Receive a weekly summary and discussion of the top papers of the week by leading researchers in the field.
Deep Learning Model for Coronary Angiography.
In Journal of cardiovascular translational research
Ling Hao, Chen Biqian, Guan Renchu, Xiao Yu, Yan Hui, Chen Qingyu, Bi Lianru, Chen Jingbo, Feng Xiaoyue, Pang Haoyu, Song Chunli
2023-Mar-16
Coronary artery stenosis, Deep learning, Diagnosis, Instance segmentation, Object detection
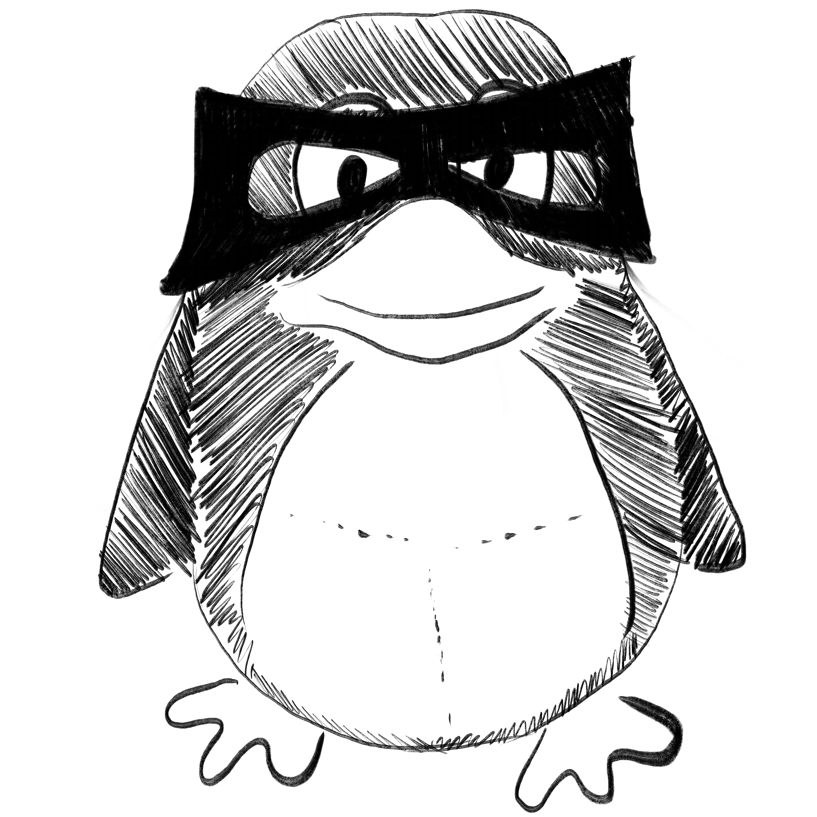
Heterogeneous intercalated metal-organic framework active materials for fast-charging non-aqueous Li-ion capacitors.
In Nature communications ; h5-index 260.0
Ogihara Nobuhiro, Hasegawa Masaki, Kumagai Hitoshi, Mikita Riho, Nagasako Naoyuki
2023-Mar-16
Prognostic value of deep learning-based fibrosis quantification on chest CT in idiopathic pulmonary fibrosis.
In European radiology ; h5-index 62.0
OBJECTIVE :
METHODS :
RESULTS :
CONCLUSION :
KEY POINTS :
Nam Ju Gang, Choi Yunhee, Lee Sang-Min, Yoon Soon Ho, Goo Jin Mo, Kim Hyungjin
2023-Mar-16
Deep learning, Idiopathic pulmonary fibrosis, Multidetector computed tomography, Prognosis
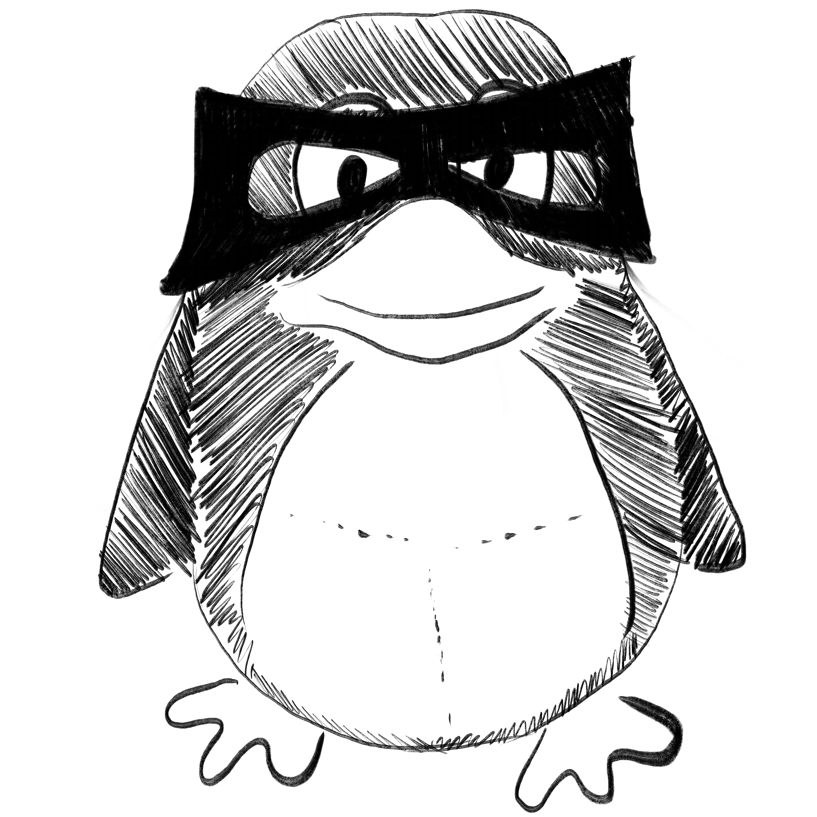
Ultrafast MRI using deep learning echoplanar imaging for a comprehensive assessment of acute ischemic stroke.
In European radiology ; h5-index 62.0
OBJECTIVES :
METHODS :
RESULTS :
CONCLUSIONS :
KEY POINTS :
Verclytte Sebastien, Gnanih Robin, Verdun Stephane, Feiweier Thorsten, Clifford Bryan, Ambarki Khalid, Pasquini Marta, Ding Juliette
2023-Mar-16
Artificial intelligence, Ischemic stroke, Magnetic resonance imaging
Synthetic T2-weighted fat sat based on a generative adversarial network shows potential for scan time reduction in spine imaging in a multicenter test dataset.
In European radiology ; h5-index 62.0
OBJECTIVES :
METHODS :
RESULTS :
DISCUSSION :
KEY POINTS :
Schlaeger Sarah, Drummer Katharina, El Husseini Malek, Kofler Florian, Sollmann Nico, Schramm Severin, Zimmer Claus, Wiestler Benedikt, Kirschke Jan S
2023-Mar-16
Artificial intelligence, Magnetic resonance imaging, Spine
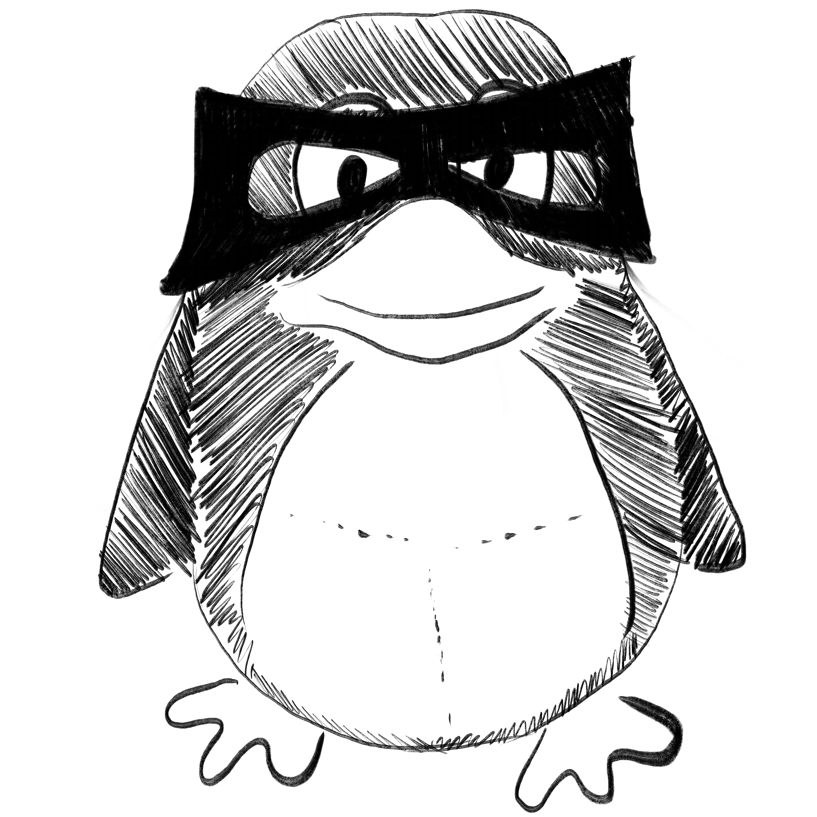
Distribution of [11C]-JNJ-42491293 in the marmoset brain: a positron emission tomography study.
In Naunyn-Schmiedeberg's archives of pharmacology
Kang Min Su, Hamadjida Adjia, Bédard Dominique, Nuara Stephen G, Gourdon Jim C, Frey Stephen, Aliaga Arturo, Ross Karen, Hopewell Robert, Bdair Hussein, Mathieu Axel, Tardif Christine Lucas, Soucy Jean-Paul, Massarweh Gassan, Rosa-Neto Pedro, Huot Philippe
2023-Mar-16
AZD8529, Marmoset, PET, Positive allosteric modulator, [11C]-JNJ-42491293, mGlu2 receptor
Weekly Summary
Receive a weekly summary and discussion of the top papers of the week by leading researchers in the field.