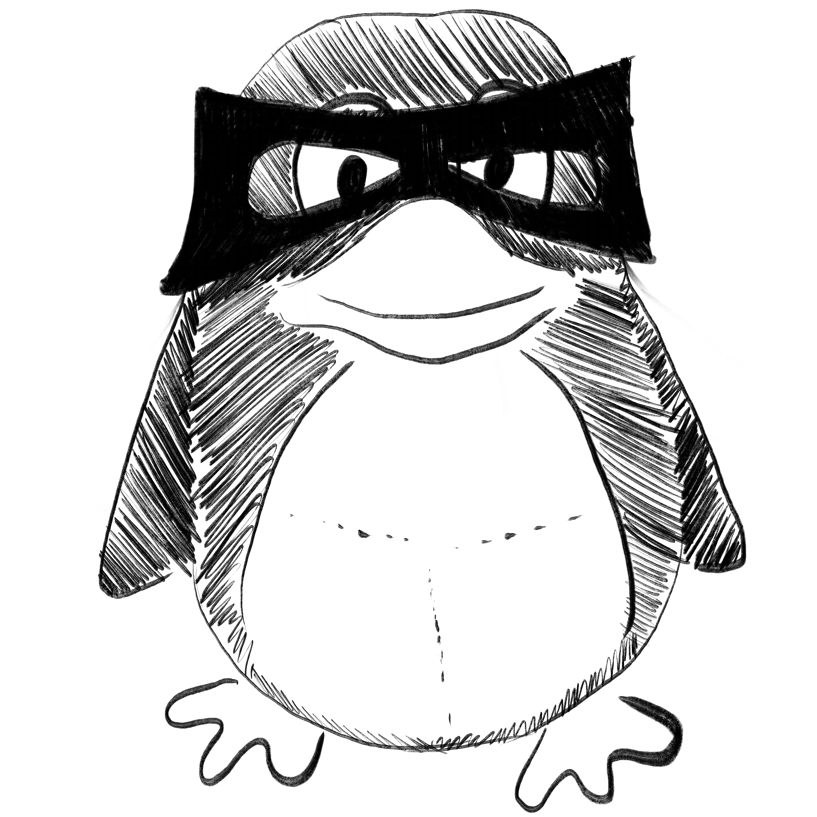
A self-adaptive hardware with resistive switching synapses for experience-based neurocomputing.
In Nature communications ; h5-index 260.0
Bianchi S, Muñoz-Martin I, Covi E, Bricalli A, Piccolboni G, Regev A, Molas G, Nodin J F, Andrieu F, Ielmini D
2023-Mar-21
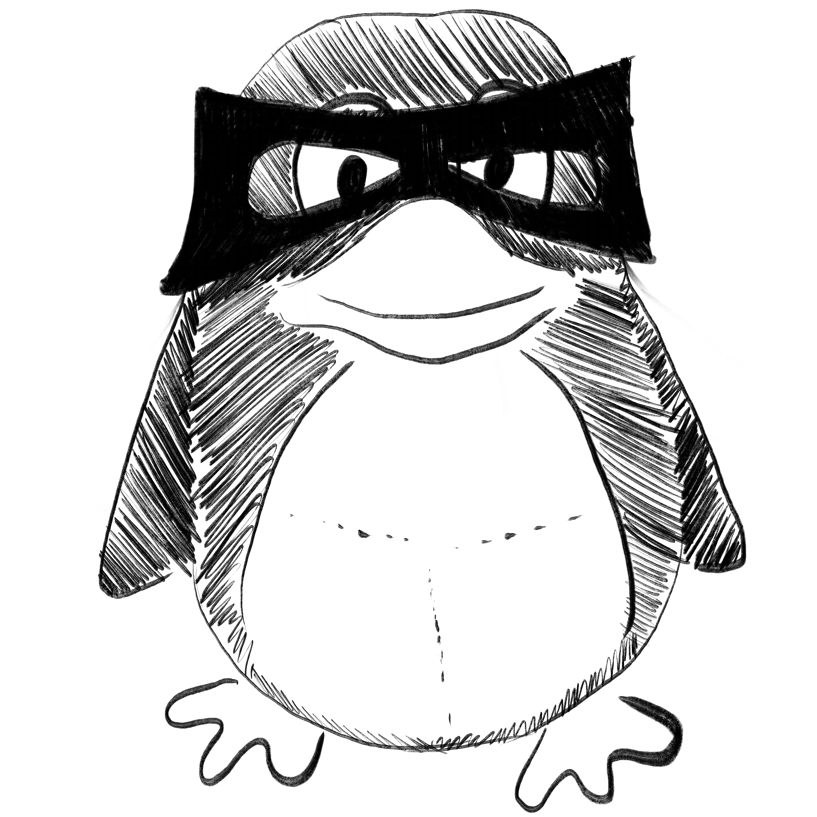
Accelerating network layouts using graph neural networks.
In Nature communications ; h5-index 260.0
Both Csaba, Dehmamy Nima, Yu Rose, Barabási Albert-László
2023-Mar-21
Clinical and genetic associations of deep learning-derived cardiac magnetic resonance-based left ventricular mass.
In Nature communications ; h5-index 260.0
Khurshid Shaan, Lazarte Julieta, Pirruccello James P, Weng Lu-Chen, Choi Seung Hoan, Hall Amelia W, Wang Xin, Friedman Samuel F, Nauffal Victor, Biddinger Kiran J, Aragam Krishna G, Batra Puneet, Ho Jennifer E, Philippakis Anthony A, Ellinor Patrick T, Lubitz Steven A
2023-Mar-21
Multiomic signatures of body mass index identify heterogeneous health phenotypes and responses to a lifestyle intervention.
In Nature medicine ; h5-index 170.0
Watanabe Kengo, Wilmanski Tomasz, Diener Christian, Earls John C, Zimmer Anat, Lincoln Briana, Hadlock Jennifer J, Lovejoy Jennifer C, Gibbons Sean M, Magis Andrew T, Hood Leroy, Price Nathan D, Rappaport Noa
2023-Mar-20
AI Cardiac MRI Scar Analysis Aids Prediction of Major Arrhythmic Events in the Multicenter DERIVATE Registry.
In Radiology ; h5-index 91.0
Ghanbari Fahime, Joyce Thomas, Lorenzoni Valentina, Guaricci Andrea I, Pavon Anna-Giulia, Fusini Laura, Andreini Daniele, Rabbat Mark G, Aquaro Giovanni Donato, Abete Raffaele, Bogaert Jan, Camastra Giovanni, Carigi Samuela, Carrabba Nazario, Casavecchia Grazia, Censi Stefano, Cicala Gloria, De Cecco Carlo N, De Lazzari Manuel, Di Giovine Gabriella, Di Roma Mauro, Focardi Marta, Gaibazzi Nicola, Gismondi Annalaura, Gravina Matteo, Lanzillo Chiara, Lombardi Massimo, Lozano-Torres Jordi, Masi Ambra, Moro Claudio, Muscogiuri Giuseppe, Nese Alberto, Pradella Silvia, Sbarbati Stefano, Schoepf U Joseph, Valentini Adele, Crelier Gérard, Masci Pier Giorgio, Pontone Gianluca, Kozerke Sebastian, Schwitter Juerg
2023-Mar-21
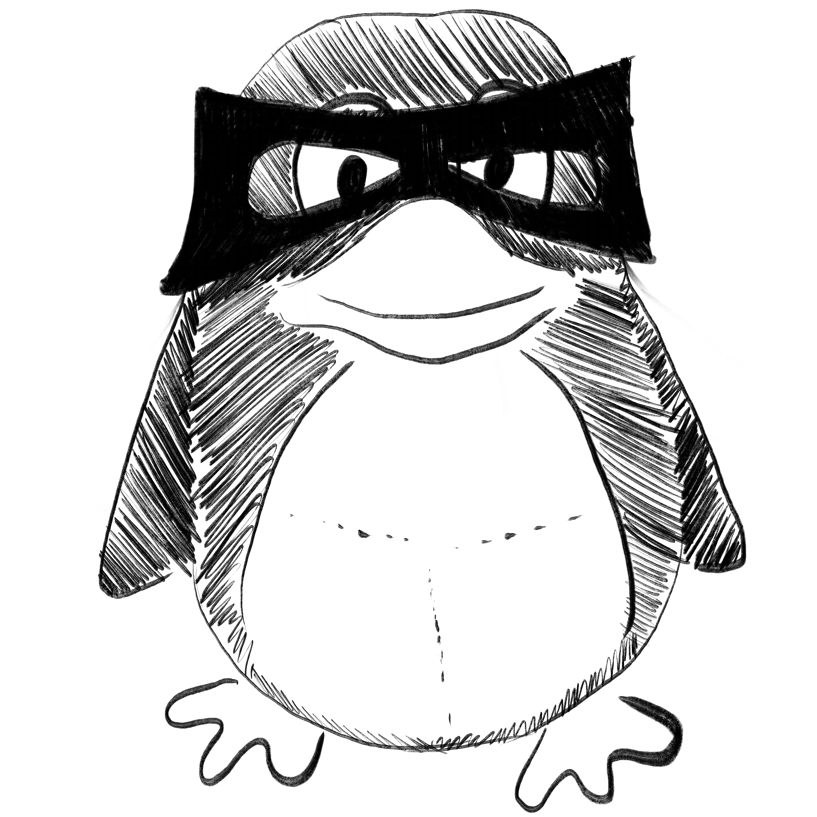
Metheor: Ultrafast DNA methylation heterogeneity calculation from bisulfite read alignments.
In PLoS computational biology
Lee Dohoon, Koo Bonil, Yang Jeewon, Kim Sun
2023-Mar-20
PCR-like performance of rapid test with permselective tunable nanotrap.
In Nature communications ; h5-index 260.0
Park Seong Jun, Lee Seungmin, Lee Dongtak, Lee Na Eun, Park Jeong Soo, Hong Ji Hye, Jang Jae Won, Kim Hyunji, Roh Seokbeom, Lee Gyudo, Lee Dongho, Cho Sung-Yeon, Park Chulmin, Lee Dong-Gun, Lee Raeseok, Nho Dukhee, Yoon Dae Sung, Yoo Yong Kyoung, Lee Jeong Hoon
2023-Mar-18
PCR-like performance of rapid test with permselective tunable nanotrap.
In Nature communications ; h5-index 260.0
Park Seong Jun, Lee Seungmin, Lee Dongtak, Lee Na Eun, Park Jeong Soo, Hong Ji Hye, Jang Jae Won, Kim Hyunji, Roh Seokbeom, Lee Gyudo, Lee Dongho, Cho Sung-Yeon, Park Chulmin, Lee Dong-Gun, Lee Raeseok, Nho Dukhee, Yoon Dae Sung, Yoo Yong Kyoung, Lee Jeong Hoon
2023-Mar-18
Recalibration of a Deep Learning Model for Low-Dose Computed Tomographic Images to Inform Lung Cancer Screening Intervals.
In JAMA network open
IMPORTANCE :
OBJECTIVE :
DESIGN, SETTING, AND PARTICIPANTS :
EXPOSURES :
MAIN OUTCOMES AND MEASURES :
RESULTS :
CONCLUSIONS AND RELEVANCE :
Landy Rebecca, Wang Vivian L, Baldwin David R, Pinsky Paul F, Cheung Li C, Castle Philip E, Skarzynski Martin, Robbins Hilary A, Katki Hormuzd A
2023-Mar-01
A longitudinal circulating tumor DNA-based model associated with survival in metastatic non-small-cell lung cancer.
In Nature medicine ; h5-index 170.0
Assaf Zoe June F, Zou Wei, Fine Alexander D, Socinski Mark A, Young Amanda, Lipson Doron, Freidin Jonathan F, Kennedy Mark, Polisecki Eliana, Nishio Makoto, Fabrizio David, Oxnard Geoffrey R, Cummings Craig, Rode Anja, Reck Martin, Patil Namrata S, Lee Mark, Shames David S, Schulze Katja
2023-Mar-16
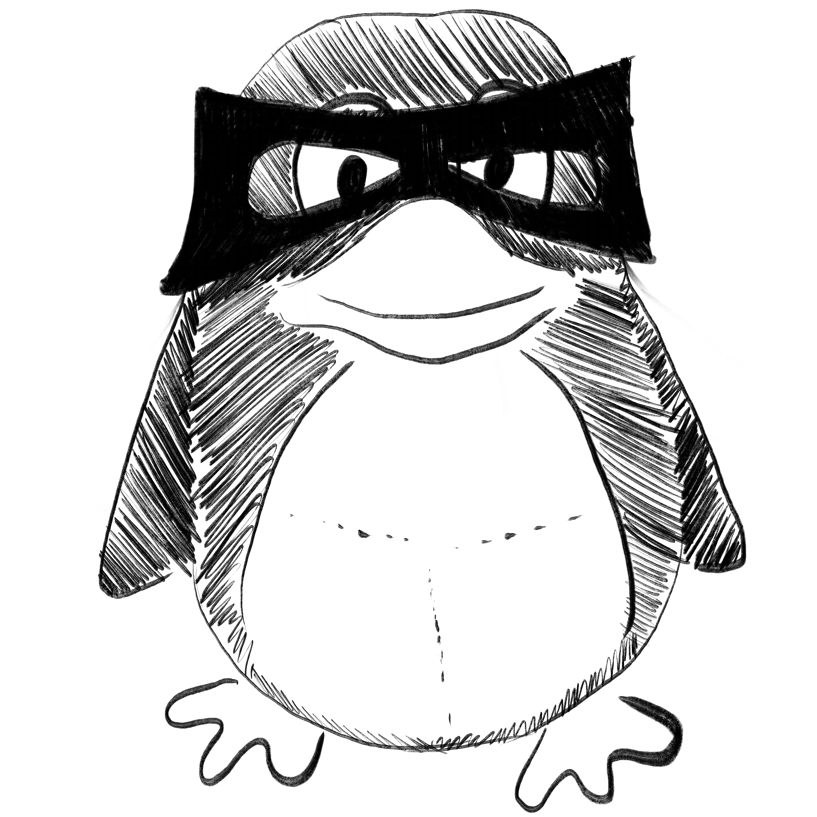
Heterogeneous intercalated metal-organic framework active materials for fast-charging non-aqueous Li-ion capacitors.
In Nature communications ; h5-index 260.0
Ogihara Nobuhiro, Hasegawa Masaki, Kumagai Hitoshi, Mikita Riho, Nagasako Naoyuki
2023-Mar-16
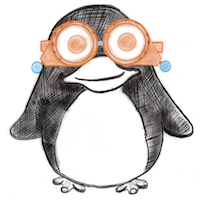
Visual electrophysiology and "the potential of the potentials".
In Eye (London, England) ; h5-index 41.0
Mahroo Omar A
2023-Mar-16
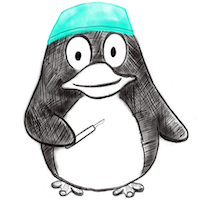
Artificial Intelligence for Hip Fracture Detection and Outcome Prediction: A Systematic Review and Meta-analysis.
In JAMA network open
IMPORTANCE :
OBJECTIVE :
DATA SOURCES :
STUDY SELECTION :
DATA EXTRACTION AND SYNTHESIS :
MAIN OUTCOMES AND MEASURES :
RESULTS :
CONCLUSIONS AND RELEVANCE :
Lex Johnathan R, Di Michele Joseph, Koucheki Robert, Pincus Daniel, Whyne Cari, Ravi Bheeshma
2023-Mar-01
Development of a Machine Learning Model to Estimate US Firearm Homicides in Near Real Time.
In JAMA network open
IMPORTANCE :
OBJECTIVE :
DESIGN, SETTING, AND PARTICIPANTS :
MAIN OUTCOMES AND MEASURES :
RESULTS :
CONCLUSIONS AND RELEVANCE :
Swedo Elizabeth A, Alic Alen, Law Royal K, Sumner Steven A, Chen May S, Zwald Marissa L, Van Dyke Miriam E, Bowen Daniel A, Mercy James A
2023-Mar-01
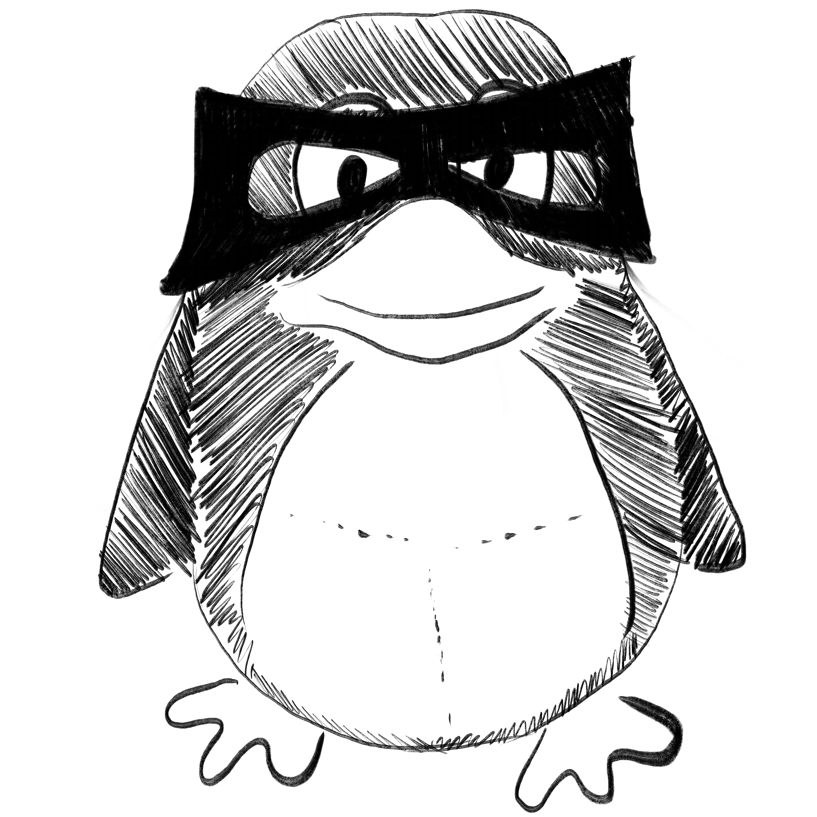
Machine Learning-Based Prediction of Attention-Deficit/Hyperactivity Disorder and Sleep Problems With Wearable Data in Children.
In JAMA network open
IMPORTANCE :
OBJECTIVE :
DESIGN, SETTING, AND PARTICIPANTS :
MAIN OUTCOMES AND MEASURES :
RESULTS :
CONCLUSIONS AND RELEVANCE :
Kim Won-Pyo, Kim Hyun-Jin, Pack Seung Pil, Lim Jae-Hyun, Cho Chul-Hyun, Lee Heon-Jeong
2023-Mar-01
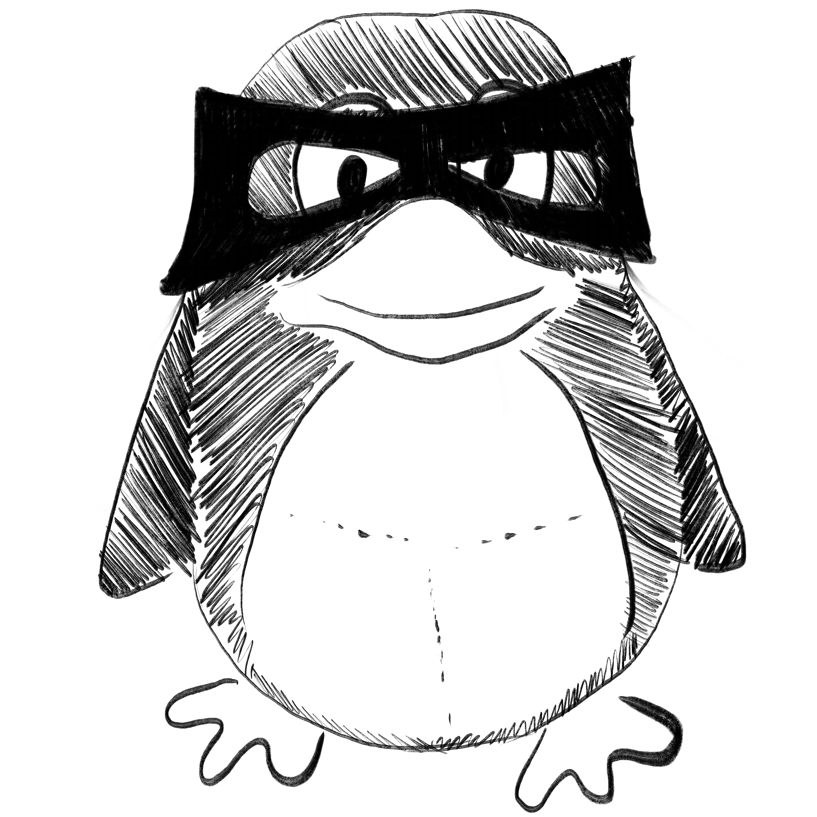
Attention is not all you need: the complicated case of ethically using large language models in healthcare and medicine.
In EBioMedicine
Harrer Stefan
2023-Mar-14
AI ethics, AI trustworthiness, Augmented human intelligence, Foundation models, Generative artificial intelligence, Information management, Large language models
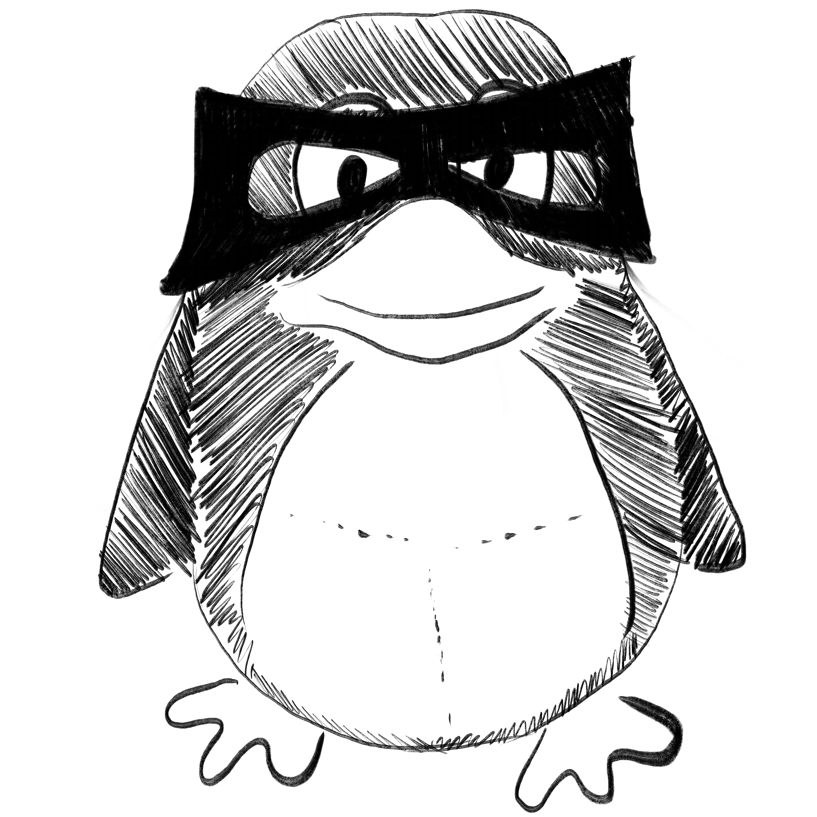
Revealing influencing factors on global waste distribution via deep-learning based dumpsite detection from satellite imagery.
In Nature communications ; h5-index 260.0
Sun Xian, Yin Dongshuo, Qin Fei, Yu Hongfeng, Lu Wanxuan, Yao Fanglong, He Qibin, Huang Xingliang, Yan Zhiyuan, Wang Peijin, Deng Chubo, Liu Nayu, Yang Yiran, Liang Wei, Wang Ruiping, Wang Cheng, Yokoya Naoto, Hänsch Ronny, Fu Kun
2023-Mar-15
Investigation of liquid biopsy analytes in peripheral blood of individuals after SARS-CoV-2 infection.
In EBioMedicine
BACKGROUND :
METHODS :
FINDINGS :
INTERPRETATION :
FUNDING :
Qi Elizabeth, Courcoubetis George, Liljegren Emmett, Herrera Ergueen, Nguyen Nathalie, Nadri Maimoona, Ghandehari Sara, Kazemian Elham, Reckamp Karen L, Merin Noah M, Merchant Akil, Mason Jeremy, Figueiredo Jane C, Shishido Stephanie N, Kuhn Peter
2023-Mar-13
COVID-19, Liquid biopsy, Long COVID, Post-COVID sequelae, Post-acute COVID-19 syndrome (PACS), SARS-CoV-2
Investigation of liquid biopsy analytes in peripheral blood of individuals after SARS-CoV-2 infection.
In EBioMedicine
BACKGROUND :
METHODS :
FINDINGS :
INTERPRETATION :
FUNDING :
Qi Elizabeth, Courcoubetis George, Liljegren Emmett, Herrera Ergueen, Nguyen Nathalie, Nadri Maimoona, Ghandehari Sara, Kazemian Elham, Reckamp Karen L, Merin Noah M, Merchant Akil, Mason Jeremy, Figueiredo Jane C, Shishido Stephanie N, Kuhn Peter
2023-Mar-13
COVID-19, Liquid biopsy, Long COVID, Post-COVID sequelae, Post-acute COVID-19 syndrome (PACS), SARS-CoV-2
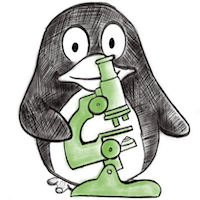
1,2,4,5-Tetrazine-tethered probes for fluorogenically imaging superoxide in live cells with ultrahigh specificity.
In Nature communications ; h5-index 260.0
Jiang Xuefeng, Li Min, Wang Yule, Wang Chao, Wang Yingchao, Shen Tianruo, Shen Lili, Liu Xiaogang, Wang Yi, Li Xin
2023-Mar-14
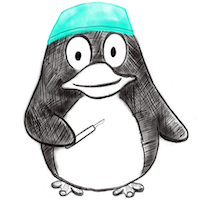
Pathologist Validation of a Machine Learning-Derived Feature for Colon Cancer Risk Stratification.
In JAMA network open
IMPORTANCE :
OBJECTIVE :
DESIGN, SETTING, AND PARTICIPANTS :
MAIN OUTCOMES AND MEASURES :
RESULTS :
CONCLUSIONS AND RELEVANCE :
L’Imperio Vincenzo, Wulczyn Ellery, Plass Markus, Müller Heimo, Tamini Nicolò, Gianotti Luca, Zucchini Nicola, Reihs Robert, Corrado Greg S, Webster Dale R, Peng Lily H, Chen Po-Hsuan Cameron, Lavitrano Marialuisa, Liu Yun, Steiner David F, Zatloukal Kurt, Pagni Fabio
2023-Mar-01
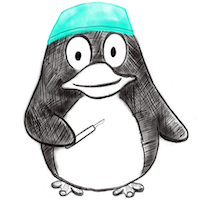
An artificial intelligence approach for predicting death or organ failure after hospitalization for COVID-19: development of a novel risk prediction tool and comparisons with ISARIC-4C, CURB-65, qSOFA, and MEWS scoring systems.
In Respiratory research ; h5-index 45.0
BACKGROUND :
METHODS :
RESULTS :
CONCLUSION :
Kwok Stephen Wai Hang, Wang Guanjin, Sohel Ferdous, Kashani Kianoush B, Zhu Ye, Wang Zhen, Antpack Eduardo, Khandelwal Kanika, Pagali Sandeep R, Nanda Sanjeev, Abdalrhim Ahmed D, Sharma Umesh M, Bhagra Sumit, Dugani Sagar, Takahashi Paul Y, Murad Mohammad H, Yousufuddin Mohammed
2023-Mar-13
COVID-19, Machine learning algorithms, Mortality, Organ failure, Prediction models
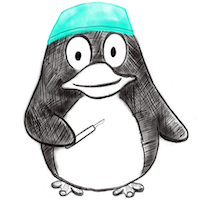
An artificial intelligence approach for predicting death or organ failure after hospitalization for COVID-19: development of a novel risk prediction tool and comparisons with ISARIC-4C, CURB-65, qSOFA, and MEWS scoring systems.
In Respiratory research ; h5-index 45.0
BACKGROUND :
METHODS :
RESULTS :
CONCLUSION :
Kwok Stephen Wai Hang, Wang Guanjin, Sohel Ferdous, Kashani Kianoush B, Zhu Ye, Wang Zhen, Antpack Eduardo, Khandelwal Kanika, Pagali Sandeep R, Nanda Sanjeev, Abdalrhim Ahmed D, Sharma Umesh M, Bhagra Sumit, Dugani Sagar, Takahashi Paul Y, Murad Mohammad H, Yousufuddin Mohammed
2023-Mar-13
COVID-19, Machine learning algorithms, Mortality, Organ failure, Prediction models
A non-antibiotic-disrupted gut microbiome is associated with clinical responses to CD19-CAR-T cell cancer immunotherapy.
In Nature medicine ; h5-index 170.0
Stein-Thoeringer Christoph K, Saini Neeraj Y, Zamir Eli, Blumenberg Viktoria, Schubert Maria-Luisa, Mor Uria, Fante Matthias A, Schmidt Sabine, Hayase Eiko, Hayase Tomo, Rohrbach Roman, Chang Chia-Chi, McDaniel Lauren, Flores Ivonne, Gaiser Rogier, Edinger Matthias, Wolff Daniel, Heidenreich Martin, Strati Paolo, Nair Ranjit, Chihara Dai, Fayad Luis E, Ahmed Sairah, Iyer Swaminathan P, Steiner Raphael E, Jain Preetesh, Nastoupil Loretta J, Westin Jason, Arora Reetakshi, Wang Michael L, Turner Joel, Menges Meghan, Hidalgo-Vargas Melanie, Reid Kayla, Dreger Peter, Schmitt Anita, Müller-Tidow Carsten, Locke Frederick L, Davila Marco L, Champlin Richard E, Flowers Christopher R, Shpall Elizabeth J, Poeck Hendrik, Neelapu Sattva S, Schmitt Michael, Subklewe Marion, Jain Michael D, Jenq Robert R, Elinav Eran
2023-Mar-13
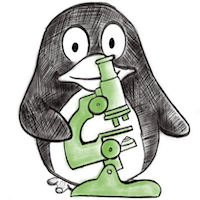
Interpretable and context-free deconvolution of multi-scale whole transcriptomic data with UniCell deconvolve.
In Nature communications ; h5-index 260.0
Charytonowicz Daniel, Brody Rachel, Sebra Robert
2023-Mar-11
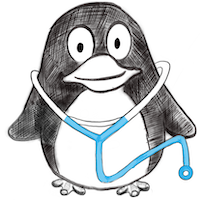
Effects of gastroesophageal reflux disease treatment with proton pump inhibitors on the risk of acute exacerbation and pneumonia in patients with COPD.
In Respiratory research ; h5-index 45.0
BACKGROUND :
METHODS :
RESULTS :
CONCLUSIONS :
Kang Jieun, Lee Rugyeom, Lee Sei Won
2023-Mar-11
Acute exacerbation, Chronic obstructive pulmonary disease, Gastroesophageal reflux disease, Pneumonia, Proton pump inhibitor
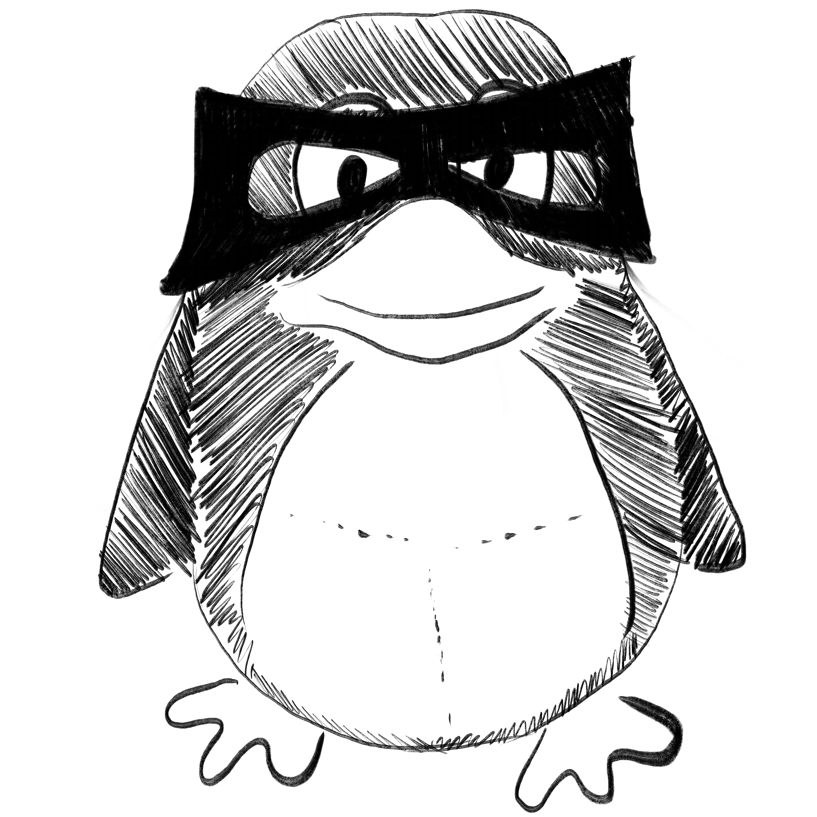
Smartphone-based platforms implementing microfluidic detection with image-based artificial intelligence.
In Nature communications ; h5-index 260.0
Wang Bangfeng, Li Yiwei, Zhou Mengfan, Han Yulong, Zhang Mingyu, Gao Zhaolong, Liu Zetai, Chen Peng, Du Wei, Zhang Xingcai, Feng Xiaojun, Liu Bi-Feng
2023-Mar-11
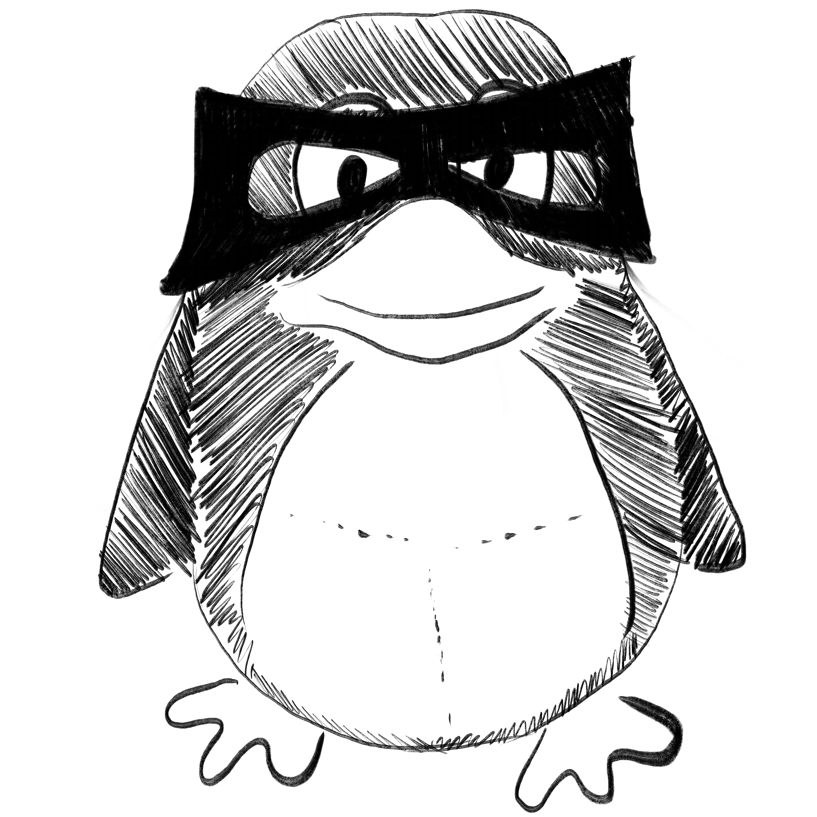
A deep intronic TCTN2 variant activating a cryptic exon predicted by SpliceRover in a patient with Joubert syndrome.
In Journal of human genetics
Hiraide Takuya, Shimizu Kenji, Okumura Yoshinori, Miyamoto Sachiko, Nakashima Mitsuko, Ogata Tsutomu, Saitsu Hirotomo
2023-Mar-10
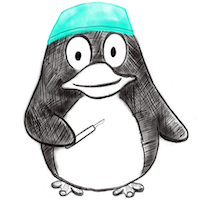
Mechanism of Injury and Age Predict Operative Intervention in Pediatric Perineal Injury.
In Pediatric emergency care
OBJECTIVES :
METHODS :
RESULTS :
CONCLUSIONS :
McLaughlin Christopher J, Martin Kathryn L
2023-Mar-07
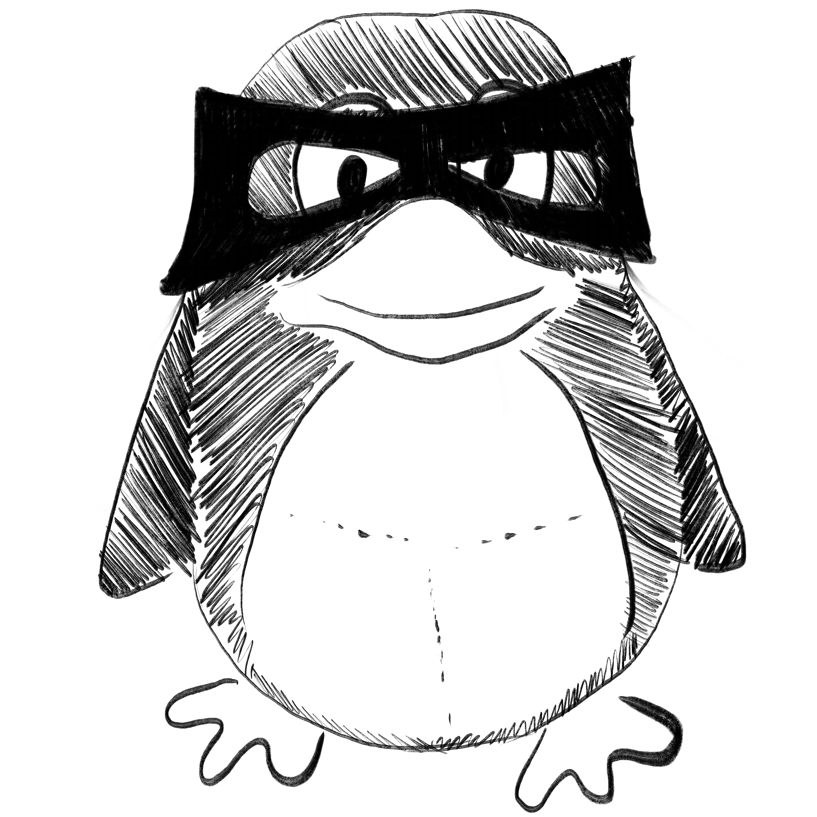
Human-machine collaboration for improving semiconductor process development.
In Nature ; h5-index 368.0
Kanarik Keren J, Osowiecki Wojciech T, Lu Yu Joe, Talukder Dipongkar, Roschewsky Niklas, Park Sae Na, Kamon Mattan, Fried David M, Gottscho Richard A
2023-Mar-08
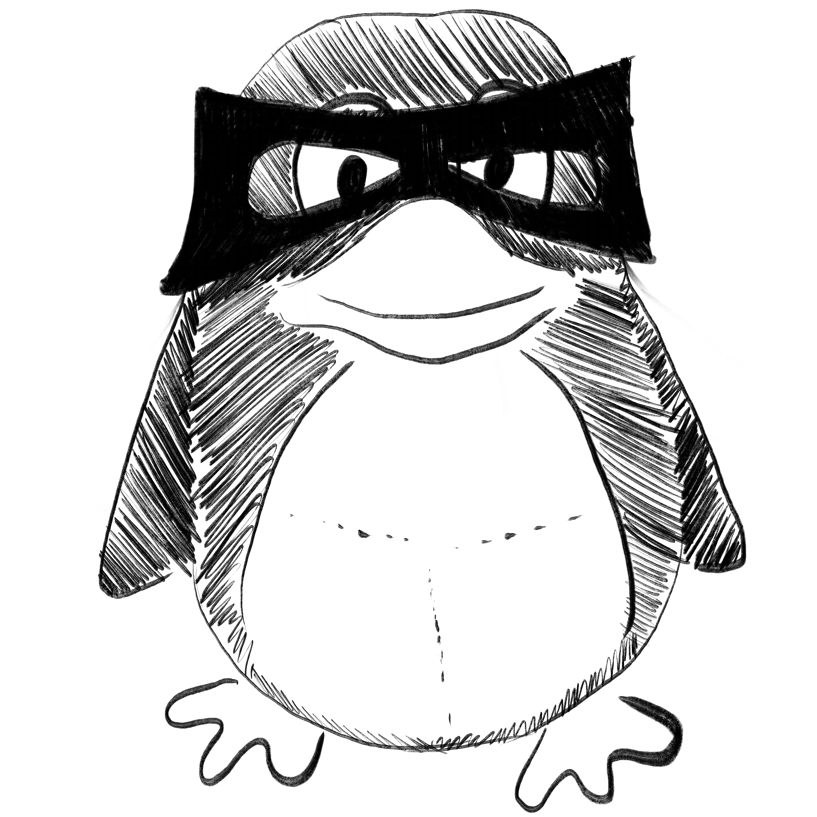
Diagnosis of Alzheimer's Disease and Tauopathies on Whole Slide Histopathology Images Using a Weakly Supervised Deep Learning Algorithm.
In Laboratory investigation; a journal of technical methods and pathology ; h5-index 42.0
Kim Minji, Sekiya Hiroaki, Yao Gary, Martin Nicholas B, Castanedes-Casey Monica, Dickson Dennis W, Hwang Tae Hyun, Koga Shunsuke
2023-Mar-06
Alzheimer’s disease, CLAM, Grad-CAM, Pick’s disease, corticobasal degeneration, globular glial tauopathy, neuropathology, progressive supranuclear palsy, tauopathy, weakly supervised deep learning
Autonomous Chest Radiograph Reporting Using AI: Estimation of Clinical Impact.
In Radiology ; h5-index 91.0
Plesner Louis L, Müller Felix C, Nybing Janus D, Laustrup Lene C, Rasmussen Finn, Nielsen Olav W, Boesen Mikael, Andersen Michael B
2023-Mar-07
Deep Learning for Head and Neck CT Angiography: Stenosis and Plaque Classification.
In Radiology ; h5-index 91.0
Fu Fan, Shan Yi, Yang Guang, Zheng Chao, Zhang Miao, Rong Dongdong, Wang Ximing, Lu Jie
2023-Mar-07
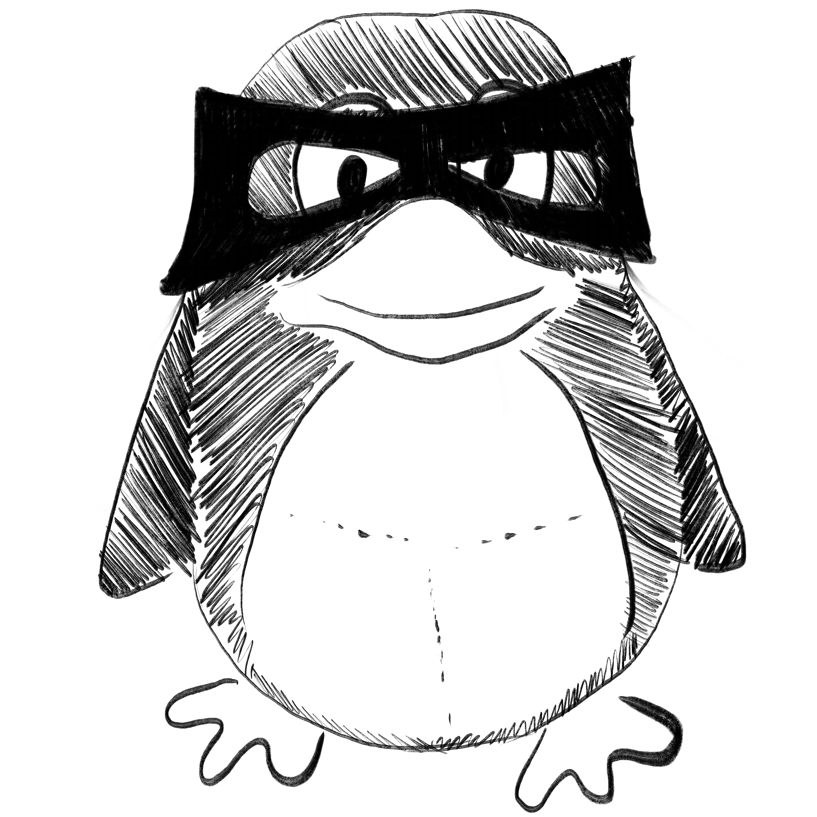
Predicting functional effects of ion channel variants using new phenotypic machine learning methods.
In PLoS computational biology
Boßelmann Christian Malte, Hedrich Ulrike B S, Lerche Holger, Pfeifer Nico
2023-Mar-06
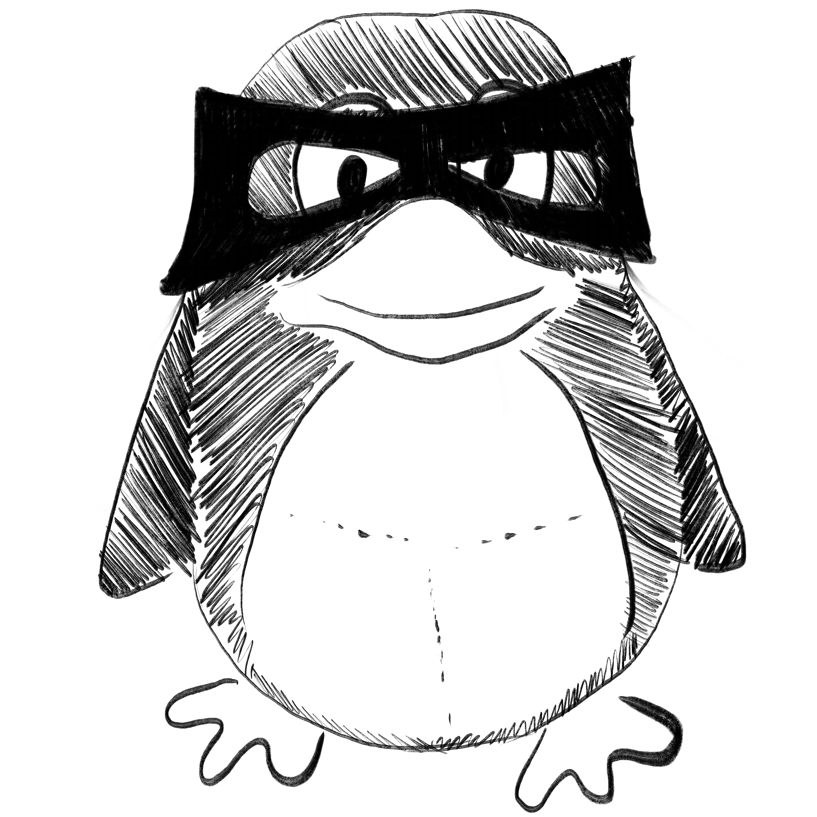
CustOmics: A versatile deep-learning based strategy for multi-omics integration.
In PLoS computational biology
Benkirane Hakim, Pradat Yoann, Michiels Stefan, Cournède Paul-Henry
2023-Mar-06
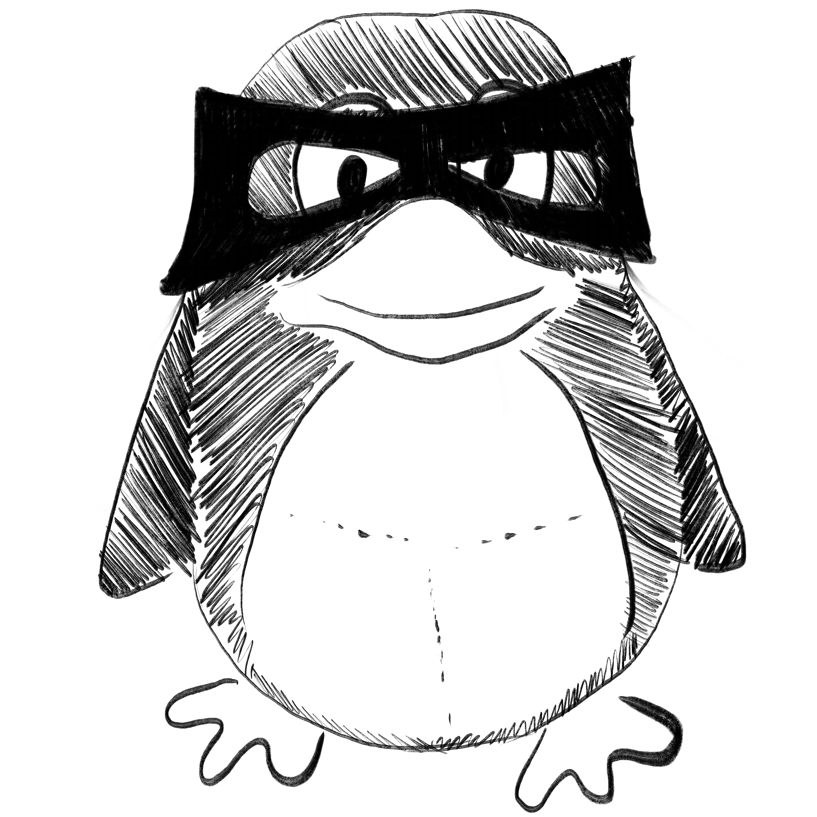
Evaluation of Risk of Bias in Neuroimaging-Based Artificial Intelligence Models for Psychiatric Diagnosis: A Systematic Review.
In JAMA network open
IMPORTANCE :
OBJECTIVE :
EVIDENCE REVIEW :
FINDINGS :
CONCLUSIONS AND RELEVANCE :
Chen Zhiyi, Liu Xuerong, Yang Qingwu, Wang Yan-Jiang, Miao Kuan, Gong Zheng, Yu Yang, Leonov Artemiy, Liu Chunlei, Feng Zhengzhi, Chuan-Peng Hu
2023-Mar-01
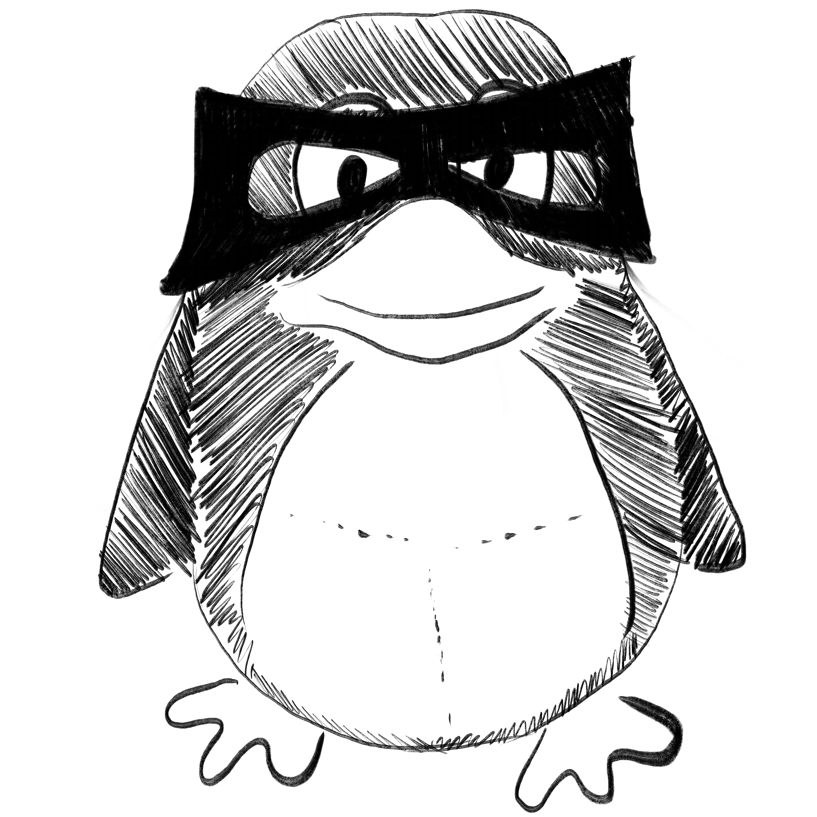
An AI-Aided Diagnostic Framework for Hematologic Neoplasms Based on Morphologic Features and Medical Expertise.
In Laboratory investigation; a journal of technical methods and pathology ; h5-index 42.0
Li Nan, Fan Liquan, Xu Hang, Zhang Xiwen, Bai Zanzhou, Li Miaohui, Xiong Shumin, Jiang Lu, Yang Jie, Chen Saijuan, Qiao Yu, Chen Bing
2023-Jan-10
artificial intelligence, bone marrow morphology, diagnosis, hematology, multimodal features
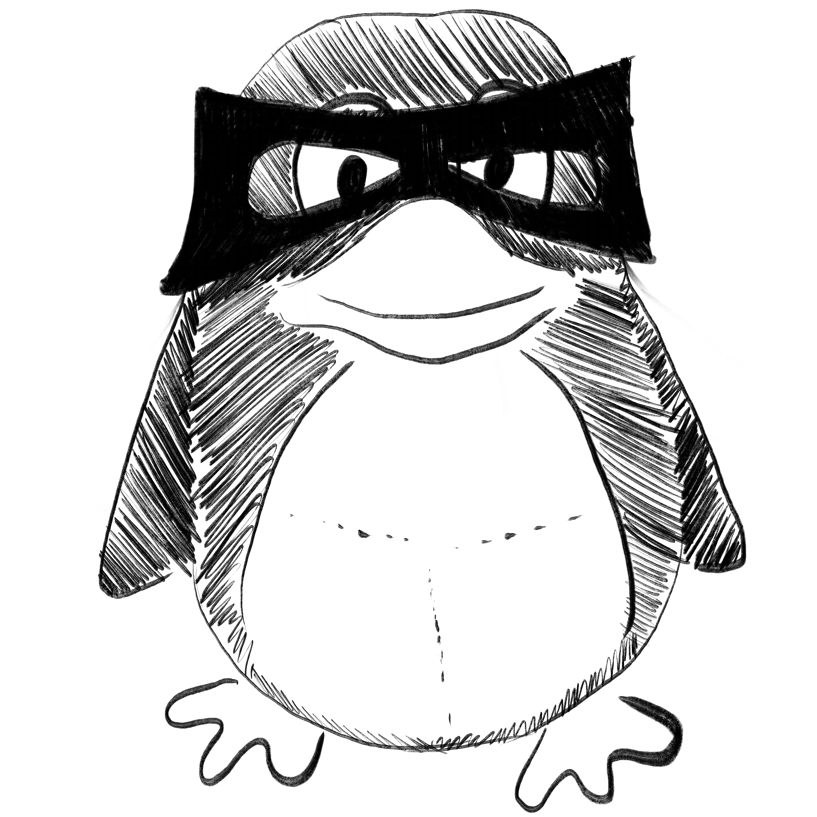
Revealing the importance of prenatal gut microbiome in offspring neurodevelopment in humans.
In EBioMedicine
BACKGROUND :
METHODS :
FINDINGS :
INTERPRETATION :
FUNDING :
Sun Zheng, Lee-Sarwar Kathleen, Kelly Rachel S, Lasky-Su Jessica A, Litonjua Augusto A, Weiss Scott T, Liu Yang-Yu
2023-Mar-01
Ages and stages questionnaire, Childhood neurodevelopment, Early-life gut microbiome, Maternal gut microbiome
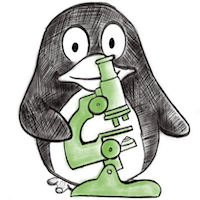
Integrated Cytometry With Machine Learning Applied to High-Content Imaging of Human Kidney Tissue for In Situ Cell Classification and Neighborhood Analysis.
In Laboratory investigation; a journal of technical methods and pathology ; h5-index 42.0
Winfree Seth, McNutt Andrew T, Khochare Suraj, Borgard Tyler J, Barwinska Daria, Sabo Angela R, Ferkowicz Michael J, Williams James C, Lingeman James E, Gulbronson Connor J, Kelly Katherine J, Sutton Timothy A, Dagher Pierre C, Eadon Michael T, Dunn Kenneth W, El-Achkar Tarek M
2023-Feb-04
3D, CODEX, FIJI is just ImageJ, confocal microscopy, cytometry, machine learning
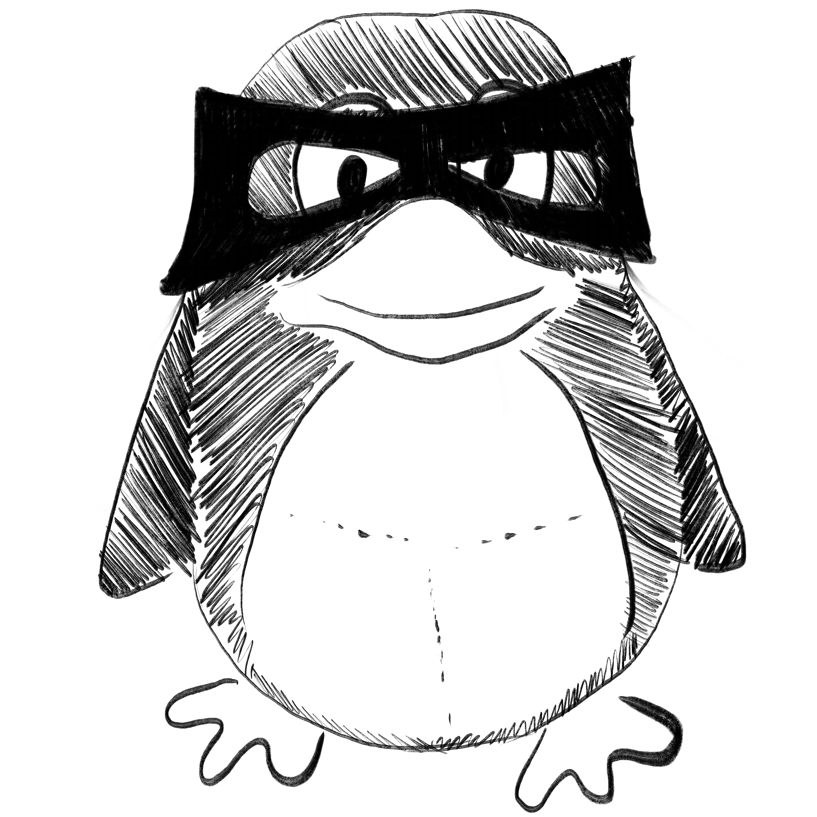
Cryo-EM structure of human heptameric pannexin 2 channel.
In Nature communications ; h5-index 260.0
Zhang Hang, Wang Shiyu, Zhang Zhenzhen, Hou Mengzhuo, Du Chunyu, Zhao Zhenye, Vogel Horst, Li Zhifang, Yan Kaige, Zhang Xiaokang, Lu Jianping, Liang Yujie, Yuan Shuguang, Wang Daping, Zhang Huawei
2023-Mar-03
Artificial intelligence (AI) for breast cancer screening: BreastScreen population-based cohort study of cancer detection.
In EBioMedicine
BACKGROUND :
METHODS :
FINDINGS :
INTERPRETATION :
FUNDING :
Marinovich M Luke, Wylie Elizabeth, Lotter William, Lund Helen, Waddell Andrew, Madeley Carolyn, Pereira Gavin, Houssami Nehmat
2023-Feb-28
Artificial intelligence, Breast neoplasms, Diagnostic screening programs, Sensitivity and specificity
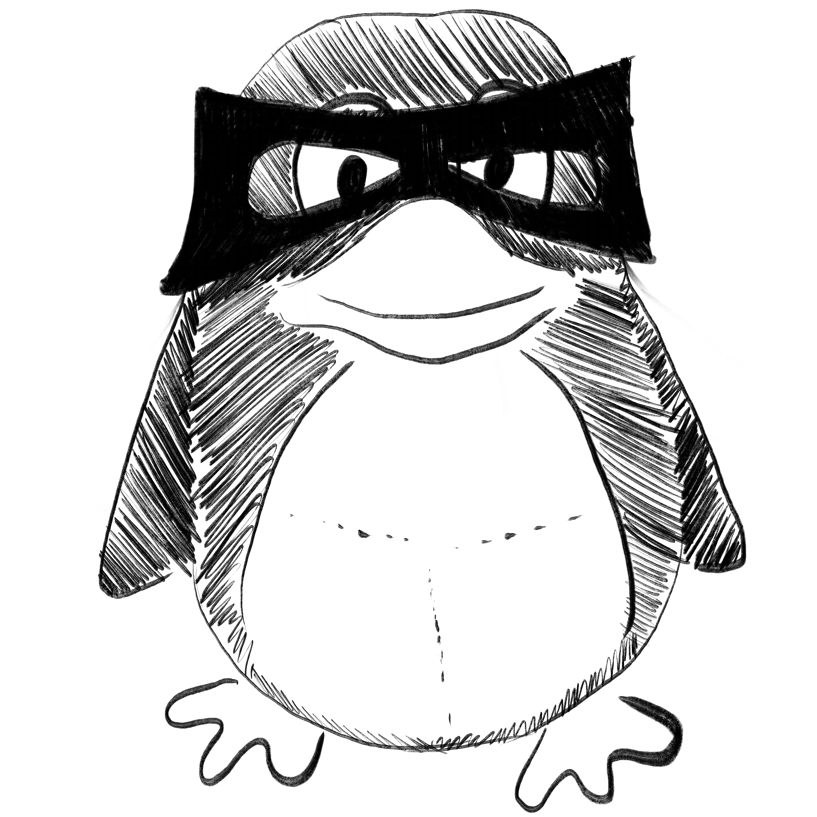
Assessment of Natural Language Processing of Electronic Health Records to Measure Goals-of-Care Discussions as a Clinical Trial Outcome.
In JAMA network open
IMPORTANCE :
OBJECTIVE :
DESIGN, SETTING, AND PARTICIPANTS :
MAIN OUTCOMES AND MEASURES :
RESULTS :
CONCLUSIONS AND RELEVANCE :
Lee Robert Y, Kross Erin K, Torrence Janaki, Li Kevin S, Sibley James, Cohen Trevor, Lober William B, Engelberg Ruth A, Curtis J Randall
2023-Mar-01
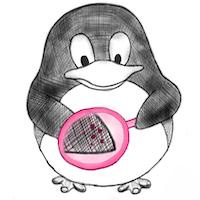
A deep-learning algorithm to classify skin lesions from mpox virus infection.
In Nature medicine ; h5-index 170.0
Thieme Alexander H, Zheng Yuanning, Machiraju Gautam, Sadee Chris, Mittermaier Mirja, Gertler Maximilian, Salinas Jorge L, Srinivasan Krithika, Gyawali Prashnna, Carrillo-Perez Francisco, Capodici Angelo, Uhlig Maximilian, Habenicht Daniel, Löser Anastassia, Kohler Maja, Schuessler Maximilian, Kaul David, Gollrad Johannes, Ma Jackie, Lippert Christoph, Billick Kendall, Bogoch Isaac, Hernandez-Boussard Tina, Geldsetzer Pascal, Gevaert Olivier
2023-Mar-02
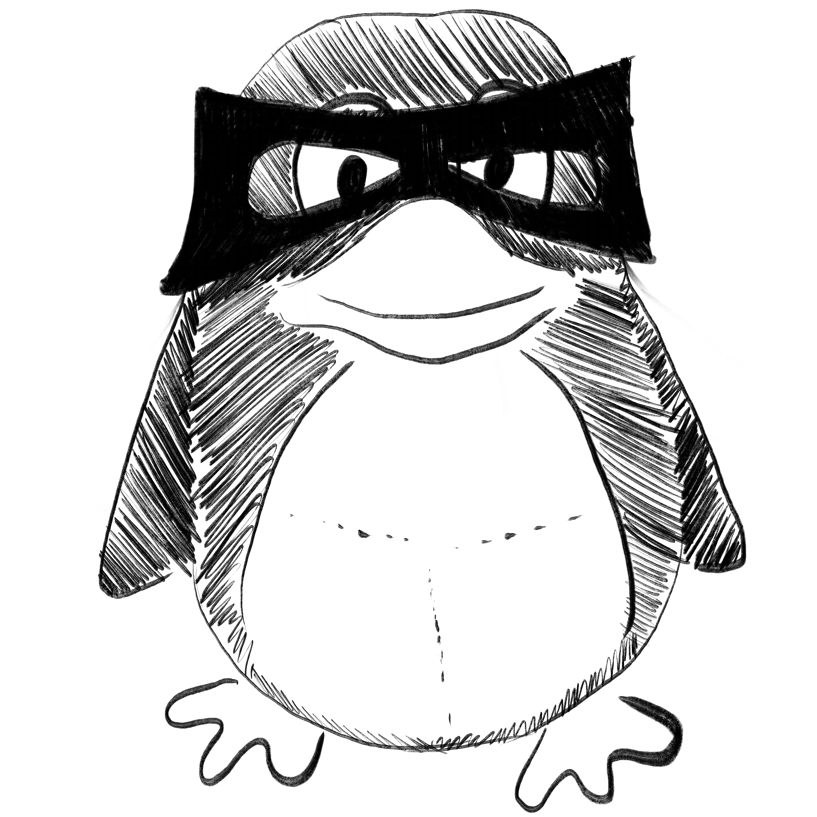
Sub-continental-scale carbon stocks of individual trees in African drylands.
In Nature ; h5-index 368.0
Tucker Compton, Brandt Martin, Hiernaux Pierre, Kariryaa Ankit, Rasmussen Kjeld, Small Jennifer, Igel Christian, Reiner Florian, Melocik Katherine, Meyer Jesse, Sinno Scott, Romero Eric, Glennie Erin, Fitts Yasmin, Morin August, Pinzon Jorge, McClain Devin, Morin Paul, Porter Claire, Loeffler Shane, Kergoat Laurent, Issoufou Bil-Assanou, Savadogo Patrice, Wigneron Jean-Pierre, Poulter Benjamin, Ciais Philippe, Kaufmann Robert, Myneni Ranga, Saatchi Sassan, Fensholt Rasmus
2023-Mar
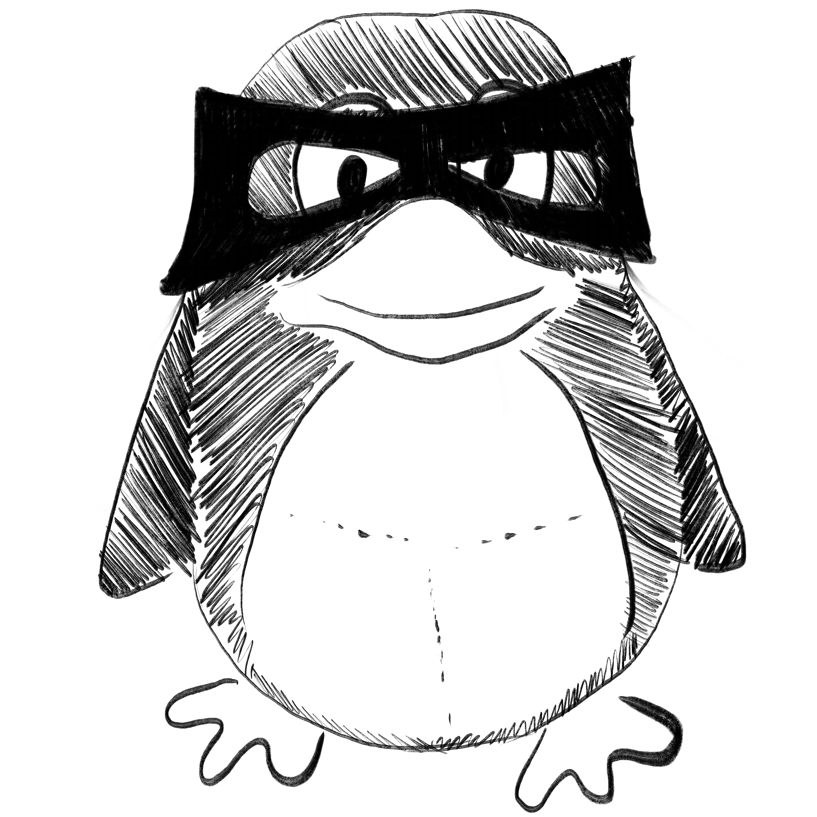
Prediction of transition state structures of gas-phase chemical reactions via machine learning.
In Nature communications ; h5-index 260.0
Choi Sunghwan
2023-Mar-01
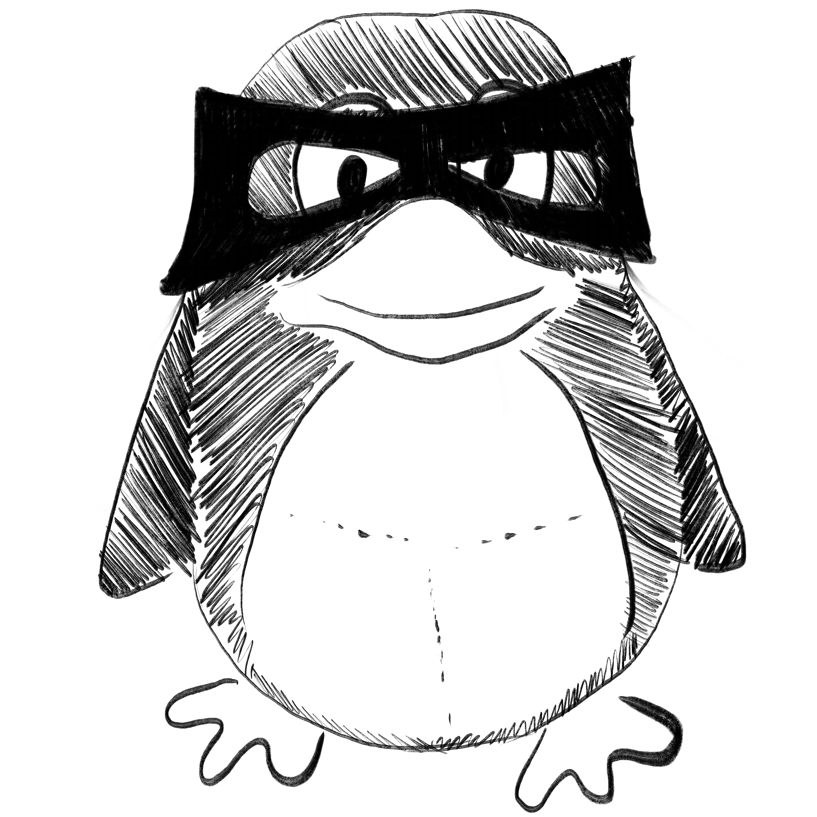
Automatic and accurate ligand structure determination guided by cryo-electron microscopy maps.
In Nature communications ; h5-index 260.0
Muenks Andrew, Zepeda Samantha, Zhou Guangfeng, Veesler David, DiMaio Frank
2023-Mar-01
Circulating tumor DNA reveals complex biological features with clinical relevance in metastatic breast cancer.
In Nature communications ; h5-index 260.0
Prat Aleix, Brasó-Maristany Fara, Martínez-Sáez Olga, Sanfeliu Esther, Xia Youli, Bellet Meritxell, Galván Patricia, Martínez Débora, Pascual Tomás, Marín-Aguilera Mercedes, Rodríguez Anna, Chic Nuria, Adamo Barbara, Paré Laia, Vidal Maria, Margelí Mireia, Ballana Ester, Gómez-Rey Marina, Oliveira Mafalda, Felip Eudald, Matito Judit, Sánchez-Bayona Rodrigo, Suñol Anna, Saura Cristina, Ciruelos Eva, Tolosa Pablo, Muñoz Montserrat, González-Farré Blanca, Villagrasa Patricia, Parker Joel S, Perou Charles M, Vivancos Ana
2023-Mar-01
Development and validation of echocardiography-based machine-learning models to predict mortality.
In EBioMedicine
BACKGROUND :
METHODS :
FINDINGS :
INTERPRETATION :
FUNDING :
Valsaraj Akshay, Kalmady Sunil Vasu, Sharma Vaibhav, Frost Matthew, Sun Weijie, Sepehrvand Nariman, Ong Marcus, Equibec Cyril, Dyck Jason R B, Anderson Todd, Becher Harald, Weeks Sarah, Tromp Jasper, Hung Chung-Lieh, Ezekowitz Justin A, Kaul Padma
2023-Feb-27
Deep learning, Echocardiography, Functional status, Heart failure, Machine learning, Mortality, Prognostic models
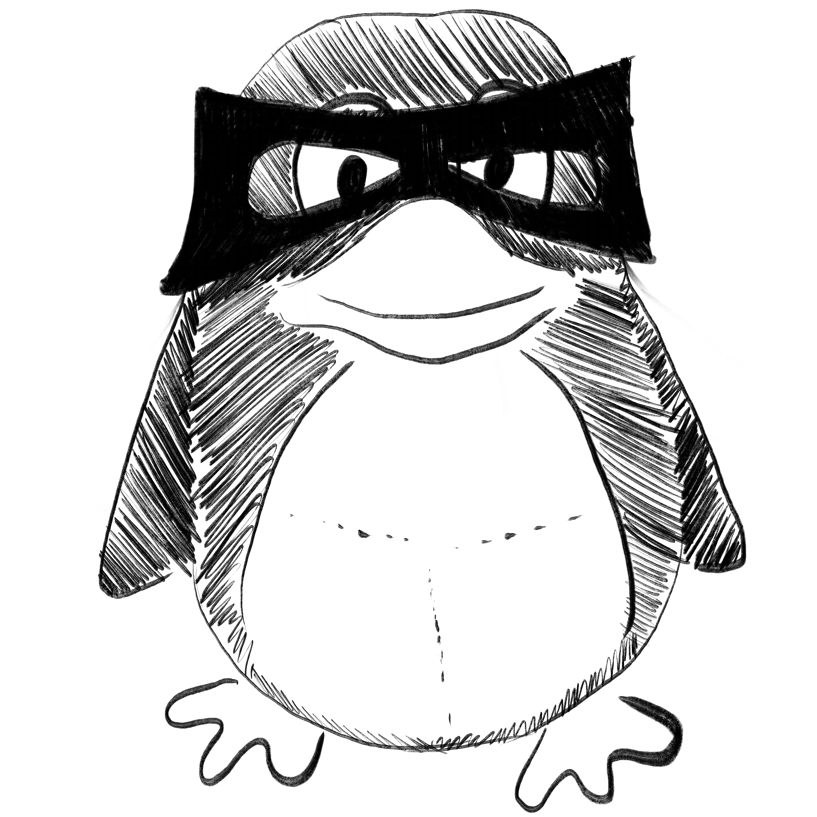
Identifying subtypes of chronic kidney disease with machine learning: development, internal validation and prognostic validation using linked electronic health records in 350,067 individuals.
In EBioMedicine
BACKGROUND :
METHODS :
FINDINGS :
MEDICATIONS :
INTERPRETATION :
FUNDING :
Dashtban Ashkan, Mizani Mehrdad A, Pasea Laura, Denaxas Spiros, Corbett Richard, Mamza Jil B, Gao He, Morris Tamsin, Hemingway Harry, Banerjee Amitava
2023-Feb-27
CKD subtype, Cluster analysis, Machine learning, Survival analysis, Unsupervised clustering
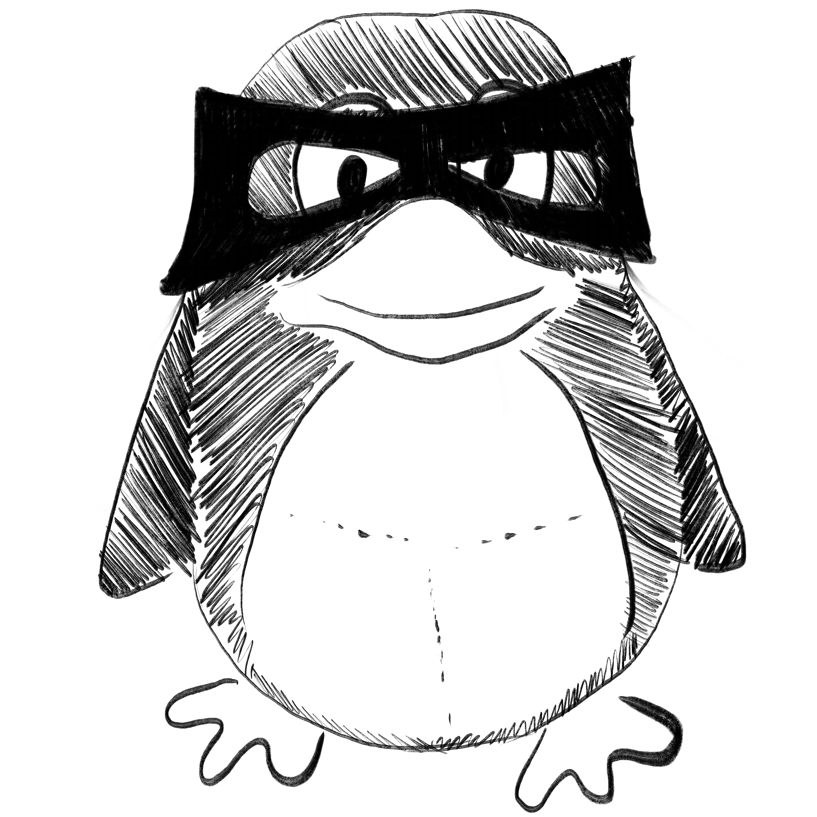
Inferring protein fitness landscapes from laboratory evolution experiments.
In PLoS computational biology
D’Costa Sameer, Hinds Emily C, Freschlin Chase R, Song Hyebin, Romero Philip A
2023-Mar-01
Benchmarking omics-based prediction of asthma development in children.
In Respiratory research ; h5-index 45.0
BACKGROUND :
OBJECTIVE :
METHOD :
RESULTS :
CONCLUSIONS :
Wang Xu-Wen, Wang Tong, Schaub Darius P, Chen Can, Sun Zheng, Ke Shanlin, Hecker Julian, Maaser-Hecker Anna, Zeleznik Oana A, Zeleznik Roman, Litonjua Augusto A, DeMeo Dawn L, Lasky-Su Jessica, Silverman Edwin K, Liu Yang-Yu, Weiss Scott T
2023-Feb-26
Asthma, Disease status, Multi-omics, Prediction
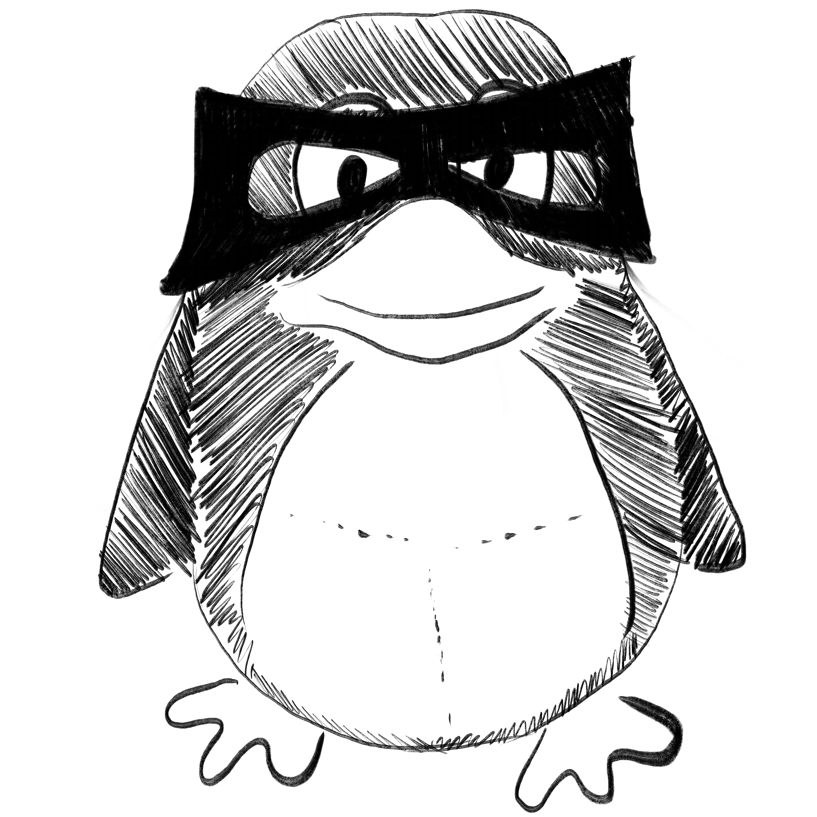
A framework to identify ethical concerns with ML-guided care workflows: a case study of mortality prediction to guide advance care planning.
In Journal of the American Medical Informatics Association : JAMIA
OBJECTIVE :
MATERIALS AND METHODS :
RESULTS :
DISCUSSION :
CONCLUSION :
Cagliero Diana, Deuitch Natalie, Shah Nigam, Feudtner Chris, Char Danton
2023-Feb-24
artificial intelligence, clinical, end-of-life care, ethics, machine learning, palliative care
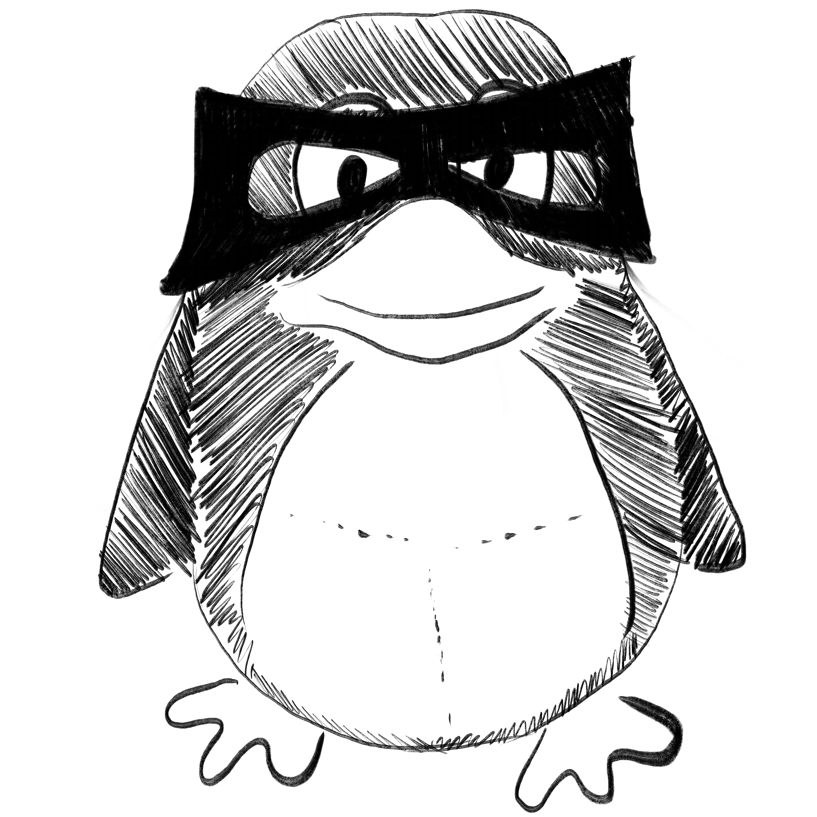
Field-dependent deep learning enables high-throughput whole-cell 3D super-resolution imaging.
In Nature methods ; h5-index 152.0
Fu Shuang, Shi Wei, Luo Tingdan, He Yingchuan, Zhou Lulu, Yang Jie, Yang Zhichao, Liu Jiadong, Liu Xiaotian, Guo Zhiyong, Yang Chengyu, Liu Chao, Huang Zhen-Li, Ries Jonas, Zhang Mingjie, Xi Peng, Jin Dayong, Li Yiming
2023-Feb-23
Targeted plasma proteomics reveals signatures discriminating COVID-19 from sepsis with pneumonia.
In Respiratory research ; h5-index 45.0
BACKGROUND :
METHODS :
RESULTS :
CONCLUSIONS :
Palma Medina Laura M, Babačić Haris, Dzidic Majda, Parke Åsa, Garcia Marina, Maleki Kimia T, Unge Christian, Lourda Magda, Kvedaraite Egle, Chen Puran, Muvva Jagadeeswara Rao, Cornillet Martin, Emgård Johanna, Moll Kirsten, Michaëlsson Jakob, Flodström-Tullberg Malin, Brighenti Susanna, Buggert Marcus, Mjösberg Jenny, Malmberg Karl-Johan, Sandberg Johan K, Gredmark-Russ Sara, Rooyackers Olav, Svensson Mattias, Chambers Benedict J, Eriksson Lars I, Pernemalm Maria, Björkström Niklas K, Aleman Soo, Ljunggren Hans-Gustaf, Klingström Jonas, Strålin Kristoffer, Norrby-Teglund Anna
2023-Feb-24
COVID-19, Community acquired pneumonia, Olink proximity extension assays, Sepsis, Septic shock
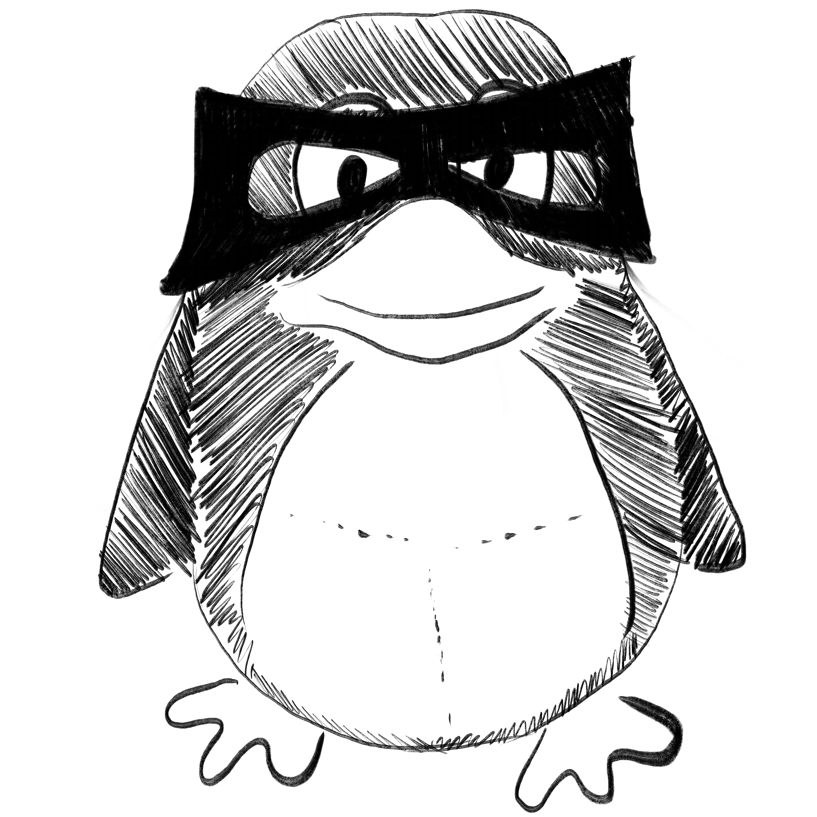
Social complexity, life-history and lineage influence the molecular basis of castes in vespid wasps.
In Nature communications ; h5-index 260.0
Wyatt Christopher Douglas Robert, Bentley Michael Andrew, Taylor Daisy, Favreau Emeline, Brock Ryan Edward, Taylor Benjamin Aaron, Bell Emily, Leadbeater Ellouise, Sumner Seirian
2023-Feb-24
Targeted plasma proteomics reveals signatures discriminating COVID-19 from sepsis with pneumonia.
In Respiratory research ; h5-index 45.0
BACKGROUND :
METHODS :
RESULTS :
CONCLUSIONS :
Palma Medina Laura M, Babačić Haris, Dzidic Majda, Parke Åsa, Garcia Marina, Maleki Kimia T, Unge Christian, Lourda Magda, Kvedaraite Egle, Chen Puran, Muvva Jagadeeswara Rao, Cornillet Martin, Emgård Johanna, Moll Kirsten, Michaëlsson Jakob, Flodström-Tullberg Malin, Brighenti Susanna, Buggert Marcus, Mjösberg Jenny, Malmberg Karl-Johan, Sandberg Johan K, Gredmark-Russ Sara, Rooyackers Olav, Svensson Mattias, Chambers Benedict J, Eriksson Lars I, Pernemalm Maria, Björkström Niklas K, Aleman Soo, Ljunggren Hans-Gustaf, Klingström Jonas, Strålin Kristoffer, Norrby-Teglund Anna
2023-Feb-24
COVID-19, Community acquired pneumonia, Olink proximity extension assays, Sepsis, Septic shock
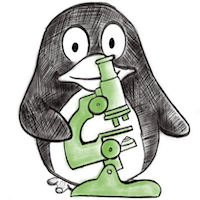
Unstained Tissue Imaging and Virtual Hematoxylin and Eosin Staining of Histologic Whole Slide Images.
In Laboratory investigation; a journal of technical methods and pathology ; h5-index 42.0
Koivukoski Sonja, Khan Umair, Ruusuvuori Pekka, Latonen Leena
2023-Jan-25
HE staining, computational histology, digital pathology, histology, virtual staining, whole slide imaging (WSI)
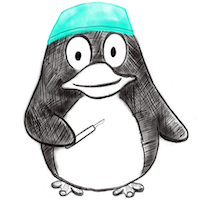
NIR-II fluorescence imaging-guided colorectal cancer surgery targeting CEACAM5 by a nanobody.
In EBioMedicine
BACKGROUND :
METHODS :
FINDINGS :
INTERPRETATION :
FUNDINGS :
Guo Xiaoyong, Li Changjian, Jia Xiaohua, Qu Yawei, Li Miaomiao, Cao Caiguang, Zhang Zeyu, Qu Qiaojun, Luo Shuangling, Tang Jianqiang, Liu Haifeng, Hu Zhenhua, Tian Jie
2023-Feb-16
CEACAM5, Colorectal cancer, Molecular imaging, Nanobody, Second near-infrared window
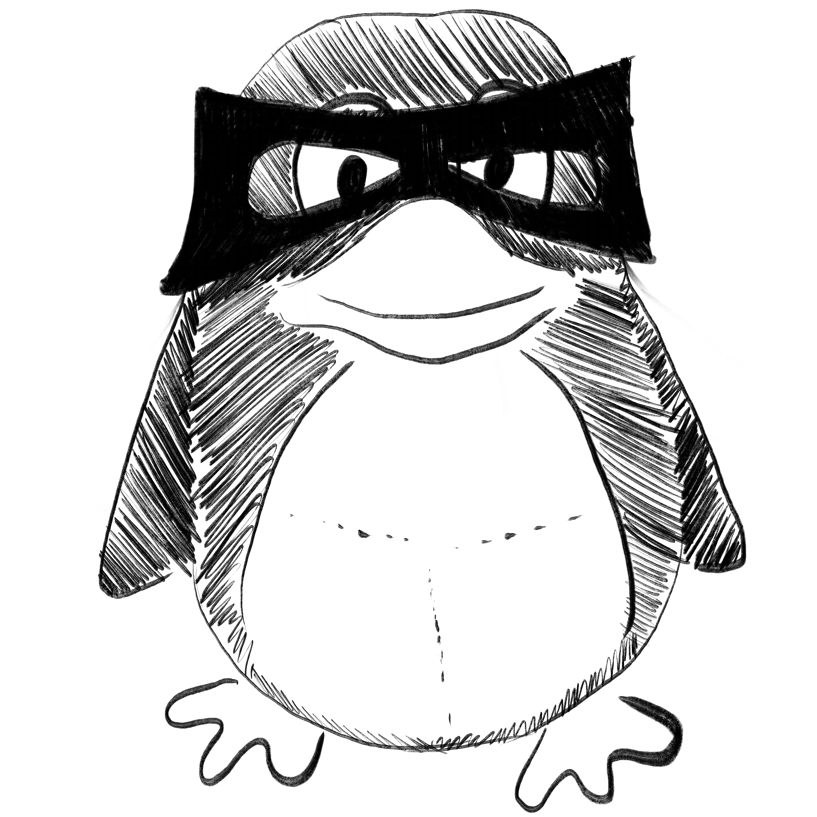
Network of hotspot interactions cluster tau amyloid folds.
In Nature communications ; h5-index 260.0
Mullapudi Vishruth, Vaquer-Alicea Jaime, Bommareddy Vaibhav, Vega Anthony R, Ryder Bryan D, White Charles L, Diamond Marc I, Joachimiak Lukasz A
2023-Feb-16
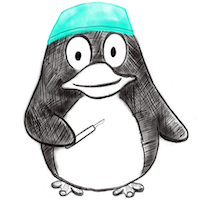
How the UK public views the use of diagnostic decision aids by physicians: a vignette-based experiment.
In Journal of the American Medical Informatics Association : JAMIA
OBJECTIVE :
MATERIALS AND METHODS :
RESULTS :
DISCUSSION :
CONCLUSION :
Nurek Martine, Kostopoulou Olga
2023-Feb-16
artificial intelligence, decision aids, diagnosis, health regulatory focus, individual differences, trust in physicians, worry
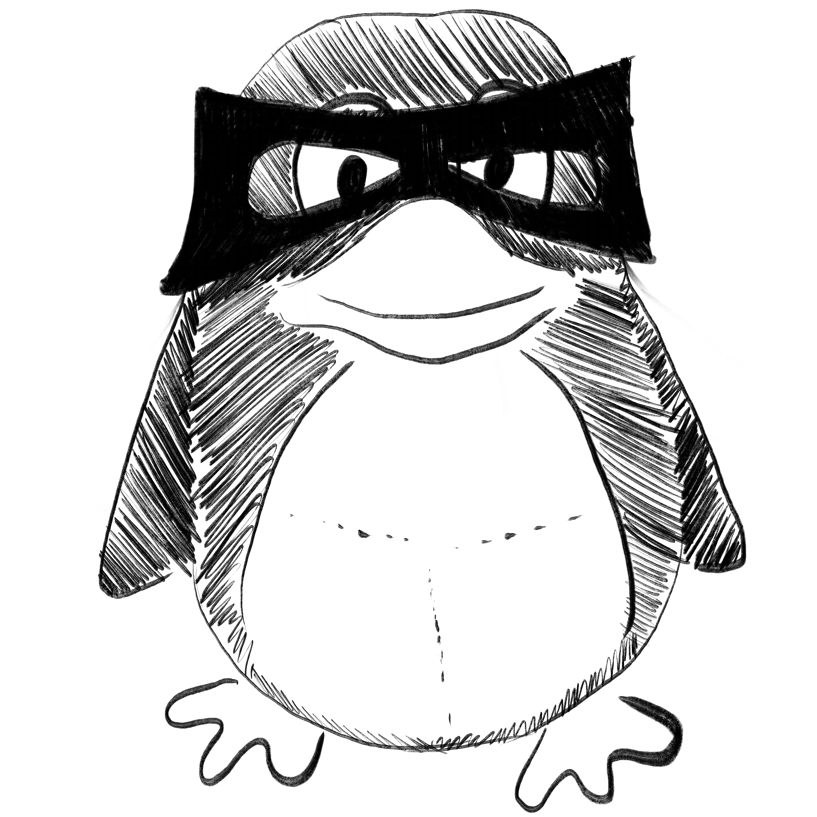
The 2022 n2c2/UW shared task on extracting social determinants of health.
In Journal of the American Medical Informatics Association : JAMIA
OBJECTIVE :
MATERIALS AND METHODS :
RESULTS :
CONCLUSIONS :
Lybarger Kevin, Yetisgen Meliha, Uzuner Özlem
2023-Feb-16
data mining, electronic health records, machine learning, natural language processing, social determinants of health
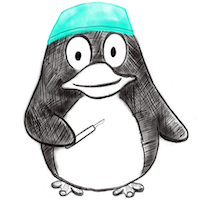
A hierarchical multilabel graph attention network method to predict the deterioration paths of chronic hepatitis B patients.
In Journal of the American Medical Informatics Association : JAMIA
OBJECTIVE :
MATERIALS AND METHODS :
RESULTS :
DISCUSSION AND CONCLUSION :
Wu Zejian Eric, Xu Da, Hu Paul Jen-Hwa, Huang Ting-Shuo
2023-Feb-15
chronic hepatitis B patients, deep learning, deterioration path predictions, graph attention network, predictive analytics
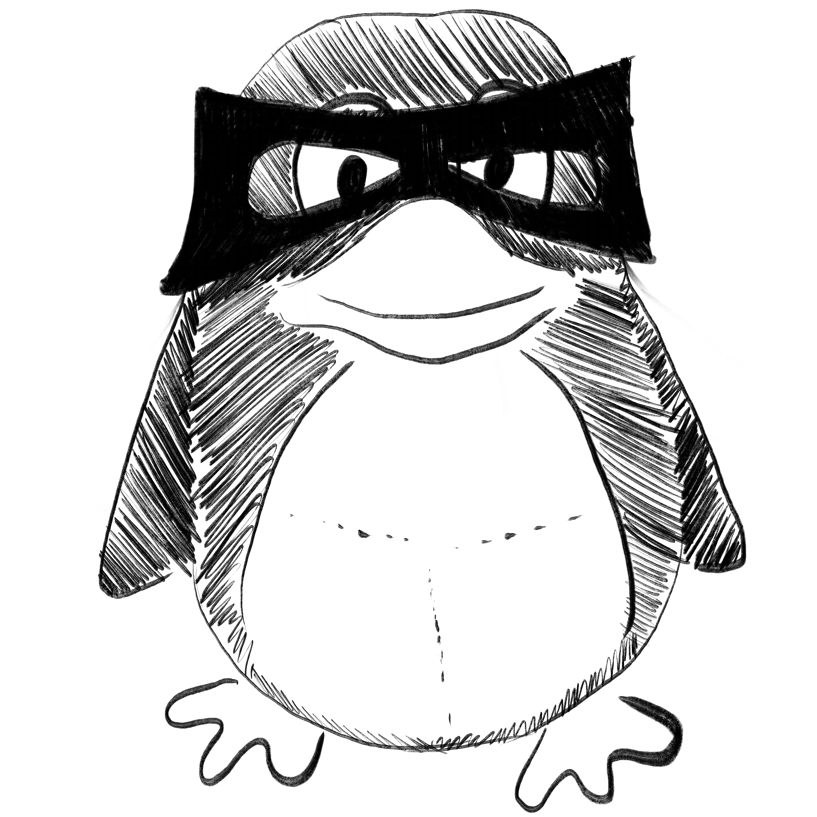
Algorithmic encoding of protected characteristics in chest X-ray disease detection models.
In EBioMedicine
BACKGROUND :
METHODS :
FINDINGS :
INTERPRETATION :
FUNDING :
Glocker Ben, Jones Charles, Bernhardt Mélanie, Winzeck Stefan
2023-Feb-13
Algorithmic bias, Artificial intelligence, Image-based disease detection, Subgroup disparities
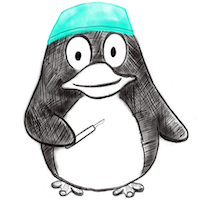
Data-driven longitudinal characterization of neonatal health and morbidity.
In Science translational medicine ; h5-index 138.0
De Francesco Davide, Reiss Jonathan D, Roger Jacquelyn, Tang Alice S, Chang Alan L, Becker Martin, Phongpreecha Thanaphong, Espinosa Camilo, Morin Susanna, Berson Eloïse, Thuraiappah Melan, Le Brian L, Ravindra Neal G, Payrovnaziri Seyedeh Neelufar, Mataraso Samson, Kim Yeasul, Xue Lei, Rosenstein Melissa G, Oskotsky Tomiko, Marić Ivana, Gaudilliere Brice, Carvalho Brendan, Bateman Brian T, Angst Martin S, Prince Lawrence S, Blumenfeld Yair J, Benitz William E, Fuerch Janene H, Shaw Gary M, Sylvester Karl G, Stevenson David K, Sirota Marina, Aghaeepour Nima
2023-Feb-15
The gut microbiome and early-life growth in a population with high prevalence of stunting.
In Nature communications ; h5-index 260.0
Robertson Ruairi C, Edens Thaddeus J, Carr Lynnea, Mutasa Kuda, Gough Ethan K, Evans Ceri, Geum Hyun Min, Baharmand Iman, Gill Sandeep K, Ntozini Robert, Smith Laura E, Chasekwa Bernard, Majo Florence D, Tavengwa Naume V, Mutasa Batsirai, Francis Freddy, Tome Joice, Stoltzfus Rebecca J, Humphrey Jean H, Prendergast Andrew J, Manges Amee R
2023-Feb-14
Deep Learning-based Approach to Predict Pulmonary Function at Chest CT.
In Radiology ; h5-index 91.0
Park Hyunjung, Yun Jihye, Lee Sang Min, Hwang Hye Jeon, Seo Joon Beom, Jung Young Ju, Hwang Jeongeun, Lee Se Hee, Lee Sei Won, Kim Namkug
2023-Feb-14
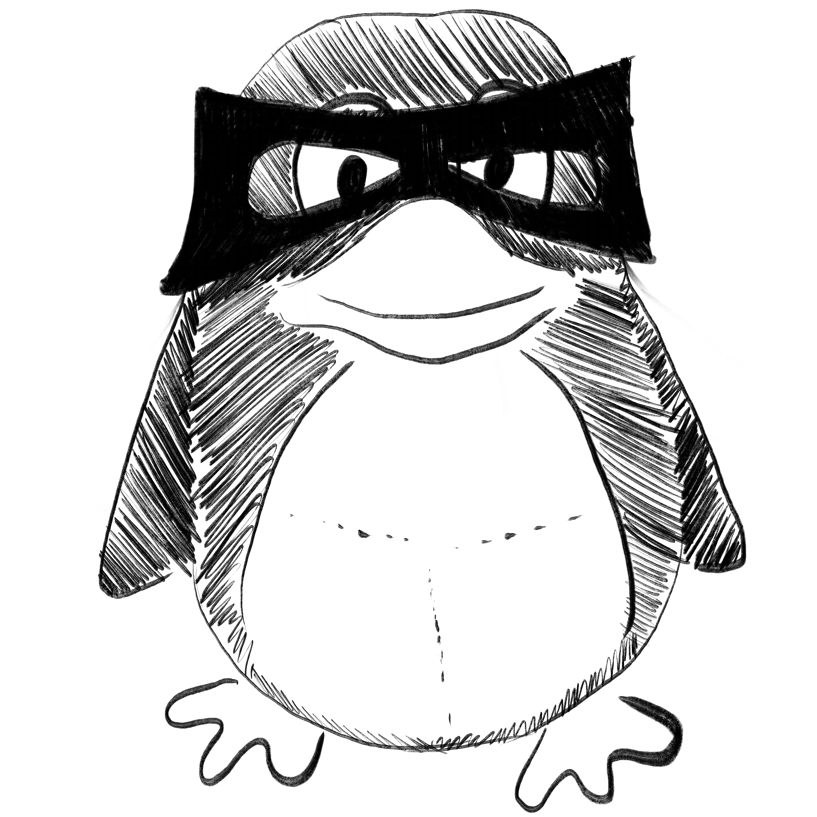
AI reveals insights into link between CD33 and cognitive impairment in Alzheimer's Disease.
In PLoS computational biology
Raschka Tamara, Sood Meemansa, Schultz Bruce, Altay Aybuge, Ebeling Christian, Fröhlich Holger
2023-Feb-13
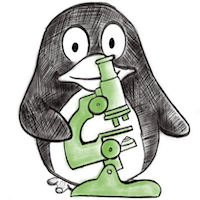
Predicting 1p/19q co-deletion status from magnetic resonance imaging using deep learning in adult-type diffuse lower-grade gliomas: a discovery and validation study.
In Laboratory investigation; a journal of technical methods and pathology ; h5-index 42.0
Yan Jing, Zhang Shenghai, Sun Qiuchang, Wang Weiwei, Duan Wenchao, Wang Li, Ding Tianqing, Pei Dongling, Sun Chen, Wang Wenqing, Liu Zhen, Hong Xuanke, Wang Xiangxiang, Guo Yu, Li Wencai, Cheng Jingliang, Liu Xianzhi, Li Zhi-Cheng, Zhang Zhenyu
2022-Feb
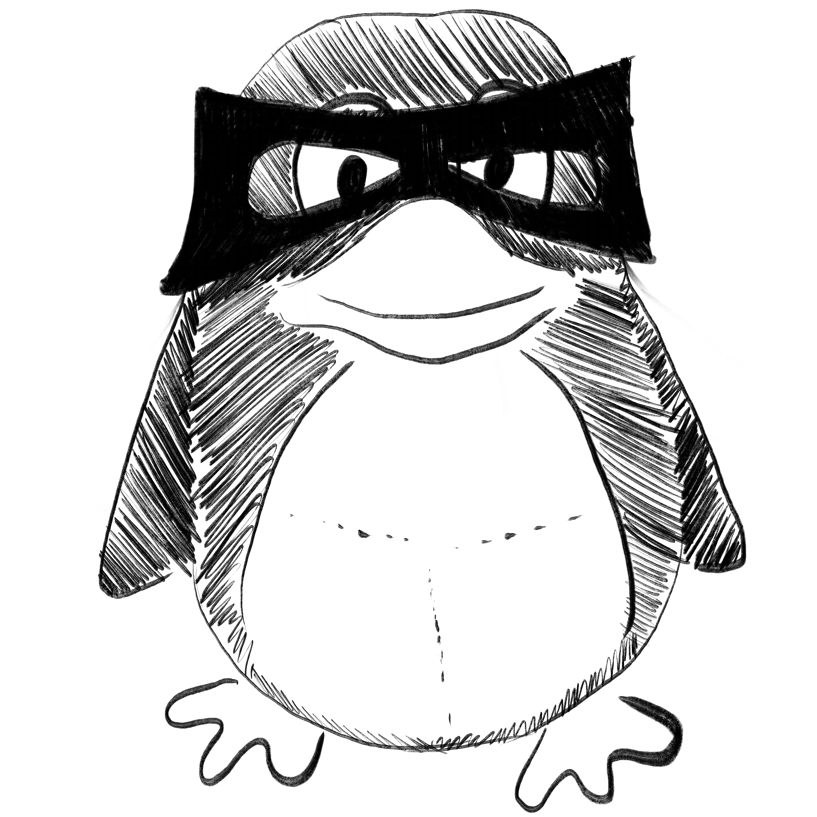
A convolutional neural network model for survival prediction based on prognosis-related cascaded Wx feature selection.
In Laboratory investigation; a journal of technical methods and pathology ; h5-index 42.0
Yin Qingyan, Chen Wangwang, Zhang Chunxia, Wei Zhi
2022-Oct
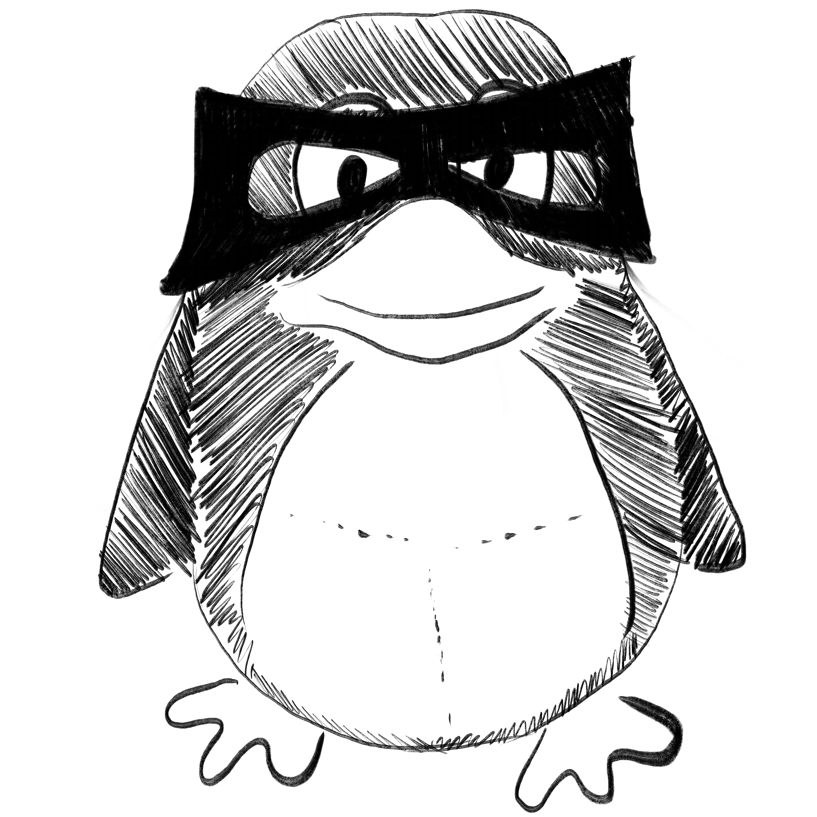
Modeling CRISPR-Cas13d on-target and off-target effects using machine learning approaches.
In Nature communications ; h5-index 260.0
Cheng Xiaolong, Li Zexu, Shan Ruocheng, Li Zihan, Wang Shengnan, Zhao Wenchang, Zhang Han, Chao Lumen, Peng Jian, Fei Teng, Li Wei
2023-Feb-10
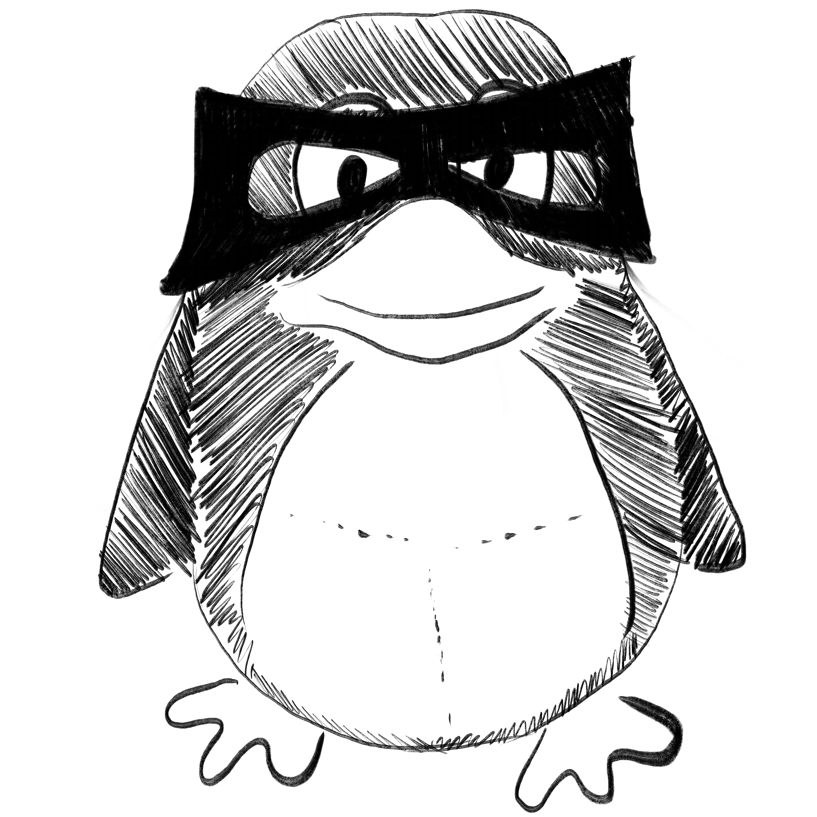
Multi-omics and machine learning reveal context-specific gene regulatory activities of PML::RARA in acute promyelocytic leukemia.
In Nature communications ; h5-index 260.0
Villiers William, Kelly Audrey, He Xiaohan, Kaufman-Cook James, Elbasir Abdurrahman, Bensmail Halima, Lavender Paul, Dillon Richard, Mifsud Borbála, Osborne Cameron S
2023-Feb-09
Altered global signal topography in Alzheimer's disease.
In EBioMedicine
BACKGROUND :
METHODS :
FINDINGS :
INTERPRETATION :
FUNDING :
Chen Pindong, Zhao Kun, Zhang Han, Wei Yongbin, Wang Pan, Wang Dawei, Song Chengyuan, Yang Hongwei, Zhang Zengqiang, Yao Hongxiang, Qu Yida, Kang Xiaopeng, Du Kai, Fan Lingzhong, Han Tong, Yu Chunshui, Zhou Bo, Jiang Tianzi, Zhou Yuying, Lu Jie, Han Ying, Zhang Xi, Liu Bing, Liu Yong
2023-Feb-07
“Alzheimers disease”, Functional network, Global signal, Transcriptomics
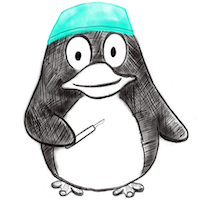
UCseek: ultrasensitive early detection and recurrence monitoring of urothelial carcinoma by shallow-depth genome-wide bisulfite sequencing of urinary sediment DNA.
In EBioMedicine
BACKGROUND :
METHODS :
FINDINGS :
INTERPRETATION :
FUNDING :
Wang Ping, Shi Yue, Zhang Jianye, Shou Jianzhong, Zhang Mingxin, Zou Daojia, Liang Yuan, Li Juan, Tan Yezhen, Zhang Mei, Bi Xingang, Zhou Liqun, Ci Weimin, Li Xuesong
2023-Feb-07
Diagnosis, Machine learning, Molecular diagnostics, Relapse monitoring, Tumor markers, Urothelial carcinoma
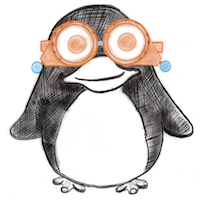
Neurologic Dysfunction Assessment in Parkinson Disease Based on Fundus Photographs Using Deep Learning.
In JAMA ophthalmology ; h5-index 58.0
IMPORTANCE :
OBJECTIVE :
DESIGN, SETTINGS, AND PARTICIPANTS :
MAIN OUTCOMES AND MEASURES :
RESULTS :
CONCLUSIONS AND RELEVANCE :
Ahn Sangil, Shin Jitae, Song Su Jeong, Yoon Won Tae, Sagong Min, Jeong Areum, Kim Joon Hyung, Yu Hyeong Gon
2023-Feb-09
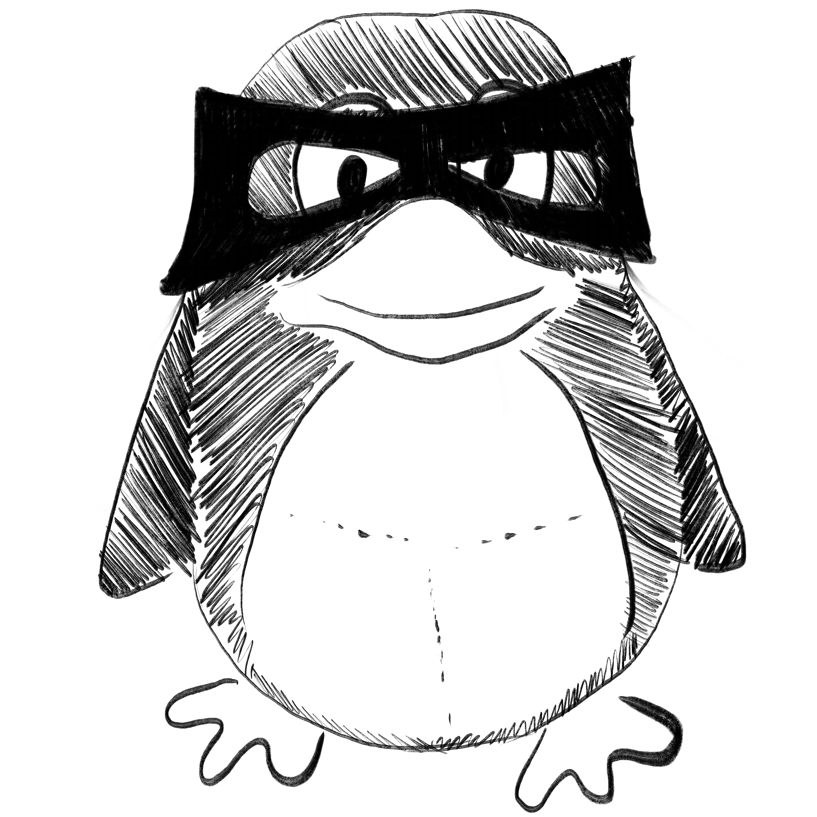
Association of Recent SARS-CoV-2 Infection With New-Onset Alcohol Use Disorder, January 2020 Through January 2022.
In JAMA network open
IMPORTANCE :
OBJECTIVE :
DESIGN, SETTING, AND PARTICIPANTS :
EXPOSURES :
MAIN OUTCOMES AND MEASURES :
RESULTS :
CONCLUSIONS AND RELEVANCE :
Olaker Veronica R, Kendall Ellen K, Wang Christina X, Parran Theodore V, Terebuh Pauline, Kaelber David C, Xu Rong, Davis Pamela B
2023-Feb-01
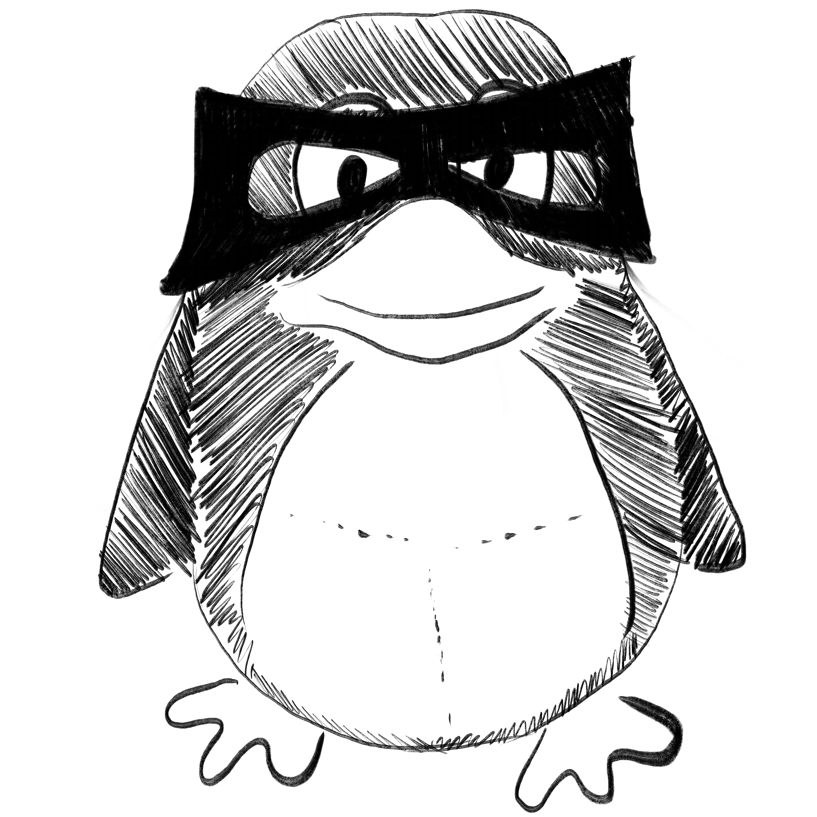
Association of Recent SARS-CoV-2 Infection With New-Onset Alcohol Use Disorder, January 2020 Through January 2022.
In JAMA network open
IMPORTANCE :
OBJECTIVE :
DESIGN, SETTING, AND PARTICIPANTS :
EXPOSURES :
MAIN OUTCOMES AND MEASURES :
RESULTS :
CONCLUSIONS AND RELEVANCE :
Olaker Veronica R, Kendall Ellen K, Wang Christina X, Parran Theodore V, Terebuh Pauline, Kaelber David C, Xu Rong, Davis Pamela B
2023-Feb-01
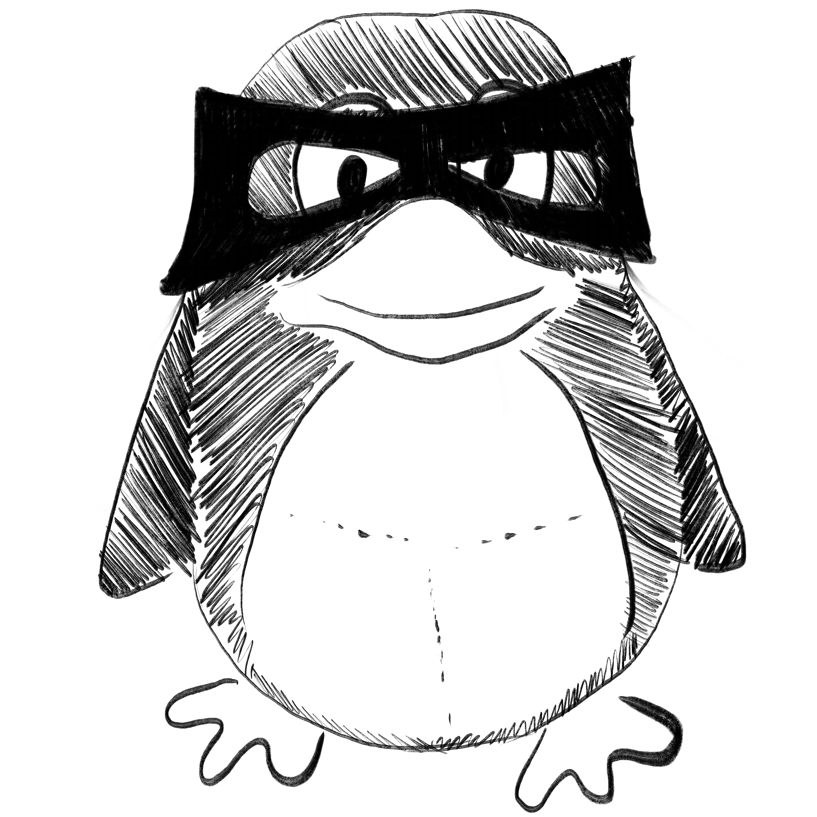
Dissecting cell identity via network inference and in silico gene perturbation.
In Nature ; h5-index 368.0
Kamimoto Kenji, Stringa Blerta, Hoffmann Christy M, Jindal Kunal, Solnica-Krezel Lilianna, Morris Samantha A
2023-Feb-08
Cartography of Genomic Interactions Enables Deep Analysis of Single-Cell Expression Data.
In Nature communications ; h5-index 260.0
Islam Md Tauhidul, Xing Lei
2023-Feb-08
Comparison of Chest Radiograph Captions Based on Natural Language Processing vs Completed by Radiologists.
In JAMA network open
IMPORTANCE :
OBJECTIVE :
DESIGN, SETTING, AND PARTICIPANTS :
EXPOSURES :
MAIN OUTCOMES AND MEASURES :
RESULTS :
CONCLUSIONS AND RELEVANCE :
Zhang Yaping, Liu Mingqian, Zhang Lu, Wang Lingyun, Zhao Keke, Hu Shundong, Chen Xu, Xie Xueqian
2023-Feb-01
AI Improves Nodule Detection on Chest Radiographs in a Health Screening Population: A Randomized Controlled Trial.
In Radiology ; h5-index 91.0
Nam Ju Gang, Hwang Eui Jin, Kim Jayoun, Park Nanhee, Lee Eun Hee, Kim Hyun Jin, Nam Miyeon, Lee Jong Hyuk, Park Chang Min, Goo Jin Mo
2023-Feb-07
Beyond Breast Density: Risk Measures for Breast Cancer in Multiple Imaging Modalities.
In Radiology ; h5-index 91.0
Acciavatti Raymond J, Lee Su Hyun, Reig Beatriu, Moy Linda, Conant Emily F, Kontos Despina, Moon Woo Kyung
2023-Feb-07
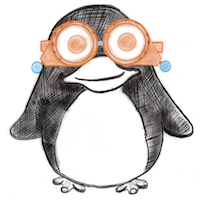
Multicenter Evaluation of AI-generated DIR and PSIR for Cortical and Juxtacortical Multiple Sclerosis Lesion Detection.
In Radiology ; h5-index 91.0
Bouman Piet M, Noteboom Samantha, Nobrega Santos Fernando A, Beck Erin S, Bliault Gregory, Castellaro Marco, Calabrese Massimiliano, Chard Declan T, Eichinger Paul, Filippi Massimo, Inglese Matilde, Lapucci Caterina, Marciniak Andrzej, Moraal Bastiaan, Morales Pinzon Alfredo, Mühlau Mark, Preziosa Paolo, Reich Daniel S, Rocca Maria A, Schoonheim Menno M, Twisk Jos W R, Wiestler Benedict, Jonkman Laura E, Guttmann Charles R G, Geurts Jeroen J G, Steenwijk Martijn D
2023-Feb-07
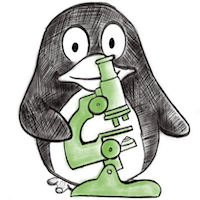
Deepfake Histologic Images for Enhancing Digital Pathology.
In Laboratory investigation; a journal of technical methods and pathology ; h5-index 42.0
Falahkheirkhah Kianoush, Tiwari Saumya, Yeh Kevin, Gupta Sounak, Herrera-Hernandez Loren, McCarthy Michael R, Jimenez Rafael E, Cheville John C, Bhargava Rohit
2023-Jan
computer vision, deep learning, deepfake pathology, digital pathology synthetic data
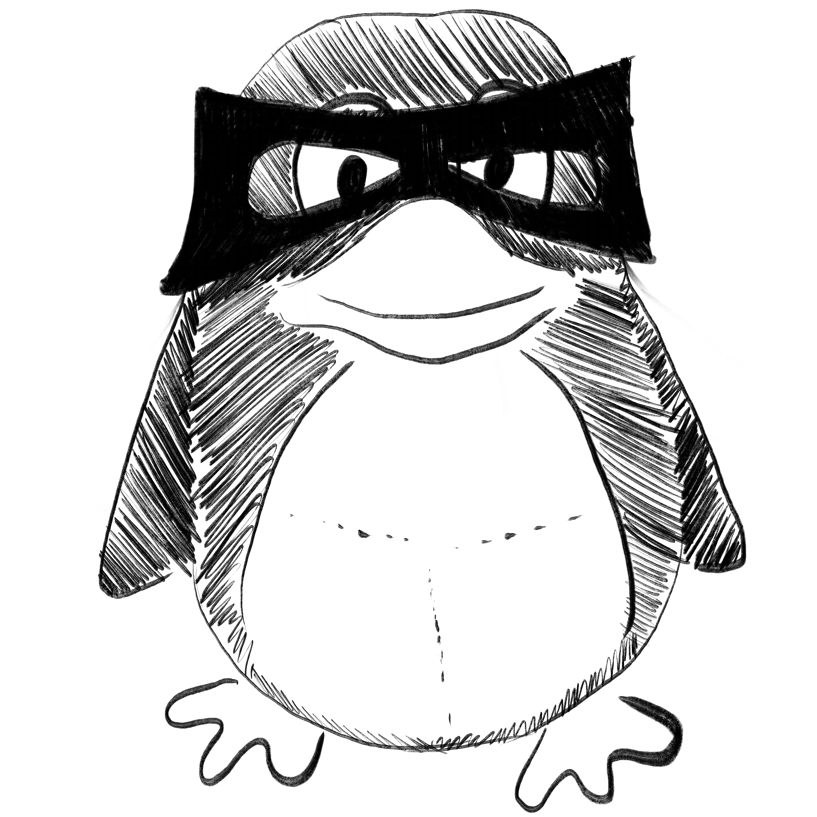
Funneling modulatory peptide design with generative models: Discovery and characterization of disruptors of calcineurin protein-protein interactions.
In PLoS computational biology
Tubiana Jérôme, Adriana-Lifshits Lucia, Nissan Michael, Gabay Matan, Sher Inbal, Sova Marina, Wolfson Haim J, Gal Maayan
2023-Feb-02
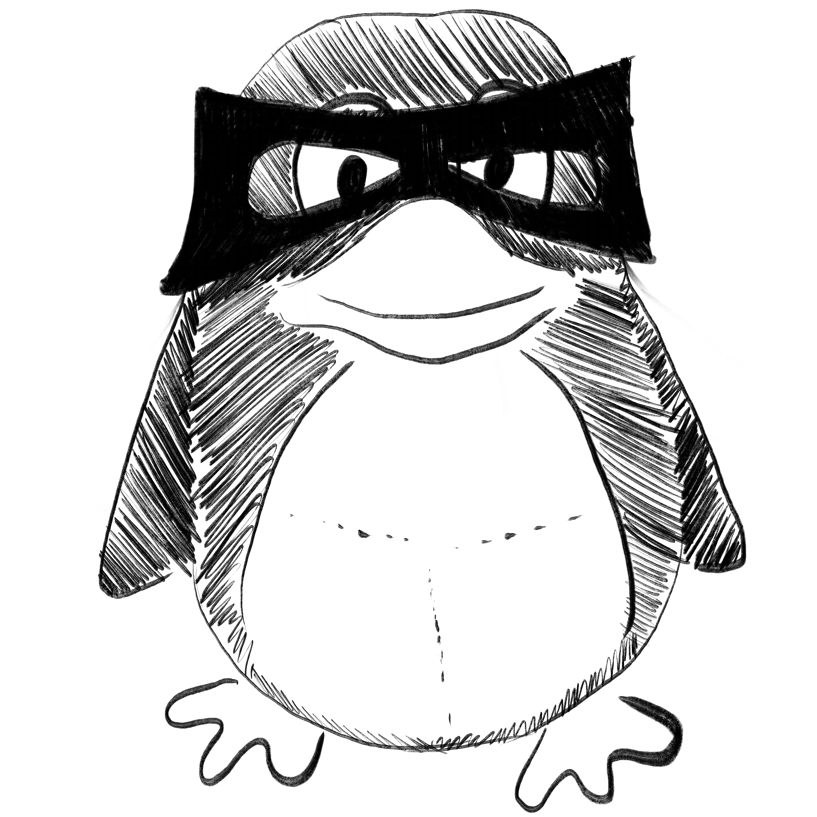
Assessment of Pediatric Telemedicine Using Remote Physical Examinations With a Mobile Medical Device: A Nonrandomized Controlled Trial.
In JAMA network open
IMPORTANCE :
OBJECTIVE :
DESIGN, SETTING, AND PARTICIPANTS :
MAIN OUTCOMES AND MEASURES :
RESULTS :
CONCLUSIONS AND RELEVANCE :
TRIAL REGISTRATION :
Wagner Rafaela, Lima Thalita Cecília, Silva Marielen Ribeiro Tavares da, Rabha Anna Clara Pereira, Ricieri Marinei Campos, Fachi Mariana Millan, Afonso Rogério Carballo, Motta Fábio Araújo
2023-Feb-01
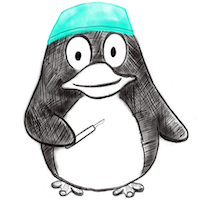
Single-cell spatial landscapes of the lung tumour immune microenvironment.
In Nature ; h5-index 368.0
Sorin Mark, Rezanejad Morteza, Karimi Elham, Fiset Benoit, Desharnais Lysanne, Perus Lucas J M, Milette Simon, Yu Miranda W, Maritan Sarah M, Doré Samuel, Pichette Émilie, Enlow William, Gagné Andréanne, Wei Yuhong, Orain Michele, Manem Venkata S K, Rayes Roni, Siegel Peter M, Camilleri-Broët Sophie, Fiset Pierre Olivier, Desmeules Patrice, Spicer Jonathan D, Quail Daniela F, Joubert Philippe, Walsh Logan A
2023-Feb-01
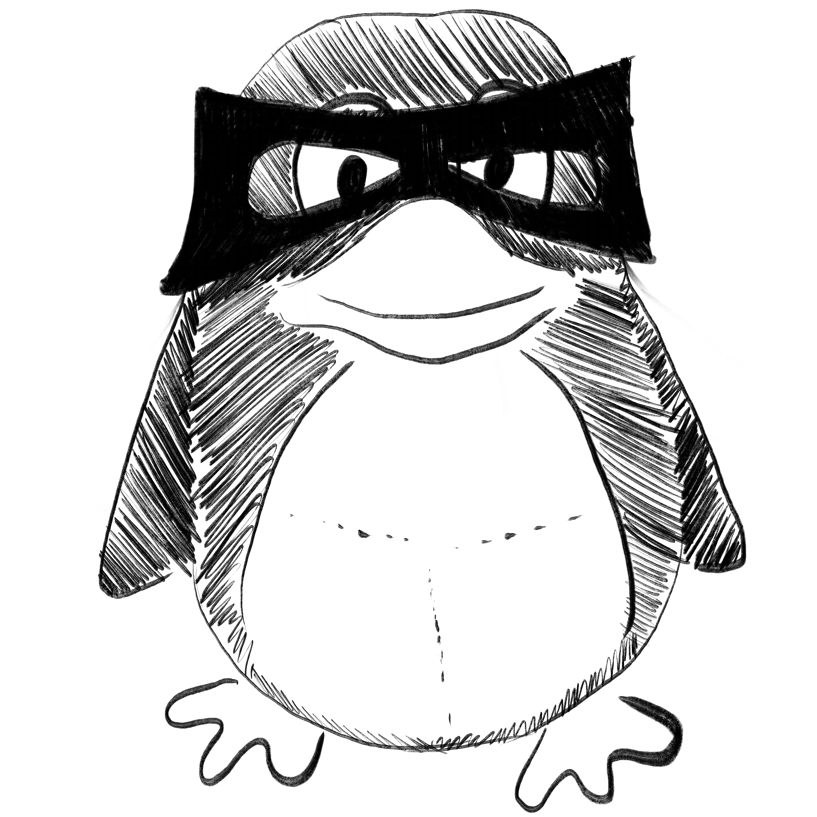
Automated extraction of clinical measures from videos of oculofacial disorders using machine learning: feasibility, validity and reliability.
In Eye (London, England) ; h5-index 41.0
OBJECTIVES :
METHODS :
RESULTS :
CONCLUSIONS :
Schulz Christopher B, Clarke Holly, Makuloluwe Sarith, Thomas Peter B, Kang Swan
2023-Feb-01
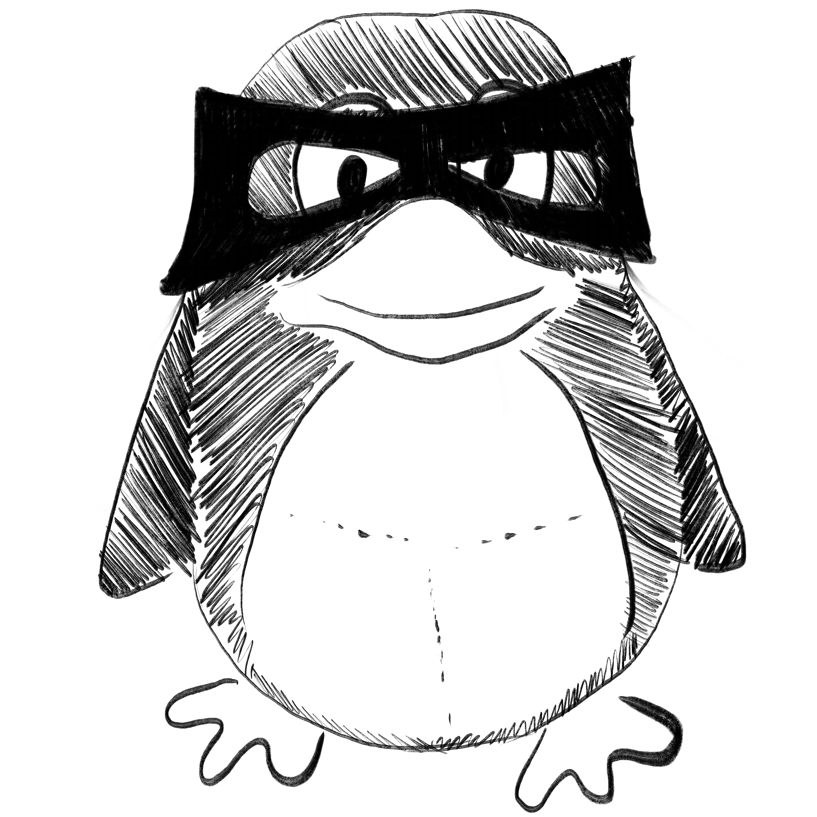
Highly-scaled and fully-integrated 3-dimensional ferroelectric transistor array for hardware implementation of neural networks.
In Nature communications ; h5-index 260.0
Kim Ik-Jyae, Kim Min-Kyu, Lee Jang-Sik
2023-Jan-31
Development and Validation of a Deep Learning-Based Synthetic Bone-Suppressed Model for Pulmonary Nodule Detection in Chest Radiographs.
In JAMA network open
IMPORTANCE :
OBJECTIVES :
DESIGN, SETTING, AND PARTICIPANTS :
EXPOSURES :
MAIN OUTCOMES AND MEASURES :
RESULTS :
CONCLUSIONS AND RELEVANCE :
Kim Hwiyoung, Lee Kye Ho, Han Kyunghwa, Lee Ji Won, Kim Jin Young, Im Dong Jin, Hong Yoo Jin, Choi Byoung Wook, Hur Jin
2023-Jan-03
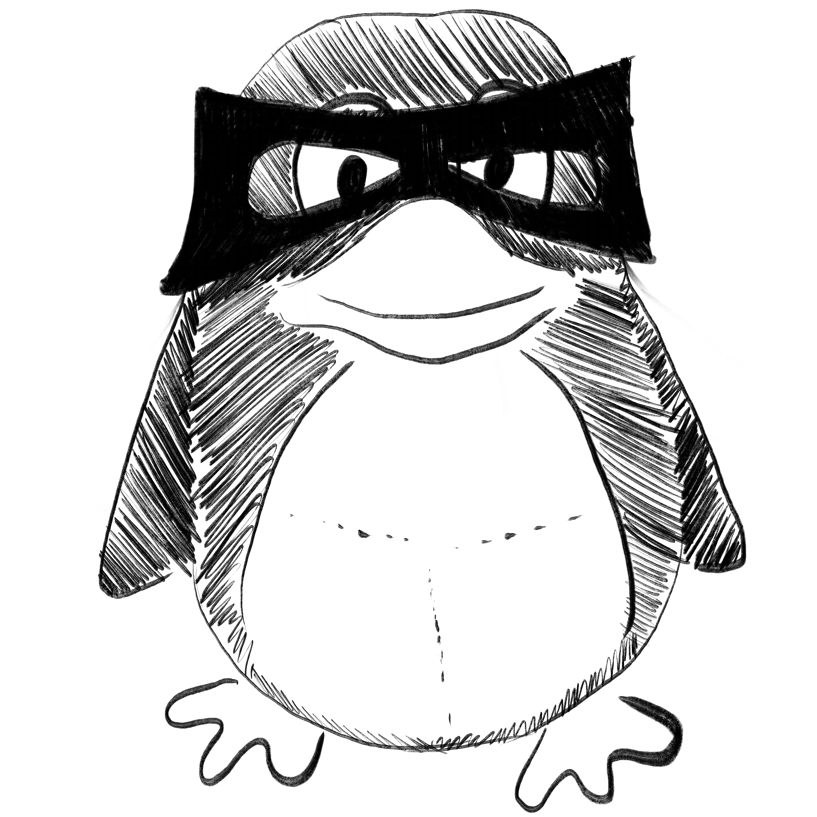
Assessment of the Role of Artificial Intelligence in the Association Between Time of Day and Colonoscopy Quality.
In JAMA network open
IMPORTANCE :
OBJECTIVE :
DESIGN, SETTING, AND PARTICIPANTS :
EXPOSURE :
MAIN OUTCOMES AND MEASURES :
RESULTS :
CONCLUSIONS AND RELEVANCE :
Lu Zihua, Zhang Lihui, Yao Liwen, Gong Dexin, Wu Lianlian, Xia Meiqing, Zhang Jun, Zhou Wei, Huang Xu, He Chunping, Wu Huiling, Zhang Chenxia, Li Xun, Yu Honggang
2023-Jan-03
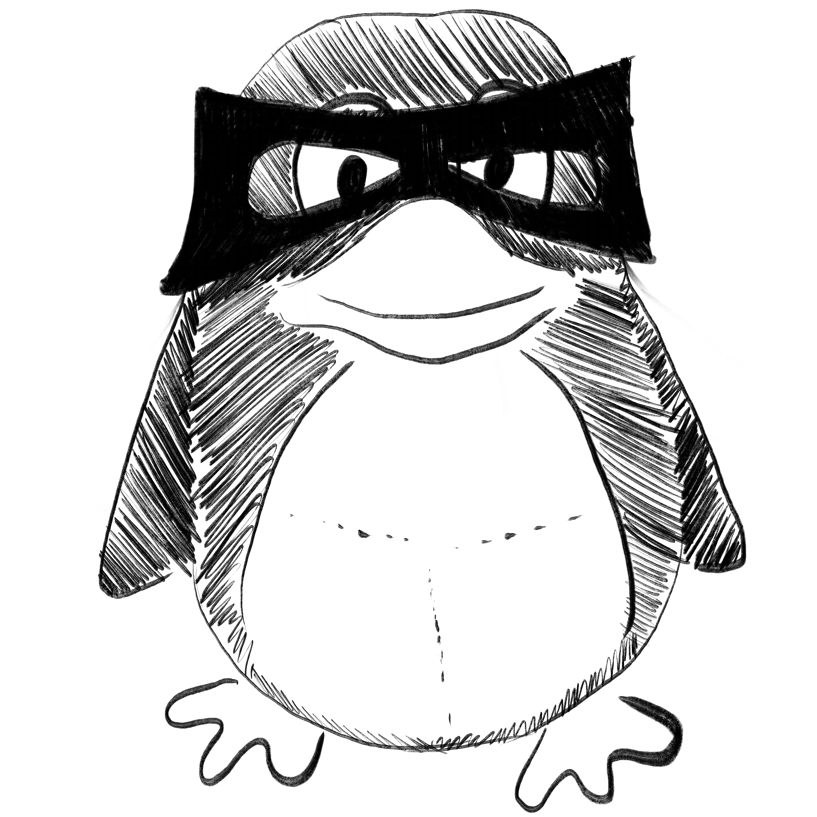
Machine Learning-Based Prediction of Hospital Admission Among Children in an Emergency Care Center.
In Pediatric emergency care
OBJECTIVES :
METHODS :
RESULTS :
CONCLUSIONS :
Hatachi Takeshi, Hashizume Takao, Taniguchi Masashi, Inata Yu, Aoki Yoshihiro, Kawamura Atsushi, Takeuchi Muneyuki
2023-Feb-01
Deep Learning Image Reconstruction for CT: Technical Principles and Clinical Prospects.
In Radiology ; h5-index 91.0
Koetzier Lennart R, Mastrodicasa Domenico, Szczykutowicz Timothy P, van der Werf Niels R, Wang Adam S, Sandfort Veit, van der Molen Aart J, Fleischmann Dominik, Willemink Martin J
2023-Jan-31
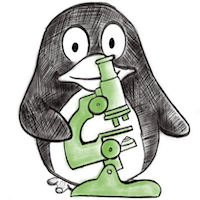
Next-Generation Morphometry for pathomics-data mining in histopathology.
In Nature communications ; h5-index 260.0
Hölscher David L, Bouteldja Nassim, Joodaki Mehdi, Russo Maria L, Lan Yu-Chia, Sadr Alireza Vafaei, Cheng Mingbo, Tesar Vladimir, Stillfried Saskia V, Klinkhammer Barbara M, Barratt Jonathan, Floege Jürgen, Roberts Ian S D, Coppo Rosanna, Costa Ivan G, Bülow Roman D, Boor Peter
2023-Jan-28
Development and Validation of a Deep Learning Algorithm to Differentiate Colon Carcinoma From Acute Diverticulitis in Computed Tomography Images.
In JAMA network open
IMPORTANCE :
OBJECTIVE :
DESIGN, SETTING, AND PARTICIPANTS :
MAIN OUTCOMES AND MEASURES :
RESULTS :
CONCLUSIONS AND RELEVANCE :
Ziegelmayer Sebastian, Reischl Stefan, Havrda Hannah, Gawlitza Joshua, Graf Markus, Lenhart Nicolas, Nehls Nadja, Lemke Tristan, Wilhelm Dirk, Lohöfer Fabian, Burian Egon, Neumann Philipp-Alexander, Makowski Marcus, Braren Rickmer
2023-Jan-03
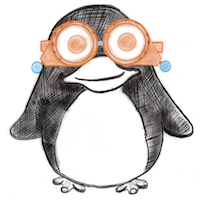
Early detection of visual impairment in young children using a smartphone-based deep learning system.
In Nature medicine ; h5-index 170.0
Chen Wenben, Li Ruiyang, Yu Qinji, Xu Andi, Feng Yile, Wang Ruixin, Zhao Lanqin, Lin Zhenzhe, Yang Yahan, Lin Duoru, Wu Xiaohang, Chen Jingjing, Liu Zhenzhen, Wu Yuxuan, Dang Kang, Qiu Kexin, Wang Zilong, Zhou Ziheng, Liu Dong, Wu Qianni, Li Mingyuan, Xiang Yifan, Li Xiaoyan, Lin Zhuoling, Zeng Danqi, Huang Yunjian, Mo Silang, Huang Xiucheng, Sun Shulin, Hu Jianmin, Zhao Jun, Wei Meirong, Hu Shoulong, Chen Liang, Dai Bingfa, Yang Huasheng, Huang Danping, Lin Xiaoming, Liang Lingyi, Ding Xiaoyan, Yang Yangfan, Wu Pengsen, Zheng Feihui, Stanojcic Nick, Li Ji-Peng Olivia, Cheung Carol Y, Long Erping, Chen Chuan, Zhu Yi, Yu-Wai-Man Patrick, Wang Ruixuan, Zheng Wei-Shi, Ding Xiaowei, Lin Haotian
2023-Jan-26
OWL: an optimized and independently validated machine learning prediction model for lung cancer screening based on the UK Biobank, PLCO, and NLST populations.
In EBioMedicine
BACKGROUND :
METHODS :
FINDINGS :
INTERPRETATION :
FUNDING :
Pan Zoucheng, Zhang Ruyang, Shen Sipeng, Lin Yunzhi, Zhang Longyao, Wang Xiang, Ye Qian, Wang Xuan, Chen Jiajin, Zhao Yang, Christiani David C, Li Yi, Chen Feng, Wei Yongyue
2023-Jan-24
External validation, Lung cancer, Machine learning, Risk prediction, UK Biobank
A wearable cardiac ultrasound imager.
In Nature ; h5-index 368.0
Hu Hongjie, Huang Hao, Li Mohan, Gao Xiaoxiang, Yin Lu, Qi Ruixiang, Wu Ray S, Chen Xiangjun, Ma Yuxiang, Shi Keren, Li Chenghai, Maus Timothy M, Huang Brady, Lu Chengchangfeng, Lin Muyang, Zhou Sai, Lou Zhiyuan, Gu Yue, Chen Yimu, Lei Yusheng, Wang Xinyu, Wang Ruotao, Yue Wentong, Yang Xinyi, Bian Yizhou, Mu Jing, Park Geonho, Xiang Shu, Cai Shengqiang, Corey Paul W, Wang Joseph, Xu Sheng
2023-Jan
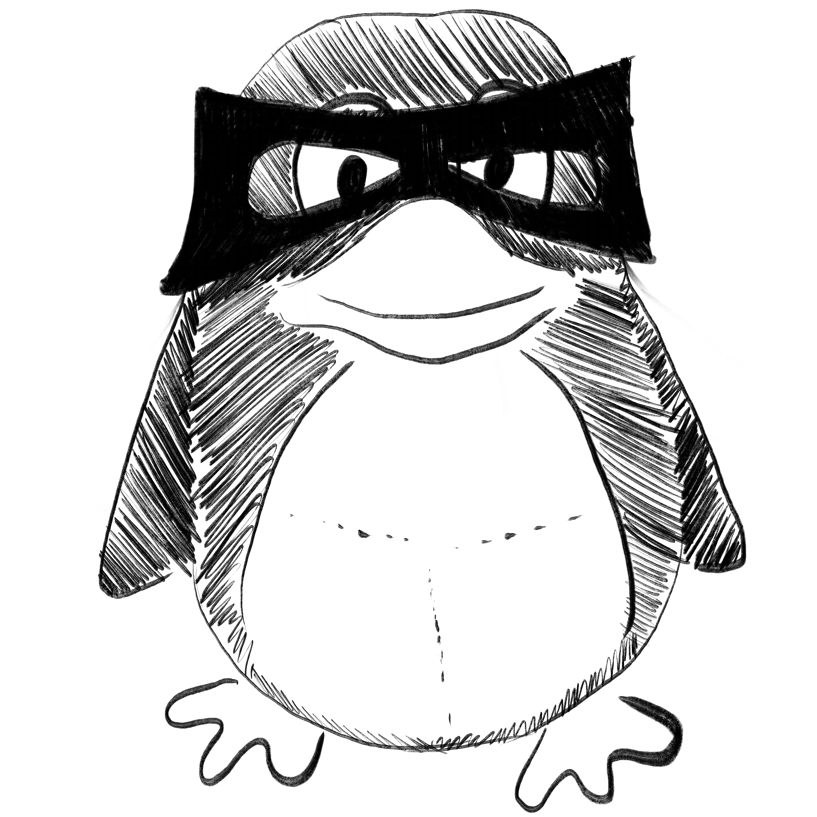
Topological identification and interpretation for single-cell gene regulation elucidation across multiple platforms using scMGCA.
In Nature communications ; h5-index 260.0
Yu Zhuohan, Su Yanchi, Lu Yifu, Yang Yuning, Wang Fuzhou, Zhang Shixiong, Chang Yi, Wong Ka-Chun, Li Xiangtao
2023-Jan-25
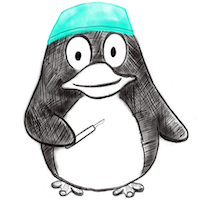
Delineating epileptogenic networks using brain imaging data and personalized modeling in drug-resistant epilepsy.
In Science translational medicine ; h5-index 138.0
Wang Huifang E, Woodman Marmaduke, Triebkorn Paul, Lemarechal Jean-Didier, Jha Jayant, Dollomaja Borana, Vattikonda Anirudh Nihalani, Sip Viktor, Medina Villalon Samuel, Hashemi Meysam, Guye Maxime, Makhalova Julia, Bartolomei Fabrice, Jirsa Viktor
2023-Jan-25
Development and Validation of a Machine Learning Model for Detection and Classification of Tertiary Lymphoid Structures in Gastrointestinal Cancers.
In JAMA network open
IMPORTANCE :
OBJECTIVE :
DESIGN, SETTING, AND PARTICIPANTS :
MAIN OUTCOMES AND MEASURES :
RESULTS :
CONCLUSIONS AND RELEVANCE :
Li Zhe, Jiang Yuming, Li Bailiang, Han Zhen, Shen Jeanne, Xia Yong, Li Ruijiang
2023-Jan-03
Predictive Accuracy of Stroke Risk Prediction Models Across Black and White Race, Sex, and Age Groups.
In JAMA ; h5-index 211.0
IMPORTANCE :
OBJECTIVE :
DESIGN, SETTING, AND PARTICIPANTS :
EXPOSURES :
MAIN OUTCOMES AND MEASURES :
RESULTS :
CONCLUSIONS AND RELEVANCE :
Hong Chuan, Pencina Michael J, Wojdyla Daniel M, Hall Jennifer L, Judd Suzanne E, Cary Michael, Engelhard Matthew M, Berchuck Samuel, Xian Ying, D’Agostino Ralph, Howard George, Kissela Brett, Henao Ricardo
2023-Jan-24
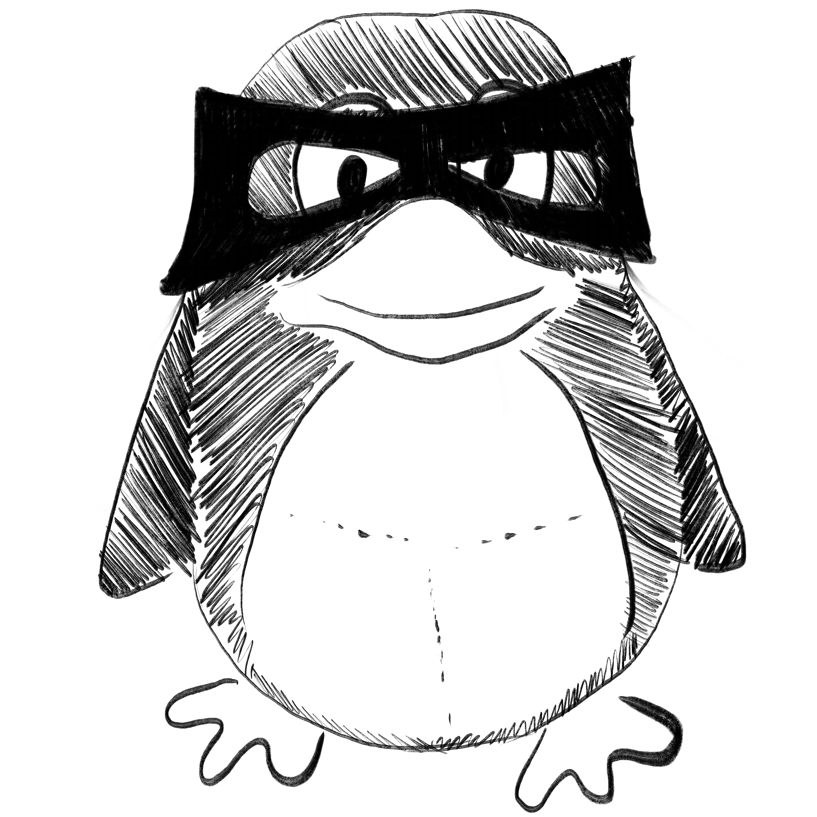
Explainable deep learning for insights in El Niño and river flows.
In Nature communications ; h5-index 260.0
Liu Yumin, Duffy Kate, Dy Jennifer G, Ganguly Auroop R
2023-Jan-20
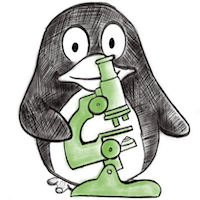
Multiplexed 3D atlas of state transitions and immune interaction in colorectal cancer.
In Cell ; h5-index 250.0
Lin Jia-Ren, Wang Shu, Coy Shannon, Chen Yu-An, Yapp Clarence, Tyler Madison, Nariya Maulik K, Heiser Cody N, Lau Ken S, Santagata Sandro, Sorger Peter K
2023-Jan-19
3D microscopy, PD1-PDL1 interaction, cellular, colorectal cancer, intermixed molecular, large-scale, morphological features, multiplexed imaging, spatial gradients, spatial proteomics, spatial transcriptomics, tertiary lymphoid structures, tumor atlas, tumor budding
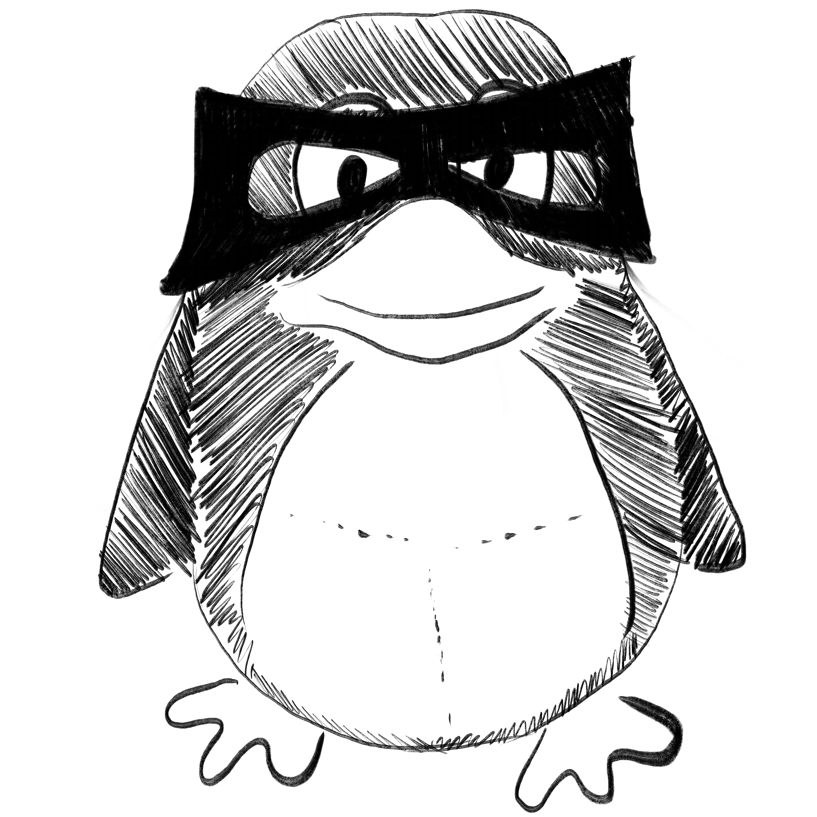
Multienzyme deep learning models improve peptide de novo sequencing by mass spectrometry proteomics.
In PLoS computational biology
Gueto-Tettay Carlos, Tang Di, Happonen Lotta, Heusel Moritz, Khakzad Hamed, Malmström Johan, Malmström Lars
2023-Jan-20
Association of Neighborhood Deprivation With Prostate Cancer and Immune Markers in African American and European American Men.
In JAMA network open
IMPORTANCE :
OBJECTIVE :
DESIGN, SETTING, AND PARTICIPANTS :
EXPOSURES :
MAIN OUTCOMES AND MEASURES :
RESULTS :
CONCLUSIONS AND RELEVANCE :
Pichardo Margaret S, Minas Tsion Zewdu, Pichardo Catherine M, Bailey-Whyte Maeve, Tang Wei, Dorsey Tiffany H, Wooten William, Ryan Brid M, Loffredo Christopher A, Ambs Stefan
2023-Jan-03
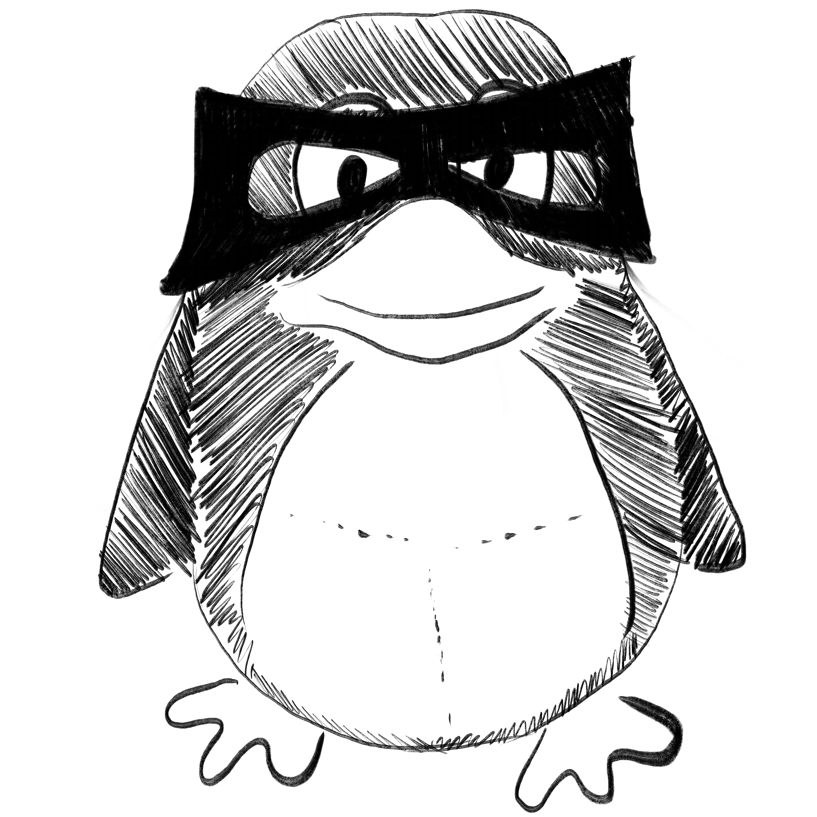
A wearable motion capture suit and machine learning predict disease progression in Friedreich's ataxia.
In Nature medicine ; h5-index 170.0
Kadirvelu Balasundaram, Gavriel Constantinos, Nageshwaran Sathiji, Chan Jackson Ping Kei, Nethisinghe Suran, Athanasopoulos Stavros, Ricotti Valeria, Voit Thomas, Giunti Paola, Festenstein Richard, Faisal A Aldo
2023-Jan-19
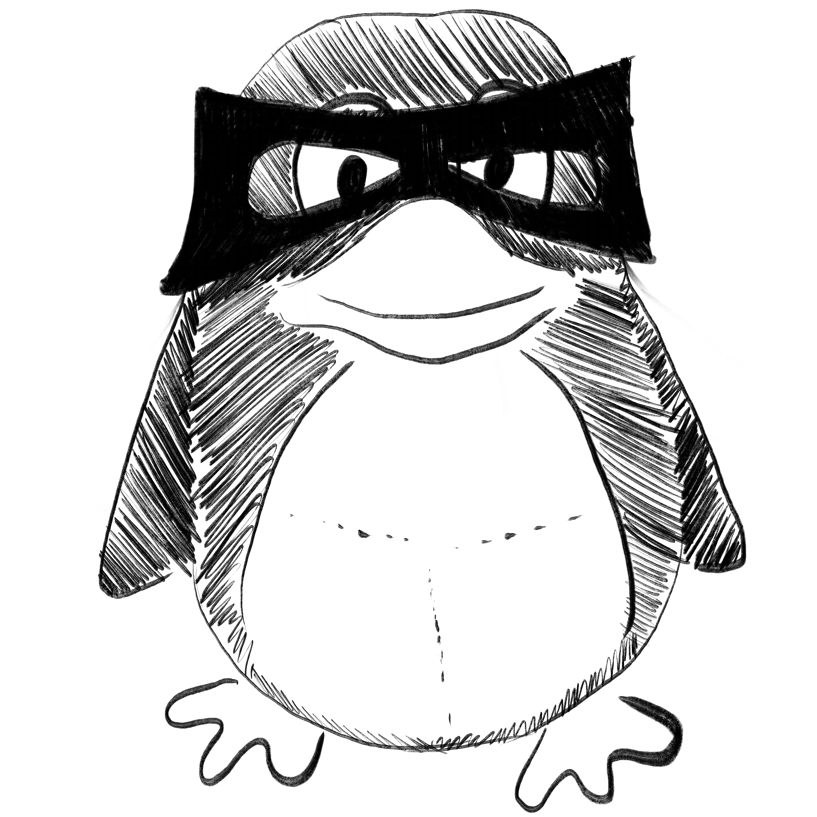
Federated learning for predicting histological response to neoadjuvant chemotherapy in triple-negative breast cancer.
In Nature medicine ; h5-index 170.0
Ogier du Terrail Jean, Leopold Armand, Joly Clément, Béguier Constance, Andreux Mathieu, Maussion Charles, Schmauch Benoît, Tramel Eric W, Bendjebbar Etienne, Zaslavskiy Mikhail, Wainrib Gilles, Milder Maud, Gervasoni Julie, Guerin Julien, Durand Thierry, Livartowski Alain, Moutet Kelvin, Gautier Clément, Djafar Inal, Moisson Anne-Laure, Marini Camille, Galtier Mathieu, Balazard Félix, Dubois Rémy, Moreira Jeverson, Simon Antoine, Drubay Damien, Lacroix-Triki Magali, Franchet Camille, Bataillon Guillaume, Heudel Pierre-Etienne
2023-Jan-19
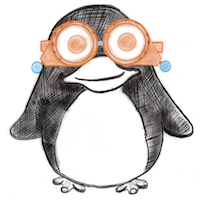
Assessment of Retinopathy of Prematurity Regression and Reactivation Using an Artificial Intelligence-Based Vascular Severity Score.
In JAMA network open
IMPORTANCE :
OBJECTIVE :
DESIGN, SETTING, AND PARTICIPANTS :
INTERVENTIONS :
MAIN OUTCOMES AND MEASURES :
RESULTS :
CONCLUSIONS AND RELEVANCE :
Eilts Sonja K, Pfeil Johanna M, Poschkamp Broder, Krohne Tim U, Eter Nicole, Barth Teresa, Guthoff Rainer, Lagrèze Wolf, Grundel Milena, Bründer Marie-Christine, Busch Martin, Kalpathy-Cramer Jayashree, Chiang Michael F, Chan R V Paul, Coyner Aaron S, Ostmo Susan, Campbell J Peter, Stahl Andreas
2023-Jan-03
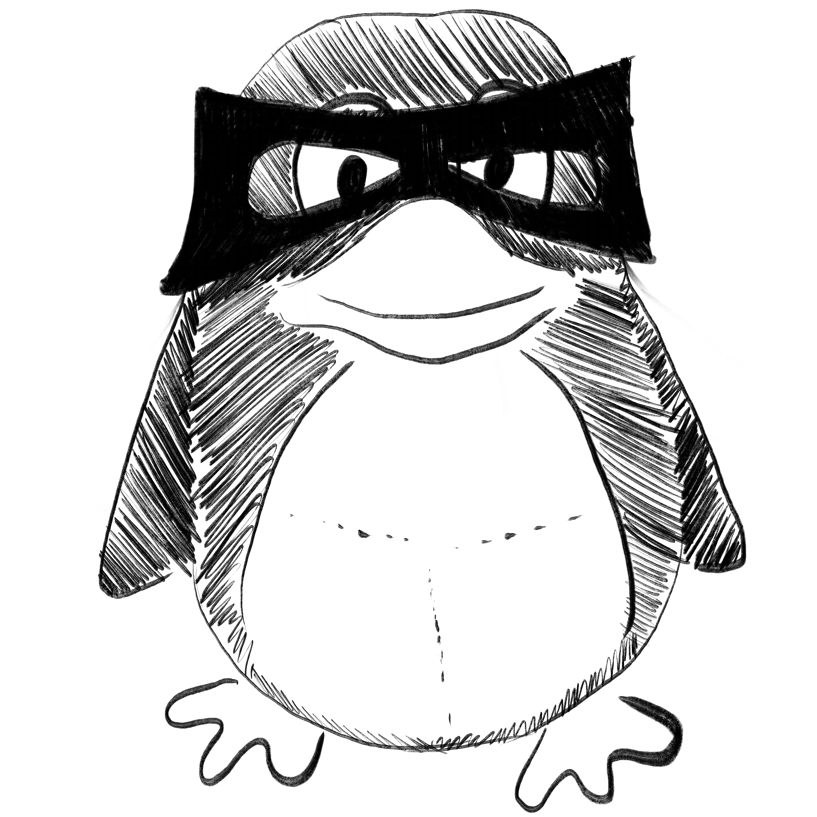
Wearable full-body motion tracking of activities of daily living predicts disease trajectory in Duchenne muscular dystrophy.
In Nature medicine ; h5-index 170.0
Ricotti Valeria, Kadirvelu Balasundaram, Selby Victoria, Festenstein Richard, Mercuri Eugenio, Voit Thomas, Faisal A Aldo
2023-Jan-19
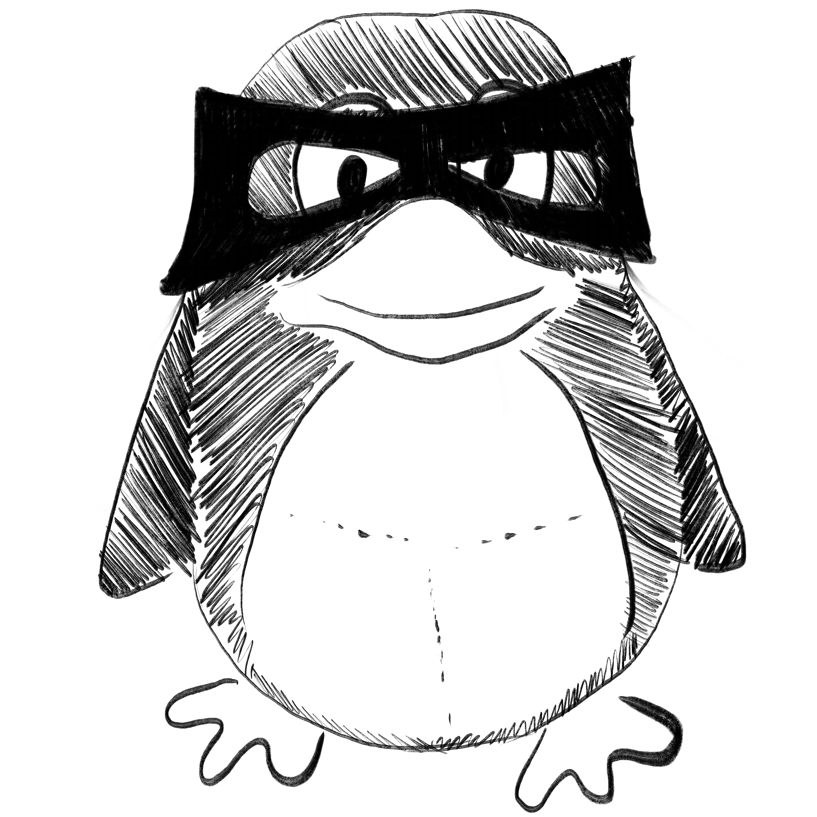
BMI-adjusted adipose tissue volumes exhibit depot-specific and divergent associations with cardiometabolic diseases.
In Nature communications ; h5-index 260.0
Agrawal Saaket, Klarqvist Marcus D R, Diamant Nathaniel, Stanley Takara L, Ellinor Patrick T, Mehta Nehal N, Philippakis Anthony, Ng Kenney, Claussnitzer Melina, Grinspoon Steven K, Batra Puneet, Khera Amit V
2023-Jan-17
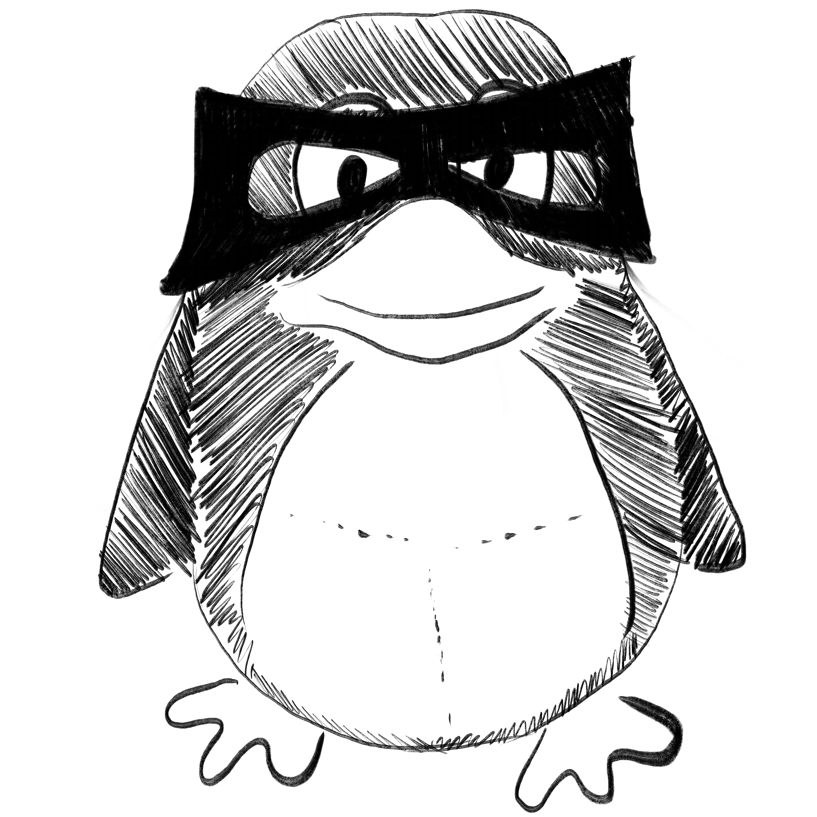
Transcriptional vulnerabilities of striatal neurons in human and rodent models of Huntington's disease.
In Nature communications ; h5-index 260.0
Matsushima Ayano, Pineda Sergio Sebastian, Crittenden Jill R, Lee Hyeseung, Galani Kyriakitsa, Mantero Julio, Tombaugh Geoffrey, Kellis Manolis, Heiman Myriam, Graybiel Ann M
2023-Jan-17
Deep Learning Reconstruction Enables Prospectively Accelerated Clinical Knee MRI.
In Radiology ; h5-index 91.0
Johnson Patricia M, Lin Dana J, Zbontar Jure, Zitnick C Lawrence, Sriram Anuroop, Muckley Matthew, Babb James S, Kline Mitchell, Ciavarra Gina, Alaia Erin, Samim Mohammad, Walter William R, Calderon Liz, Pock Thomas, Sodickson Daniel K, Recht Michael P, Knoll Florian
2023-Jan-17
Deep Learning Analysis of Chest Radiographs to Triage Patients with Acute Chest Pain Syndrome.
In Radiology ; h5-index 91.0
Kolossváry Márton, Raghu Vineet K, Nagurney John T, Hoffmann Udo, Lu Michael T
2023-Jan-17
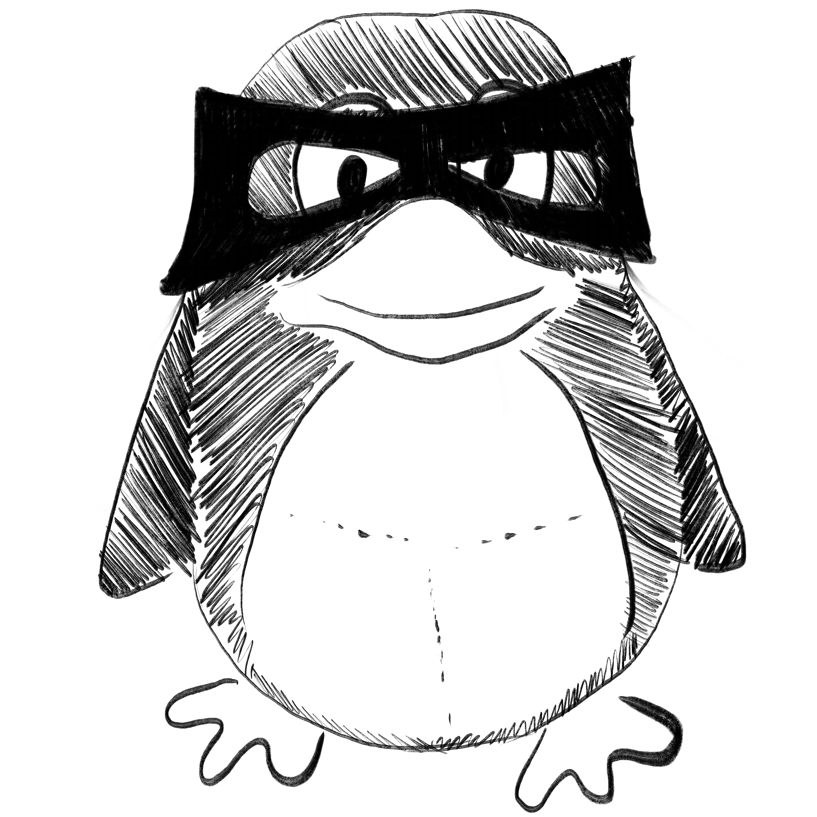
Risk Factors for Pediatric Sepsis in the Emergency Department: A Machine Learning Pilot Study.
In Pediatric emergency care
OBJECTIVE :
METHODS :
RESULTS :
CONCLUSIONS :
Mercurio Laura, Pou Sovijja, Duffy Susan, Eickhoff Carsten
2023-Jan-17
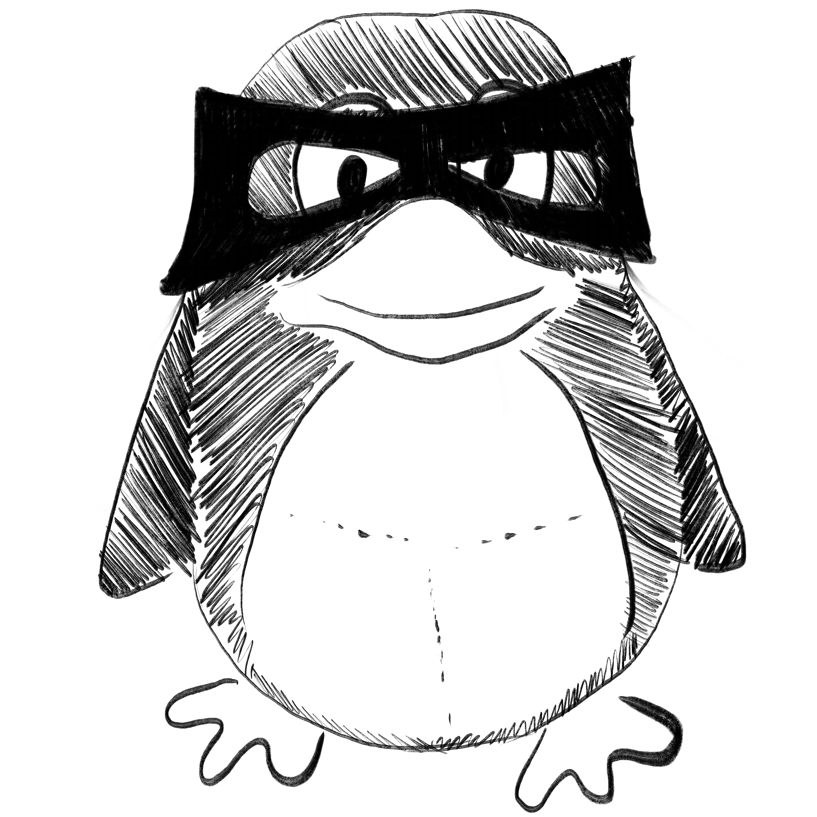
The next generation of evidence-based medicine.
In Nature medicine ; h5-index 170.0
Subbiah Vivek
2023-Jan-16
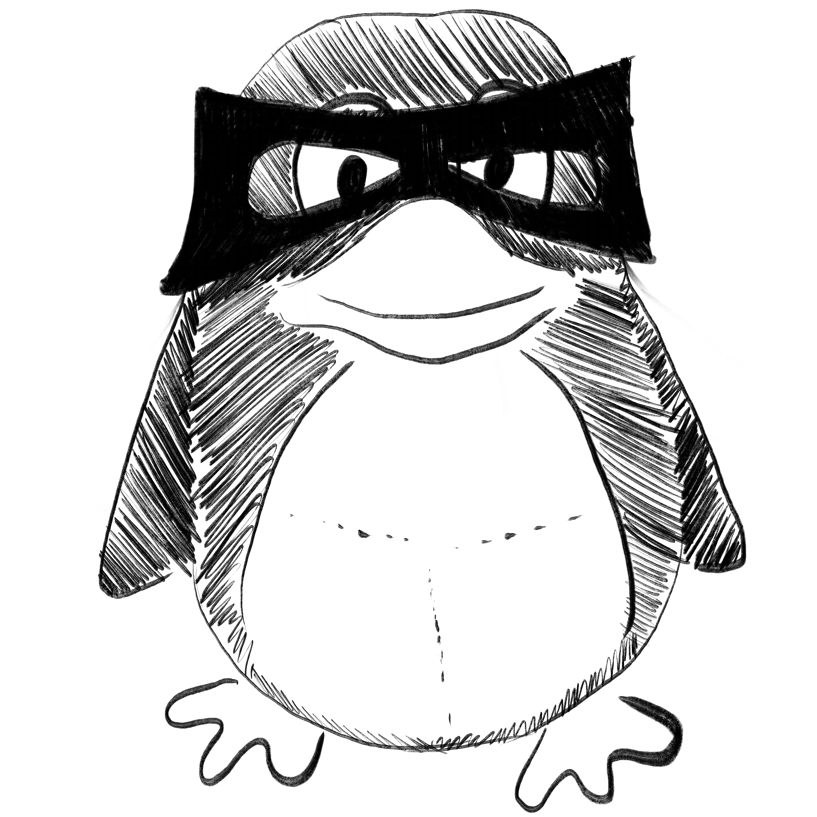
Comparative analysis of genome-scale, base-resolution DNA methylation profiles across 580 animal species.
In Nature communications ; h5-index 260.0
Klughammer Johanna, Romanovskaia Daria, Nemc Amelie, Posautz Annika, Seid Charlotte A, Schuster Linda C, Keinath Melissa C, Lugo Ramos Juan Sebastian, Kosack Lindsay, Evankow Ann, Printz Dieter, Kirchberger Stefanie, Ergüner Bekir, Datlinger Paul, Fortelny Nikolaus, Schmidl Christian, Farlik Matthias, Skjærven Kaja, Bergthaler Andreas, Liedvogel Miriam, Thaller Denise, Burger Pamela A, Hermann Marcela, Distel Martin, Distel Daniel L, Kübber-Heiss Anna, Bock Christoph
2023-Jan-16
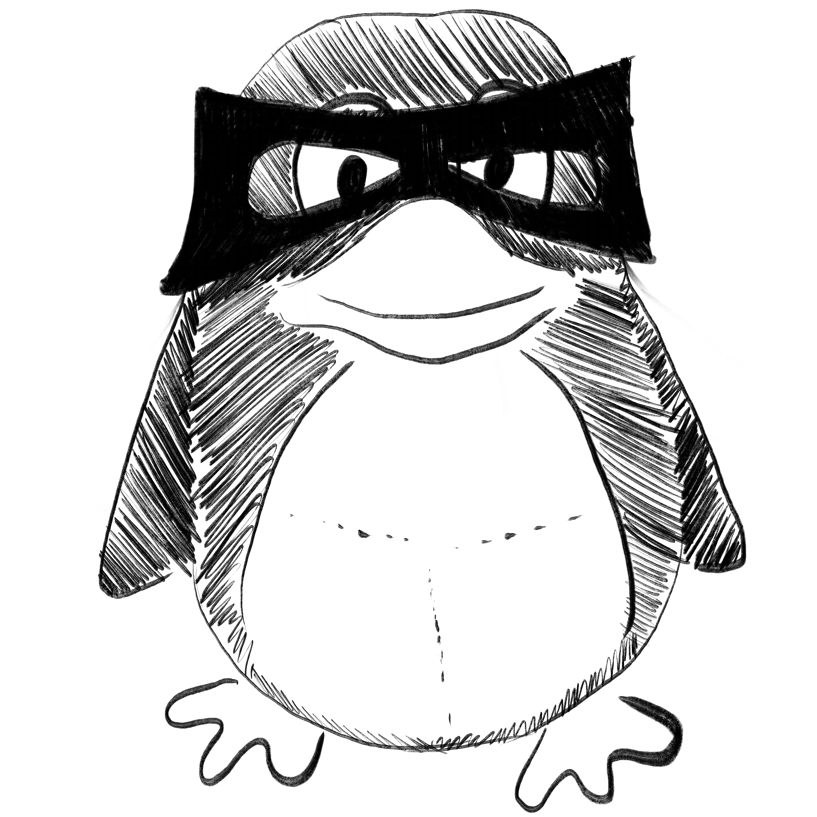
The next generation of evidence-based medicine.
In Nature medicine ; h5-index 170.0
Subbiah Vivek
2023-Jan-16
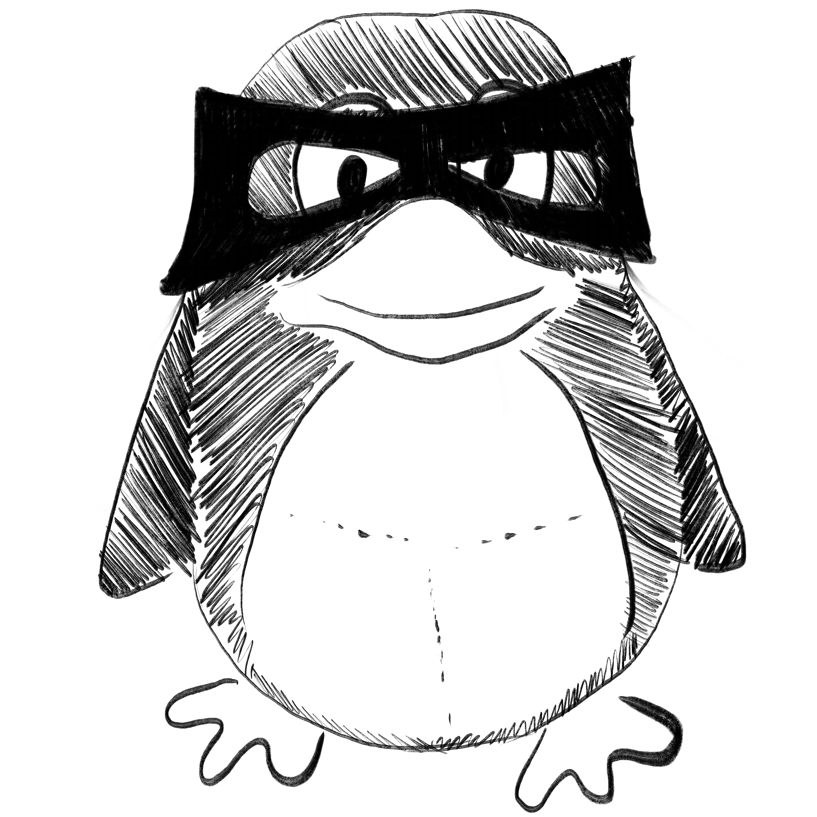
Transformer for one stop interpretable cell type annotation.
In Nature communications ; h5-index 260.0
Chen Jiawei, Xu Hao, Tao Wanyu, Chen Zhaoxiong, Zhao Yuxuan, Han Jing-Dong J
2023-Jan-14
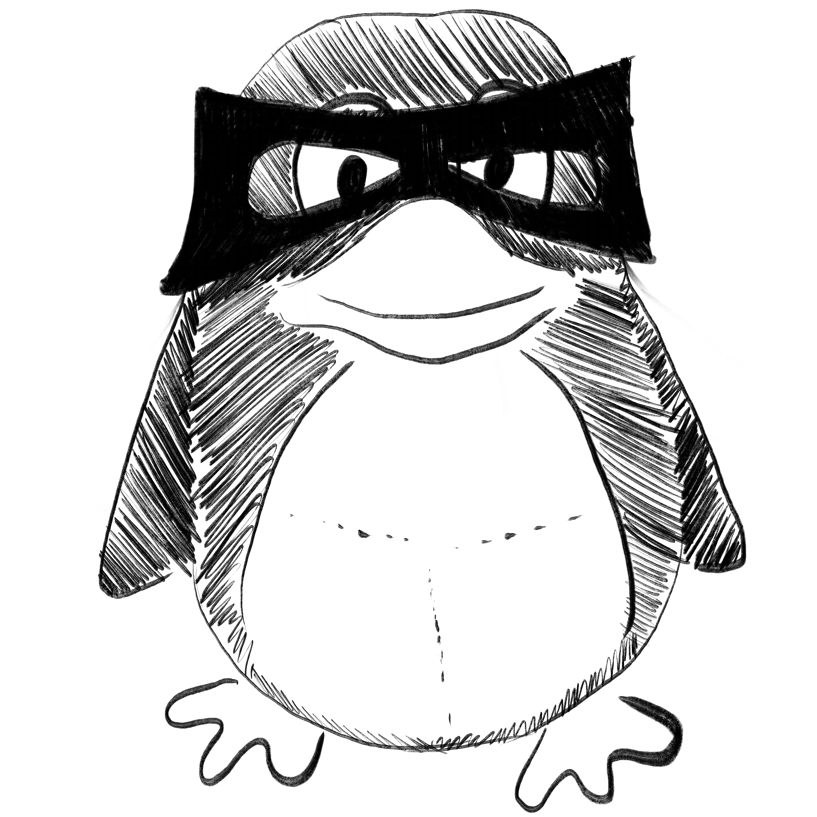
Transformer for one stop interpretable cell type annotation.
In Nature communications ; h5-index 260.0
Chen Jiawei, Xu Hao, Tao Wanyu, Chen Zhaoxiong, Zhao Yuxuan, Han Jing-Dong J
2023-Jan-14
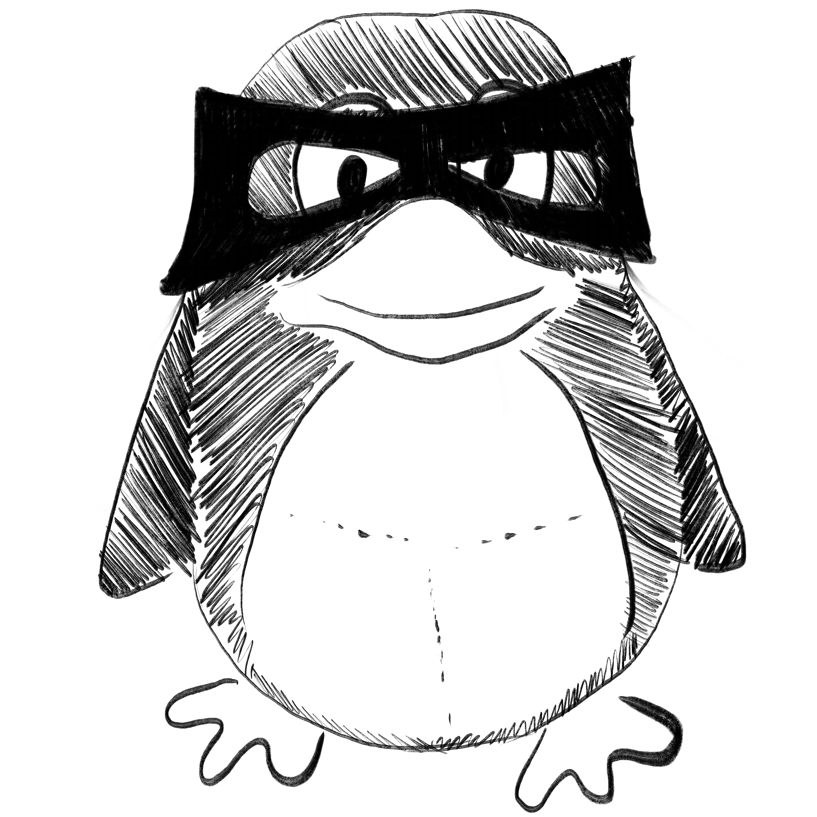
Effect of a Machine Learning Recommender System and Viral Peer Marketing Intervention on Smoking Cessation: A Randomized Clinical Trial.
In JAMA network open
IMPORTANCE :
OBJECTIVE :
DESIGN, SETTING, AND PARTICIPANTS :
INTERVENTIONS :
MAIN OUTCOMES AND MEASURES :
RESULTS :
CONCLUSIONS AND RELEVANCE :
TRIAL REGISTRATION :
Faro Jamie M, Chen Jinying, Flahive Julie, Nagawa Catherine S, Orvek Elizabeth A, Houston Thomas K, Allison Jeroan J, Person Sharina D, Smith Bridget M, Blok Amanda C, Sadasivam Rajani S
2023-Jan-03
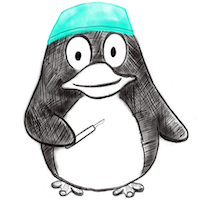
Genome-wide analysis of aberrant position and sequence of plasma DNA fragment ends in patients with cancer.
In Science translational medicine ; h5-index 138.0
Budhraja Karan K, McDonald Bradon R, Stephens Michelle D, Contente-Cuomo Tania, Markus Havell, Farooq Maria, Favaro Patricia F, Connor Sydney, Byron Sara A, Egan Jan B, Ernst Brenda, McDaniel Timothy K, Sekulic Aleksandar, Tran Nhan L, Prados Michael D, Borad Mitesh J, Berens Michael E, Pockaj Barbara A, LoRusso Patricia M, Bryce Alan, Trent Jeffrey M, Murtaza Muhammed
2023-Jan-11
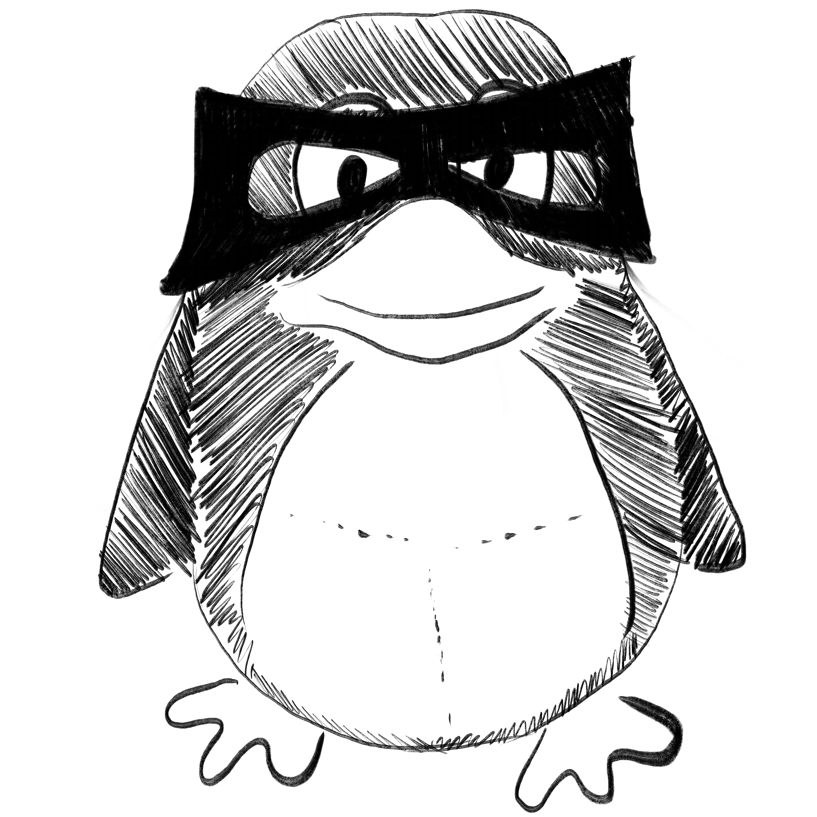
Spikebench: An open benchmark for spike train time-series classification.
In PLoS computational biology
Lazarevich Ivan, Prokin Ilya, Gutkin Boris, Kazantsev Victor
2023-Jan-10
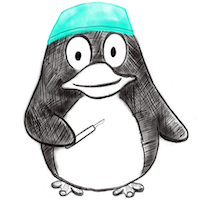
Multistain deep learning for prediction of prognosis and therapy response in colorectal cancer.
In Nature medicine ; h5-index 170.0
Foersch Sebastian, Glasner Christina, Woerl Ann-Christin, Eckstein Markus, Wagner Daniel-Christoph, Schulz Stefan, Kellers Franziska, Fernandez Aurélie, Tserea Konstantina, Kloth Michael, Hartmann Arndt, Heintz Achim, Weichert Wilko, Roth Wilfried, Geppert Carol, Kather Jakob Nikolas, Jesinghaus Moritz
2023-Jan-09
Galaxy Training: A powerful framework for teaching!
In PLoS computational biology
Hiltemann Saskia, Rasche Helena, Gladman Simon, Hotz Hans-Rudolf, Larivière Delphine, Blankenberg Daniel, Jagtap Pratik D, Wollmann Thomas, Bretaudeau Anthony, Goué Nadia, Griffin Timothy J, Royaux Coline, Le Bras Yvan, Mehta Subina, Syme Anna, Coppens Frederik, Droesbeke Bert, Soranzo Nicola, Bacon Wendi, Psomopoulos Fotis, Gallardo-Alba Cristóbal, Davis John, Föll Melanie Christine, Fahrner Matthias, Doyle Maria A, Serrano-Solano Beatriz, Fouilloux Anne Claire, van Heusden Peter, Maier Wolfgang, Clements Dave, Heyl Florian, Grüning Björn, Batut Bérénice
2023-Jan
Early response evaluation by single cell signaling profiling in acute myeloid leukemia.
In Nature communications ; h5-index 260.0
Tislevoll Benedicte Sjo, Hellesøy Monica, Fagerholt Oda Helen Eck, Gullaksen Stein-Erik, Srivastava Aashish, Birkeland Even, Kleftogiannis Dimitrios, Ayuda-Durán Pilar, Piechaczyk Laure, Tadele Dagim Shiferaw, Skavland Jørn, Panagiotis Baliakas, Hovland Randi, Andresen Vibeke, Seternes Ole Morten, Tvedt Tor Henrik Anderson, Aghaeepour Nima, Gavasso Sonia, Porkka Kimmo, Jonassen Inge, Fløisand Yngvar, Enserink Jorrit, Blaser Nello, Gjertsen Bjørn Tore
2023-Jan-07
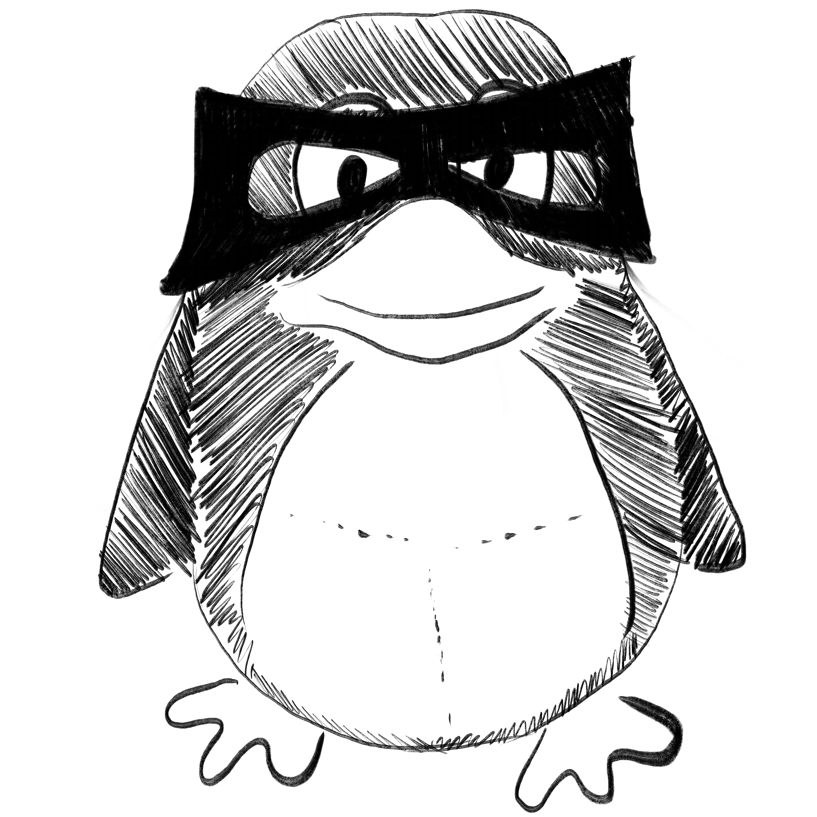
Over-optimism in unsupervised microbiome analysis: Insights from network learning and clustering.
In PLoS computational biology
Ullmann Theresa, Peschel Stefanie, Finger Philipp, Müller Christian L, Boulesteix Anne-Laure
2023-Jan-06
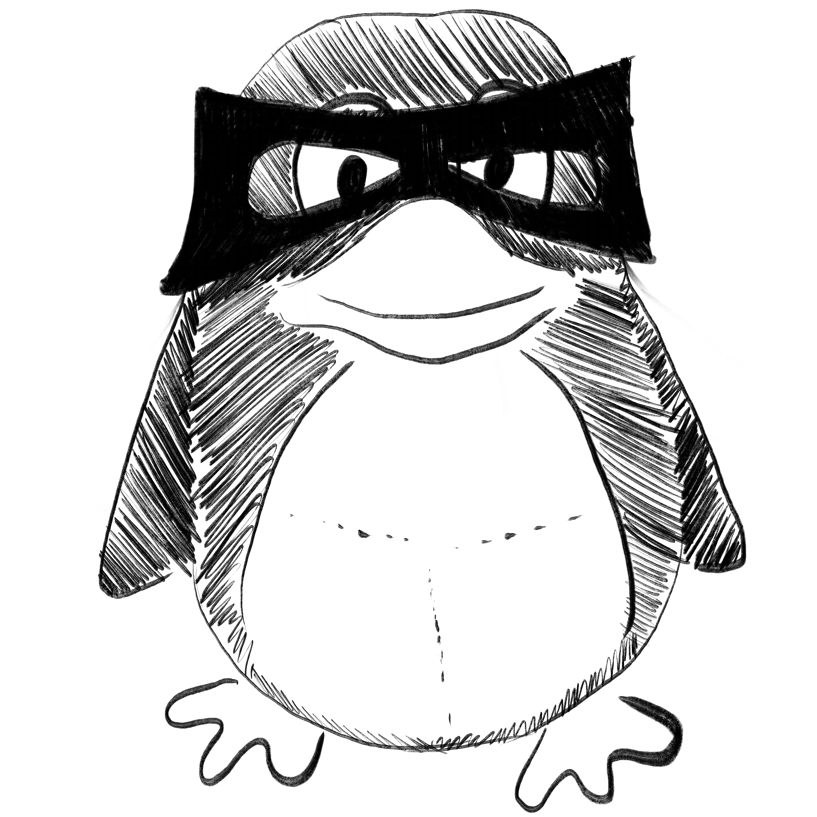
Photonic machine learning with on-chip diffractive optics.
In Nature communications ; h5-index 260.0
Fu Tingzhao, Zang Yubin, Huang Yuyao, Du Zhenmin, Huang Honghao, Hu Chengyang, Chen Minghua, Yang Sigang, Chen Hongwei
2023-Jan-05
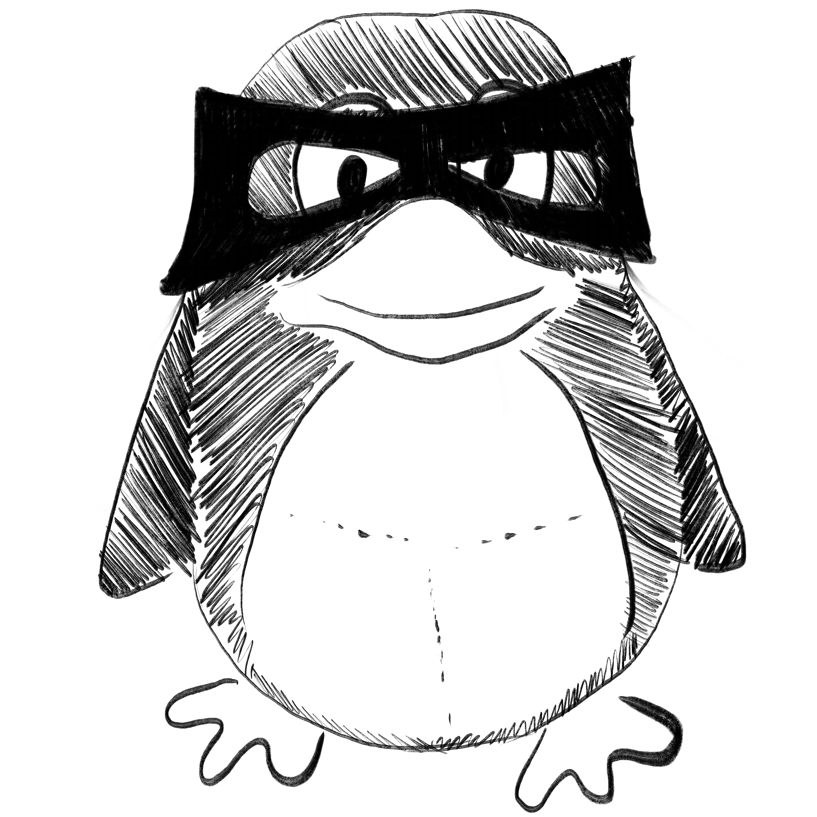
Microcomb-based integrated photonic processing unit.
In Nature communications ; h5-index 260.0
Bai Bowen, Yang Qipeng, Shu Haowen, Chang Lin, Yang Fenghe, Shen Bitao, Tao Zihan, Wang Jing, Xu Shaofu, Xie Weiqiang, Zou Weiwen, Hu Weiwei, Bowers John E, Wang Xingjun
2023-Jan-05
Computational pathology in 2030: a Delphi study forecasting the role of AI in pathology within the next decade.
In EBioMedicine
BACKGROUND :
METHODS :
FINDINGS :
INTERPRETATION :
FUNDING :
Berbís M Alvaro, McClintock David S, Bychkov Andrey, Van der Laak Jeroen, Pantanowitz Liron, Lennerz Jochen K, Cheng Jerome Y, Delahunt Brett, Egevad Lars, Eloy Catarina, Farris Alton B, Fraggetta Filippo, García Del Moral Raimundo, Hartman Douglas J, Herrmann Markus D, Hollemans Eva, Iczkowski Kenneth A, Karsan Aly, Kriegsmann Mark, Salama Mohamed E, Sinard John H, Tuthill J Mark, Williams Bethany, Casado-Sánchez César, Sánchez-Turrión Víctor, Luna Antonio, Aneiros-Fernández José, Shen Jeanne
2023-Jan-03
Anatomic pathology, Artificial intelligence, Computational pathology, Digital pathology, Machine learning, Pathologist workflow
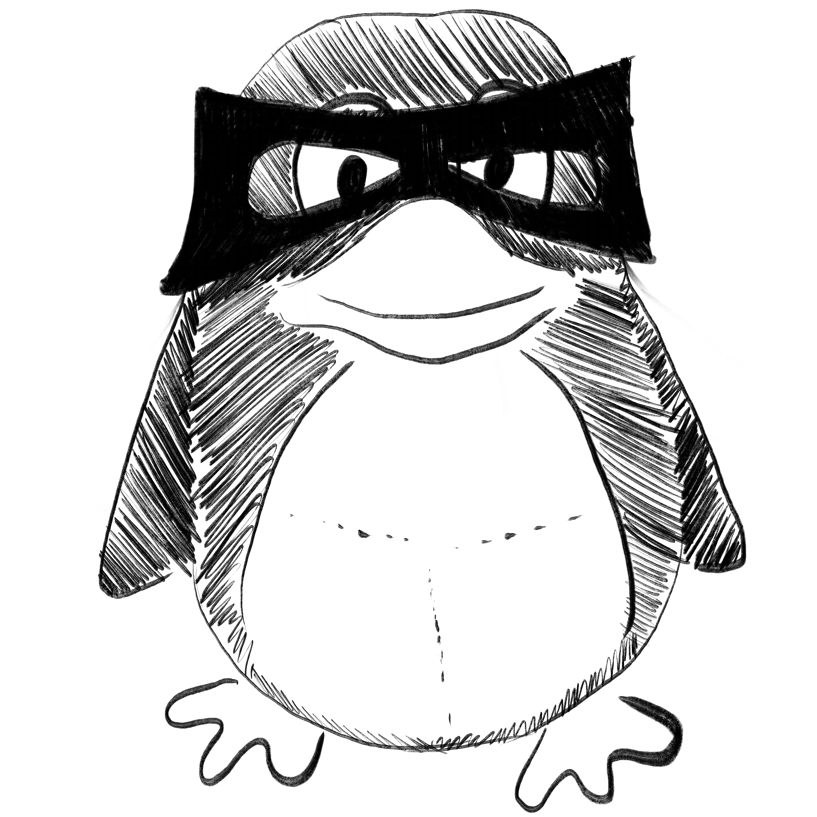
Ten quick tips for computational analysis of medical images.
In PLoS computational biology
Chicco Davide, Shiradkar Rakesh
2023-Jan
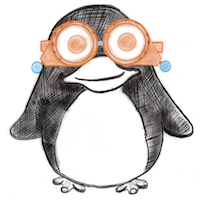
Rapid, label-free histopathological diagnosis of liver cancer based on Raman spectroscopy and deep learning.
In Nature communications ; h5-index 260.0
Huang Liping, Sun Hongwei, Sun Liangbin, Shi Keqing, Chen Yuzhe, Ren Xueqian, Ge Yuancai, Jiang Danfeng, Liu Xiaohu, Knoll Wolfgang, Zhang Qingwen, Wang Yi
2023-Jan-04
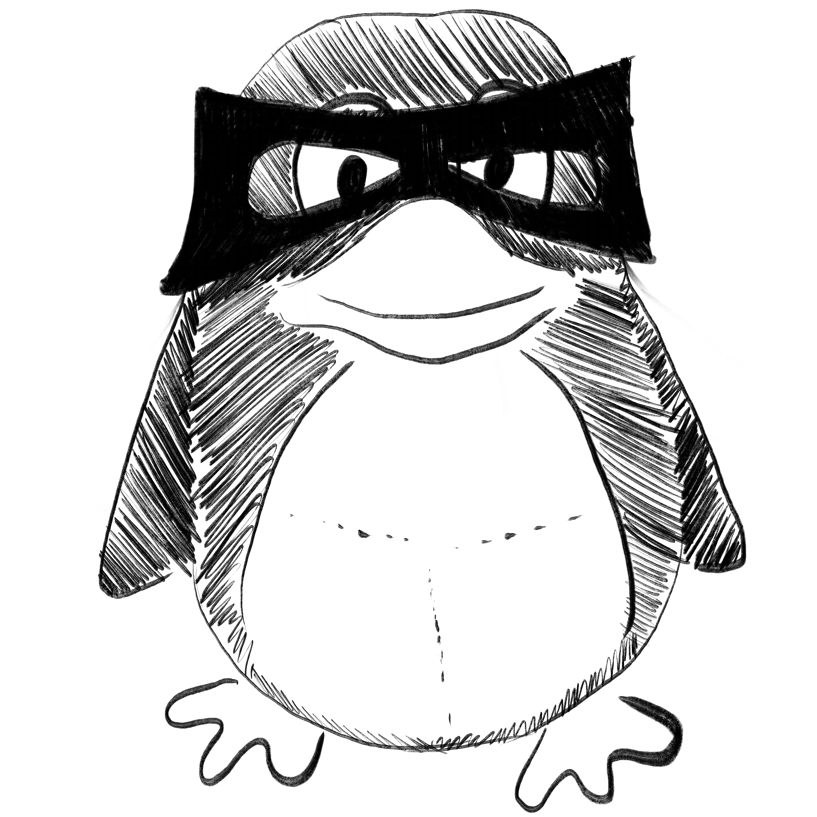
Cerebro-cerebellar networks facilitate learning through feedback decoupling.
In Nature communications ; h5-index 260.0
Boven Ellen, Pemberton Joseph, Chadderton Paul, Apps Richard, Costa Rui Ponte
2023-Jan-04
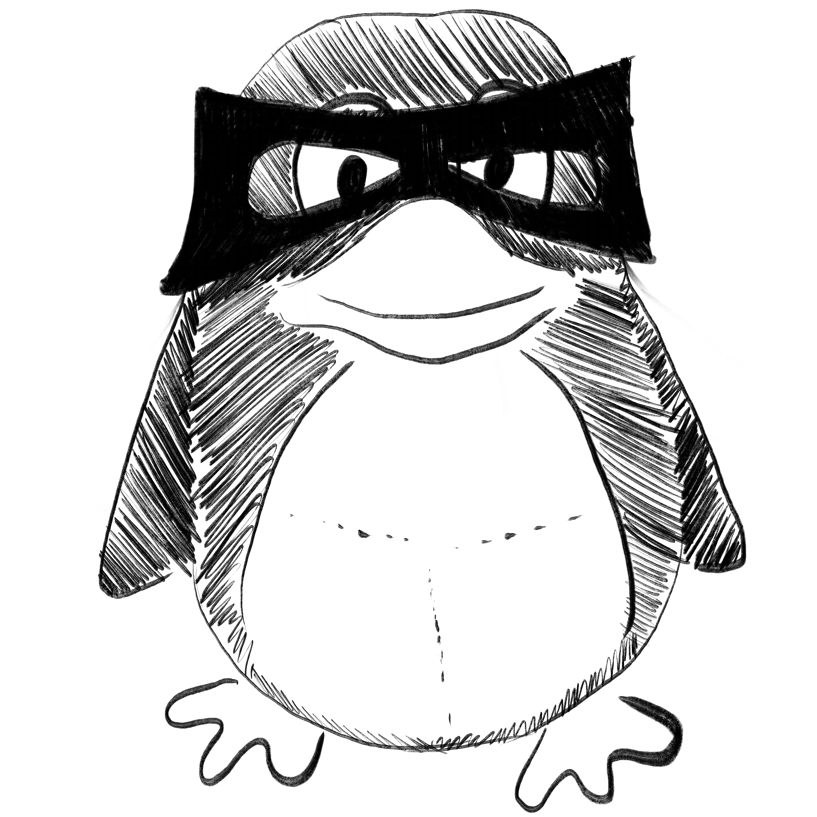
Development of a Machine Learning Model for Sonographic Assessment of Gestational Age.
In JAMA network open
IMPORTANCE :
OBJECTIVE :
DESIGN, SETTING, AND PARTICIPANTS :
MAIN OUTCOMES AND MEASURES :
RESULTS :
CONCLUSIONS AND RELEVANCE :
Lee Chace, Willis Angelica, Chen Christina, Sieniek Marcin, Watters Amber, Stetson Bethany, Uddin Akib, Wong Jonny, Pilgrim Rory, Chou Katherine, Tse Daniel, Shetty Shravya, Gomes Ryan G
2023-Jan-03
Two-dimensional Convolutional Neural Network Using Quantitative US for Noninvasive Assessment of Hepatic Steatosis in NAFLD.
In Radiology ; h5-index 91.0
Jeon Sun Kyung, Lee Jeong Min, Joo Ijin, Yoon Jeong Hee, Lee Gunwoo
2023-Jan-03
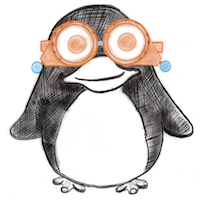
Artificial intelligence for the diagnosis of retinopathy of prematurity: A systematic review of current algorithms.
In Eye (London, England) ; h5-index 41.0
BACKGROUND/OBJECTIVES :
SUBJECT/METHODS :
RESULTS :
CONCLUSION :
Ramanathan Ashwin, Athikarisamy Sam Ebenezer, Lam Geoffrey C
2022-Dec-28
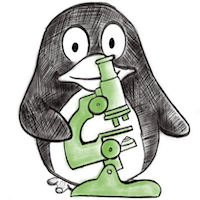
An accurate prediction of the origin for bone metastatic cancer using deep learning on digital pathological images.
In EBioMedicine
BACKGROUND :
METHODS :
FINDINGS :
INTERPRETATION :
FUNDING :
Zhu Lianghui, Shi Huijuan, Wei Huiting, Wang Chengjiang, Shi Shanshan, Zhang Fenfen, Yan Renao, Liu Yiqing, He Tingting, Wang Liyuan, Cheng Junru, Duan Hufei, Du Hong, Meng Fengjiao, Zhao Wenli, Gu Xia, Guo Linlang, Ni Yingpeng, He Yonghong, Guan Tian, Han Anjia
2022-Dec-26
Bone metastatic cancer, Deep learning, Digital pathology, Origin, Regional multiple-instance learning
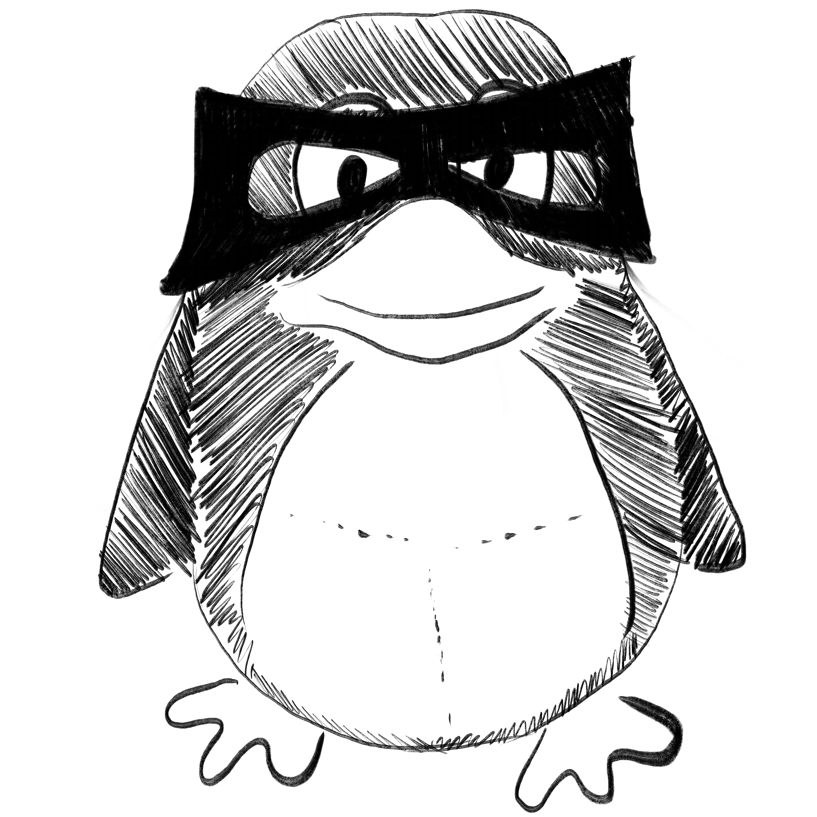
Multi-horizon predictive models for guiding extracorporeal resource allocation in critically ill COVID-19 patients.
In Journal of the American Medical Informatics Association : JAMIA
OBJECTIVE :
MATERIAL AND METHODS :
RESULTS :
DISCUSSION AND CONCLUSION :
Xue Bing, Shah Neel, Yang Hanqing, Kannampallil Thomas, Payne Philip Richard Orrin, Lu Chenyang, Said Ahmed Sameh
2022-Dec-28
COVID-19, ECMO, early alert, machine learning, prediction, resource allocation
Validation of a Deep Learning-Based Model to Predict Lung Cancer Risk Using Chest Radiographs and Electronic Medical Record Data.
In JAMA network open
IMPORTANCE :
OBJECTIVE :
DESIGN, SETTING, AND PARTICIPANTS :
EXPOSURES :
MAIN OUTCOMES AND MEASURES :
RESULTS :
CONCLUSIONS AND RELEVANCE :
Raghu Vineet K, Walia Anika S, Zinzuwadia Aniket N, Goiffon Reece J, Shepard Jo-Anne O, Aerts Hugo J W L, Lennes Inga T, Lu Michael T
2022-Dec-01
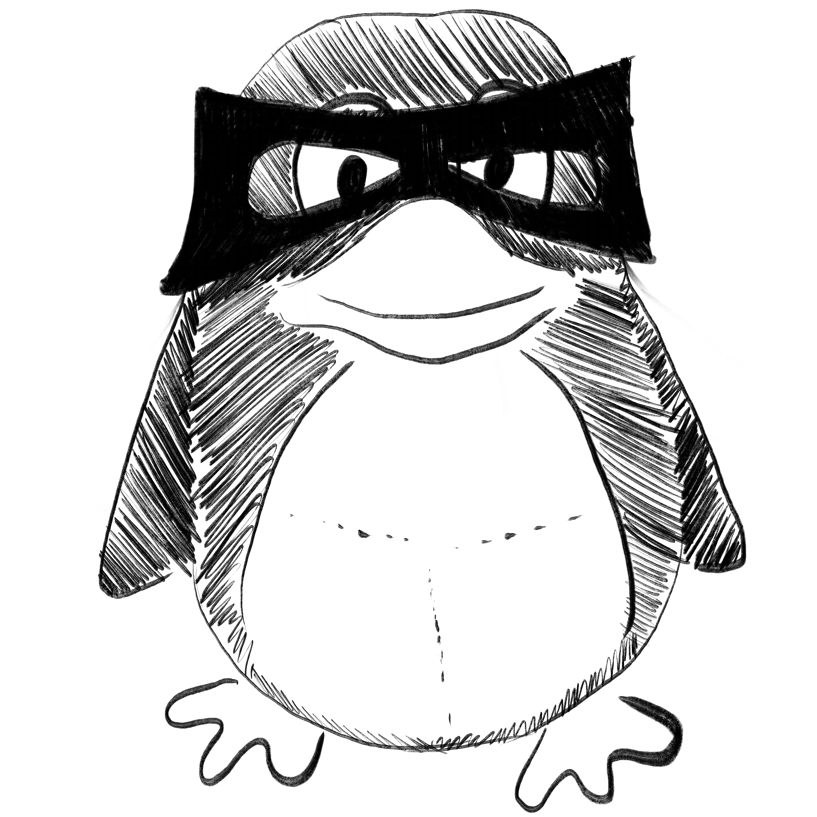
Multi-horizon predictive models for guiding extracorporeal resource allocation in critically ill COVID-19 patients.
In Journal of the American Medical Informatics Association : JAMIA
OBJECTIVE :
MATERIAL AND METHODS :
RESULTS :
DISCUSSION AND CONCLUSION :
Xue Bing, Shah Neel, Yang Hanqing, Kannampallil Thomas, Payne Philip Richard Orrin, Lu Chenyang, Said Ahmed Sameh
2022-Dec-28
COVID-19, ECMO, early alert, machine learning, prediction, resource allocation
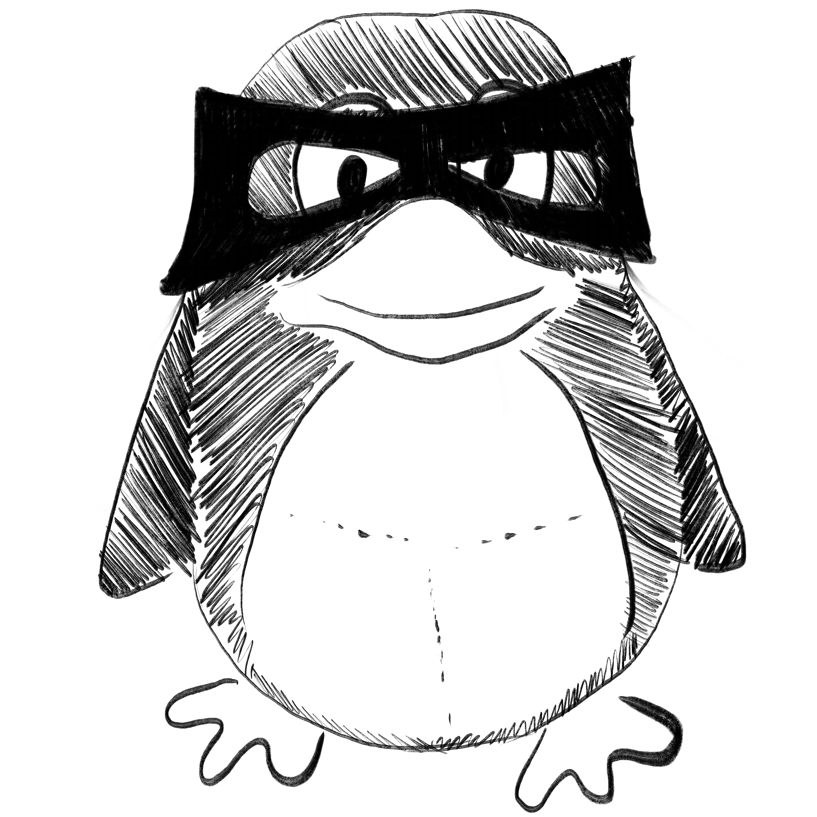
Prediction of designer-recombinases for DNA editing with generative deep learning.
In Nature communications ; h5-index 260.0
Schmitt Lukas Theo, Paszkowski-Rogacz Maciej, Jug Florian, Buchholz Frank
2022-Dec-27
Prevalence of Asymptomatic SARS-CoV-2 Infection in Japan.
In JAMA network open
IMPORTANCE :
OBJECTIVE :
DESIGN, SETTING, AND PARTICIPANTS :
MAIN OUTCOMES AND MEASURES :
RESULTS :
CONCLUSIONS AND RELEVANCE :
Suzuki Toru, Aizawa Kenichi, Shibuya Kenji, Yamanaka Shinya, Anzai Yuichiro, Kurokawa Kiyoshi, Nagai Ryozo
2022-Dec-01
Prevalence of Asymptomatic SARS-CoV-2 Infection in Japan.
In JAMA network open
IMPORTANCE :
OBJECTIVE :
DESIGN, SETTING, AND PARTICIPANTS :
MAIN OUTCOMES AND MEASURES :
RESULTS :
CONCLUSIONS AND RELEVANCE :
Suzuki Toru, Aizawa Kenichi, Shibuya Kenji, Yamanaka Shinya, Anzai Yuichiro, Kurokawa Kiyoshi, Nagai Ryozo
2022-Dec-01
Development and Validation of a Machine Learning Model to Estimate Risk of Adverse Outcomes Within 30 Days of Opioid Dispensation.
In JAMA network open
IMPORTANCE :
OBJECTIVE :
DESIGN, SETTING, AND PARTICIPANTS :
EXPOSURES :
MAIN OUTCOMES AND MEASURES :
RESULTS :
CONCLUSIONS AND RELEVANCE :
Sharma Vishal, Kulkarni Vinaykumar, Jess Ed, Gilani Fizza, Eurich Dean, Simpson Scot H, Voaklander Don, Semenchuk Michael, London Connor, Samanani Salim
2022-Dec-01
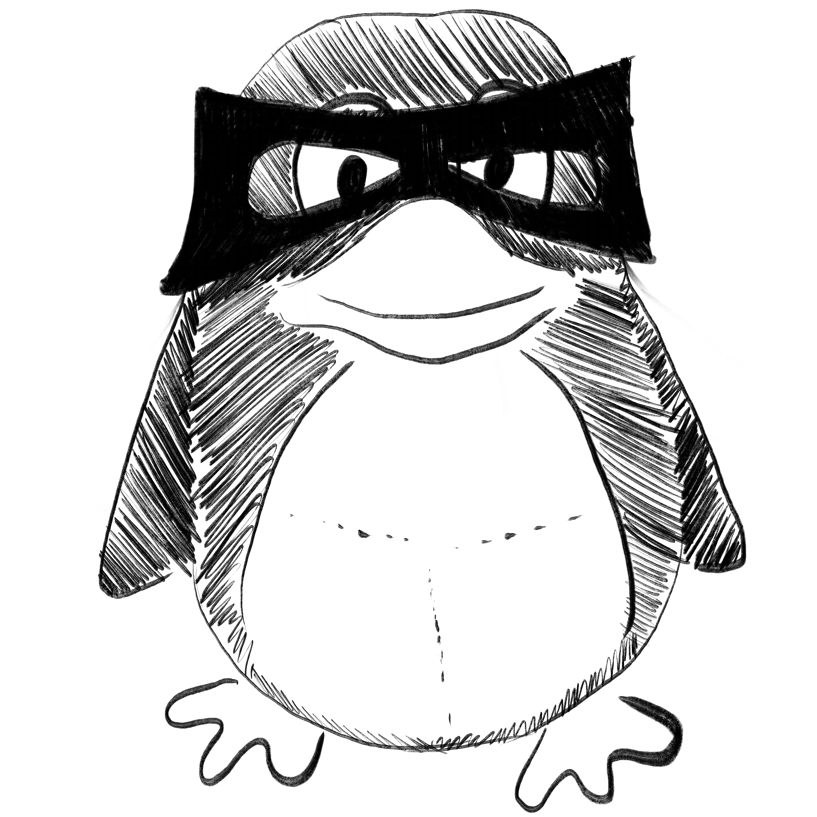
Physical deep learning with biologically inspired training method: gradient-free approach for physical hardware.
In Nature communications ; h5-index 260.0
Nakajima Mitsumasa, Inoue Katsuma, Tanaka Kenji, Kuniyoshi Yasuo, Hashimoto Toshikazu, Nakajima Kohei
2022-Dec-26
Accuracy and efficiency of an artificial intelligence-based pulmonary broncho-vascular three-dimensional reconstruction system supporting thoracic surgery: Retrospective and prospective validation study.
In EBioMedicine
BACKGROUND :
METHODS :
FINDINGS :
INTERPRETATION :
FUNDING :
Li Xiang, Zhang Shanyuan, Luo Xiang, Gao Guangming, Luo Xiangfeng, Wang Shansi, Li Shaolei, Zhao Dachuan, Wang Yaqi, Cui Xinrun, Liu Bing, Tao Ye, Xiao Bufan, Tang Lei, Yan Shi, Wu Nan
2022-Dec-22
Accuracy, Anatomy, Artificial intelligence, Efficiency, Safety, Three-dimensional reconstruction model
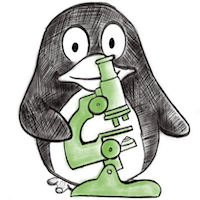
How, for whom, and in what contexts will artificial intelligence be adopted in pathology? A realist interview study.
In Journal of the American Medical Informatics Association : JAMIA
OBJECTIVE :
MATERIALS AND METHODS :
RESULTS :
CONCLUSIONS :
King Henry, Williams Bethany, Treanor Darren, Randell Rebecca
2022-Dec-24
artificial intelligence, implementation, pathology, qualitative research, realist evaluation
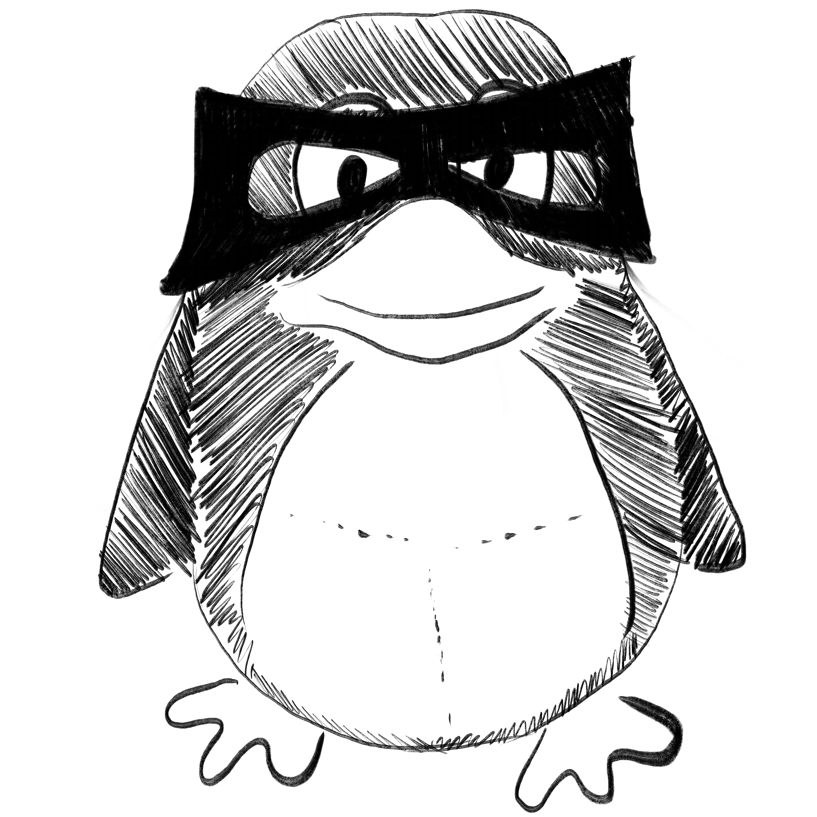
Sourcing thermotolerant poly(ethylene terephthalate) hydrolase scaffolds from natural diversity.
In Nature communications ; h5-index 260.0
Erickson Erika, Gado Japheth E, Avilán Luisana, Bratti Felicia, Brizendine Richard K, Cox Paul A, Gill Raj, Graham Rosie, Kim Dong-Jin, König Gerhard, Michener William E, Poudel Saroj, Ramirez Kelsey J, Shakespeare Thomas J, Zahn Michael, Boyd Eric S, Payne Christina M, DuBois Jennifer L, Pickford Andrew R, Beckham Gregg T, McGeehan John E
2022-Dec-21
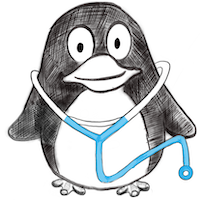
A deep learning approach to identify missing is-a relations in SNOMED CT.
In Journal of the American Medical Informatics Association : JAMIA
OBJECTIVE :
MATERIALS AND METHODS :
RESULTS :
CONCLUSIONS :
Abeysinghe Rashmie, Zheng Fengbo, Bernstam Elmer V, Shi Jay, Bodenreider Olivier, Cui Licong
2022-Dec-20
SNOMED CT, deep learning, missing is-a relations, ontology quality assurance
Inconsistent Partitioning and Unproductive Feature Associations Yield Idealized Radiomic Models.
In Radiology ; h5-index 91.0
Gidwani Mishka, Chang Ken, Patel Jay Biren, Hoebel Katharina Viktoria, Ahmed Syed Rakin, Singh Praveer, Fuller Clifton David, Kalpathy-Cramer Jayashree
2022-Dec-20
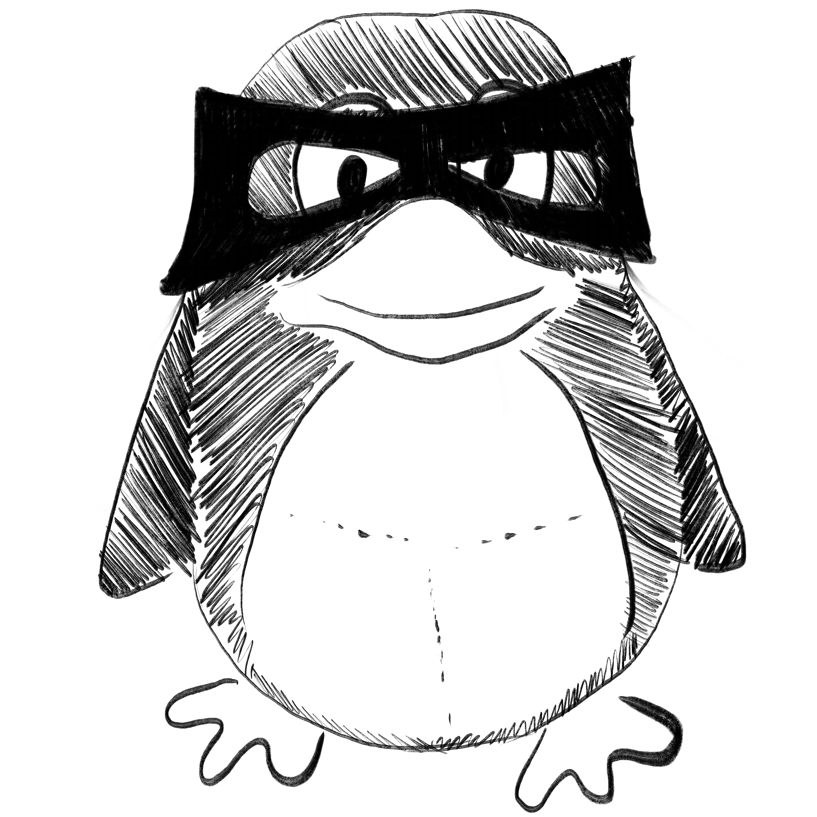
scDSSC: Deep Sparse Subspace Clustering for scRNA-seq Data.
In PLoS computational biology
Wang HaiYun, Zhao JianPing, Zheng ChunHou, Su YanSen
2022-Dec-19
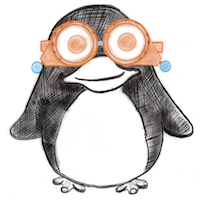
Optical coherence tomography imaging biomarkers associated with neovascular age-related macular degeneration: a systematic review.
In Eye (London, England) ; h5-index 41.0
UNLABELLED :
POPULATION :
SETTINGS :
STUDY DESIGNS :
SYSTEMATIC REVIEW REGISTRATION :
Hanson Rachel L W, Airody Archana, Sivaprasad Sobha, Gale Richard P
2022-Dec-16
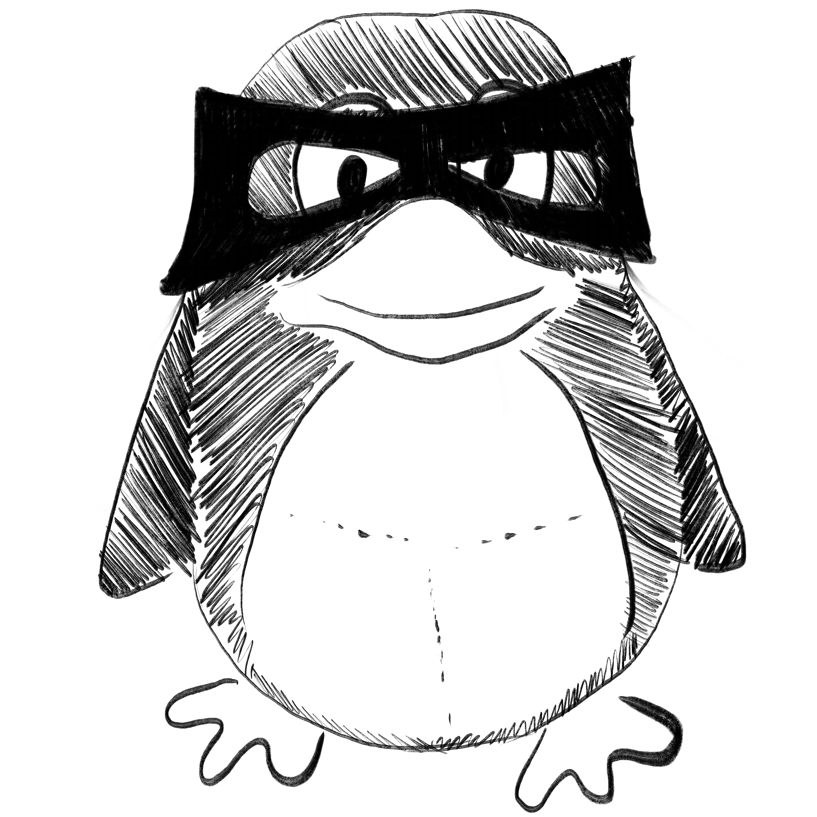
Deep embedding and alignment of protein sequences.
In Nature methods ; h5-index 152.0
Llinares-López Felipe, Berthet Quentin, Blondel Mathieu, Teboul Olivier, Vert Jean-Philippe
2022-Dec-15
Estimating diagnostic uncertainty in artificial intelligence assisted pathology using conformal prediction.
In Nature communications ; h5-index 260.0
Olsson Henrik, Kartasalo Kimmo, Mulliqi Nita, Capuccini Marco, Ruusuvuori Pekka, Samaratunga Hemamali, Delahunt Brett, Lindskog Cecilia, Janssen Emiel A M, Blilie Anders, Egevad Lars, Spjuth Ola, Eklund Martin
2022-Dec-15
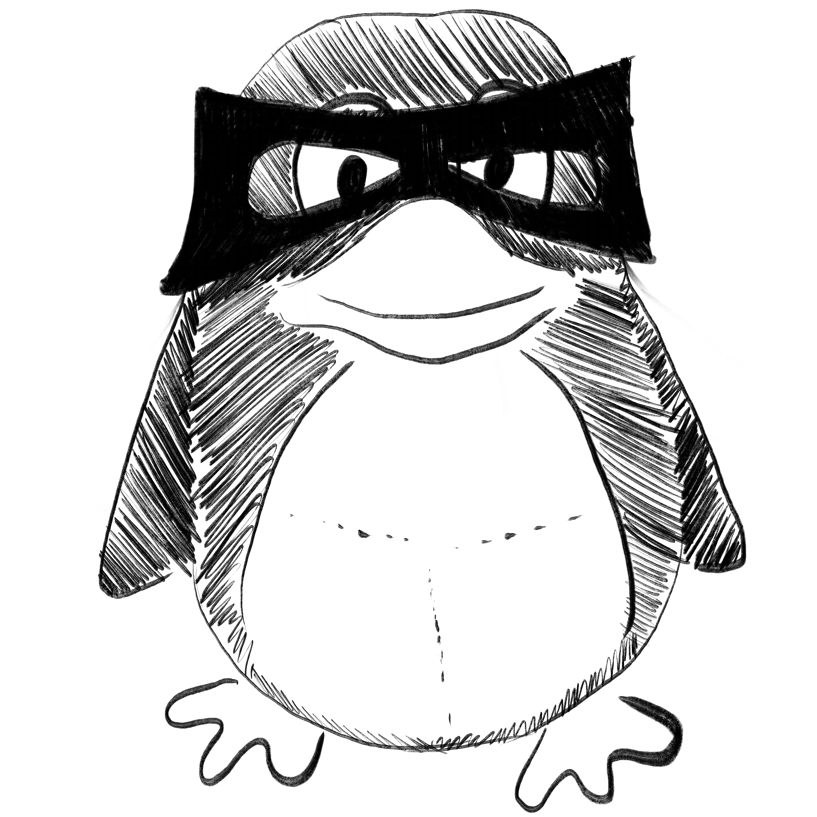
A unifying Bayesian framework for merging X-ray diffraction data.
In Nature communications ; h5-index 260.0
Dalton Kevin M, Greisman Jack B, Hekstra Doeke R
2022-Dec-15
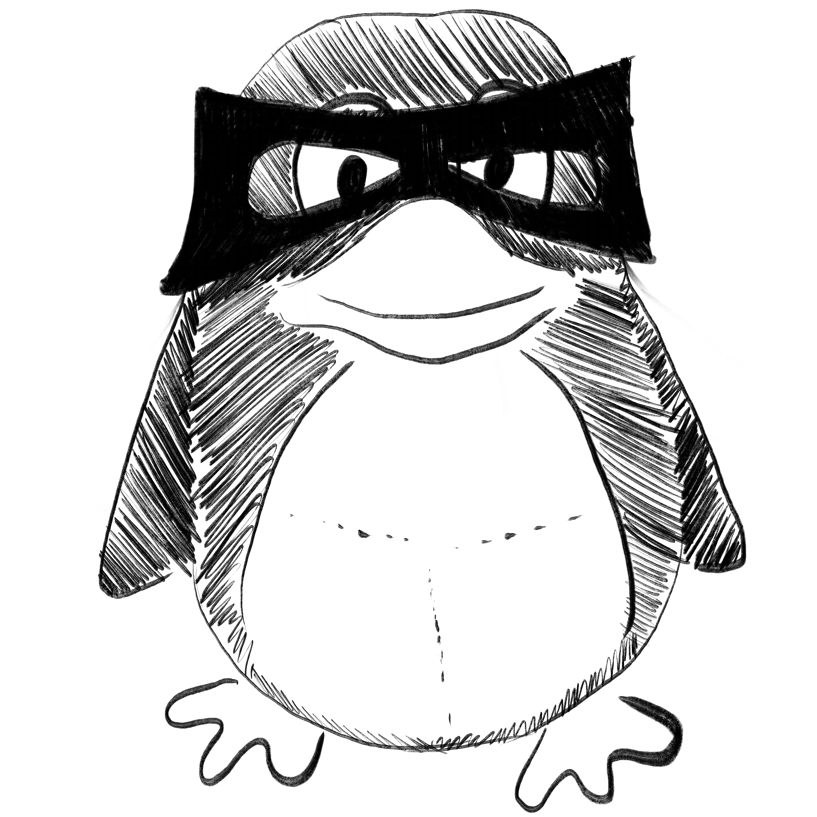
Recalibrating probabilistic forecasts of epidemics.
In PLoS computational biology
Rumack Aaron, Tibshirani Ryan J, Rosenfeld Roni
2022-Dec-15
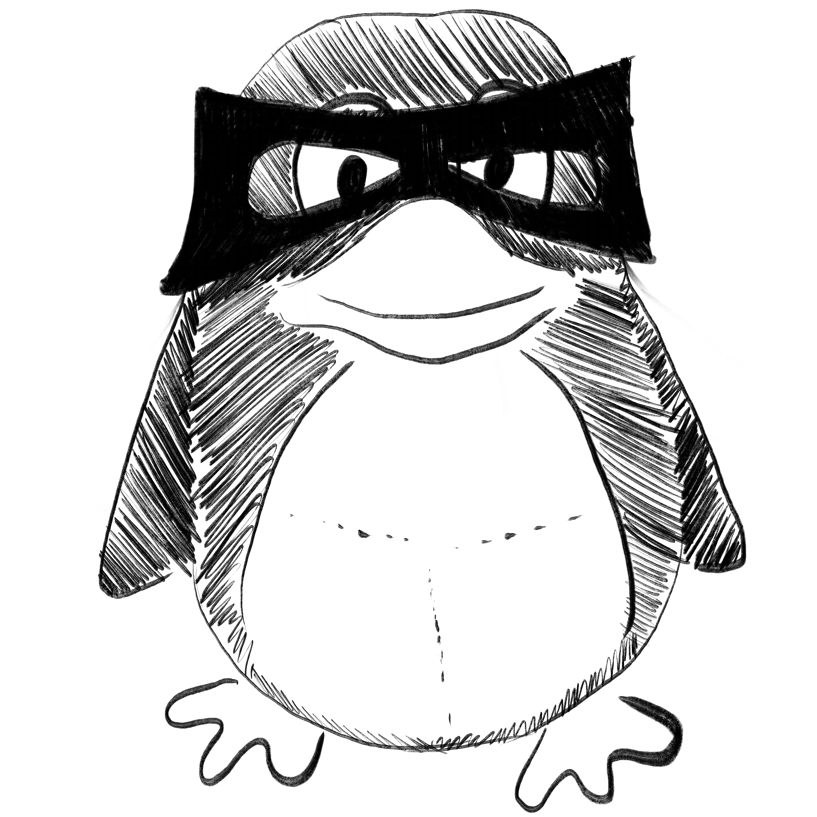
Genome-wide identification and characterization of DNA enhancers with a stacked multivariate fusion framework.
In PLoS computational biology
Wang Yansong, Hou Zilong, Yang Yuning, Wong Ka-Chun, Li Xiangtao
2022-Dec-15
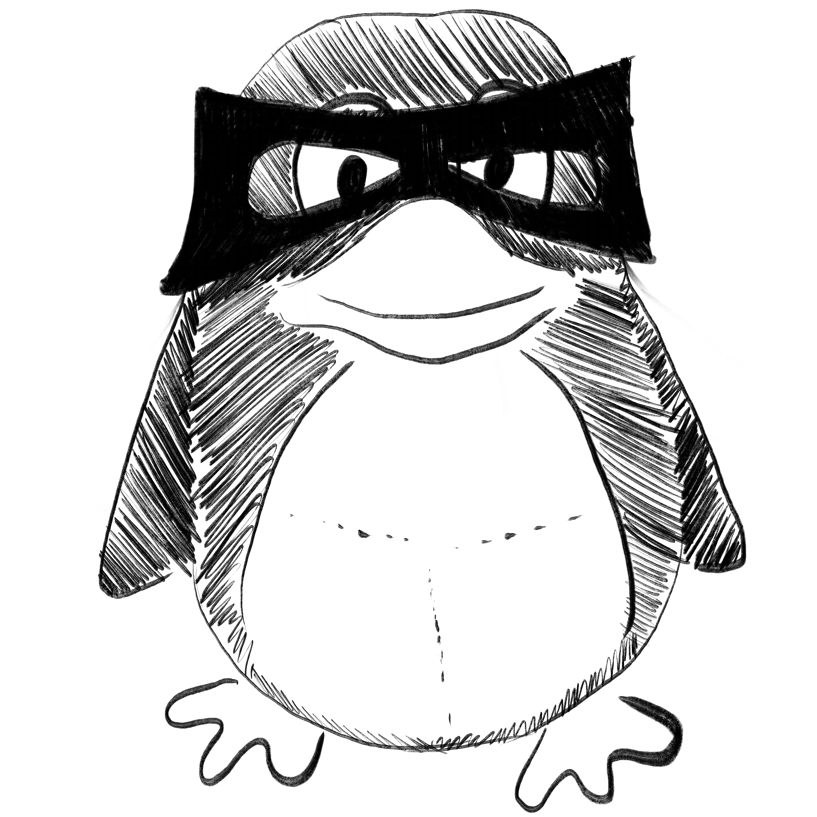
Eleven quick tips for data cleaning and feature engineering.
In PLoS computational biology
Chicco Davide, Oneto Luca, Tavazzi Erica
2022-Dec
Evaluation of an Artificial Intelligence Model for Detection of Pneumothorax and Tension Pneumothorax in Chest Radiographs.
In JAMA network open
IMPORTANCE :
OBJECTIVE :
DESIGN, SETTING, AND PARTICIPANTS :
MAIN OUTCOMES AND MEASURES :
RESULTS :
CONCLUSIONS AND RELEVANCE :
Hillis James M, Bizzo Bernardo C, Mercaldo Sarah, Chin John K, Newbury-Chaet Isabella, Digumarthy Subba R, Gilman Matthew D, Muse Victorine V, Bottrell Georgie, Seah Jarrel C Y, Jones Catherine M, Kalra Mannudeep K, Dreyer Keith J
2022-Dec-01
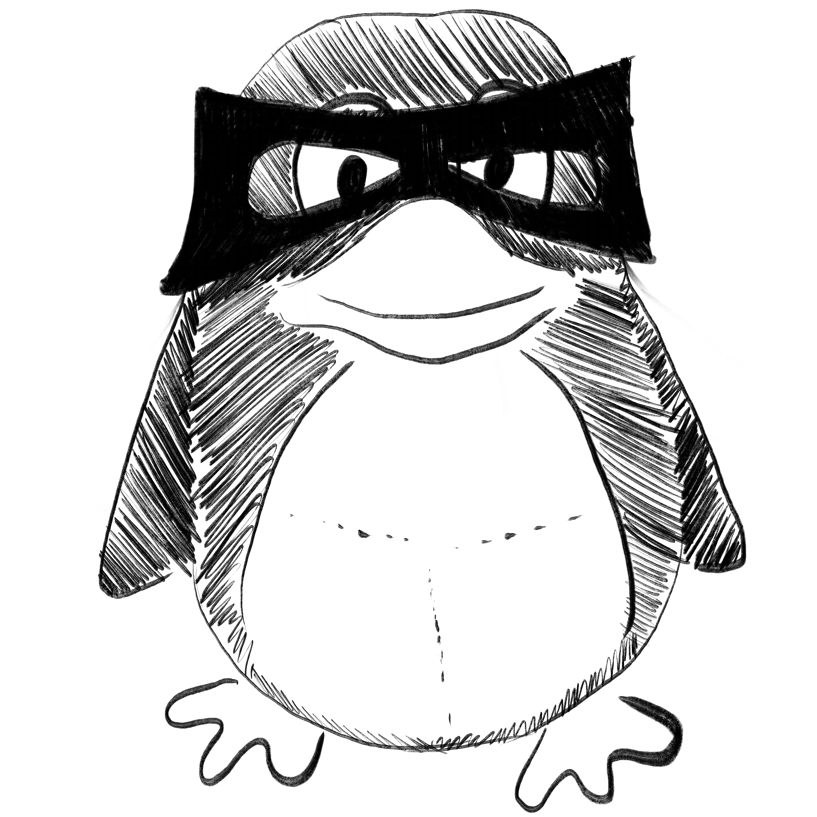
Accuracy and data efficiency in deep learning models of protein expression.
In Nature communications ; h5-index 260.0
Nikolados Evangelos-Marios, Wongprommoon Arin, Aodha Oisin Mac, Cambray Guillaume, Oyarzún Diego A
2022-Dec-15
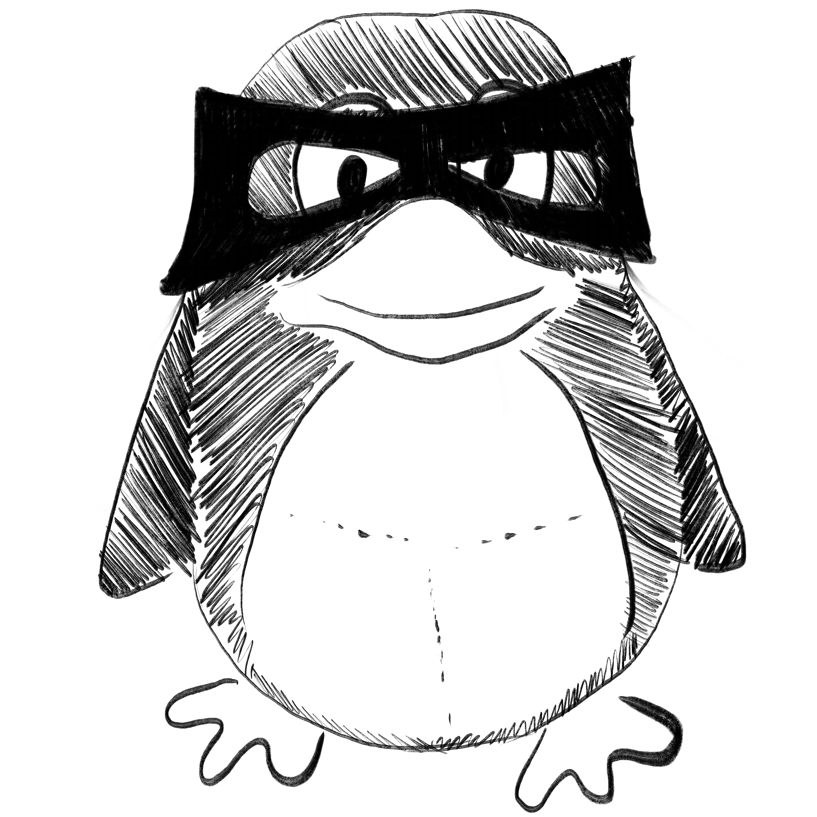
Interpreting tree ensemble machine learning models with endoR.
In PLoS computational biology
Ruaud Albane, Pfister Niklas, Ley Ruth E, Youngblut Nicholas D
2022-Dec-14
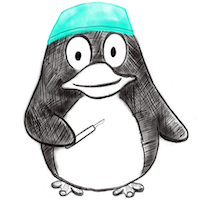
Development and Validation of a Prediction Model for Need for Massive Transfusion During Surgery Using Intraoperative Hemodynamic Monitoring Data.
In JAMA network open
IMPORTANCE :
OBJECTIVE :
DESIGN, SETTING, AND PARTICIPANTS :
EXPOSURES :
MAIN OUTCOMES AND MEASURES :
RESULTS :
CONCLUSIONS AND RELEVANCE :
Lee Seung Mi, Lee Garam, Kim Tae Kyong, Le Trang, Hao Jie, Jung Young Mi, Park Chan-Wook, Park Joong Shin, Jun Jong Kwan, Lee Hyung-Chul, Kim Dokyoon
2022-Dec-01
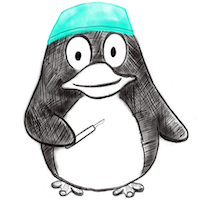
Factors Associated With Quality Care Among Adults With Rheumatoid Arthritis.
In JAMA network open
IMPORTANCE :
OBJECTIVE :
DESIGN, SETTING, AND PARTICIPANTS :
EXPOSURES :
MAIN OUTCOMES AND MEASURES :
RESULTS :
CONCLUSIONS AND RELEVANCE :
Seyferth Anne V, Cichocki Meghan N, Wang Chien-Wei, Huang Yun-Ju, Huang Yi-Wei, Chen Jung-Sheng, Kuo Chang-Fu, Chung Kevin C
2022-Dec-01
The development of an automatic speech recognition model using interview data from long-term care for older adults.
In Journal of the American Medical Informatics Association : JAMIA
OBJECTIVE :
MATERIALS AND METHODS :
RESULTS :
DISCUSSION :
CONCLUSIONS :
Hacking Coen, Verbeek Hilde, Hamers Jan P H, Aarts Sil
2022-Dec-10
artificial intelligence, automatic speech recognition, long-term care, nursing homes, older adults
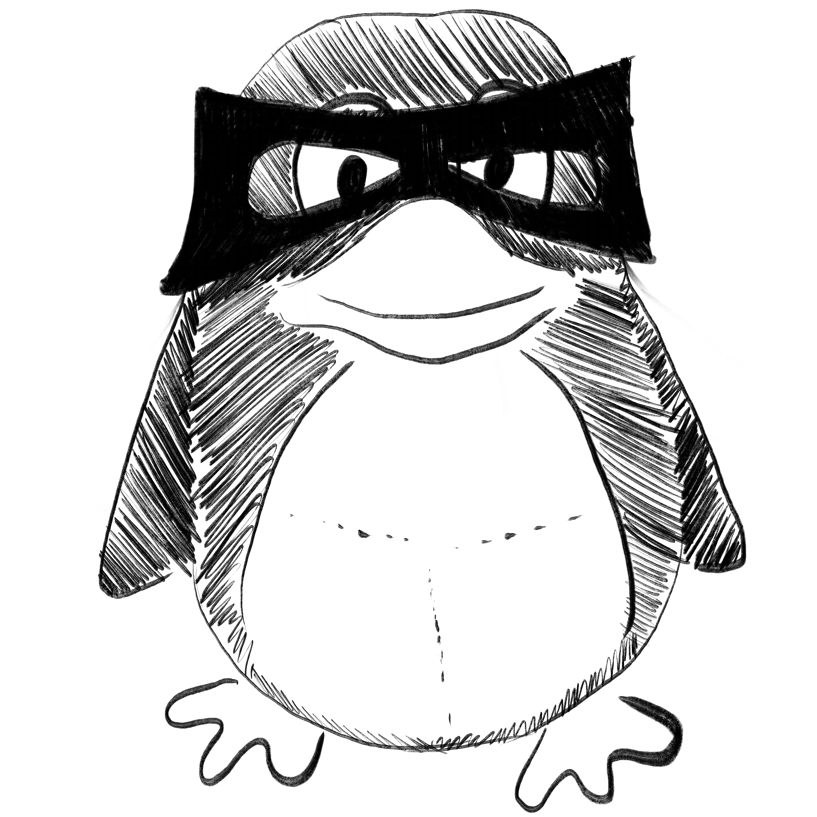
Limited conservation in cross-species comparison of GLK transcription factor binding suggested wide-spread cistrome divergence.
In Nature communications ; h5-index 260.0
Tu Xiaoyu, Ren Sibo, Shen Wei, Li Jianjian, Li Yuxiang, Li Chuanshun, Li Yangmeihui, Zong Zhanxiang, Xie Weibo, Grierson Donald, Fei Zhangjun, Giovannoni Jim, Li Pinghua, Zhong Silin
2022-Dec-09
The transcription factor DDIT3 is a potential driver of dyserythropoiesis in myelodysplastic syndromes.
In Nature communications ; h5-index 260.0
Berastegui Nerea, Ainciburu Marina, Romero Juan P, Garcia-Olloqui Paula, Alfonso-Pierola Ana, Philippe Céline, Vilas-Zornoza Amaia, San Martin-Uriz Patxi, Ruiz-Hernández Raquel, Abarrategi Ander, Ordoñez Raquel, Alignani Diego, Sarvide Sarai, Castro-Labrador Laura, Lamo-Espinosa José M, San-Julian Mikel, Jimenez Tamara, López-Cadenas Félix, Muntion Sandra, Sanchez-Guijo Fermin, Molero Antonieta, Montoro Maria Julia, Tazón Bárbara, Serrano Guillermo, Diaz-Mazkiaran Aintzane, Hernaez Mikel, Huerga Sofía, Bewicke-Copley Findlay, Rio-Machin Ana, Maurano Matthew T, Díez-Campelo María, Valcarcel David, Rouault-Pierre Kevin, Lara-Astiaso David, Ezponda Teresa, Prosper Felipe
2022-Dec-09
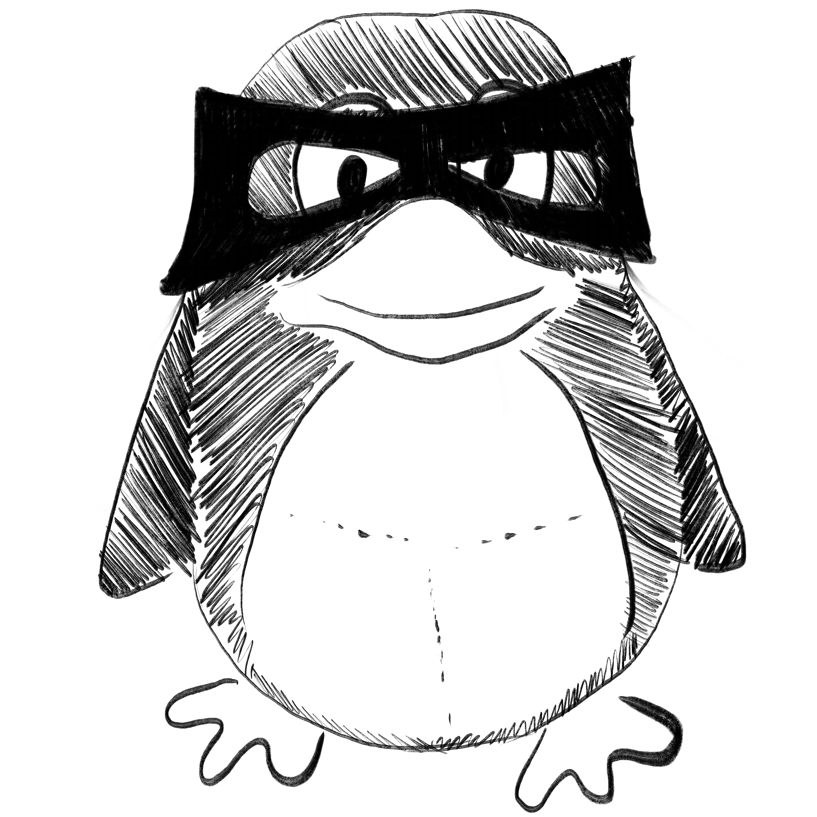
pGlycoQuant with a deep residual network for quantitative glycoproteomics at intact glycopeptide level.
In Nature communications ; h5-index 260.0
Kong Siyuan, Gong Pengyun, Zeng Wen-Feng, Jiang Biyun, Hou Xinhang, Zhang Yang, Zhao Huanhuan, Liu Mingqi, Yan Guoquan, Zhou Xinwen, Qiao Xihua, Wu Mengxi, Yang Pengyuan, Liu Chao, Cao Weiqian
2022-Dec-07
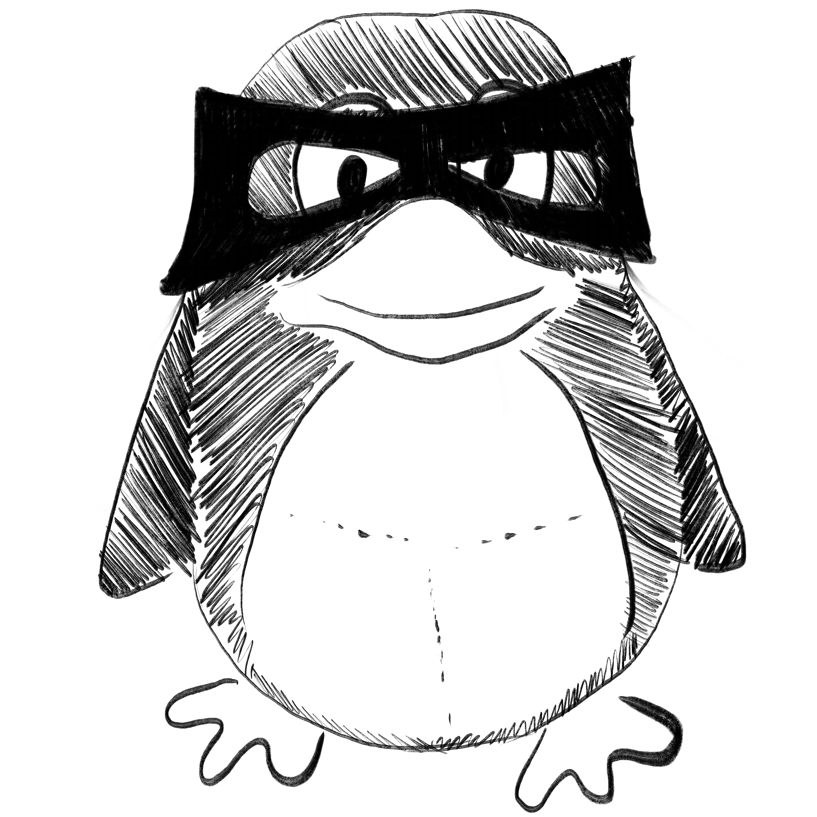
Direct retrieval of Zernike-based pupil functions using integrated diffractive deep neural networks.
In Nature communications ; h5-index 260.0
Goi Elena, Schoenhardt Steffen, Gu Min
2022-Dec-07
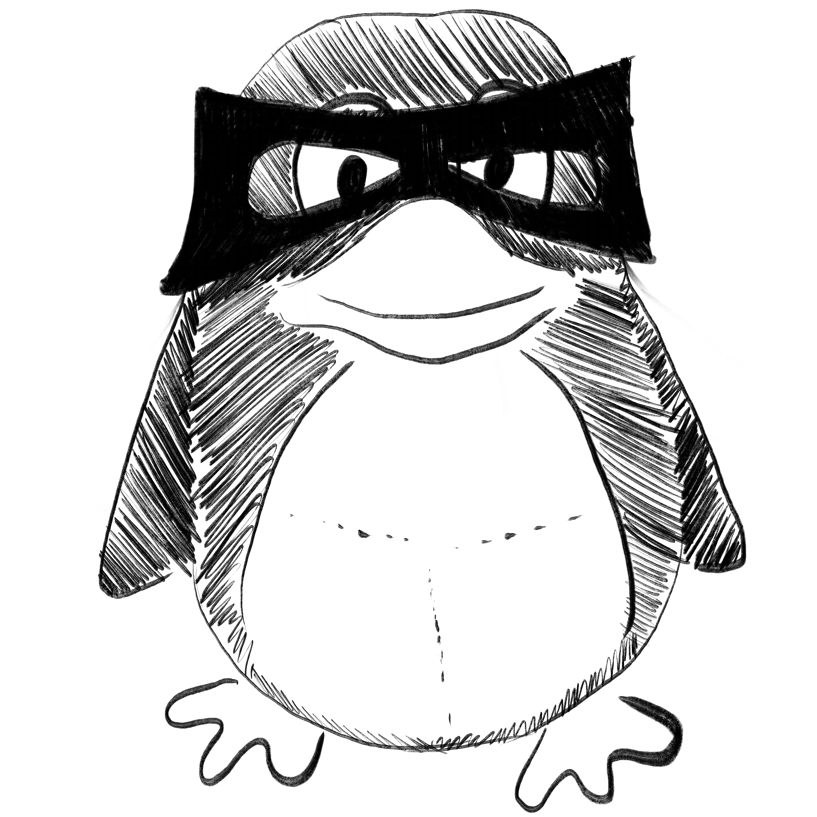
Negotiation and honesty in artificial intelligence methods for the board game of Diplomacy.
In Nature communications ; h5-index 260.0
Kramár János, Eccles Tom, Gemp Ian, Tacchetti Andrea, McKee Kevin R, Malinowski Mateusz, Graepel Thore, Bachrach Yoram
2022-Dec-06
Emerging and Evolving Concepts in Cancer Immunotherapy Imaging.
In Radiology ; h5-index 91.0
Dercle Laurent, Sun Shawn, Seban Romain-David, Mekki Ahmed, Sun Roger, Tselikas Lambros, Hans Sophie, Bernard-Tessier Alice, Mihoubi Bouvier Fadila, Aide Nicolas, Vercellino Laetitia, Rivas Alexia, Girard Antoine, Mokrane Fatima-Zohra, Manson Guillaume, Houot Roch, Lopci Egesta, Yeh Randy, Ammari Samy, Schwartz Lawrence H
2022-Dec-06
Predicting Hypoperfusion Lesion and Target Mismatch in Stroke from Diffusion-weighted MRI Using Deep Learning.
In Radiology ; h5-index 91.0
Yu Yannan, Christensen Soren, Ouyang Jiahong, Scalzo Fabien, Liebeskind David S, Lansberg Maarten G, Albers Gregory W, Zaharchuk Greg
2022-Dec-06
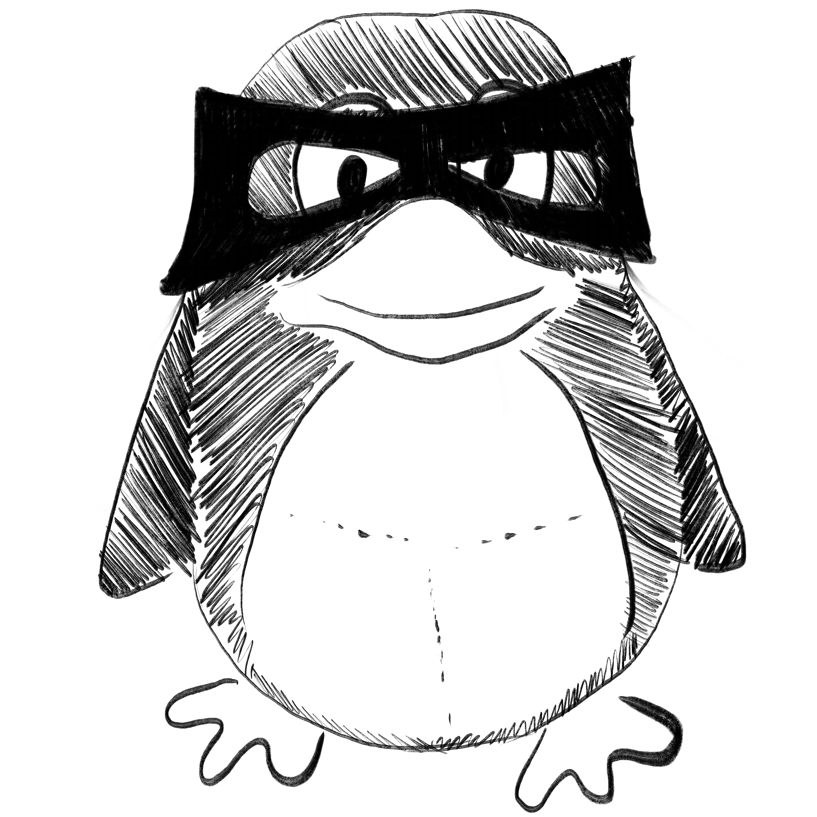
POPDx: an automated framework for patient phenotyping across 392 246 individuals in the UK Biobank study.
In Journal of the American Medical Informatics Association : JAMIA
OBJECTIVE :
MATERIALS AND METHODS :
RESULTS :
CONCLUSIONS :
Yang Lu, Wang Sheng, Altman Russ B
2022-Dec-05
AI in healthcare, UK Biobank, deep learning, machine learning, patient phenotyping, rare disease
Secuer: Ultrafast, scalable and accurate clustering of single-cell RNA-seq data.
In PLoS computational biology
Wei Nana, Nie Yating, Liu Lin, Zheng Xiaoqi, Wu Hua-Jun
2022-Dec-05
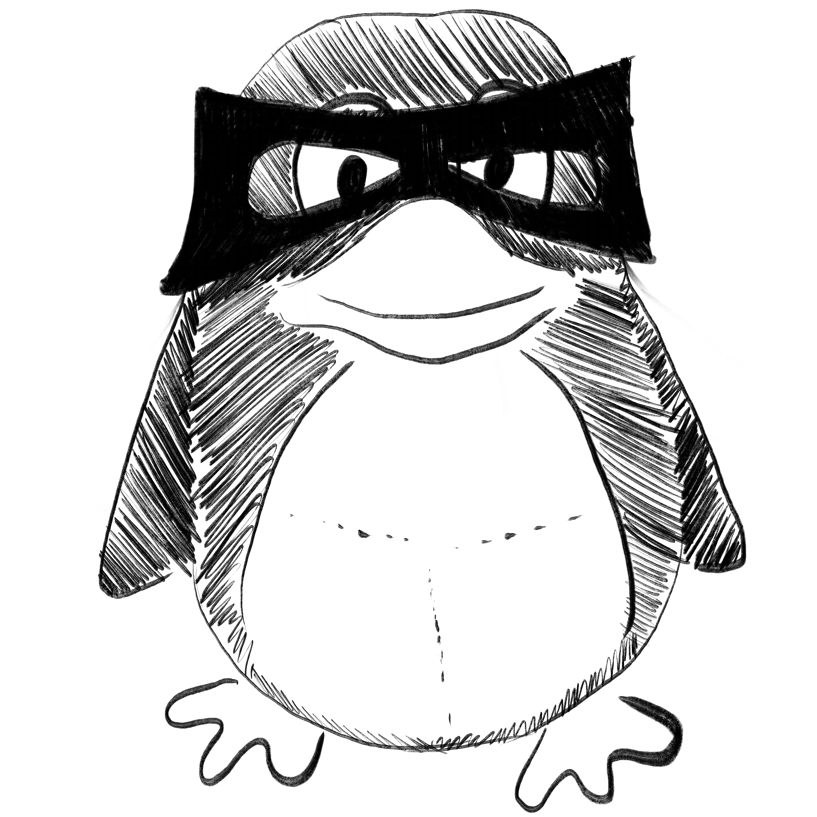
Regional mutational signature activities in cancer genomes.
In PLoS computational biology
Timmons Caitlin, Morris Quaid, Harrigan Caitlin F
2022-Dec-05
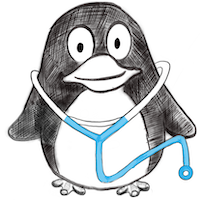
Prediction of type 2 diabetes using genome-wide polygenic risk score and metabolic profiles: A machine learning analysis of population-based 10-year prospective cohort study.
In EBioMedicine
BACKGROUND :
METHODS :
FINDINGS :
INTERPRETATION :
FUNDING :
Hahn Seok-Ju, Kim Suhyeon, Choi Young Sik, Lee Junghye, Kang Jihun
2022-Nov-30
East Asian, Genome-wide polygenic risk score, KoGES, Machine learning, Serum metabolites, Type 2 diabetes
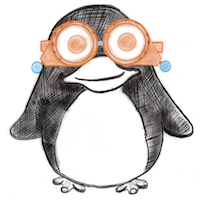
Transforming ophthalmology in the digital century-new care models with added value for patients.
In Eye (London, England) ; h5-index 41.0
Faes Livia, Maloca Peter M, Hatz Katja, Wolfensberger Thomas J, Munk Marion R, Sim Dawn A, Bachmann Lucas M, Schmid Martin K
2022-Dec-03
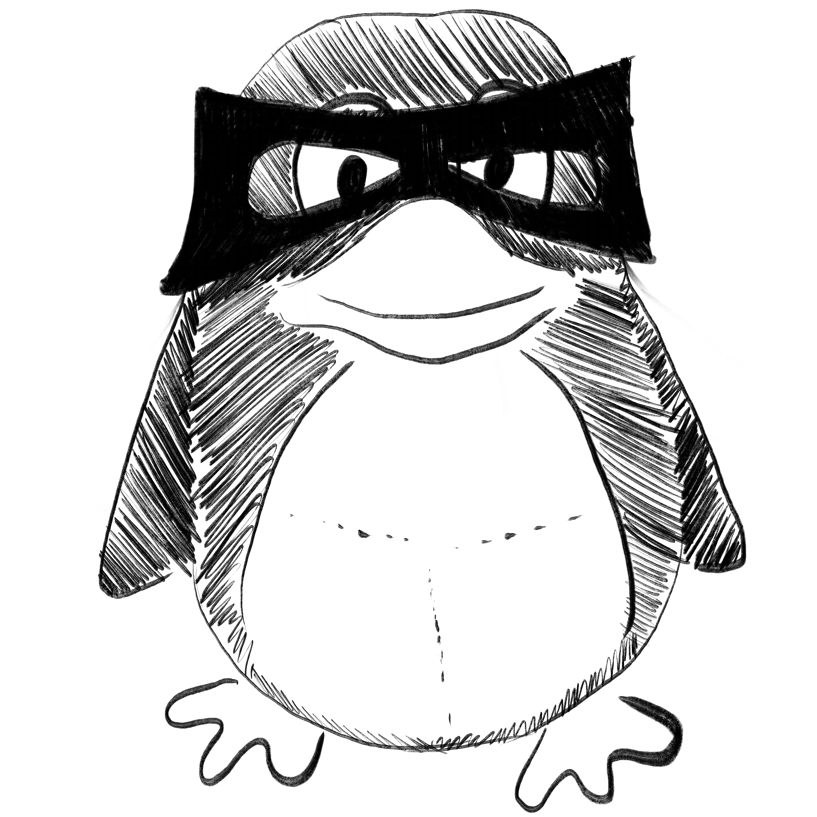
Reference panel guided topological structure annotation of Hi-C data.
In Nature communications ; h5-index 260.0
Zhang Yanlin, Blanchette Mathieu
2022-Dec-02
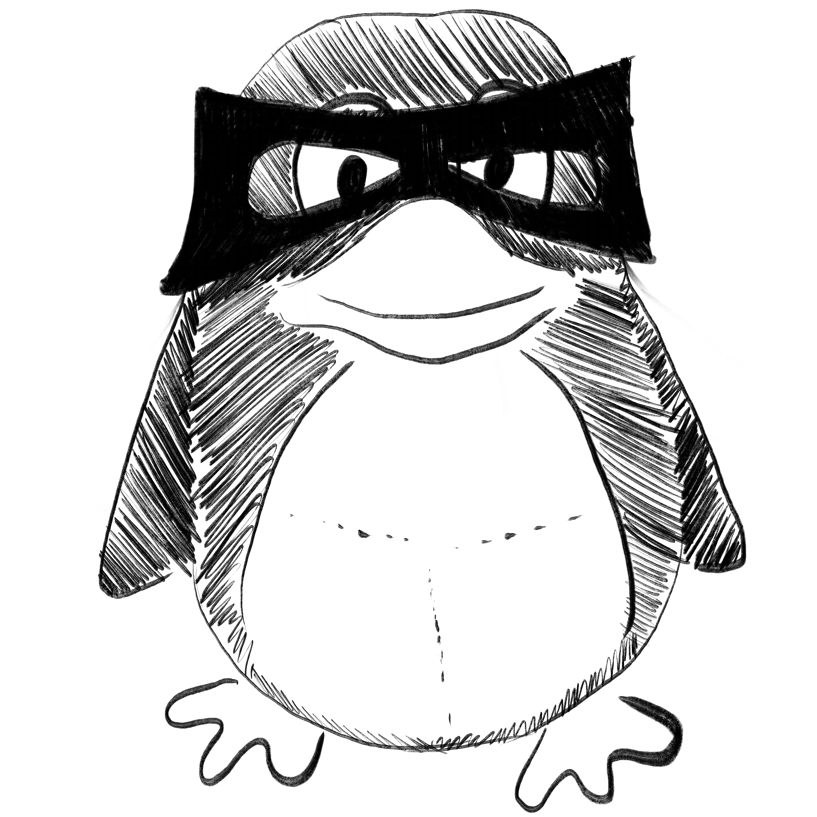
Deciphering clinical abbreviations with a privacy protecting machine learning system.
In Nature communications ; h5-index 260.0
Rajkomar Alvin, Loreaux Eric, Liu Yuchen, Kemp Jonas, Li Benny, Chen Ming-Jun, Zhang Yi, Mohiuddin Afroz, Gottweis Juraj
2022-Dec-02
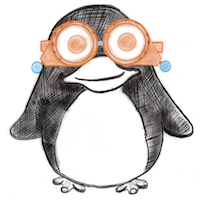
Transforming ophthalmology in the digital century-new care models with added value for patients.
In Eye (London, England) ; h5-index 41.0
Faes Livia, Maloca Peter M, Hatz Katja, Wolfensberger Thomas J, Munk Marion R, Sim Dawn A, Bachmann Lucas M, Schmid Martin K
2022-Dec-03
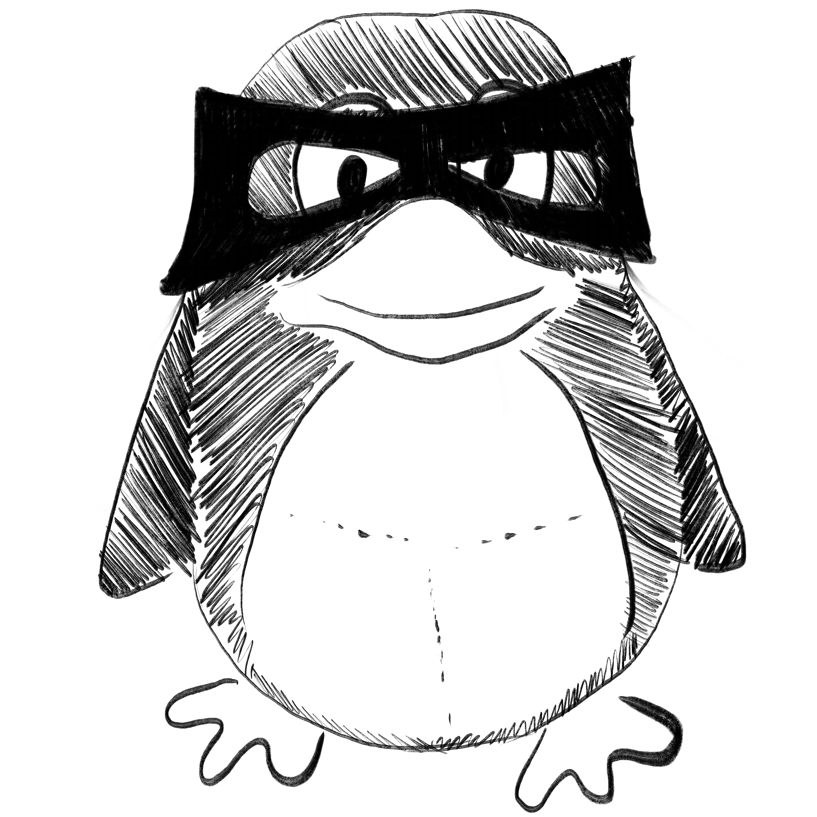
Using artificial intelligence to improve pain assessment and pain management: a scoping review.
In Journal of the American Medical Informatics Association : JAMIA
CONTEXT :
OBJECTIVES :
METHODS :
RESULTS :
CONCLUSIONS :
Zhang Meina, Zhu Linzee, Lin Shih-Yin, Herr Keela, Chi Chih-Lin, Demir Ibrahim, Dunn Lopez Karen, Chi Nai-Ching
2022-Dec-02
artificial intelligence, pain, pain assessment, pain control, pain management
Data-driven identification of post-acute SARS-CoV-2 infection subphenotypes.
In Nature medicine ; h5-index 170.0
Zhang Hao, Zang Chengxi, Xu Zhenxing, Zhang Yongkang, Xu Jie, Bian Jiang, Morozyuk Dmitry, Khullar Dhruv, Zhang Yiye, Nordvig Anna S, Schenck Edward J, Shenkman Elizabeth A, Rothman Russell L, Block Jason P, Lyman Kristin, Weiner Mark G, Carton Thomas W, Wang Fei, Kaushal Rainu
2022-Dec-01
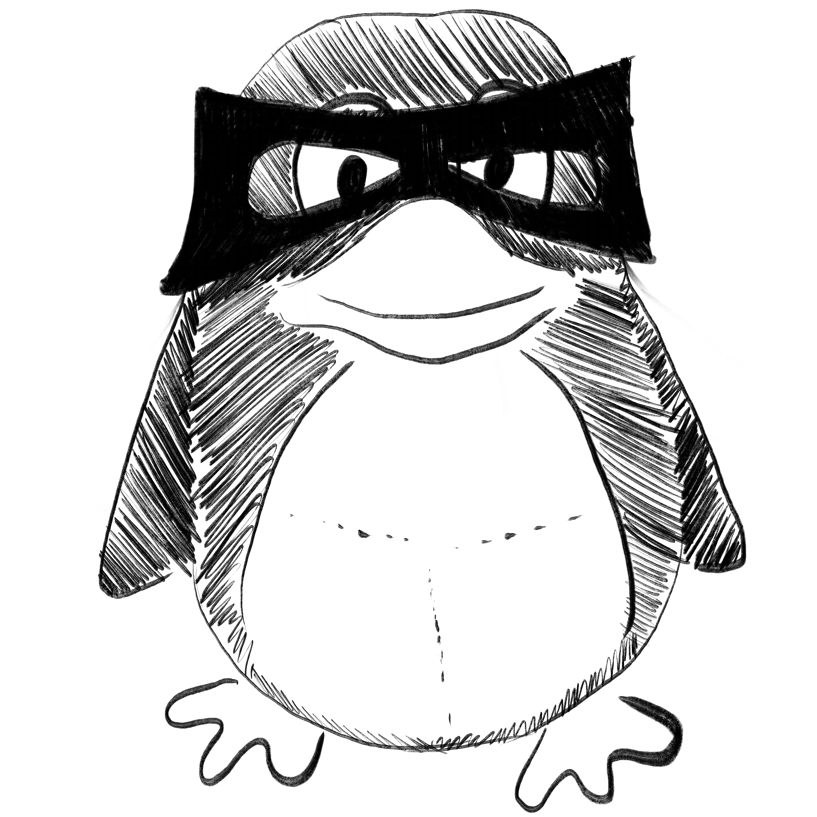
An integrated resource for functional and structural connectivity of the marmoset brain.
In Nature communications ; h5-index 260.0
Tian Xiaoguang, Chen Yuyan, Majka Piotr, Szczupak Diego, Perl Yonatan Sanz, Yen Cecil Chern-Chyi, Tong Chuanjun, Feng Furui, Jiang Haiteng, Glen Daniel, Deco Gustavo, Rosa Marcello G P, Silva Afonso C, Liang Zhifeng, Liu Cirong
2022-Dec-01
Data-driven identification of post-acute SARS-CoV-2 infection subphenotypes.
In Nature medicine ; h5-index 170.0
Zhang Hao, Zang Chengxi, Xu Zhenxing, Zhang Yongkang, Xu Jie, Bian Jiang, Morozyuk Dmitry, Khullar Dhruv, Zhang Yiye, Nordvig Anna S, Schenck Edward J, Shenkman Elizabeth A, Rothman Russell L, Block Jason P, Lyman Kristin, Weiner Mark G, Carton Thomas W, Wang Fei, Kaushal Rainu
2022-Dec-01
Ten quick tips for sequence-based prediction of protein properties using machine learning.
In PLoS computational biology
Hou Qingzhen, Waury Katharina, Gogishvili Dea, Feenstra K Anton
2022-Dec
A comparative study of pretrained language models for long clinical text.
In Journal of the American Medical Informatics Association : JAMIA
OBJECTIVE :
MATERIALS AND METHODS :
RESULTS :
DISCUSSION :
CONCLUSION :
Li Yikuan, Wehbe Ramsey M, Ahmad Faraz S, Wang Hanyin, Luo Yuan
2022-Nov-30
clinical natural language processing, named entity recognition, natural language inference, question answering, text classification
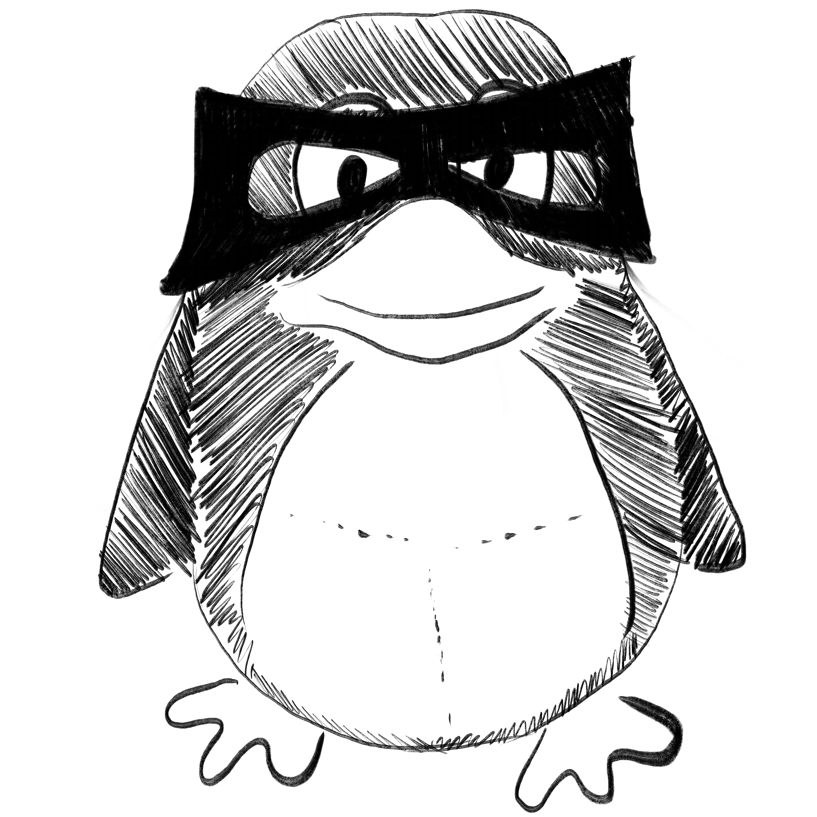
Dendrocentric learning for synthetic intelligence.
In Nature ; h5-index 368.0
Boahen Kwabena
2022-Dec
Analysis of the first genetic engineering attribution challenge.
In Nature communications ; h5-index 260.0
Crook Oliver M, Warmbrod Kelsey Lane, Lipstein Greg, Chung Christine, Bakerlee Christopher W, McKelvey T Greg, Holland Shelly R, Swett Jacob L, Esvelt Kevin M, Alley Ethan C, Bradshaw William J
2022-Nov-30
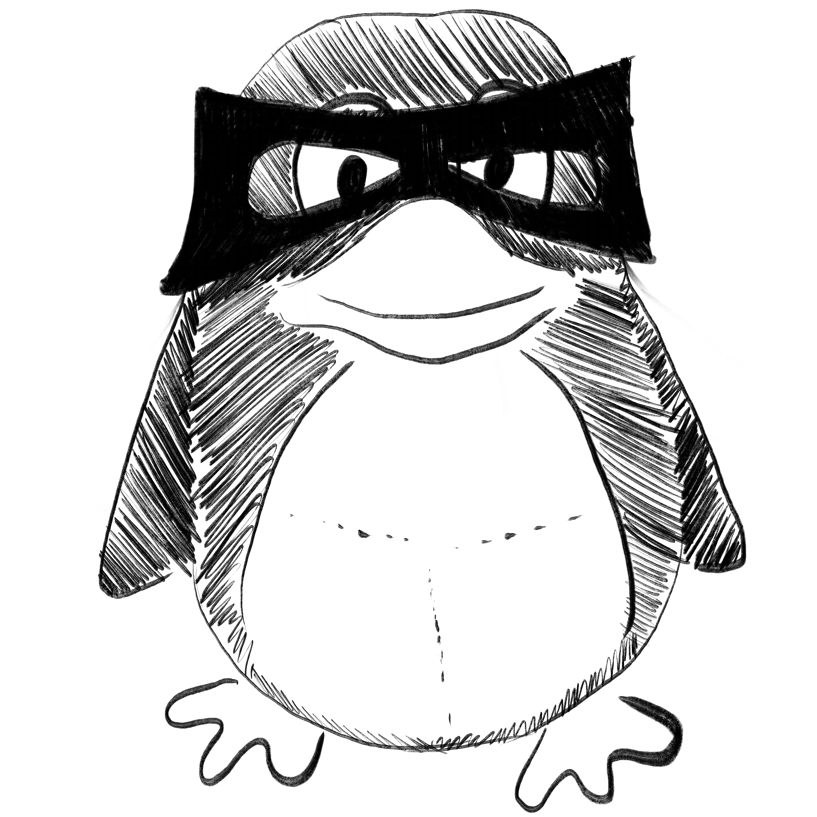
The E3 ubiquitin ligase WWP2 regulates pro-fibrogenic monocyte infiltration and activity in heart fibrosis.
In Nature communications ; h5-index 260.0
Chen Huimei, Chew Gabriel, Devapragash Nithya, Loh Jui Zhi, Huang Kevin Y, Guo Jing, Liu Shiyang, Tan Elisabeth Li Sa, Chen Shuang, Tee Nicole Gui Zhen, Mia Masum M, Singh Manvendra K, Zhang Aihua, Behmoaras Jacques, Petretto Enrico
2022-Nov-30
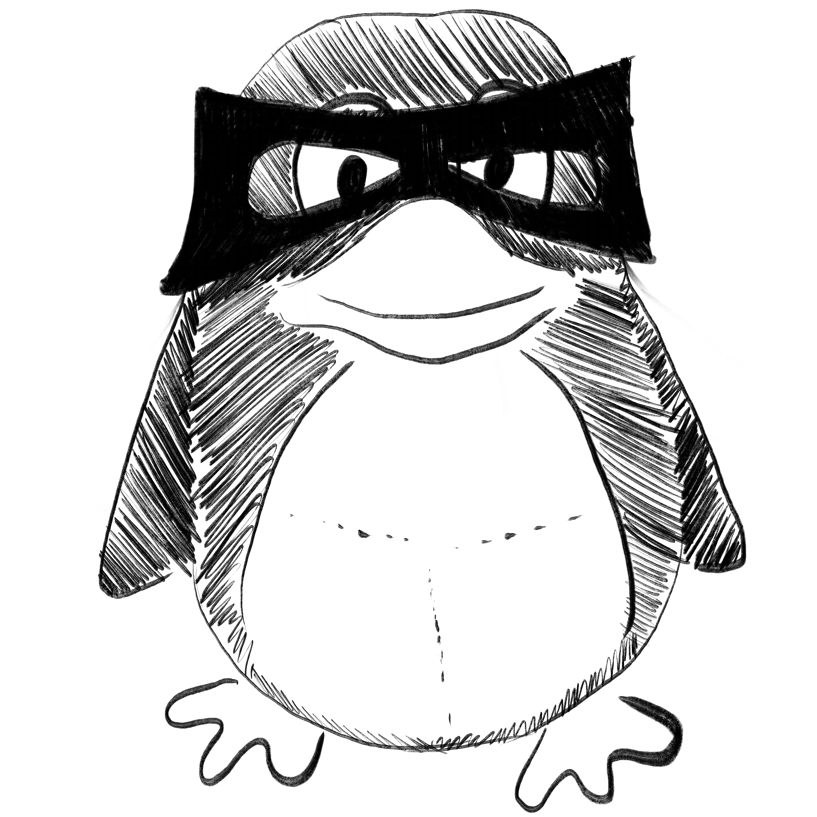
Chronic stress causes striatal disinhibition mediated by SOM-interneurons in male mice.
In Nature communications ; h5-index 260.0
Rodrigues Diana, Jacinto Luis, Falcão Margarida, Castro Ana Carolina, Cruz Alexandra, Santa Cátia, Manadas Bruno, Marques Fernanda, Sousa Nuno, Monteiro Patricia
2022-Nov-29
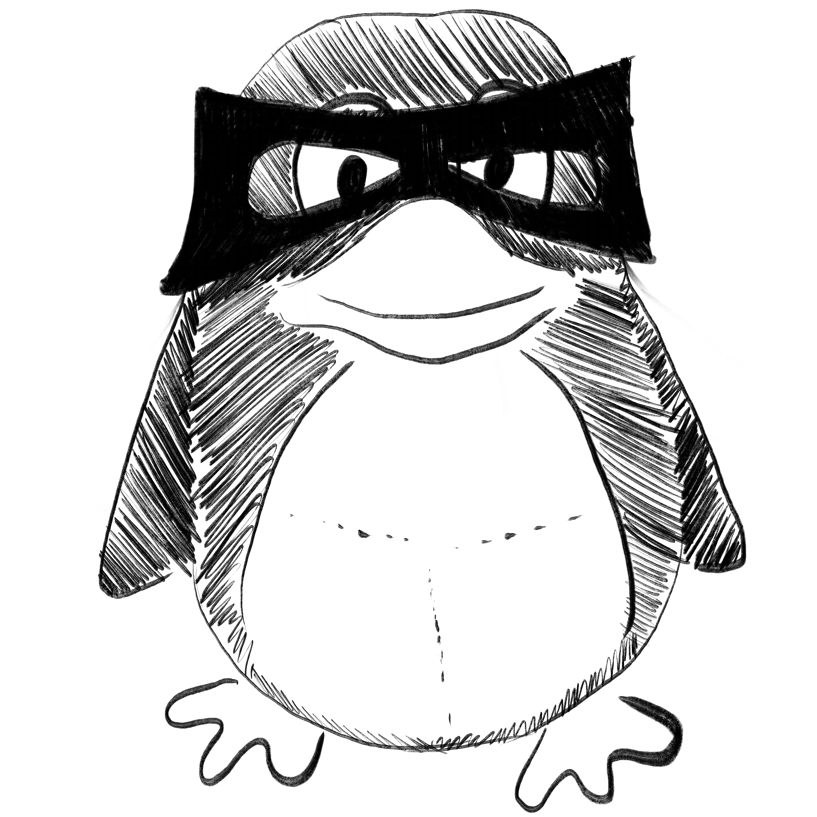
A large-scale neural network training framework for generalized estimation of single-trial population dynamics.
In Nature methods ; h5-index 152.0
Keshtkaran Mohammad Reza, Sedler Andrew R, Chowdhury Raeed H, Tandon Raghav, Basrai Diya, Nguyen Sarah L, Sohn Hansem, Jazayeri Mehrdad, Miller Lee E, Pandarinath Chethan
2022-Nov-28
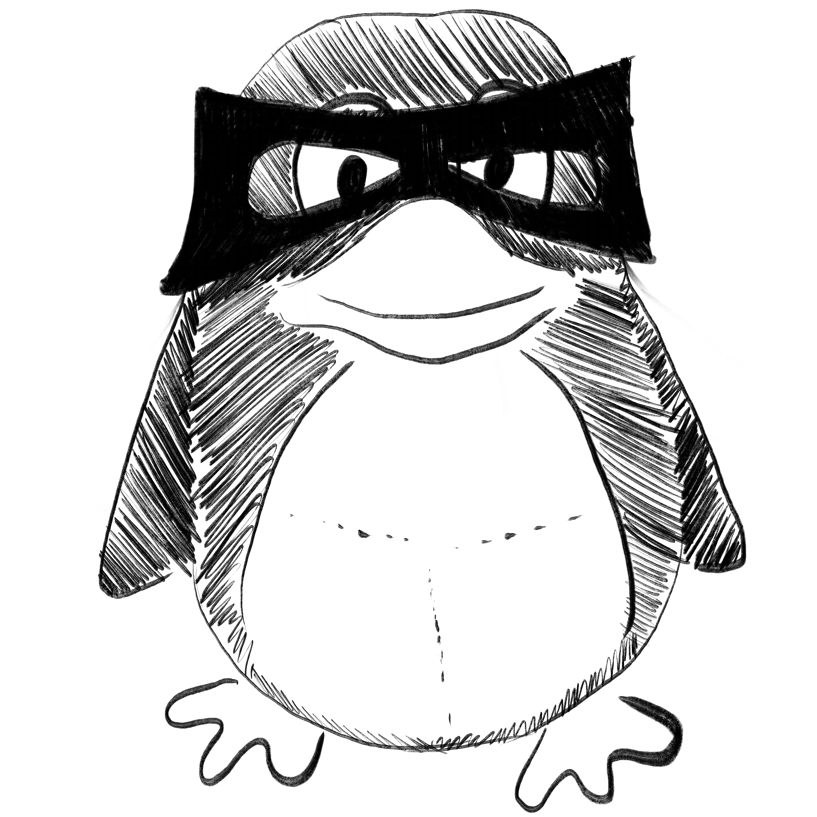
AlphaFill: enriching AlphaFold models with ligands and cofactors.
In Nature methods ; h5-index 152.0
Hekkelman Maarten L, de Vries Ida, Joosten Robbie P, Perrakis Anastassis
2022-Nov-24
Automated deidentification of radiology reports combining transformer and "hide in plain sight" rule-based methods.
In Journal of the American Medical Informatics Association : JAMIA
OBJECTIVE :
MATERIALS AND METHODS :
RESULTS :
DISCUSSION :
CONCLUSIONS :
Chambon Pierre J, Wu Christopher, Steinkamp Jackson M, Adleberg Jason, Cook Tessa S, Langlotz Curtis P
2022-Nov-23
NLP, deidentification, machine learning, radiology, transformer
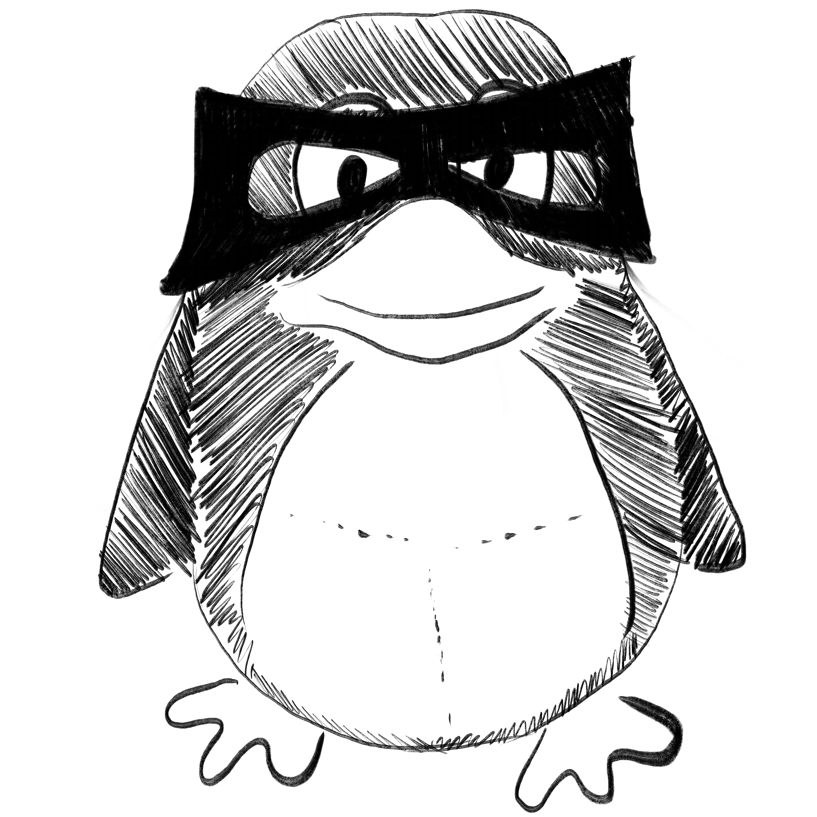
DeepPROTACs is a deep learning-based targeted degradation predictor for PROTACs.
In Nature communications ; h5-index 260.0
Li Fenglei, Hu Qiaoyu, Zhang Xianglei, Sun Renhong, Liu Zhuanghua, Wu Sanan, Tian Siyuan, Ma Xinyue, Dai Zhizhuo, Yang Xiaobao, Gao Shenghua, Bai Fang
2022-Nov-21
Emerging Technology in Musculoskeletal MRI and CT.
In Radiology ; h5-index 91.0
Kijowski Richard, Fritz Jan
2022-Nov-22
χ-Separation Imaging for Diagnosis of Multiple Sclerosis versus Neuromyelitis Optica Spectrum Disorder.
In Radiology ; h5-index 91.0
Kim Woojun, Shin Hyeong-Geol, Lee Hyebin, Park Dohoon, Kang Junghwa, Nam Yoonho, Lee Jongho, Jang Jinhee
2022-Nov-22
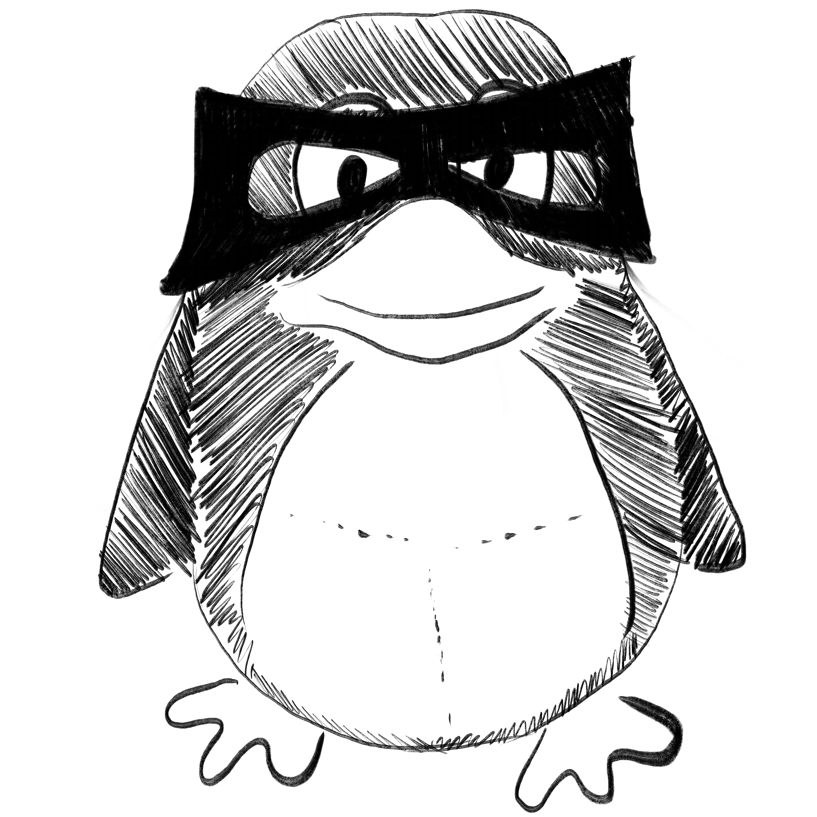
Machine learning approaches for electronic health records phenotyping: a methodical review.
In Journal of the American Medical Informatics Association : JAMIA
OBJECTIVE :
MATERIALS AND METHODS :
RESULTS :
DISCUSSION :
CONCLUSION :
Yang Siyue, Varghese Paul, Stephenson Ellen, Tu Karen, Gronsbell Jessica
2022-Nov-22
cohort identification, electronic health records, machine learning, phenotyping
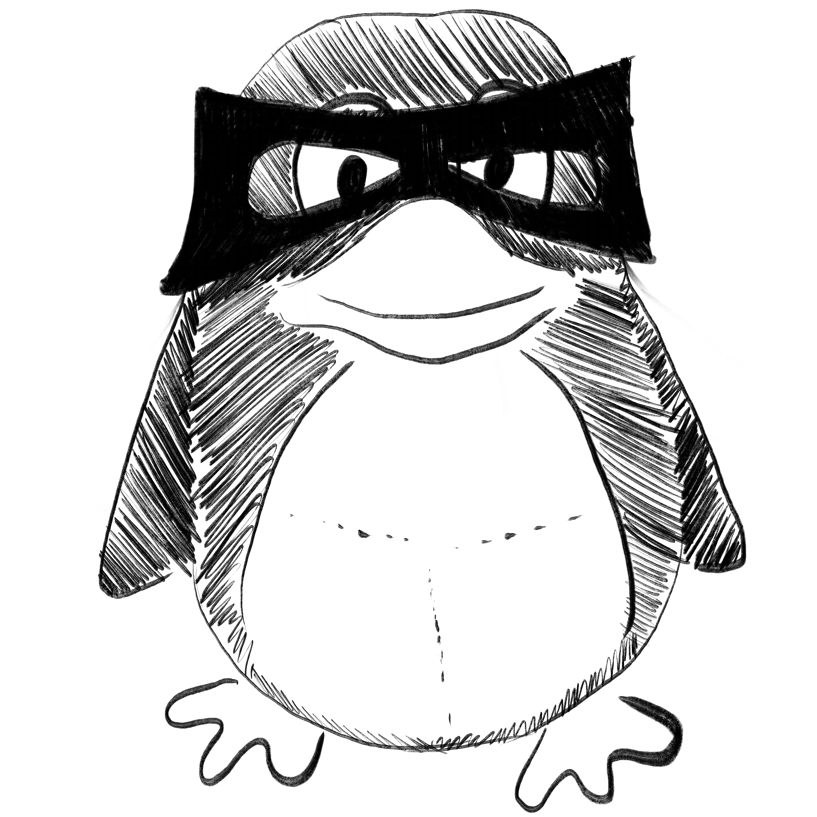
Performance drift in a mortality prediction algorithm among patients with cancer during the SARS-CoV-2 pandemic.
In Journal of the American Medical Informatics Association : JAMIA
Parikh Ravi B, Zhang Yichen, Kolla Likhitha, Chivers Corey, Courtright Katherine R, Zhu Jingsan, Navathe Amol S, Chen Jinbo
2022-Nov-21
SARS-CoV-2, algorithm drift, cancer, machine learning, mortality
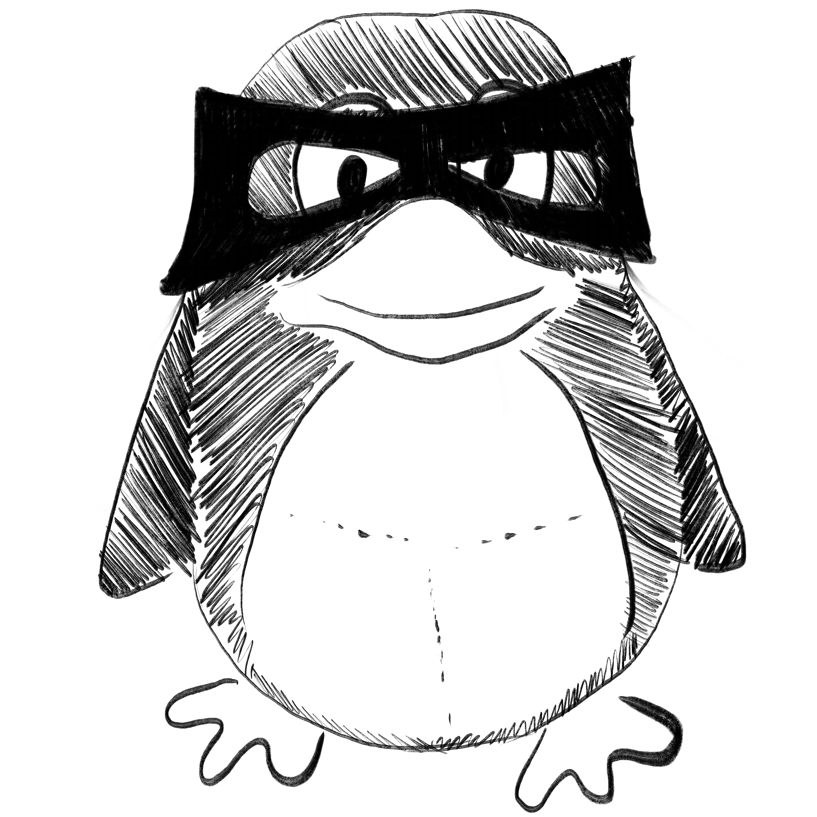
Performance drift in a mortality prediction algorithm among patients with cancer during the SARS-CoV-2 pandemic.
In Journal of the American Medical Informatics Association : JAMIA
Parikh Ravi B, Zhang Yichen, Kolla Likhitha, Chivers Corey, Courtright Katherine R, Zhu Jingsan, Navathe Amol S, Chen Jinbo
2022-Nov-21
SARS-CoV-2, algorithm drift, cancer, machine learning, mortality
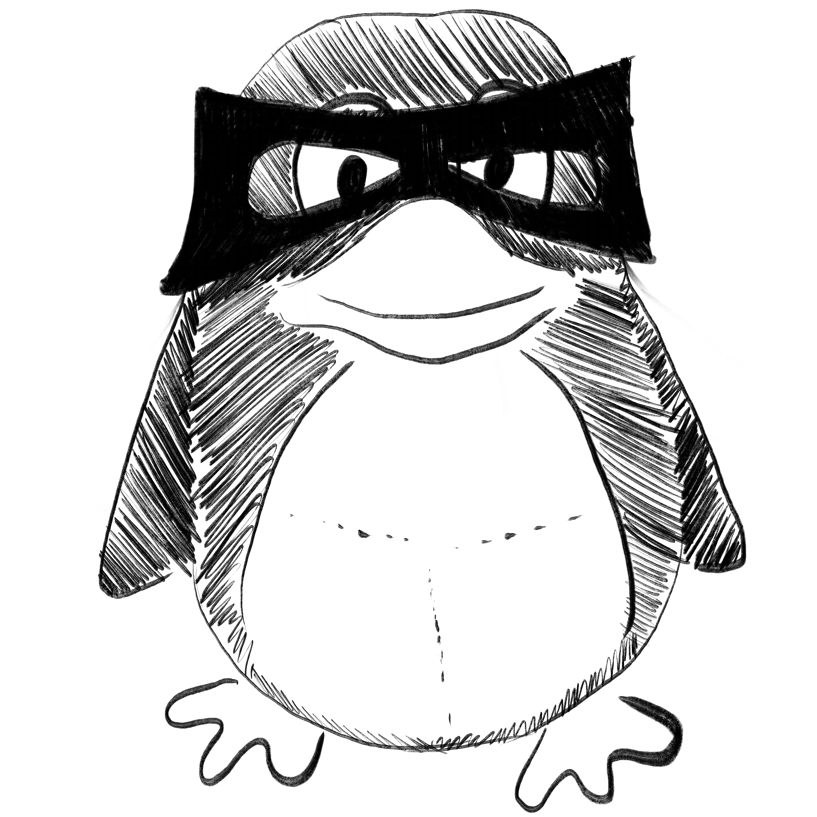
Deep reinforcement learning for optimal experimental design in biology.
In PLoS computational biology
Treloar Neythen J, Braniff Nathan, Ingalls Brian, Barnes Chris P
2022-Nov-21
External Validation of an Ensemble Model for Automated Mammography Interpretation by Artificial Intelligence.
In JAMA network open
Importance :
Objective :
Design, Setting, and Participants :
Main Outcomes and Measures :
Results :
Conclusions and Relevance :
Hsu William, Hippe Daniel S, Nakhaei Noor, Wang Pin-Chieh, Zhu Bing, Siu Nathan, Ahsen Mehmet Eren, Lotter William, Sorensen A Gregory, Naeim Arash, Buist Diana S M, Schaffter Thomas, Guinney Justin, Elmore Joann G, Lee Christoph I
2022-Nov-01
Cardiovascular CT, MRI, and PET/CT in 2021: Review of Key Articles.
In Radiology ; h5-index 91.0
Tzimas Georgios, Ryan David T, Murphy David J, Leipsic Jonathon A, Dodd Jonathan D
2022-Nov-15
Cardiovascular CT, MRI, and PET/CT in 2021: Review of Key Articles.
In Radiology ; h5-index 91.0
Tzimas Georgios, Ryan David T, Murphy David J, Leipsic Jonathon A, Dodd Jonathan D
2022-Nov-15
Deep Learning to Simulate Contrast-enhanced Breast MRI of Invasive Breast Cancer.
In Radiology ; h5-index 91.0
Chung Maggie, Calabrese Evan, Mongan John, Ray Kimberly M, Hayward Jessica H, Kelil Tatiana, Sieberg Ryan, Hylton Nola, Joe Bonnie N, Lee Amie Y
2022-Nov-15
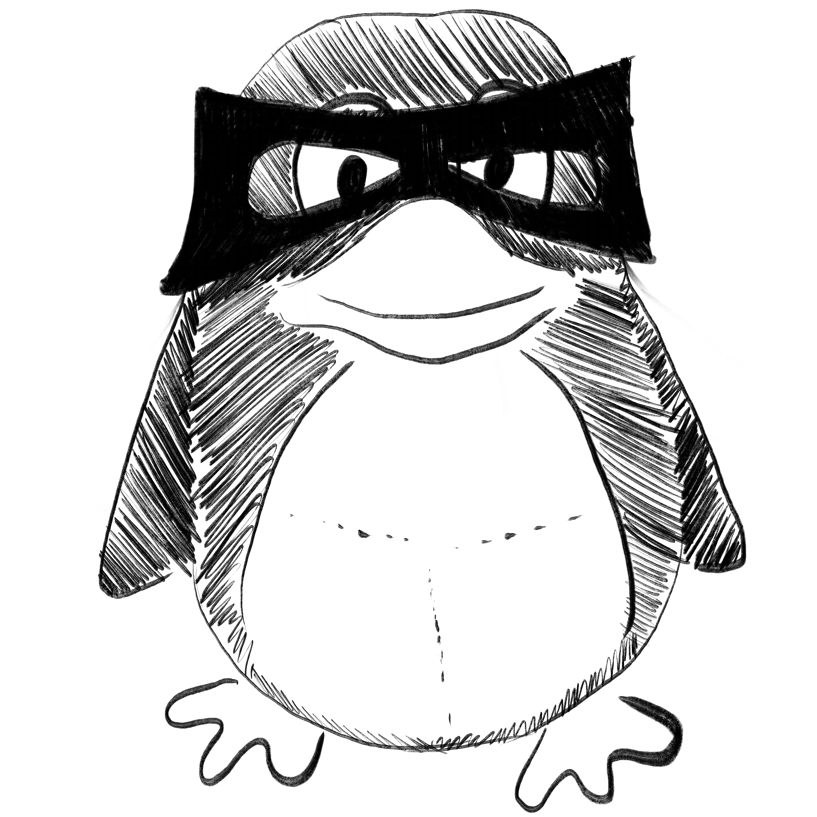
Diachronic and synchronic variation in the performance of adaptive machine learning systems: the ethical challenges.
In Journal of the American Medical Informatics Association : JAMIA
OBJECTIVES :
TARGET AUDIENCE :
SCOPE :
Hatherley Joshua, Sparrow Robert
2022-Nov-15
artificial intelligence, bioethics, federated learning, medicine, update problem
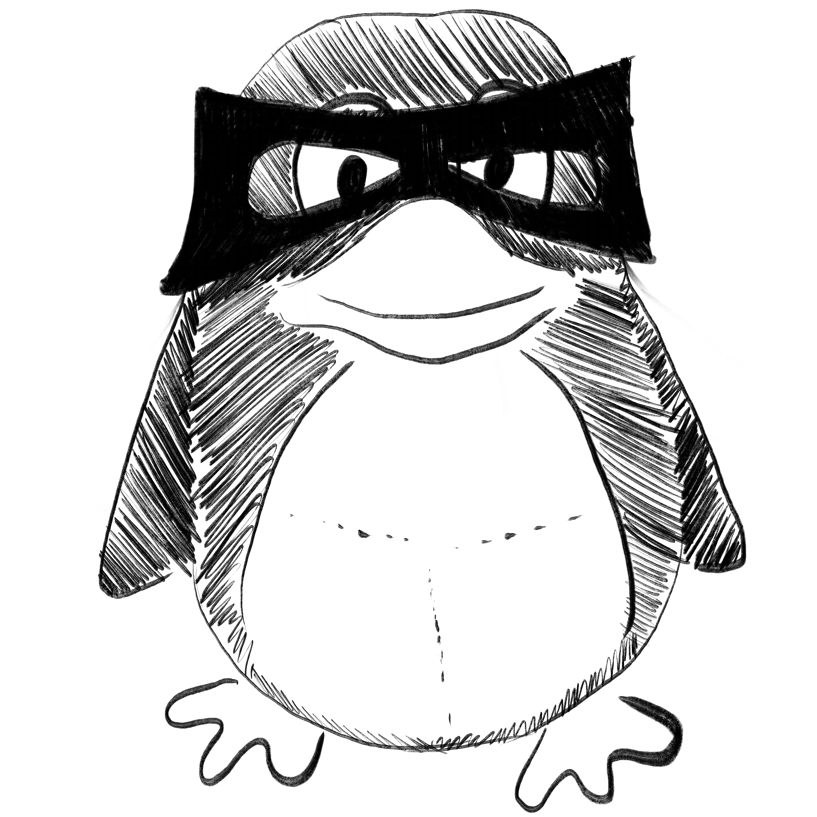
Using automated methods to detect safety problems with health information technology: a scoping review.
In Journal of the American Medical Informatics Association : JAMIA
OBJECTIVE :
MATERIALS AND METHODS :
RESULTS :
CONCLUSIONS :
Surian Didi, Wang Ying, Coiera Enrico, Magrabi Farah
2022-Nov-14
equipment failure analysis, health information technology, patient safety, review
Methods for Clinical Evaluation of Artificial Intelligence Algorithms for Medical Diagnosis.
In Radiology ; h5-index 91.0
Park Seong Ho, Han Kyunghwa, Jang Hye Young, Park Ji Eun, Lee June-Goo, Kim Dong Wook, Choi Jaesoon
2022-Nov-08
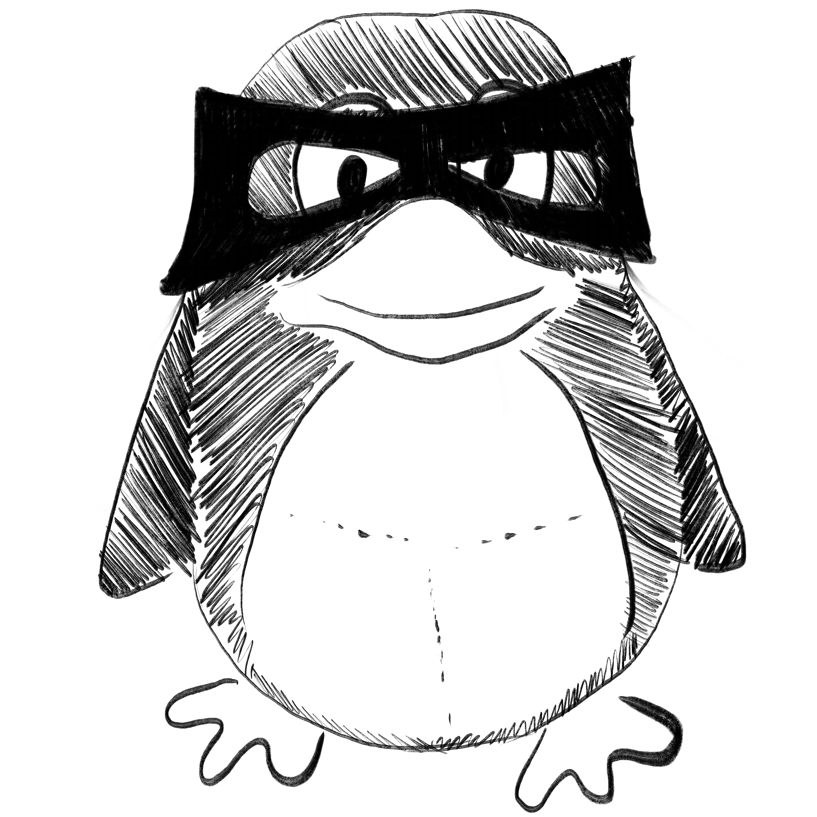
Using similar patients to predict complication in patients with diabetes, hypertension, and lipid disorder: a domain knowledge-infused convolutional neural network approach.
In Journal of the American Medical Informatics Association : JAMIA
OBJECTIVE :
MATERIALS AND METHODS :
RESULTS :
DISCUSSION AND CONCLUSIONS :
Oei Ronald Wihal, Hsu Wynne, Lee Mong Li, Tan Ngiap Chuan
2022-Nov-07
chronic diseases, convolutional neural network, domain knowledge, patient similarity
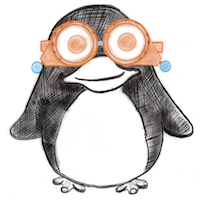
Systematic analysis and prediction of genes associated with monogenic disorders on human chromosome X.
In Nature communications ; h5-index 260.0
Leitão Elsa, Schröder Christopher, Parenti Ilaria, Dalle Carine, Rastetter Agnès, Kühnel Theresa, Kuechler Alma, Kaya Sabine, Gérard Bénédicte, Schaefer Elise, Nava Caroline, Drouot Nathalie, Engel Camille, Piard Juliette, Duban-Bedu Bénédicte, Villard Laurent, Stegmann Alexander P A, Vanhoutte Els K, Verdonschot Job A J, Kaiser Frank J, Tran Mau-Them Frédéric, Scala Marcello, Striano Pasquale, Frints Suzanna G M, Argilli Emanuela, Sherr Elliott H, Elder Fikret, Buratti Julien, Keren Boris, Mignot Cyril, Héron Delphine, Mandel Jean-Louis, Gecz Jozef, Kalscheuer Vera M, Horsthemke Bernhard, Piton Amélie, Depienne Christel
2022-Nov-02
Deep learning empowered volume delineation of whole-body organs-at-risk for accelerated radiotherapy.
In Nature communications ; h5-index 260.0
Shi Feng, Hu Weigang, Wu Jiaojiao, Han Miaofei, Wang Jiazhou, Zhang Wei, Zhou Qing, Zhou Jingjie, Wei Ying, Shao Ying, Chen Yanbo, Yu Yue, Cao Xiaohuan, Zhan Yiqiang, Zhou Xiang Sean, Gao Yaozong, Shen Dinggang
2022-Nov-02
Uncertainty-informed deep learning models enable high-confidence predictions for digital histopathology.
In Nature communications ; h5-index 260.0
Dolezal James M, Srisuwananukorn Andrew, Karpeyev Dmitry, Ramesh Siddhi, Kochanny Sara, Cody Brittany, Mansfield Aaron S, Rakshit Sagar, Bansal Radhika, Bois Melanie C, Bungum Aaron O, Schulte Jefree J, Vokes Everett E, Garassino Marina Chiara, Husain Aliya N, Pearson Alexander T
2022-Nov-02
An immune dysfunction score for stratification of patients with acute infection based on whole-blood gene expression.
In Science translational medicine ; h5-index 138.0
Cano-Gamez Eddie, Burnham Katie L, Goh Cyndi, Allcock Alice, Malick Zunaira H, Overend Lauren, Kwok Andrew, Smith David A, Peters-Sengers Hessel, Antcliffe David, McKechnie Stuart, Scicluna Brendon P, van der Poll Tom, Gordon Anthony C, Hinds Charles J, Davenport Emma E, Knight Julian C, Webster Nigel, Galley Helen, Taylor Jane, Hall Sally, Addison Jenni, Roughton Sian, Tennant Heather, Guleri Achyut, Waddington Natalia, Arawwawala Dilshan, Durcan John, Short Alasdair, Swan Karen, Williams Sarah, Smolen Susan, Mitchell-Inwang Christine, Gordon Tony, Errington Emily, Templeton Maie, Venatesh Pyda, Ward Geraldine, McCauley Marie, Baudouin Simon, Higham Charley, Soar Jasmeet, Grier Sally, Hall Elaine, Brett Stephen, Kitson David, Wilson Robert, Mountford Laura, Moreno Juan, Hall Peter, Hewlett Jackie, McKechnie Stuart, Garrard Christopher, Millo Julian, Young Duncan, Hutton Paula, Parsons Penny, Smiths Alex, Faras-Arraya Roser, Soar Jasmeet, Raymode Parizade, Thompson Jonathan, Bowrey Sarah, Kazembe Sandra, Rich Natalie, Andreou Prem, Hales Dawn, Roberts Emma, Fletcher Simon, Rosbergen Melissa, Glister Georgina, Cuesta Jeronimo Moreno, Bion Julian, Millar Joanne, Perry Elsa Jane, Willis Heather, Mitchell Natalie, Ruel Sebastian, Carrera Ronald, Wilde Jude, Nilson Annette, Lees Sarah, Kapila Atul, Jacques Nicola, Atkinson Jane, Brown Abby, Prowse Heather, Krige Anton, Bland Martin, Bullock Lynne, Harrison Donna, Mills Gary, Humphreys John, Armitage Kelsey, Laha Shond, Baldwin Jacqueline, Walsh Angela, Doherty Nicola, Drage Stephen, Ortiz-Ruiz de Gordoa Laura, Lowes Sarah, Higham Charley, Walsh Helen, Calder Verity, Swan Catherine, Payne Heather, Higgins David, Andrews Sarah, Mappleback Sarah, Hind Charles, Garrard Chris, Watson D, McLees Eleanor, Purdy Alice, Stotz Martin, Ochelli-Okpue Adaeze, Bonner Stephen, Whitehead Iain, Hugil Keith, Goodridge Victoria, Cawthor Louisa, Kuper Martin, Pahary Sheik, Bellingan Geoffrey, Marshall Richard, Montgomery Hugh, Ryu Jung Hyun, Bercades Georgia, Boluda Susan, Bentley Andrew, Mccalman Katie, Jefferies Fiona, Knight Julian, Davenport Emma, Burnham Katie, Maugeri Narelle, Radhakrishnan Jayachandran, Mi Yuxin, Allcock Alice, Goh Cyndi
2022-Nov-02
An immune dysfunction score for stratification of patients with acute infection based on whole-blood gene expression.
In Science translational medicine ; h5-index 138.0
Cano-Gamez Eddie, Burnham Katie L, Goh Cyndi, Allcock Alice, Malick Zunaira H, Overend Lauren, Kwok Andrew, Smith David A, Peters-Sengers Hessel, Antcliffe David, McKechnie Stuart, Scicluna Brendon P, van der Poll Tom, Gordon Anthony C, Hinds Charles J, Davenport Emma E, Knight Julian C, Webster Nigel, Galley Helen, Taylor Jane, Hall Sally, Addison Jenni, Roughton Sian, Tennant Heather, Guleri Achyut, Waddington Natalia, Arawwawala Dilshan, Durcan John, Short Alasdair, Swan Karen, Williams Sarah, Smolen Susan, Mitchell-Inwang Christine, Gordon Tony, Errington Emily, Templeton Maie, Venatesh Pyda, Ward Geraldine, McCauley Marie, Baudouin Simon, Higham Charley, Soar Jasmeet, Grier Sally, Hall Elaine, Brett Stephen, Kitson David, Wilson Robert, Mountford Laura, Moreno Juan, Hall Peter, Hewlett Jackie, McKechnie Stuart, Garrard Christopher, Millo Julian, Young Duncan, Hutton Paula, Parsons Penny, Smiths Alex, Faras-Arraya Roser, Soar Jasmeet, Raymode Parizade, Thompson Jonathan, Bowrey Sarah, Kazembe Sandra, Rich Natalie, Andreou Prem, Hales Dawn, Roberts Emma, Fletcher Simon, Rosbergen Melissa, Glister Georgina, Cuesta Jeronimo Moreno, Bion Julian, Millar Joanne, Perry Elsa Jane, Willis Heather, Mitchell Natalie, Ruel Sebastian, Carrera Ronald, Wilde Jude, Nilson Annette, Lees Sarah, Kapila Atul, Jacques Nicola, Atkinson Jane, Brown Abby, Prowse Heather, Krige Anton, Bland Martin, Bullock Lynne, Harrison Donna, Mills Gary, Humphreys John, Armitage Kelsey, Laha Shond, Baldwin Jacqueline, Walsh Angela, Doherty Nicola, Drage Stephen, Ortiz-Ruiz de Gordoa Laura, Lowes Sarah, Higham Charley, Walsh Helen, Calder Verity, Swan Catherine, Payne Heather, Higgins David, Andrews Sarah, Mappleback Sarah, Hind Charles, Garrard Chris, Watson D, McLees Eleanor, Purdy Alice, Stotz Martin, Ochelli-Okpue Adaeze, Bonner Stephen, Whitehead Iain, Hugil Keith, Goodridge Victoria, Cawthor Louisa, Kuper Martin, Pahary Sheik, Bellingan Geoffrey, Marshall Richard, Montgomery Hugh, Ryu Jung Hyun, Bercades Georgia, Boluda Susan, Bentley Andrew, Mccalman Katie, Jefferies Fiona, Knight Julian, Davenport Emma, Burnham Katie, Maugeri Narelle, Radhakrishnan Jayachandran, Mi Yuxin, Allcock Alice, Goh Cyndi
2022-Nov-02
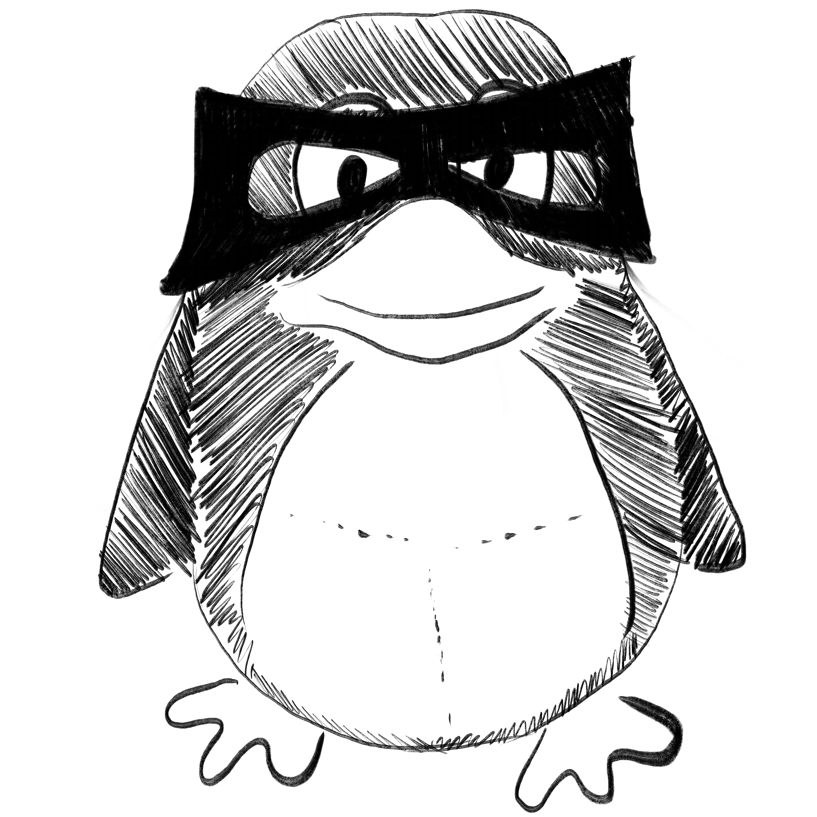
Unsupervised learning of aging principles from longitudinal data.
In Nature communications ; h5-index 260.0
Avchaciov Konstantin, Antoch Marina P, Andrianova Ekaterina L, Tarkhov Andrei E, Menshikov Leonid I, Burmistrova Olga, Gudkov Andrei V, Fedichev Peter O
2022-Nov-01
Deep Learning Reconstruction for Accelerated Spine MRI: Prospective Analysis of Interchangeability.
In Radiology ; h5-index 91.0
Almansour Haidara, Herrmann Judith, Gassenmaier Sebastian, Afat Saif, Jacoby Johann, Koerzdoerfer Gregor, Nickel Dominik, Mostapha Mahmoud, Nadar Mariappan, Othman Ahmed E
2022-Nov-01
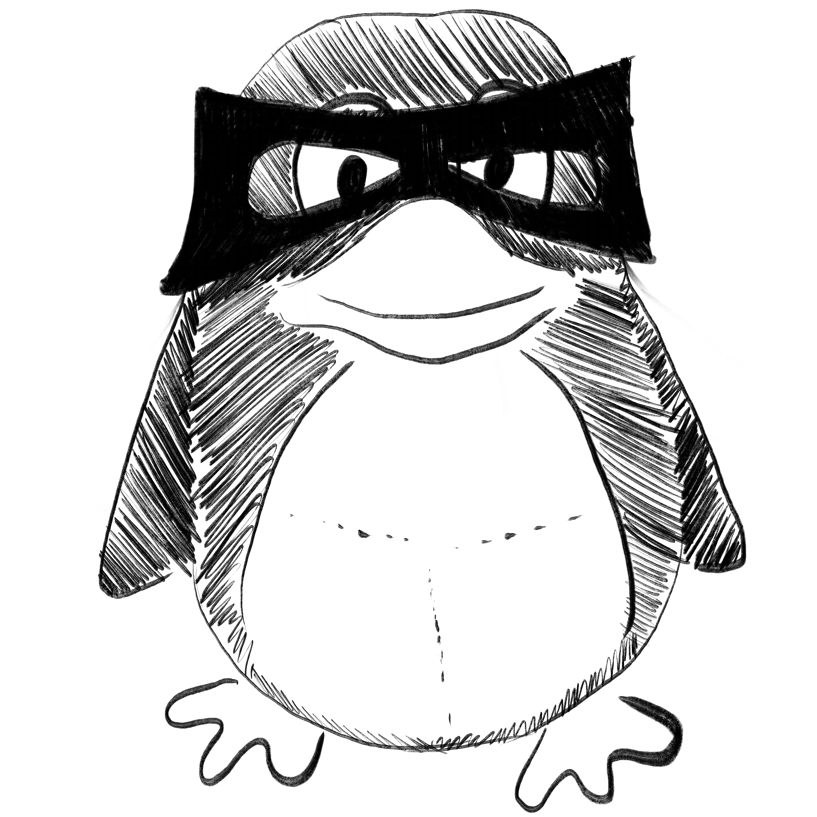
De novo analysis of bulk RNA-seq data at spatially resolved single-cell resolution.
In Nature communications ; h5-index 260.0
Liao Jie, Qian Jingyang, Fang Yin, Chen Zhuo, Zhuang Xiang, Zhang Ningyu, Shao Xin, Hu Yining, Yang Penghui, Cheng Junyun, Hu Yang, Yu Lingqi, Yang Haihong, Zhang Jinlu, Lu Xiaoyan, Shao Li, Wu Dan, Gao Yue, Chen Huajun, Fan Xiaohui
2022-Oct-30
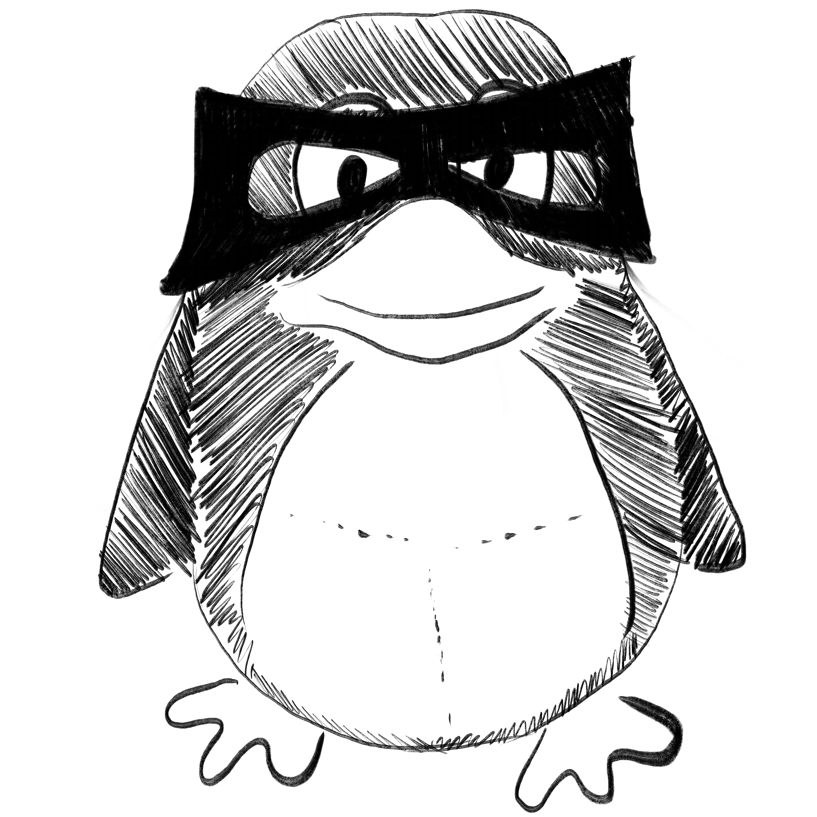
G2Φnet: Relating genotype and biomechanical phenotype of tissues with deep learning.
In PLoS computational biology
Zhang Enrui, Spronck Bart, Humphrey Jay D, Karniadakis George Em
2022-Oct-31
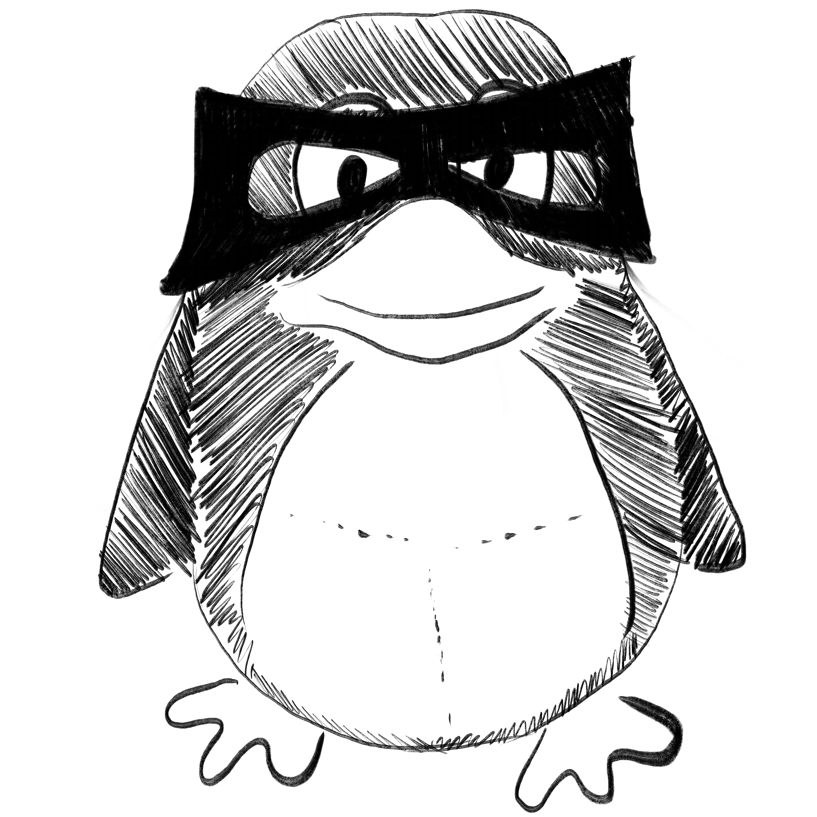
Mutual influence between language and perception in multi-agent communication games.
In PLoS computational biology
Ohmer Xenia, Marino Michael, Franke Michael, König Peter
2022-Oct-31
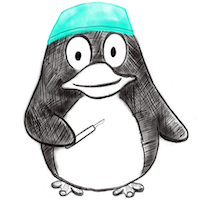
Assessing the carbon footprint of digital health interventions: a scoping review.
In Journal of the American Medical Informatics Association : JAMIA
OBJECTIVE :
MATERIALS AND METHODS :
RESULTS :
CONCLUSION :
Lokmic-Tomkins Zerina, Davies Shauna, Block Lorraine J, Cochrane Lindy, Dorin Alan, von Gerich Hanna, Lozada-Perezmitre Erika, Reid Lisa, Peltonen Laura-Maria
2022-Oct-31
assessment methods and tools, carbon emissions, digital health technologies, environmental sustainability
Deep-learning-based hepatic fat assessment (DeHFt) on non-contrast chest CT and its association with disease severity in COVID-19 infections: A multi-site retrospective study.
In EBioMedicine
BACKGROUND :
METHODS :
FINDINGS :
INTERPRETATION :
FUNDING :
Modanwal Gourav, Al-Kindi Sadeer, Walker Jonathan, Dhamdhere Rohan, Yuan Lei, Ji Mengyao, Lu Cheng, Fu Pingfu, Rajagopalan Sanjay, Madabhushi Anant
2022-Oct-26
COVID-19, Hepatic steatosis, NAFLD
Deep-learning-based hepatic fat assessment (DeHFt) on non-contrast chest CT and its association with disease severity in COVID-19 infections: A multi-site retrospective study.
In EBioMedicine
BACKGROUND :
METHODS :
FINDINGS :
INTERPRETATION :
FUNDING :
Modanwal Gourav, Al-Kindi Sadeer, Walker Jonathan, Dhamdhere Rohan, Yuan Lei, Ji Mengyao, Lu Cheng, Fu Pingfu, Rajagopalan Sanjay, Madabhushi Anant
2022-Oct-26
COVID-19, Hepatic steatosis, NAFLD
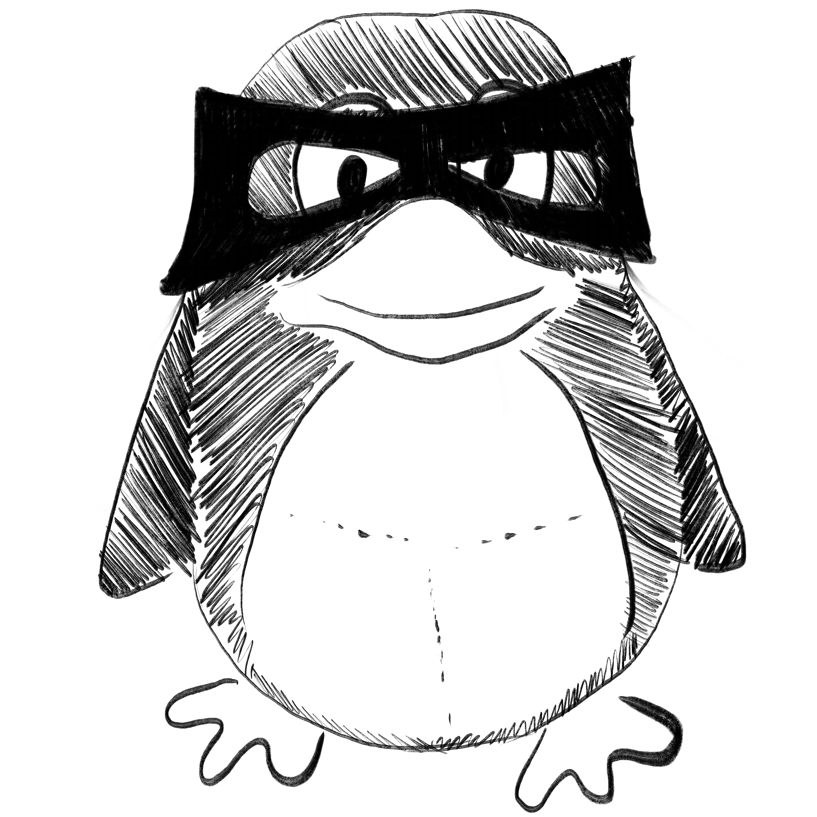
Multisite Evaluation of Prediction Models for Emergency Department Crowding Before and During the COVID-19 Pandemic.
In Journal of the American Medical Informatics Association : JAMIA
OBJECTIVE :
MATERIALS AND METHODS :
RESULTS :
DISCUSSION AND CONCLUSION :
Smith Ari J, Patterson Brian W, Pulia Michael S, Mayer John, Schwei Rebecca J, Nagarajan Radha, Liao Frank, Shah Manish N, Boutilier Justin J
2022-Oct-29
COVID-19, Emergency medicine, data drift, emergency department boarding, machine learning
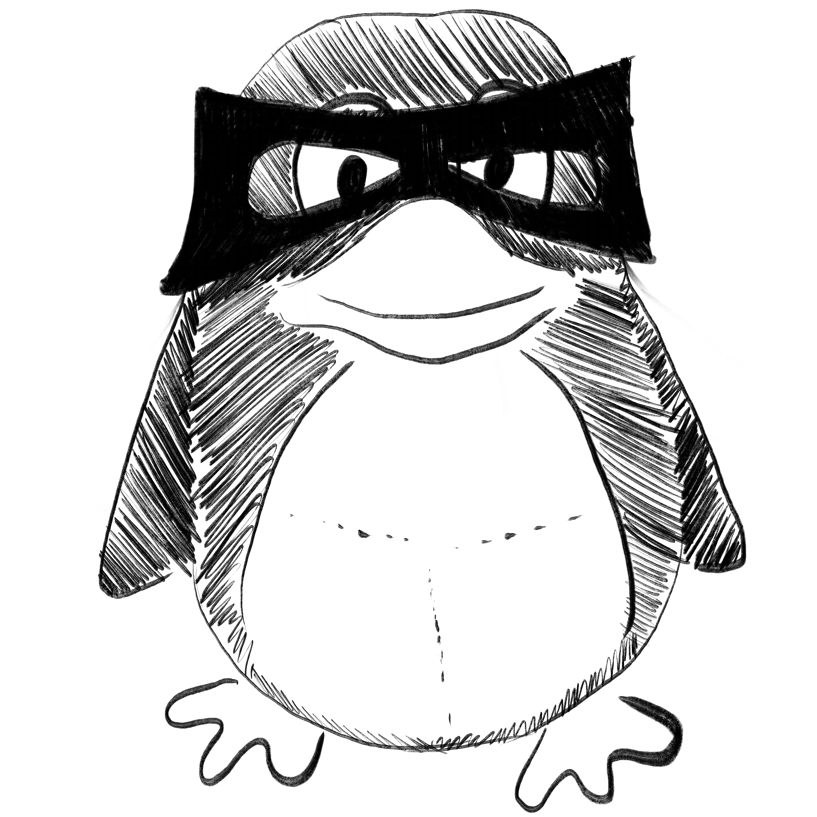
Multisite Evaluation of Prediction Models for Emergency Department Crowding Before and During the COVID-19 Pandemic.
In Journal of the American Medical Informatics Association : JAMIA
OBJECTIVE :
MATERIALS AND METHODS :
RESULTS :
DISCUSSION AND CONCLUSION :
Smith Ari J, Patterson Brian W, Pulia Michael S, Mayer John, Schwei Rebecca J, Nagarajan Radha, Liao Frank, Shah Manish N, Boutilier Justin J
2022-Oct-29
COVID-19, Emergency medicine, data drift, emergency department boarding, machine learning
Influences of rare copy-number variation on human complex traits.
In Cell ; h5-index 250.0
Hujoel Margaux L A, Sherman Maxwell A, Barton Alison R, Mukamel Ronen E, Sankaran Vijay G, Terao Chikashi, Loh Po-Ru
2022-Oct-27
Complex traits, Copy-number variants, Genetic associations, Haplotypes, Structural variation
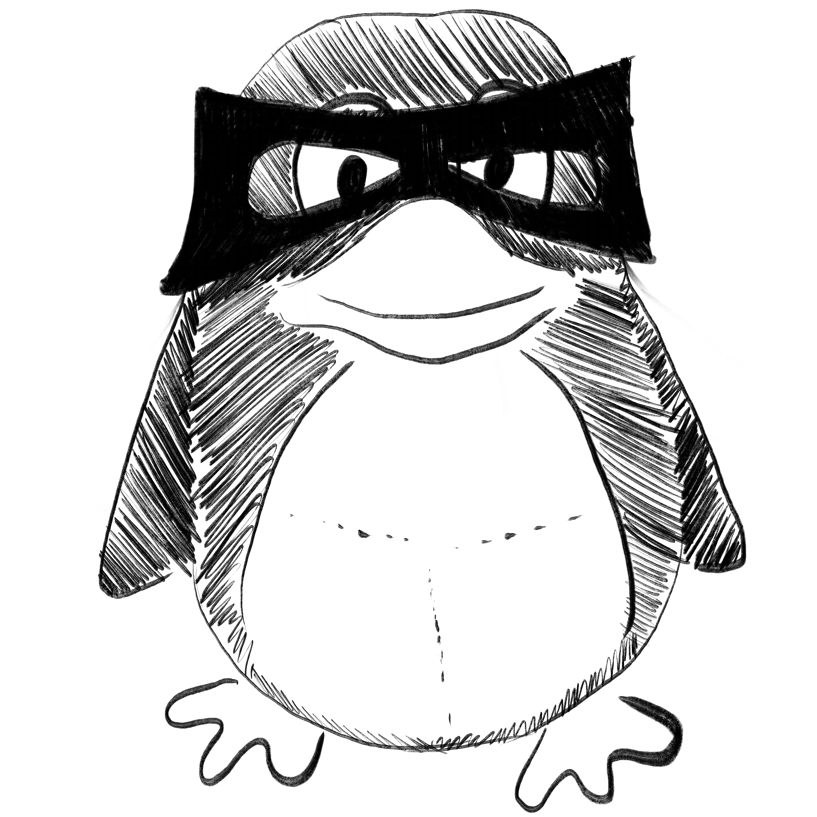
New onset delirium prediction using machine learning and long short-term memory (LSTM) in electronic health record.
In Journal of the American Medical Informatics Association : JAMIA
OBJECTIVE :
METHODS :
RESULTS :
CONCLUSION :
Liu Siru, Schlesinger Joseph J, McCoy Allison B, Reese Thomas J, Steitz Bryan, Russo Elise, Koh Brian, Wright Adam
2022-Oct-27
deep learning, delirium, explainable machine learning, predictive models
An analysis of the effects of limited training data in distributed learning scenarios for brain age prediction.
In Journal of the American Medical Informatics Association : JAMIA
OBJECTIVE :
MATERIALS AND METHODS :
RESULTS :
DISCUSSION :
CONCLUSION :
Souza Raissa, Mouches Pauline, Wilms Matthias, Tuladhar Anup, Langner Sönke, Forkert Nils D
2022-Oct-26
brain age prediction, distributed learning, machine learning
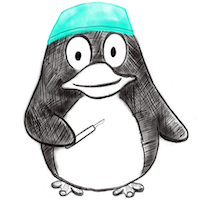
A time-aware attention model for prediction of acute kidney injury after pediatric cardiac surgery.
In Journal of the American Medical Informatics Association : JAMIA
OBJECTIVE :
MATERIALS AND METHODS :
RESULTS :
CONCLUSIONS :
Zeng Xian, Shi Shanshan, Sun Yuhan, Feng Yuqing, Tan Linhua, Lin Ru, Li Jianhua, Duan Huilong, Shu Qiang, Li Haomin
2022-Oct-26
acute kidney injury, deep machine learning, multi-perspectives evaluation, pediatric cardiac surgery, prediction model
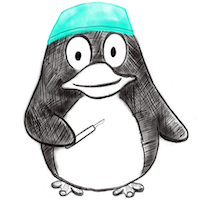
Comparison of Machine Learning Models Including Preoperative, Intraoperative, and Postoperative Data and Mortality After Cardiac Surgery.
In JAMA network open
Importance :
Objective :
Design, Setting, and Participants :
Exposure :
Main Outcomes and Measures :
Results :
Conclusions and Relevance :
Castela Forte José, Yeshmagambetova Galiya, van der Grinten Maureen L, Scheeren Thomas W L, Nijsten Maarten W N, Mariani Massimo A, Henning Robert H, Epema Anne H
2022-Oct-03
Deep Learning Segmentation and Reconstruction for CT of Chronic Total Coronary Occlusion.
In Radiology ; h5-index 91.0
Li Meiling, Ling Runjianya, Yu Lihua, Yang Wenyi, Chen Zirong, Wu Dijia, Zhang Jiayin
2022-Oct-25
Deep Learning for Estimating Lung Capacity on Chest Radiographs Predicts Survival in Idiopathic Pulmonary Fibrosis.
In Radiology ; h5-index 91.0
Kim Hyungjin, Jin Kwang Nam, Yoo Seung-Jin, Lee Chang Hoon, Lee Sang-Min, Hong Hyunsook, Witanto Joseph Nathanael, Yoon Soon Ho
2022-Oct-25
Molecular Characterization and Therapeutic Approaches to Small Cell Lung Cancer: Imaging Implications.
In Radiology ; h5-index 91.0
Park Hyesun, Tseng Shu-Chi, Sholl Lynette M, Hatabu Hiroto, Awad Mark M, Nishino Mizuki
2022-Oct-25
Virtual Biopsy by Using Artificial Intelligence-based Multimodal Modeling of Binational Mammography Data.
In Radiology ; h5-index 91.0
Barros Vesna, Tlusty Tal, Barkan Ella, Hexter Efrat, Gruen David, Guindy Michal, Rosen-Zvi Michal
2022-Oct-25
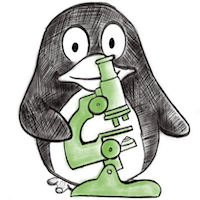
Annotation of spatially resolved single-cell data with STELLAR.
In Nature methods ; h5-index 152.0
Brbić Maria, Cao Kaidi, Hickey John W, Tan Yuqi, Snyder Michael P, Nolan Garry P, Leskovec Jure
2022-Oct-24
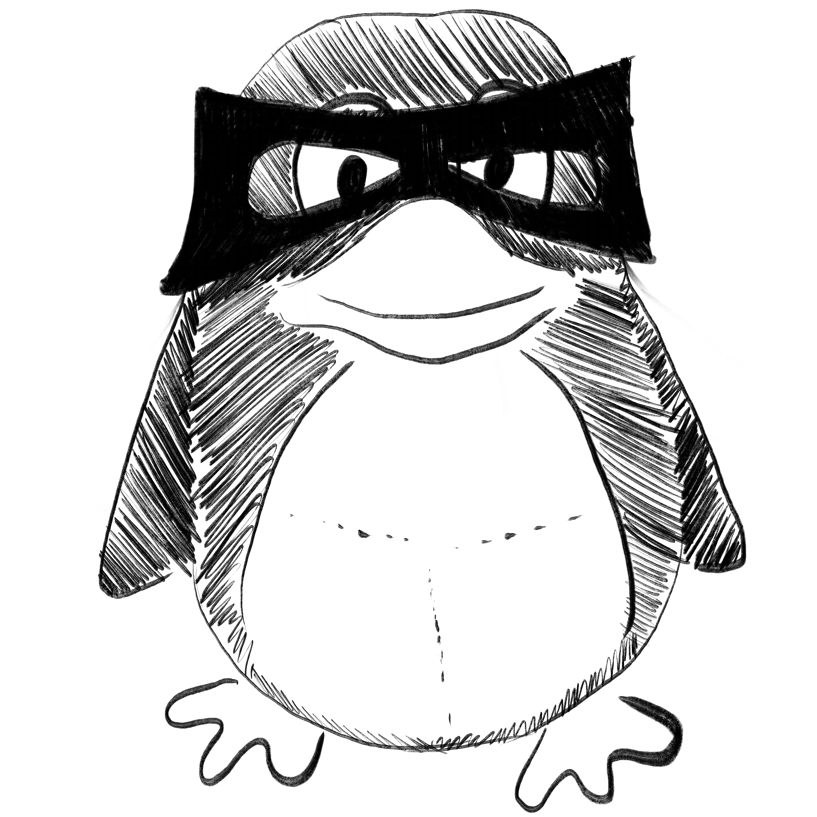
Deep mendelian randomization: Investigating the causal knowledge of genomic deep learning models.
In PLoS computational biology
Malina Stephen, Cizin Daniel, Knowles David A
2022-Oct-20
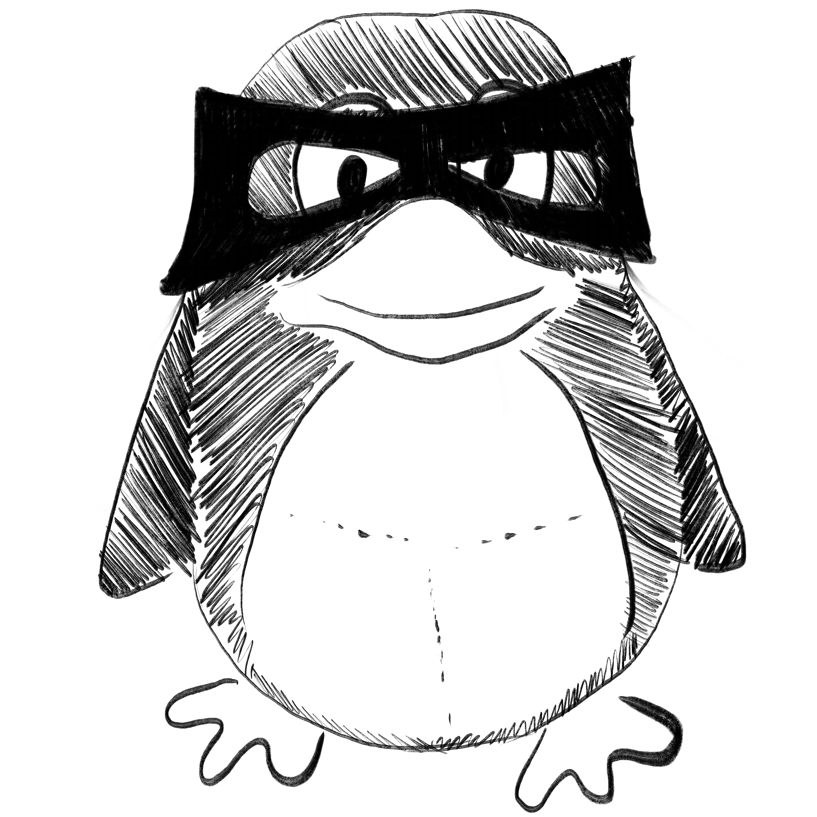
Ab initio predictions for 3D structure and stability of single- and double-stranded DNAs in ion solutions.
In PLoS computational biology
Mu Zi-Chun, Tan Ya-Lan, Zhang Ben-Gong, Liu Jie, Shi Ya-Zhou
2022-Oct-19
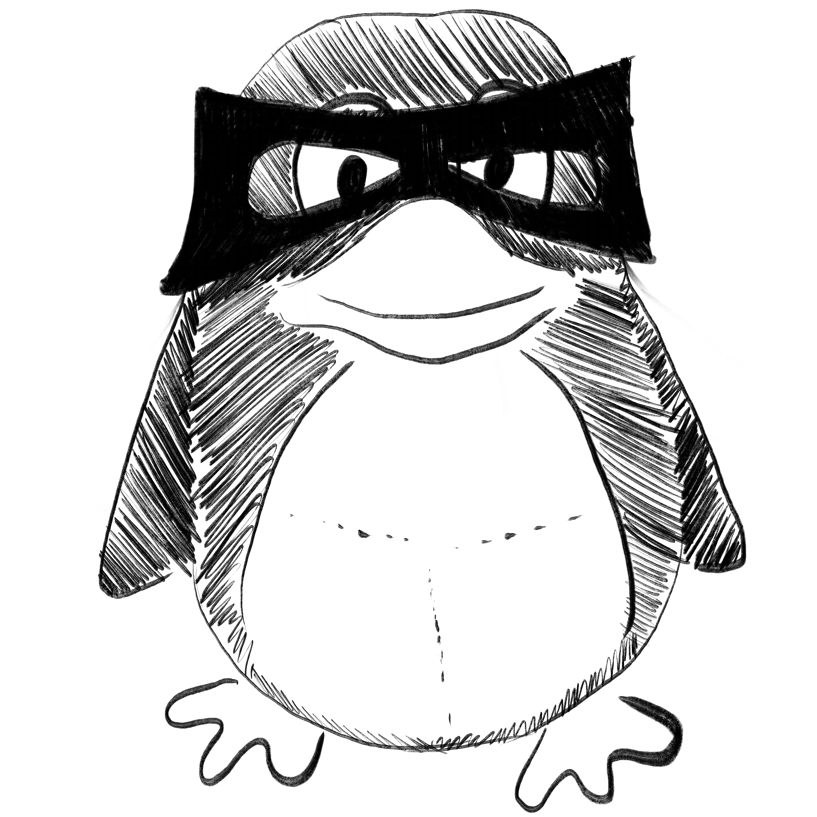
Clinical and Temporal Characterization of COVID-19 Subgroups Using Patient Vector Embeddings of Electronic Health Records.
In Journal of the American Medical Informatics Association : JAMIA
OBJECTIVE :
MATERIALS AND METHODS :
RESULTS :
DISCUSSION :
Ta Casey N, Zucker Jason E, Chiu Po-Hsiang, Fang Yilu, Natarajan Karthik, Weng Chunhua
2022-Oct-18
COVID-19, Cluster analysis, SARS-CoV-2, Unsupervised machine learning
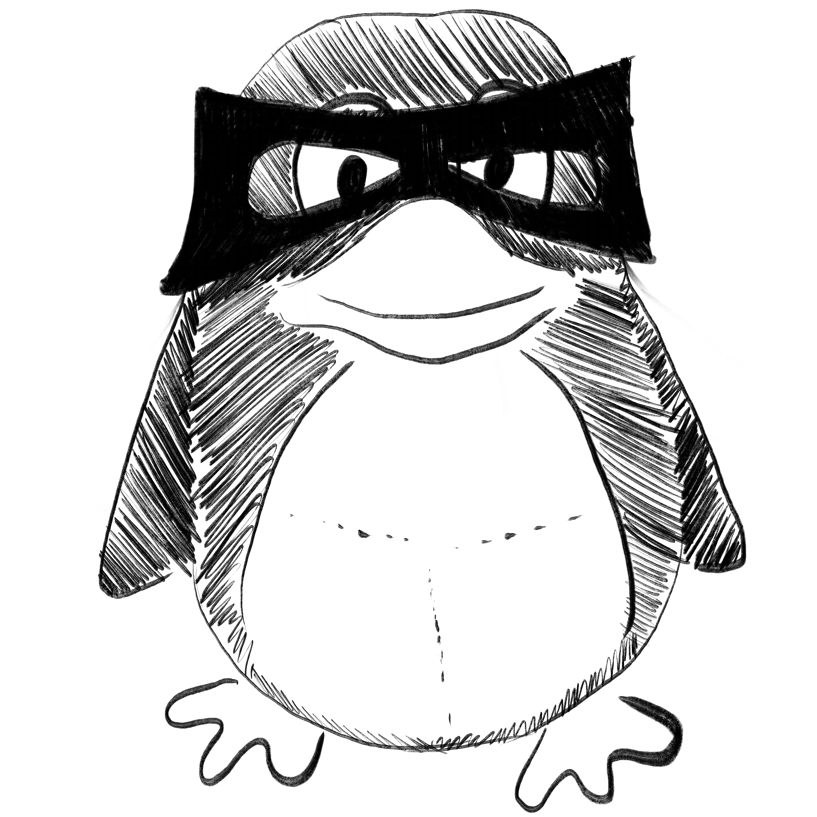
Clinical and Temporal Characterization of COVID-19 Subgroups Using Patient Vector Embeddings of Electronic Health Records.
In Journal of the American Medical Informatics Association : JAMIA
OBJECTIVE :
MATERIALS AND METHODS :
RESULTS :
DISCUSSION :
Ta Casey N, Zucker Jason E, Chiu Po-Hsiang, Fang Yilu, Natarajan Karthik, Weng Chunhua
2022-Oct-18
COVID-19, Cluster analysis, SARS-CoV-2, Unsupervised machine learning
How does the artificial intelligence-based image-assisted technique help physicians in diagnosis of pulmonary adenocarcinoma? A randomized controlled experiment of multicenter physicians in China.
In Journal of the American Medical Informatics Association : JAMIA
OBJECTIVE :
MATERIALS AND METHODS :
RESULTS :
DISCUSSION :
CONCLUSION :
Li Jiaoyang, Zhou Lingxiao, Zhan Yi, Xu Haifeng, Zhang Cheng, Shan Fei, Liu Lei
2022-Oct-13
artificial intelligence, diagnostic performance, multicenter physicians, self-efficacy, time pressure
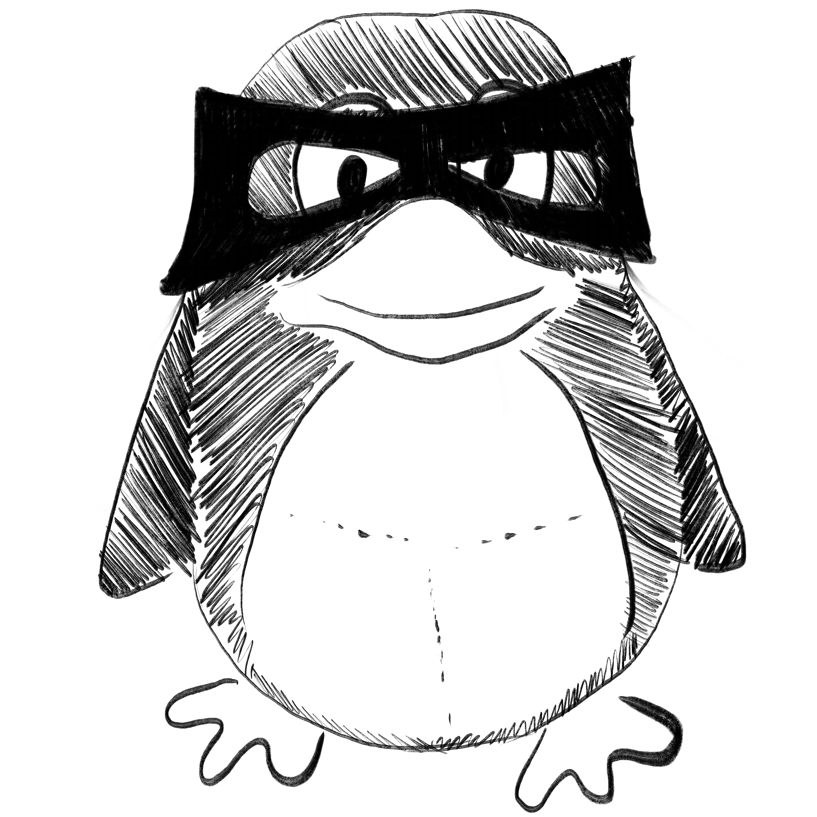
Calibrating spatiotemporal models of microbial communities to microscopy data: A review.
In PLoS computational biology
Yip Aaron, Smith-Roberge Julien, Khorasani Sara Haghayegh, Aucoin Marc G, Ingalls Brian P
2022-Oct
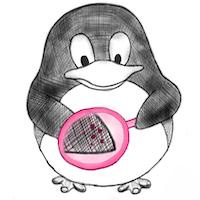
Translational gaps and opportunities for medical wearables in digital health.
In Science translational medicine ; h5-index 138.0
Xu Shuai, Kim Joohee, Walter Jessica R, Ghaffari Roozbeh, Rogers John A
2022-Oct-12
Associations of Early-Life Exposure to Submicron Particulate Matter With Childhood Asthma and Wheeze in China.
In JAMA network open
Importance :
Objective :
Design, Setting, and Participants :
Exposures :
Main Outcomes and Measures :
Results :
Conclusions and Relevance :
Wu Chuansha, Zhang Yunquan, Wei Jing, Zhao Zhuohui, Norbäck Dan, Zhang Xin, Lu Chan, Yu Wei, Wang Tingting, Zheng Xiaohong, Zhang Ling
2022-Oct-03
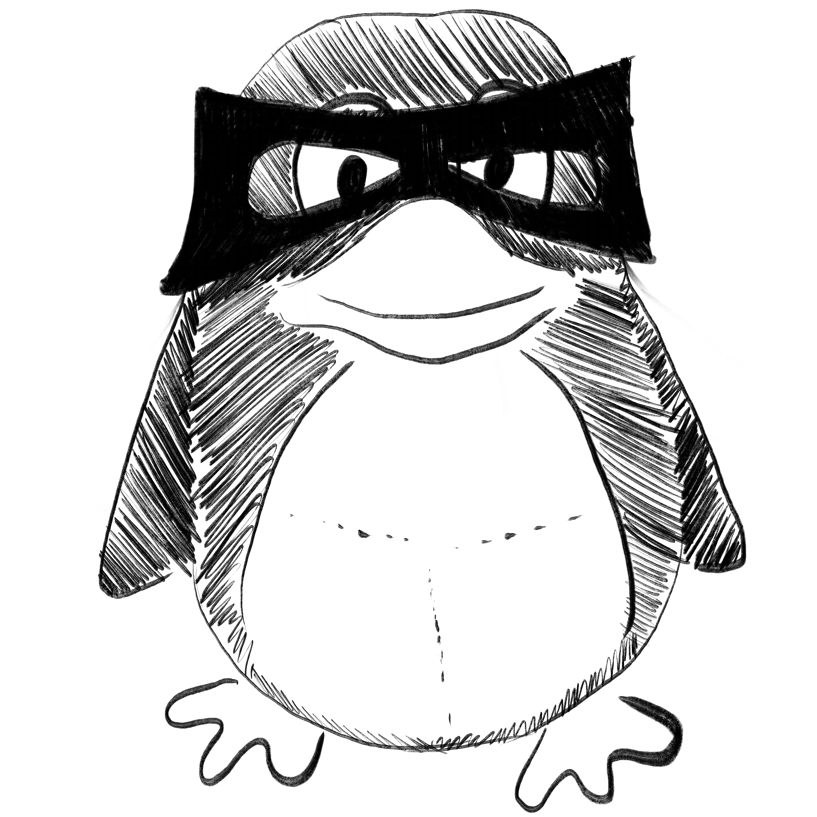
Rapid detection and recognition of whole brain activity in a freely behaving Caenorhabditis elegans.
In PLoS computational biology
Wu Yuxiang, Wu Shang, Wang Xin, Lang Chengtian, Zhang Quanshi, Wen Quan, Xu Tianqi
2022-Oct-10
Evaluation of Federated Learning Variations for COVID-19 diagnosis using Chest Radiographs from 42 US and European hospitals.
In Journal of the American Medical Informatics Association : JAMIA
OBJECTIVE :
MATERIALS AND METHODS :
RESULTS :
CONCLUSION :
Peng Le, Luo Gaoxiang, Walker Andrew, Zaiman Zachary, Jones Emma K, Gupta Hemant, Kersten Kristopher, Burns John L, Harle Christopher A, Magoc Tanja, Shickel Benjamin, Steenburg Scott D, Loftus Tyler, Melton Genevieve B, Gichoya Judy Wawira, Sun Ju, Tignanelli Christopher J
2022-Oct-10
Artificial Intelligence, COVID-19, Computer Vision, Federated Learning
Evaluation of Federated Learning Variations for COVID-19 diagnosis using Chest Radiographs from 42 US and European hospitals.
In Journal of the American Medical Informatics Association : JAMIA
OBJECTIVE :
MATERIALS AND METHODS :
RESULTS :
CONCLUSION :
Peng Le, Luo Gaoxiang, Walker Andrew, Zaiman Zachary, Jones Emma K, Gupta Hemant, Kersten Kristopher, Burns John L, Harle Christopher A, Magoc Tanja, Shickel Benjamin, Steenburg Scott D, Loftus Tyler, Melton Genevieve B, Gichoya Judy Wawira, Sun Ju, Tignanelli Christopher J
2022-Oct-10
Artificial Intelligence, COVID-19, Computer Vision, Federated Learning
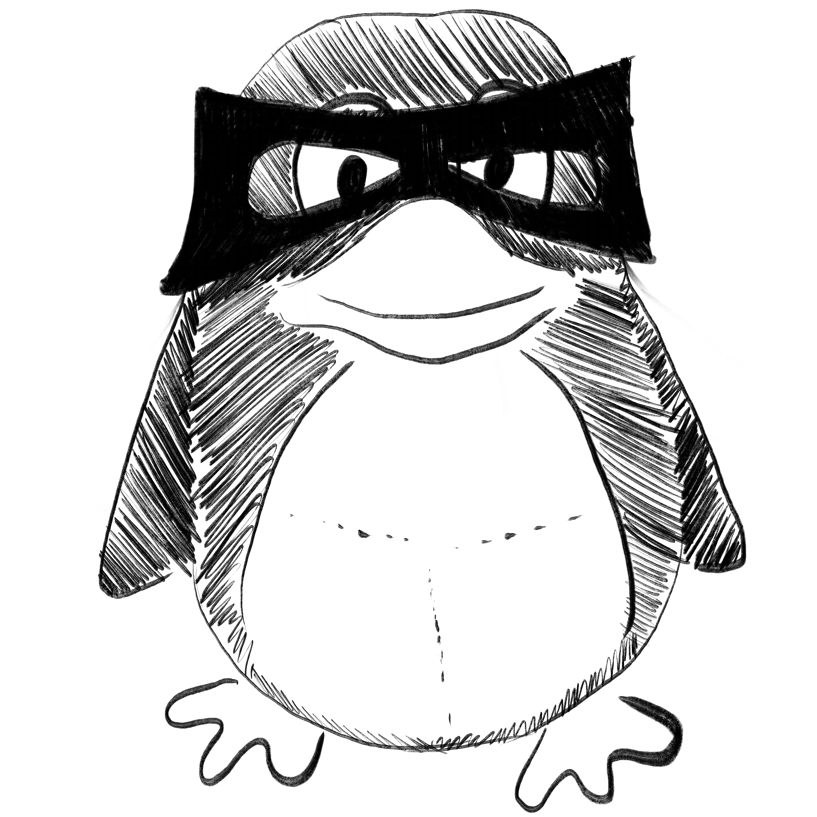
DLoopCaller: A deep learning approach for predicting genome-wide chromatin loops by integrating accessible chromatin landscapes.
In PLoS computational biology
Wang Siguo, Zhang Qinhu, He Ying, Cui Zhen, Guo Zhenghao, Han Kyungsook, Huang De-Shuang
2022-Oct-07
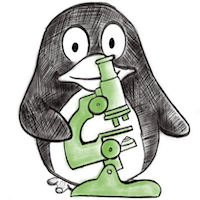
Prediction of Epstein-Barr Virus Status in Gastric Cancer Biopsy Specimens Using a Deep Learning Algorithm.
In JAMA network open
Importance :
Objective :
Design, Setting, and Participants :
Main Outcomes and Measures :
Results :
Conclusions and Relevance :
Vuong Trinh Thi Le, Song Boram, Kwak Jin T, Kim Kyungeun
2022-Oct-03
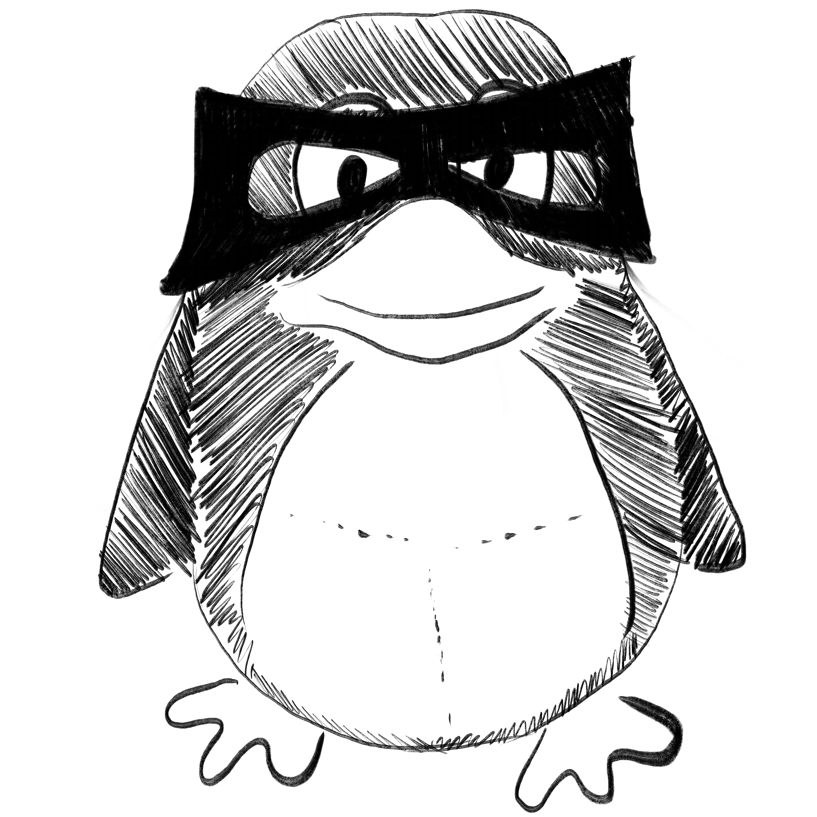
Hospital trajectories and early predictors of clinical outcomes differ between SARS-CoV-2 and influenza pneumonia.
In EBioMedicine
BACKGROUND :
METHODS :
FINDINGS :
INTERPRETATION :
FUNDING :
Lyons Patrick G, Bhavani Sivasubramanium V, Mody Aaloke, Bewley Alice, Dittman Katherine, Doyle Aisling, Windham Samuel L, Patel Tej M, Raju Bharat Neelam, Keller Matthew, Churpek Matthew M, Calfee Carolyn S, Michelson Andrew P, Kannampallil Thomas, Geng Elvin H, Sinha Pratik
2022-Oct-03
Hospital outcomes, Influenza, SARS-CoV-2, Statistical modelling, Viral pneumonia
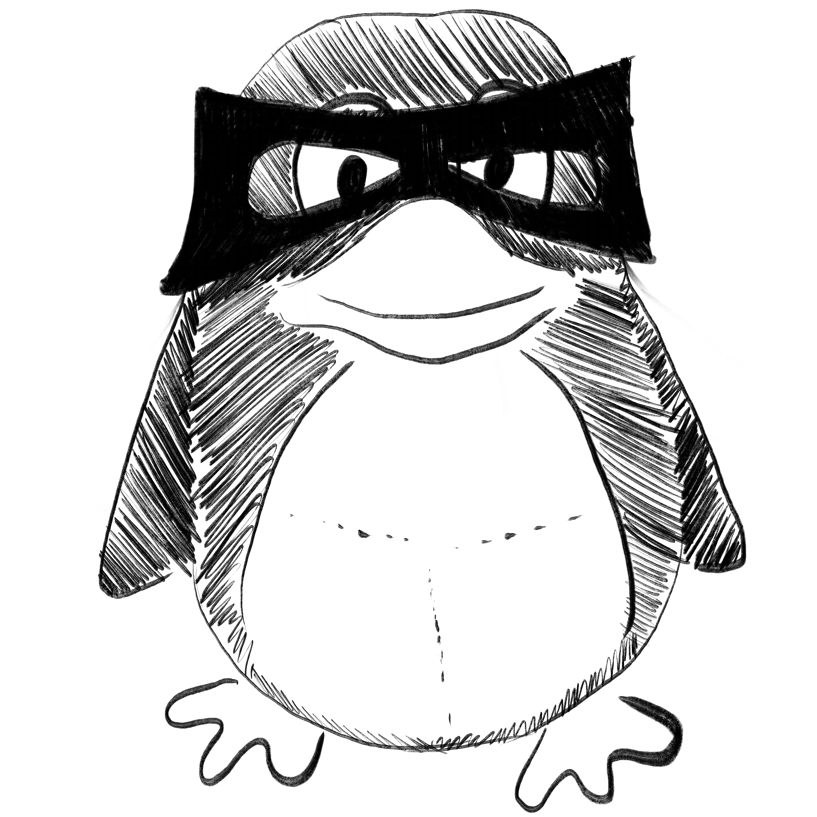
Hospital trajectories and early predictors of clinical outcomes differ between SARS-CoV-2 and influenza pneumonia.
In EBioMedicine
BACKGROUND :
METHODS :
FINDINGS :
INTERPRETATION :
FUNDING :
Lyons Patrick G, Bhavani Sivasubramanium V, Mody Aaloke, Bewley Alice, Dittman Katherine, Doyle Aisling, Windham Samuel L, Patel Tej M, Raju Bharat Neelam, Keller Matthew, Churpek Matthew M, Calfee Carolyn S, Michelson Andrew P, Kannampallil Thomas, Geng Elvin H, Sinha Pratik
2022-Oct-03
Hospital outcomes, Influenza, SARS-CoV-2, Statistical modelling, Viral pneumonia
Comparison of Natural Language Processing of Clinical Notes With a Validated Risk-Stratification Tool to Predict Severe Maternal Morbidity.
In JAMA network open
Importance :
Objective :
Design, Setting, and Participants :
Exposures :
Main Outcomes and Measures :
Results :
Conclusions and Relevance :
Clapp Mark A, Kim Ellen, James Kaitlyn E, Perlis Roy H, Kaimal Anjali J, McCoy Thomas H, Easter Sarah Rae
2022-Oct-03
Baseline host determinants of robust human HIV-1 vaccine-induced immune responses: A meta-analysis of 26 vaccine regimens.
In EBioMedicine
BACKGROUND :
METHODS :
FINDINGS :
INTERPRETATION :
FUNDING :
Huang Yunda, Zhang Yuanyuan, Seaton Kelly E, De Rosa Stephen, Heptinstall Jack, Carpp Lindsay N, Randhawa April Kaur, McKinnon Lyle R, McLaren Paul, Viegas Edna, Gray Glenda E, Churchyard Gavin, Buchbinder Susan P, Edupuganti Srilatha, Bekker Linda-Gail, Keefer Michael C, Hosseinipour Mina C, Goepfert Paul A, Cohen Kristen W, Williamson Brian D, McElrath M Juliana, Tomaras Georgia D, Thakar Juilee, Kobie James J
2022-Sep-27
Antibody, Baseline characteristics, CD4+ T cell, SuperLearner, Vaccine response heterogeneity, Variable importance measurements
Baseline host determinants of robust human HIV-1 vaccine-induced immune responses: A meta-analysis of 26 vaccine regimens.
In EBioMedicine
BACKGROUND :
METHODS :
FINDINGS :
INTERPRETATION :
FUNDING :
Huang Yunda, Zhang Yuanyuan, Seaton Kelly E, De Rosa Stephen, Heptinstall Jack, Carpp Lindsay N, Randhawa April Kaur, McKinnon Lyle R, McLaren Paul, Viegas Edna, Gray Glenda E, Churchyard Gavin, Buchbinder Susan P, Edupuganti Srilatha, Bekker Linda-Gail, Keefer Michael C, Hosseinipour Mina C, Goepfert Paul A, Cohen Kristen W, Williamson Brian D, McElrath M Juliana, Tomaras Georgia D, Thakar Juilee, Kobie James J
2022-Sep-27
Antibody, Baseline characteristics, CD4+ T cell, SuperLearner, Vaccine response heterogeneity, Variable importance measurements
Weekly Summary
Receive a weekly summary and discussion of the top papers of the week by leading researchers in the field.