Receive a weekly summary and discussion of the top papers of the week by leading researchers in the field.
Improving detection of impacted animal bones on lateral neck radiograph using a deep learning artificial intelligence algorithm.
In Insights into imaging
OBJECTIVE :
METHODS :
RESULTS :
CONCLUSION :
Chen Yueh-Sheng, Luo Sheng-Dean, Lee Chi-Hsun, Lin Jian-Feng, Lin Te-Yen, Ko Sheung-Fat, Yu Chiun-Chieh, Chiang Pi-Ling, Wang Cheng-Kang, Chiu I-Min, Huang Yii-Ting, Tai Yi-Fan, Chiang Po-Teng, Lin Wei-Che
2023-Mar-16
Animal bone impaction, Artificial intelligence, Lateral neck radiograph, Retrospective studies
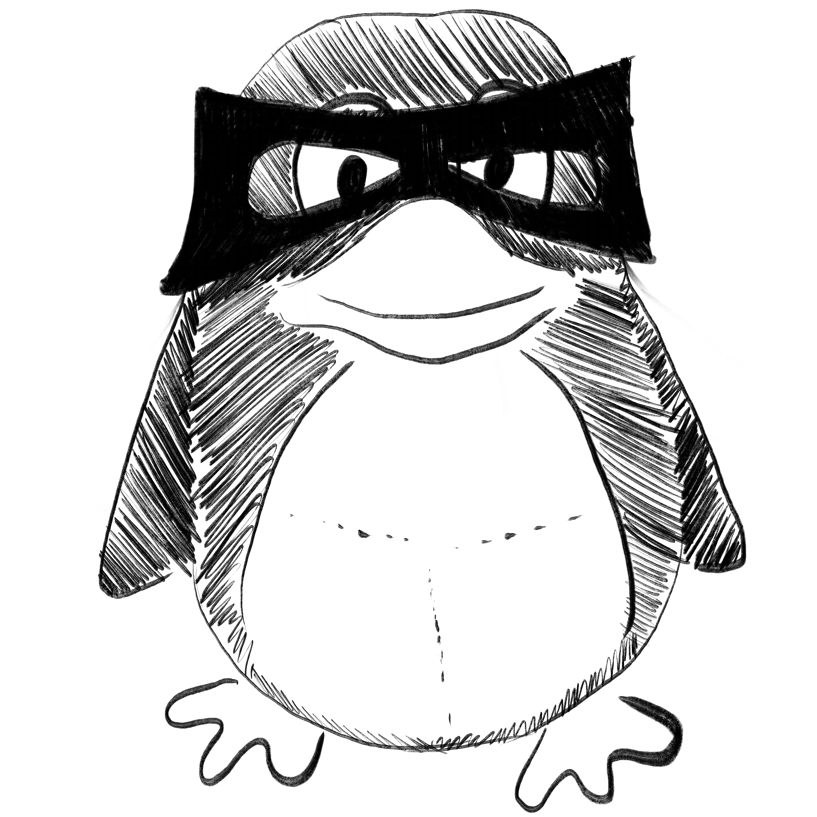
Statistics and Machine Learning in Mass Spectrometry-Based Metabolomics Analysis.
In Methods in molecular biology (Clifton, N.J.)
Fan Sili, Wilson Christopher M, Fridley Brooke L, Li Qian
2023
Imputation, Integrative analysis, Mass spectrometry, Metabolomics, Normalization, Statistical and machine learning
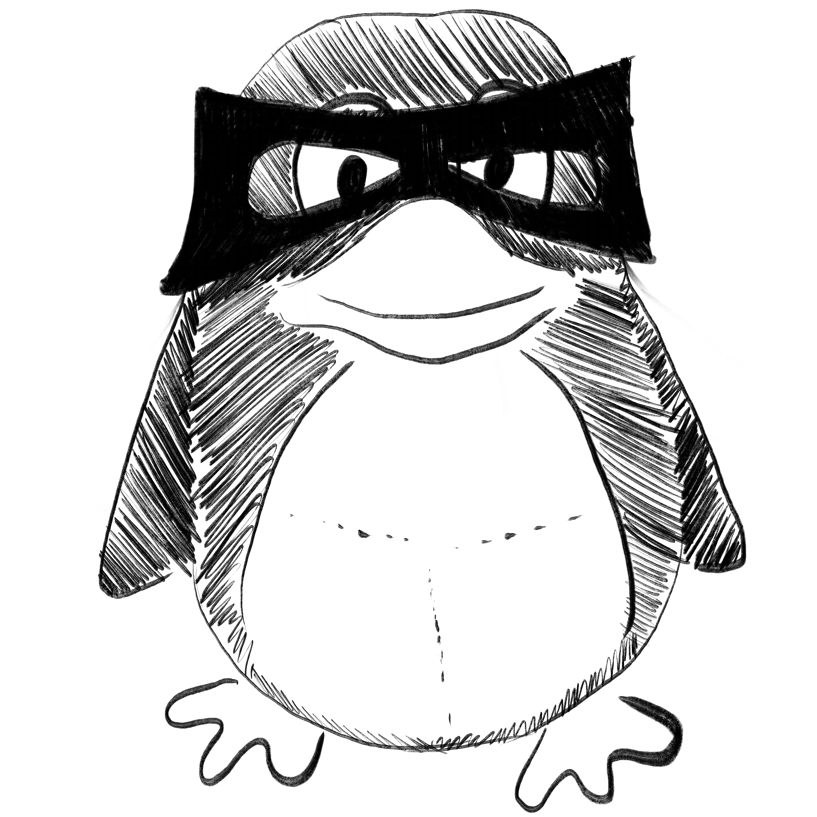
Bioinformatic and Statistical Analysis of Microbiome Data.
In Methods in molecular biology (Clifton, N.J.)
Kim Youngchul
2023
16s rRNA sequencing, Alpha diversity, Beta diversity, Metagenomics, Microbiome, Microbiome-wide association, Phylogeny tree
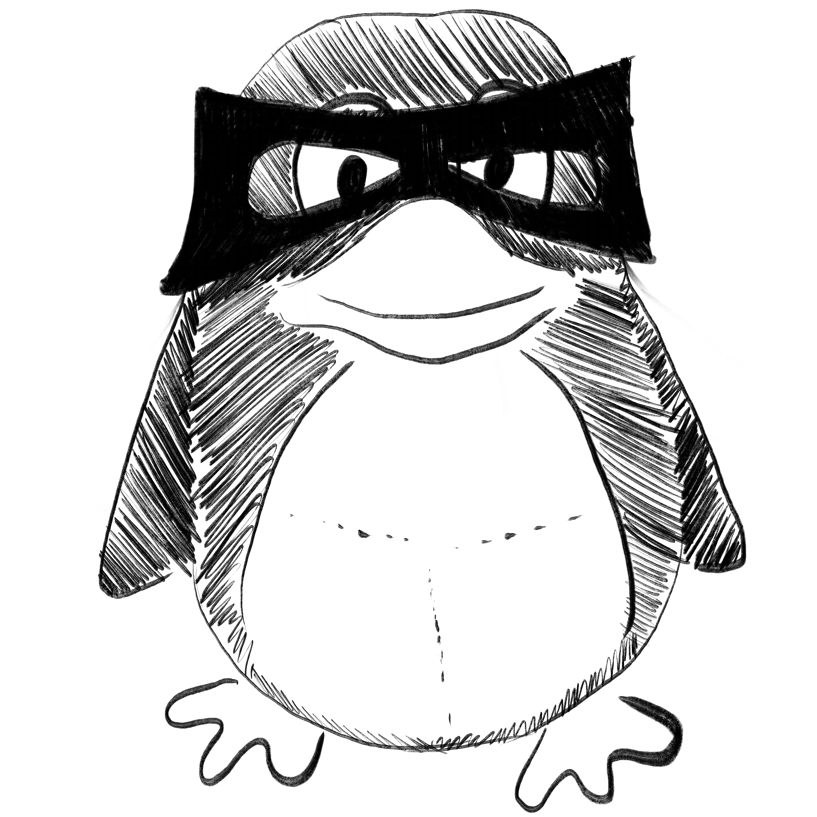
Construction of anoikis-related lncRNAs risk model: Predicts prognosis and immunotherapy response for gastric adenocarcinoma patients.
In Frontiers in pharmacology
Li Qinglin, Zhang Huangjie, Hu Jinguo, Zhang Lizhuo, Zhao Aiguang, Feng He
2023
anoikis, gastric adenocarcinoma, immunotherapy, lncRNAs, prognosis prediction
Profiling Cellular Ecosystems at Single-Cell Resolution and at Scale with EcoTyper.
In Methods in molecular biology (Clifton, N.J.)
Steen ChloƩ B, Luca Bogdan A, Alizadeh Ash A, Gentles Andrew J, Newman Aaron M
2023
Cell states, Ecosystems, Ecotypes, Single-cell RNA sequencing, Spatial transcriptomics, Tissue heterogeneity, Transcriptomics, Tumor microenvironment
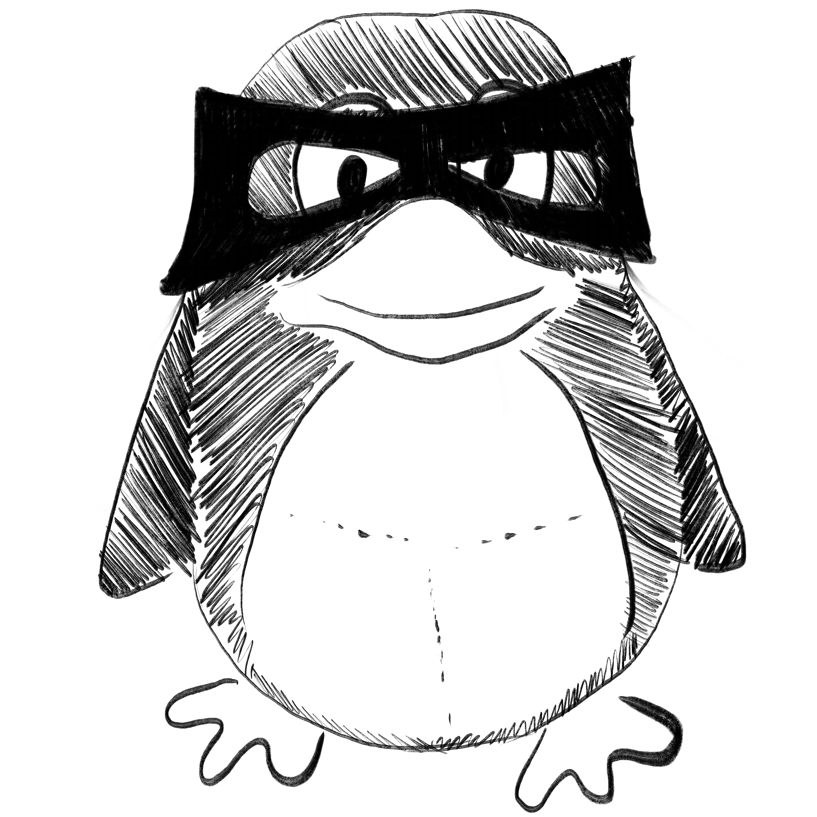
Statistical and Machine Learning Methods for Discovering Prognostic Biomarkers for Survival Outcomes.
In Methods in molecular biology (Clifton, N.J.)
Yao Sijie, Wang Xuefeng
2023
Cox regression, Elastic net, Gradient boosting, Lasso, Machine learning, Survival analysis
Weekly Summary
Receive a weekly summary and discussion of the top papers of the week by leading researchers in the field.