Receive a weekly summary and discussion of the top papers of the week by leading researchers in the field.
Recalibration of a Deep Learning Model for Low-Dose Computed Tomographic Images to Inform Lung Cancer Screening Intervals.
In JAMA network open
IMPORTANCE :
OBJECTIVE :
DESIGN, SETTING, AND PARTICIPANTS :
EXPOSURES :
MAIN OUTCOMES AND MEASURES :
RESULTS :
CONCLUSIONS AND RELEVANCE :
Landy Rebecca, Wang Vivian L, Baldwin David R, Pinsky Paul F, Cheung Li C, Castle Philip E, Skarzynski Martin, Robbins Hilary A, Katki Hormuzd A
2023-Mar-01
Using ChatGPT to evaluate cancer myths and misconceptions: artificial intelligence and cancer information.
In JNCI cancer spectrum
Johnson Skyler B, King Andy J, Warner Echo L, Aneja Sanjay, Kann Benjamin H, Bylund Carma L
2023-Mar-01
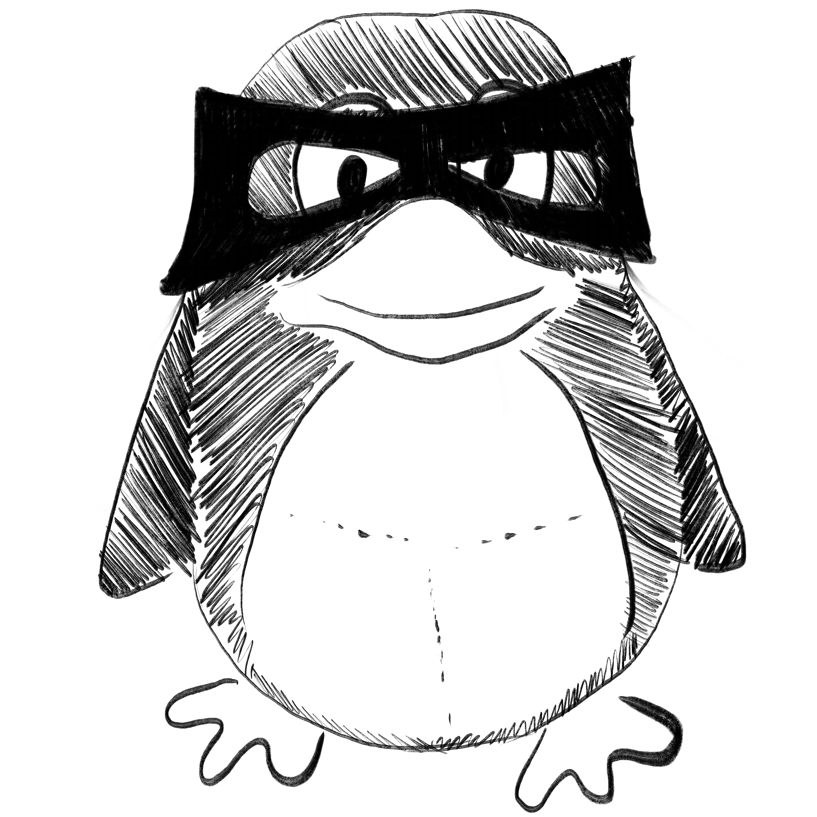
Advanced Temporally-Spatially Precise Technologies for On-Demand Neurological Disorder Intervention.
In Advanced science (Weinheim, Baden-Wurttemberg, Germany)
Chen Xiuli, Gong Yusheng, Chen Wei
2023-Mar-16
clinical translation, neurological disorder, on-demand, temporal-spatial precision
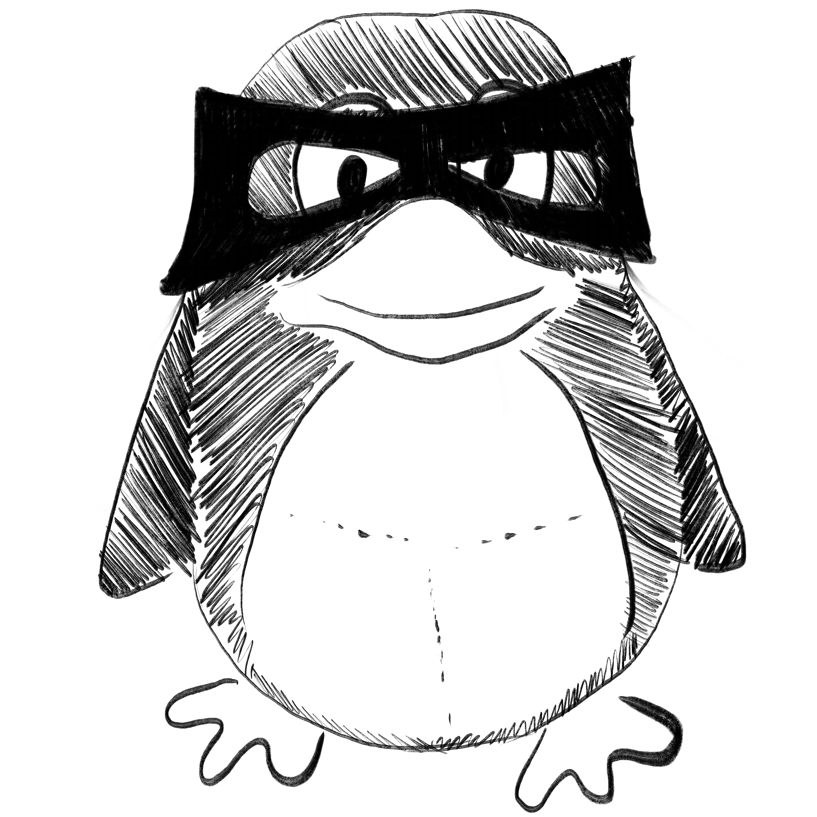
Image-Based Biological Heart Age Estimation Reveals Differential Aging Patterns Across Cardiac Chambers.
In Journal of magnetic resonance imaging : JMRI
BACKGROUND :
PURPOSE :
STUDY TYPE :
POPULATION :
FIELD STRENGTH/SEQUENCE :
ASSESSMENT :
STATISTICAL TEST :
RESULTS :
DATA CONCLUSION :
EVIDENCE LEVEL :
TECHNICAL EFFICACY :
Salih Ahmed M, Pujadas Esmeralda Ruiz, Campello Víctor M, McCracken Celeste, Harvey Nicholas C, Neubauer Stefan, Lekadir Karim, Nichols Thomas E, Petersen Steffen E, Raisi-Estabragh Zahra
2023-Mar-16
aging, cardiac health, cardiac imaging, radiomics
A Multicenter Study on Preoperative Assessment of Lymphovascular Space Invasion in Early-Stage Cervical Cancer Based on Multimodal MR Radiomics.
In Journal of magnetic resonance imaging : JMRI
BACKGROUND :
PURPOSE :
STUDY TYPE :
POPULATION :
FIELD STRENGTH/SEQUENCE :
ASSESSMENT :
STATISTICAL TESTS :
RESULTS :
DATA CONCLUSION :
EVIDENCE LEVEL :
TECHNICAL EFFICACY :
Wu Yu, Wang Shuxing, Chen Yiqing, Liao Yuting, Yin Xuntao, Li Ting, Wang Rui, Luo Xiaomei, Xu Wenchan, Zhou Jing, Wang Simin, Bu Jun, Zhang Xiaochun
2023-Mar-16
cervical cancer, lymphovascular space invasion, machine learning, magnetic resonance imaging, radiomics
Efficient structured reporting in radiology using an intelligent dialogue system based on speech recognition and natural language processing.
In Insights into imaging
BACKGROUND :
RESULTS :
CONCLUSION :
Jorg Tobias, Kämpgen Benedikt, Feiler Dennis, Müller Lukas, Düber Christoph, Mildenberger Peter, Jungmann Florian
2023-Mar-16
Dialogue system, Natural language processing, Speech recognition, Structured reporting
Weekly Summary
Receive a weekly summary and discussion of the top papers of the week by leading researchers in the field.