Receive a weekly summary and discussion of the top papers of the week by leading researchers in the field.
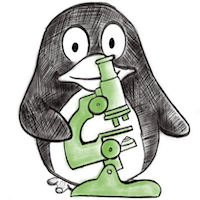
Renal enhanced CT images reveal the tandem mechanism between tumor cells and immunocytes based on bulk/single-cell RNA sequencing.
In Functional & integrative genomics
Liang Haote, Wu Keming, Wu Rongrong, Huang KaTe, Deng Zhexian, Chen Hongde
2023-Mar-18
Clear cell renal carcinoma, Enhanced CT images, Metabolic reprogramming, Single-cell RNA sequencing, Tumor microenvironment
Improved Decision Making for Water Lead Testing in U.S. Child Care Facilities Using Machine-Learned Bayesian Networks.
In Environmental science & technology ; h5-index 132.0
Mulhern Riley E, Kondash A J, Norman Ed, Johnson Joseph, Levine Keith, McWilliams Andrea, Napier Melanie, Weber Frank, Stella Laurie, Wood Erica, Lee Pow Jackson Crystal, Colley Sarah, Cajka Jamie, MacDonald Gibson Jacqueline, Hoponick Redmon Jennifer
2023-Mar-18
children’s health, drinking water, lead, machine learning, risk assessment
Prediction of Renal Function 1 Year After Transplantation Using Machine Learning Methods Based on Ultrasound Radiomics Combined With Clinical and Imaging Features.
In Ultrasonic imaging
Zhu Lili, Huang Renjun, Zhou Zhiyong, Fan Qingmin, Yan Junchen, Wan Xiaojing, Zhao Xiaojun, He Yao, Dong Fenglin
2023-Mar-18
chronic kidney disease, kidney transplantation, machine learning, radiomics, ultrasound
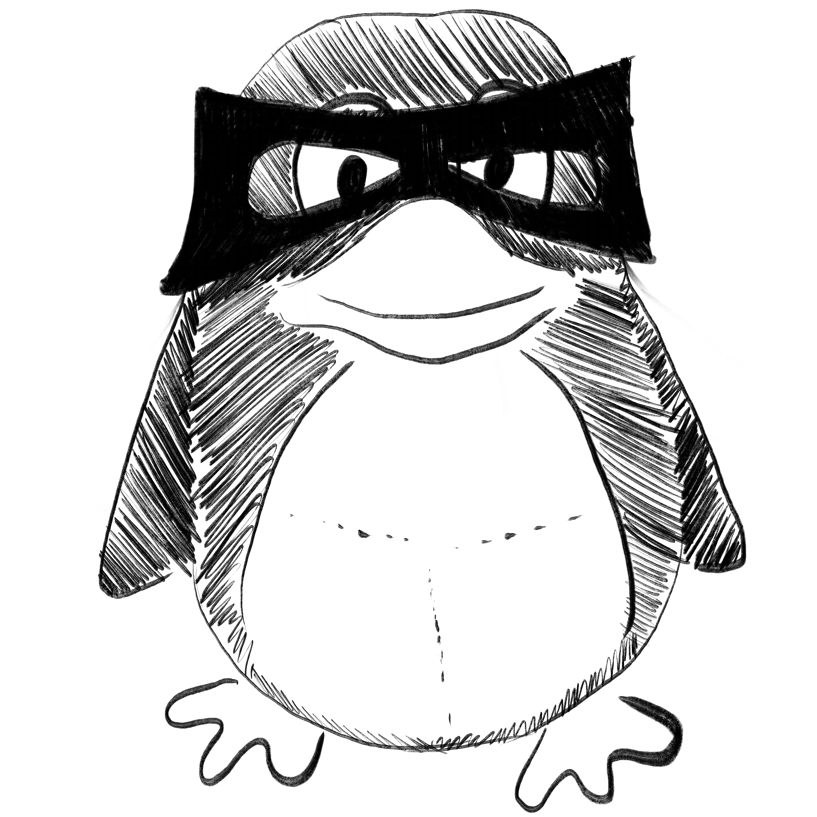
Nonparametric failure time: Time-to-event machine learning with heteroskedastic bayesian additive regression trees and low information omnibus dirichlet process mixtures.
In Biometrics
Sparapani R A, Logan B R, Maiers M J, Laud P W, McCulloch R E
2023-Mar-18
Accelerated failure time, BART, LIO prior hierarchy, Thompson sampling variable selection, constrained DPM, hematopoietic stem cell transplant, non-proportional hazards, survival analysis
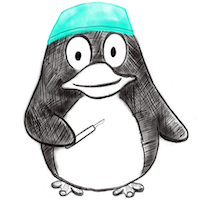
Can a Novel Natural Language Processing Model and Artificial Intelligence Automatically Generate Billing Codes From Spine Surgical Operative Notes?
In Global spine journal
STUDY DESIGN :
OBJECTIVE :
METHODS :
RESULTS :
CONCLUSIONS :
Zaidat Bashar, Tang Justin, Arvind Varun, Geng Eric A, Cho Brian, Duey Akiro H, Dominy Calista, Riew Kiehyun D, Cho Samuel K, Kim Jun S
2023-Mar-18
ACDF, PCDF, artificial intelligence, cervical, disc replacement, fusion, natural language processing
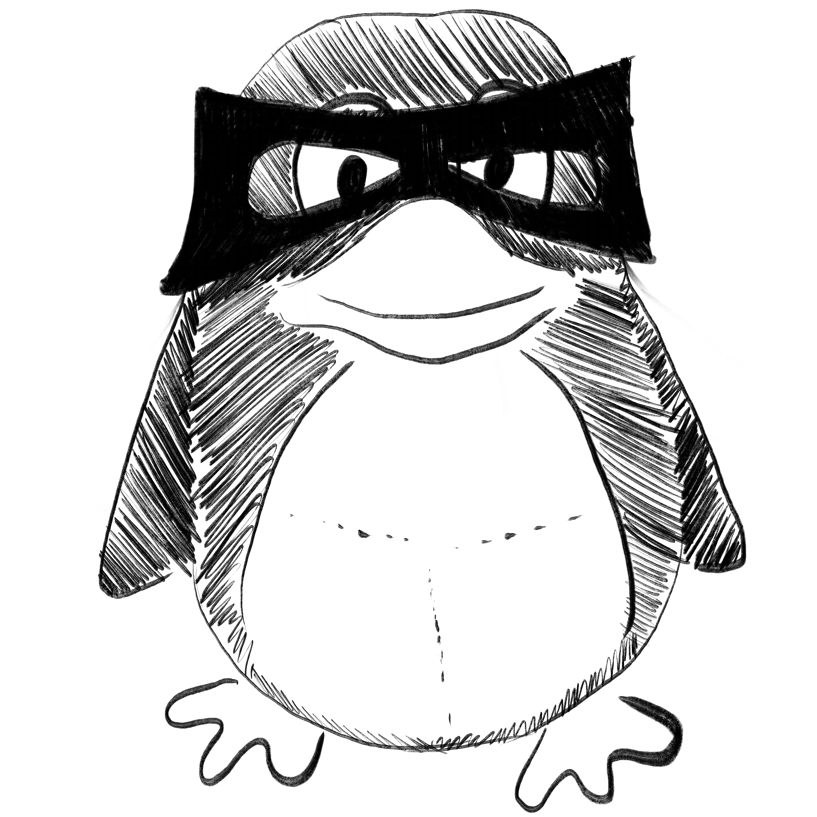
Higher-order structure formation using refined monomer structures of lipid raft markers, Stomatin, Prohibitin, Flotillin, and HflK/C-related proteins.
In FEBS open bio
Yokoyama Hideshi, Matsui Ikuo
2023-Mar-17
ColabFold: AlphaFold2, SPFH, ab initio docking, lipid rafts, membrane protease, stomatin specific protease
Weekly Summary
Receive a weekly summary and discussion of the top papers of the week by leading researchers in the field.