Receive a weekly summary and discussion of the top papers of the week by leading researchers in the field.
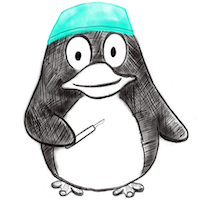
The evolution of facial reanimation techniques.
In American journal of otolaryngology ; h5-index 23.0
Pan Debbie R, Clark Nicholas W, Chiang Harry, Kahmke Russel R, Phillips Brett T, Barrett Dane M
2023-Mar-01
Facial paralysis, Facial reanimation, Free muscle transfer
A new method for predicting the prognosis of ischemic stroke based vascular structure features and lesion location features.
In Clinical imaging
OBJECTIVE :
METHODS :
RESULTS :
CONCLUSION :
Weng Suiqing, Sun Xilin, Wang Hao, Song Bin, Zhu Jie
2023-Mar-15
Machine learning, Prognosis prediction, Stroke, Stroke location, Vascular structure
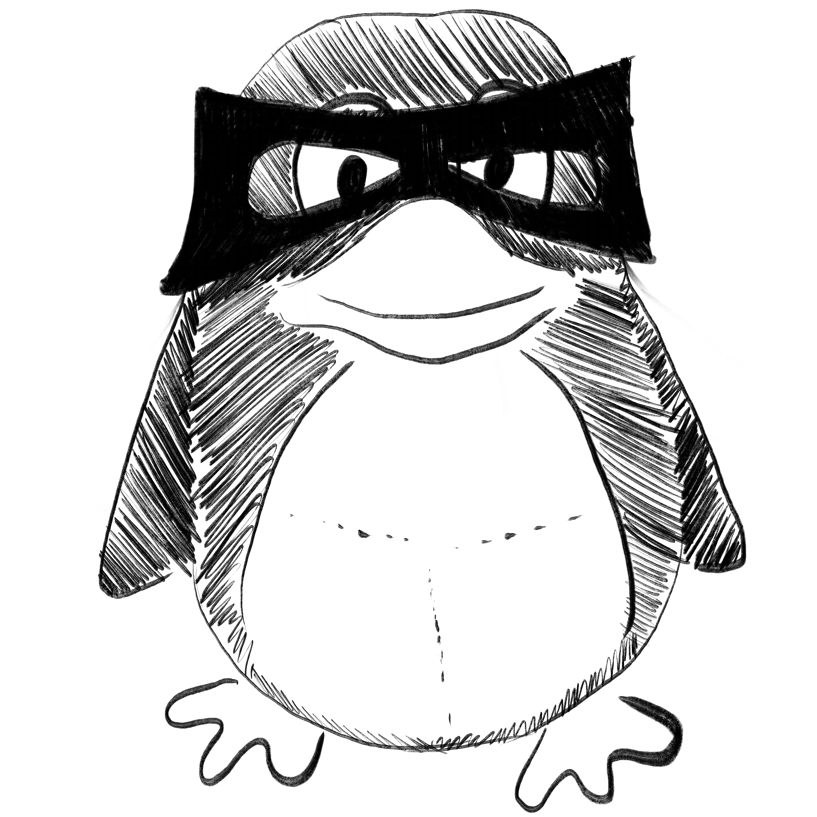
Characterization of micropollutants in urban stormwater using high-resolution monitoring and machine learning.
In Water research
Yun Daeun, Kang Daeho, Cho Kyung Hwa, Baek Sang-Soo, Jeon Junho
2023-Mar-12
First flush effects, Hierarchical clustering analysis, High-resolution mass spectrometry, Micropollutant, Partial least squares regression
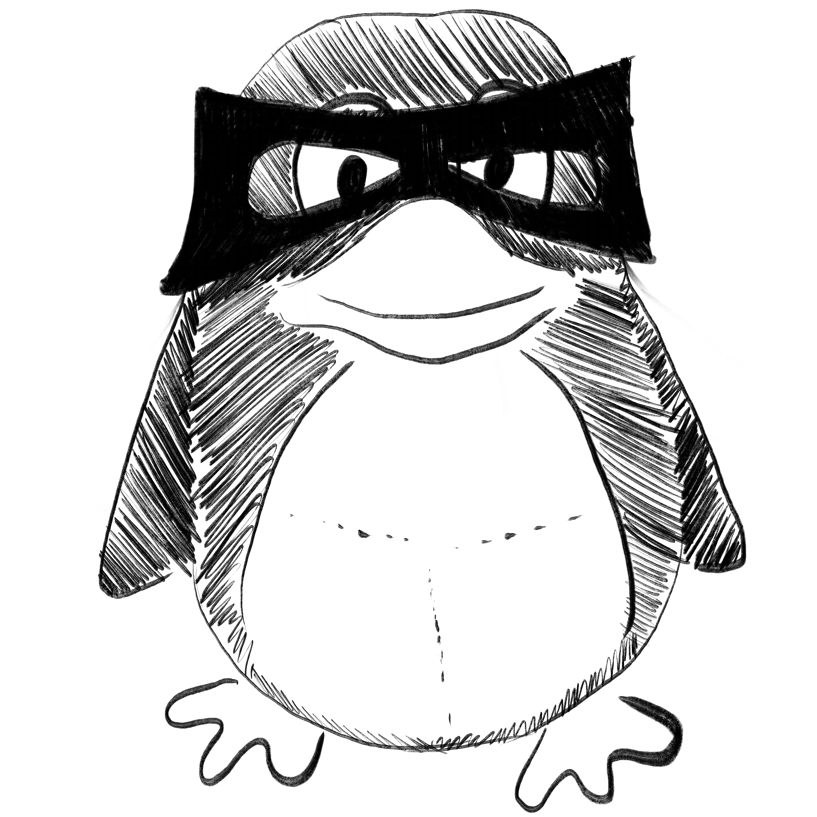
Two-Branch network for brain tumor segmentation using attention mechanism and super-resolution reconstruction.
In Computers in biology and medicine
Jia Zhaohong, Zhu Hongxin, Zhu Junan, Ma Ping
2023-Mar-15
3D U-Net, Attention mechanism, Brain tumor segmentation, MRI, Shared encoder, Super-resolution image reconstruction
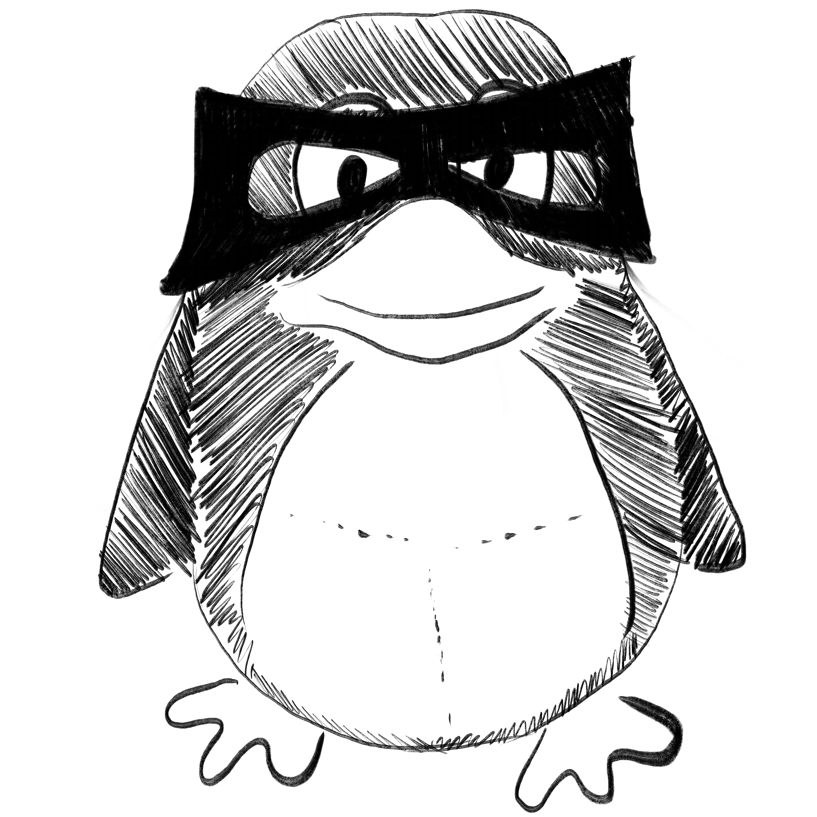
PRA-Net: Part-and-Relation Attention Network for depression recognition from facial expression.
In Computers in biology and medicine
Liu Zhenyu, Yuan Xiaoyan, Li Yutong, Shangguan Zixuan, Zhou Li, Hu Bin
2023-Jan-24
Automatic depression detection, End-to-end network, Facial expression, Part features, Relation attention
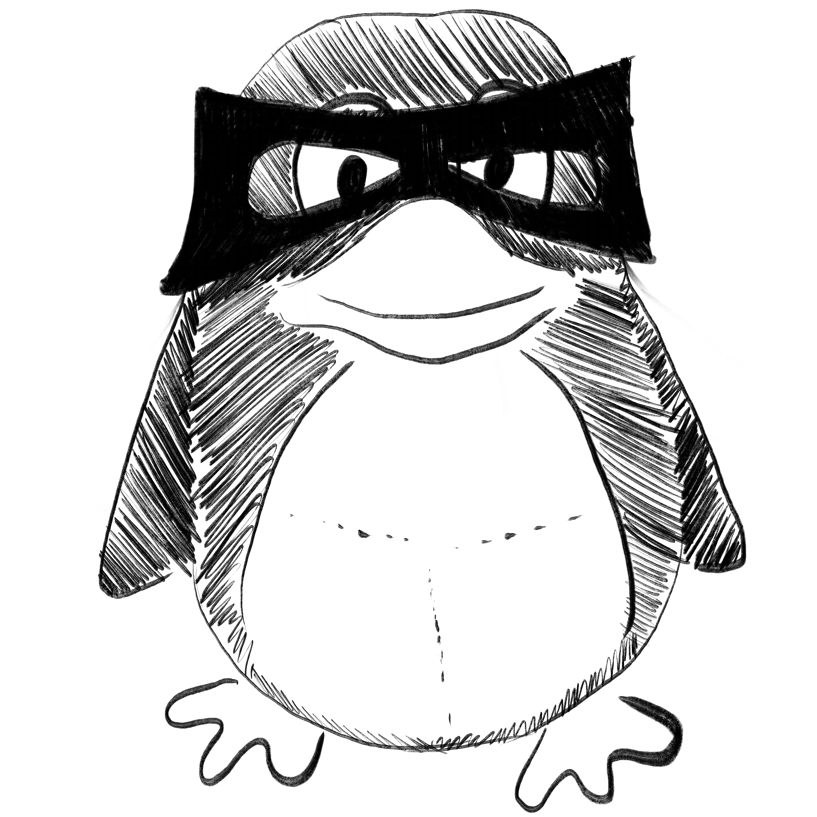
Measuring water holding capacity in pork meat images using deep learning.
In Meat science
de Sousa Reis Vinicius Clemente, Ferreira Isaura Maria, Durval Mariah Castro, Antunes Robson Carlos, Backes Andre Ricardo
2023-Mar-16
Computer vision system, Semantic image segmentation, Water holding capacity
Weekly Summary
Receive a weekly summary and discussion of the top papers of the week by leading researchers in the field.