Receive a weekly summary and discussion of the top papers of the week by leading researchers in the field.
AI co-pilot: content-based image retrieval for the reading of rare diseases in chest CT.
In Scientific reports ; h5-index 158.0
Haubold Johannes, Zeng Ke, Farhand Sepehr, Stalke Sarah, Steinberg Hannah, Bos Denise, Meetschen Mathias, Kureishi Anisa, Zensen Sebastian, Goeser Tim, Maier Sandra, Forsting Michael, Nensa Felix
2023-Mar-16
Development and clinical utility analysis of a prostate zonal segmentation model on T2-weighted imaging: a multicenter study.
In Insights into imaging
OBJECTIVES :
METHODS :
RESULTS :
CONCLUSIONS :
Xu Lili, Zhang Gumuyang, Zhang Daming, Zhang Jiahui, Zhang Xiaoxiao, Bai Xin, Chen Li, Peng Qianyu, Jin Ru, Mao Li, Li Xiuli, Jin Zhengyu, Sun Hao
2023-Mar-16
Deep learning, Magnetic resonance imaging, Multicenter study, Prostate, Segmentation
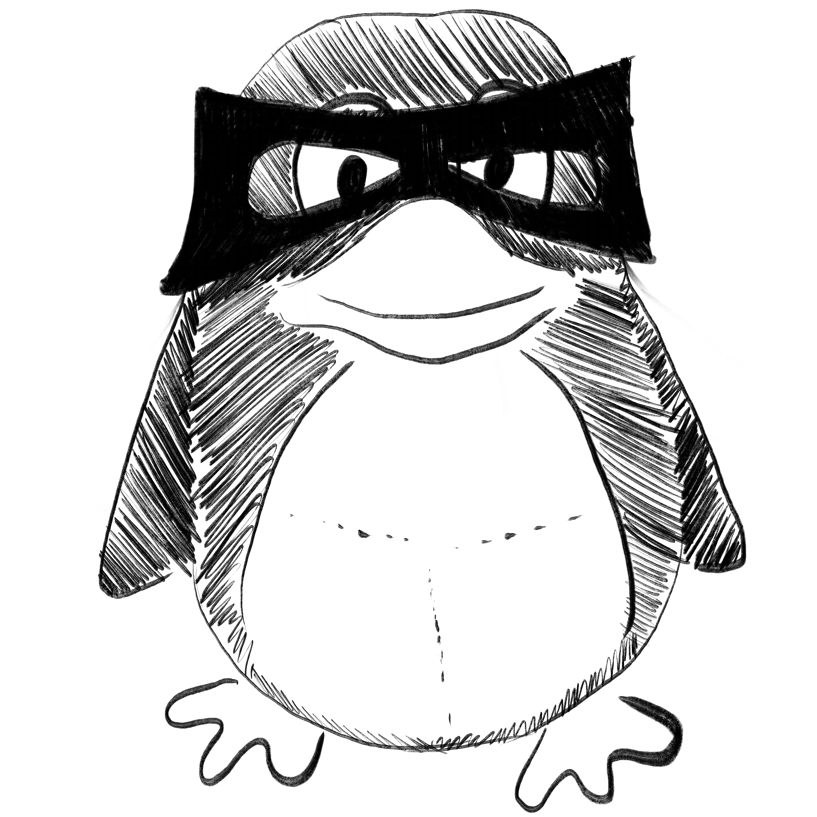
LST-EMG-Net: Long short-term transformer feature fusion network for sEMG gesture recognition.
In Frontiers in neurorobotics
Zhang Wenli, Zhao Tingsong, Zhang Jianyi, Wang Yufei
2023
gesture recognition, human-computer interaction, multi-head attention, multi-scale features, sEMG signals, stroke rehabilitation
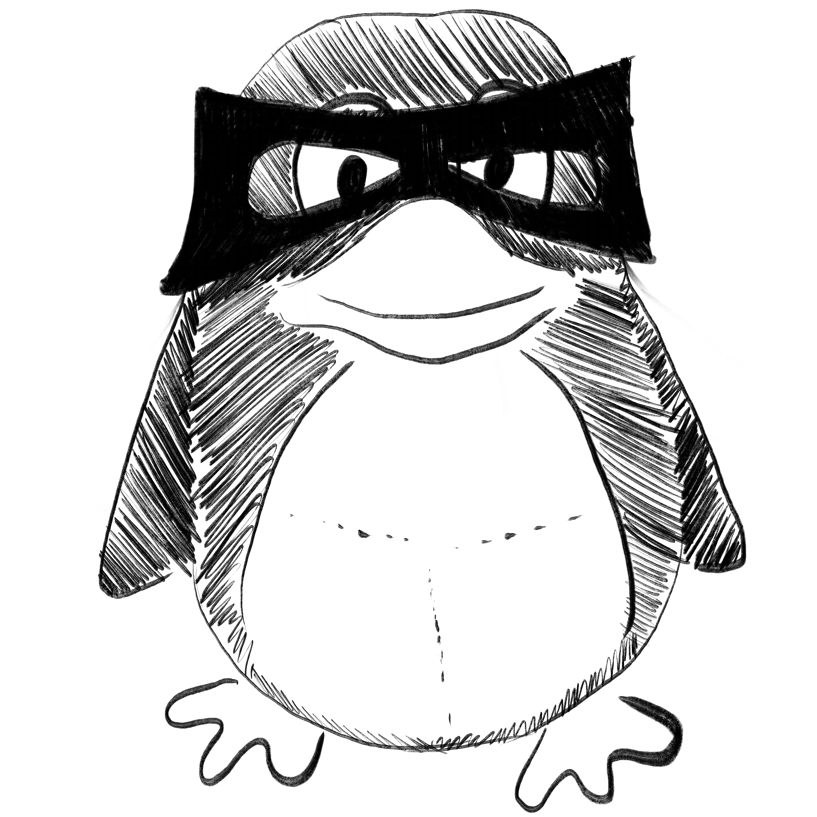
Identification of medicinal plant-based phytochemicals as a potential inhibitor for SARS-CoV-2 main protease (Mpro) using molecular docking and deep learning methods.
In Computers in biology and medicine
Hossain Alomgir, Rahman Md Ekhtiar, Rahman Md Siddiqur, Nasirujjaman Khondokar, Matin Mohammad Nurul, Faruqe Md Omar, Rabbee Muhammad Fazle
2023-Mar-11
Catechin gallate, Deep learning, Main protease, Molecular docking, SARS-CoV-2
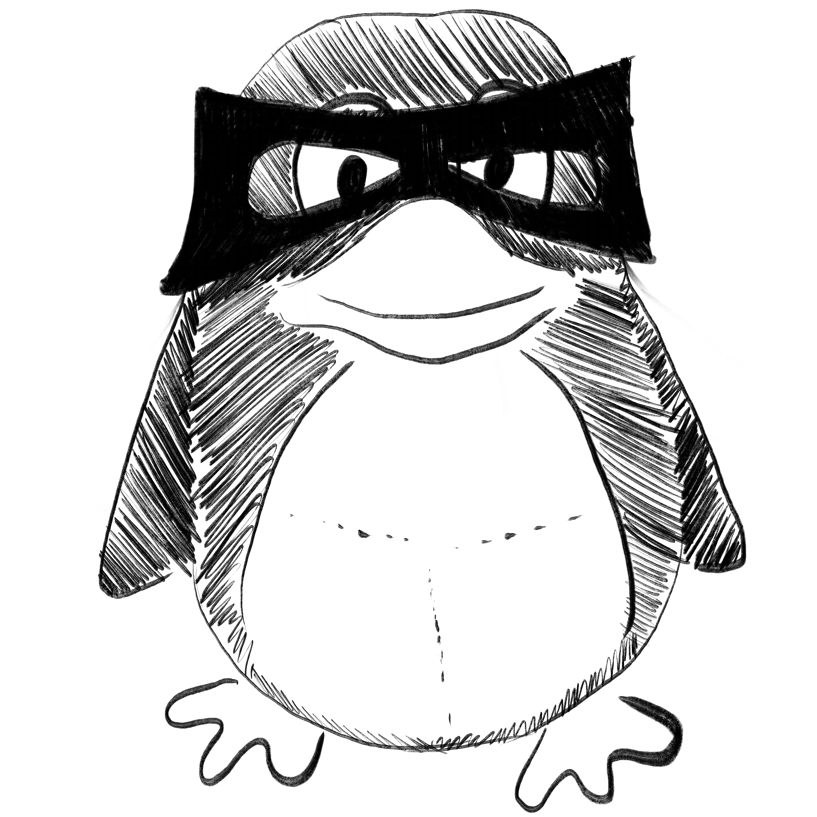
Predicting heart failure onset in the general population using a novel data-mining artificial intelligence method.
In Scientific reports ; h5-index 158.0
Miyashita Yohei, Hitsumoto Tatsuro, Fukuda Hiroki, Kim Jiyoong, Washio Takashi, Kitakaze Masafumi
2023-Mar-16
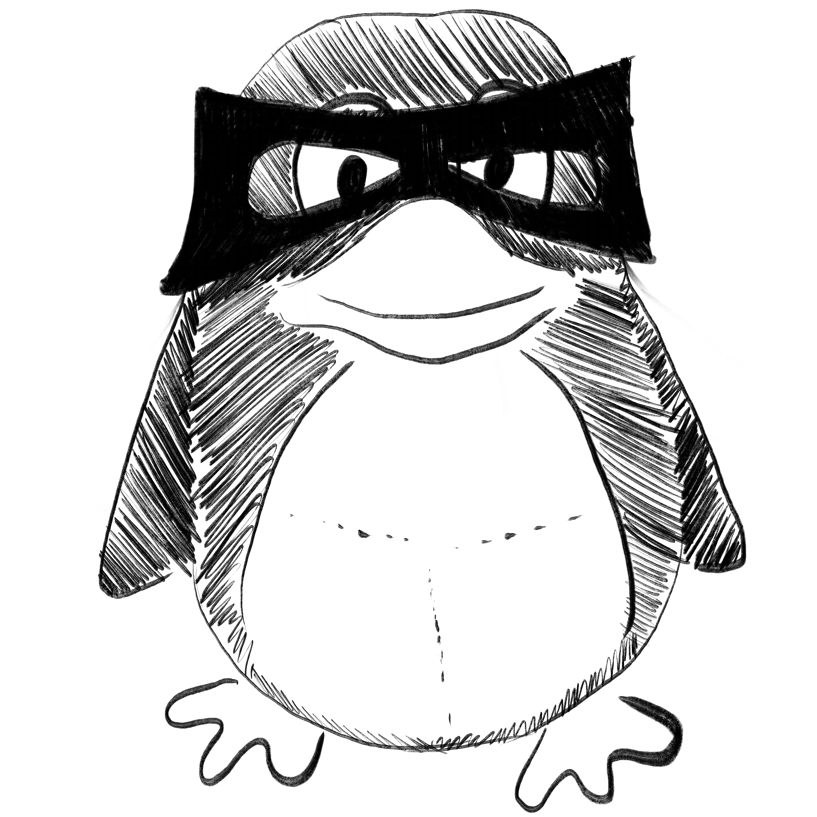
Critically synchronized brain waves form an effective, robust and flexible basis for human memory and learning.
In Scientific reports ; h5-index 158.0
Galinsky Vitaly L, Frank Lawrence R
2023-Mar-16
Weekly Summary
Receive a weekly summary and discussion of the top papers of the week by leading researchers in the field.