Receive a weekly summary and discussion of the top papers of the week by leading researchers in the field.
A hybrid model- and deep learning-based framework for functional lung image synthesis from multi-inflation CT and hyperpolarized gas MRI.
In Medical physics ; h5-index 59.0
BACKGROUND :
PURPOSE :
METHODS :
RESULTS :
CONCLUSIONS :
Astley Joshua R, Biancardi Alberto M, Marshall Helen, Hughes Paul J C, Collier Guilhem J, Hatton Matthew Q, Wild Jim M, Tahir Bilal A
2023-Mar-17
18 Claremont crescent, POLARIS, S10 2TA, Sheffield, United Kingdom
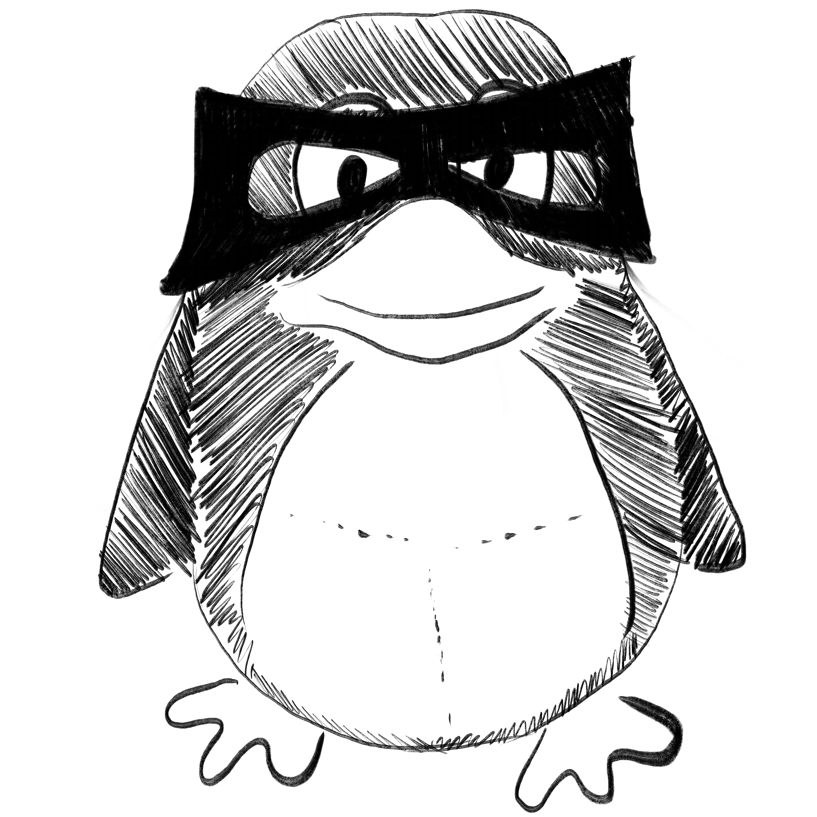
Multi-task adaptive pooling enabled synergetic learning of RNA modification across tissue, type and species from low-resolution epitranscriptomes.
In Briefings in bioinformatics
Song Yiyou, Wang Yue, Wang Xuan, Huang Daiyun, Nguyen Anh, Meng Jia
2023-Mar-17
ConvMixer, RNA modification, adaptive pooling, low-resolution epitranscriptomes, multi-task learning, transformer
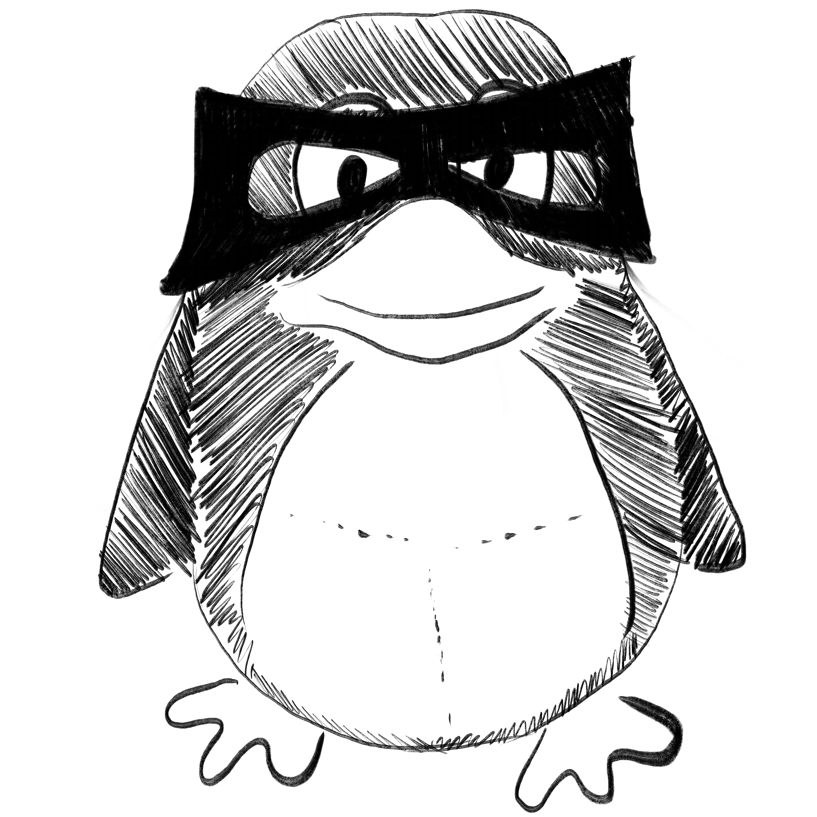
Identification of FCN1 as a novel macrophage infiltration-associated biomarker for diagnosis of pediatric inflammatory bowel diseases.
In Journal of translational medicine
BACKGROUND :
METHODS :
RESULTS :
CONCLUSIONS :
Chen Xixi, Gao Yuanqi, Xie Jinfang, Hua Huiying, Pan Chun, Huang Jiebin, Jing Mengxia, Chen Xuehua, Xu Chundi, Gao Yujing, Li Pu
2023-Mar-17
Diagnostic biomarkers, FCN1, Inflammation, Macrophages, Pediatric inflammatory bowel disease
Effect of Dataset Size and Medical Image Modality on Convolutional Neural Network Model Performance for Automated Segmentation: A CT and MR Renal Tumor Imaging Study.
In Journal of digital imaging
Gottlich Harrison C, Gregory Adriana V, Sharma Vidit, Khanna Abhinav, Moustafa Amr U, Lohse Christine M, Potretzke Theodora A, Korfiatis Panagiotis, Potretzke Aaron M, Denic Aleksandar, Rule Andrew D, Takahashi Naoki, Erickson Bradley J, Leibovich Bradley C, Kline Timothy L
2023-Mar-17
Deep learning, Kidney, Machine learning model performance, Nephrectomy, Semantic segmentation, Similarity metrics
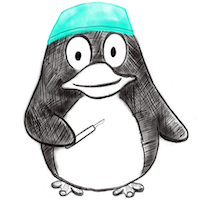
Surgery or comfort care for neonates with surgical necrotizing enterocolitis: Lessons learned from behavioral artificial intelligence technology.
In Frontiers in pediatrics
BACKGROUND :
METHODS :
RESULTS :
CONCLUSION :
van Varsseveld Otis C, Ten Broeke Annebel, Chorus Caspar G, Heyning Nicolaas, Kooi Elisabeth M W, Hulscher Jan B F
2023
artificial intelligence, choice analysis, comfort care, critical care, decision making, decision support, necrotizing enterocolitis
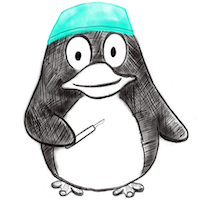
Non-invasive estimation of muscle fibre size from high-density electromyography.
In The Journal of physiology ; h5-index 67.0
Casolo Andrea, Maeo Sumiaki, Balshaw Thomas G, Lanza Marcel B, Martin Neil R W, Nuccio Stefano, Moro Tatiana, Paoli Antonio, Felici Francesco, Maffulli Nicola, Eskofier Bjoern, Kinfe Thomas M, Folland Jonathan P, Farina Dario, Vecchio Alessandro Del
2023-Mar-16
Motor unit, conduction velocity, high-density surface electromyography, muscle fibre size
Weekly Summary
Receive a weekly summary and discussion of the top papers of the week by leading researchers in the field.