Receive a weekly summary and discussion of the top papers of the week by leading researchers in the field.
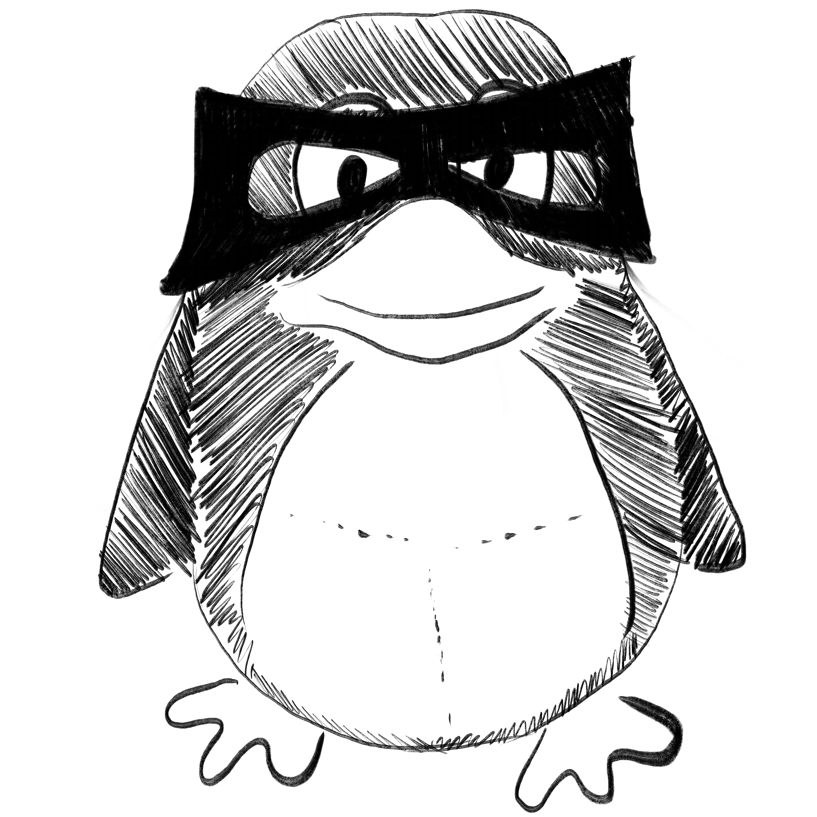
WASCO: A Wasserstein-based statistical tool to compare conformational ensembles of intrinsically disordered proteins.
In Journal of molecular biology ; h5-index 65.0
González-Delgado Javier, Sagar Amin, Zanon Christophe, Lindorff-Larsen Kresten, Bernadó Pau, Neuvial Pierre, Cortés Juan
2023-Mar-17
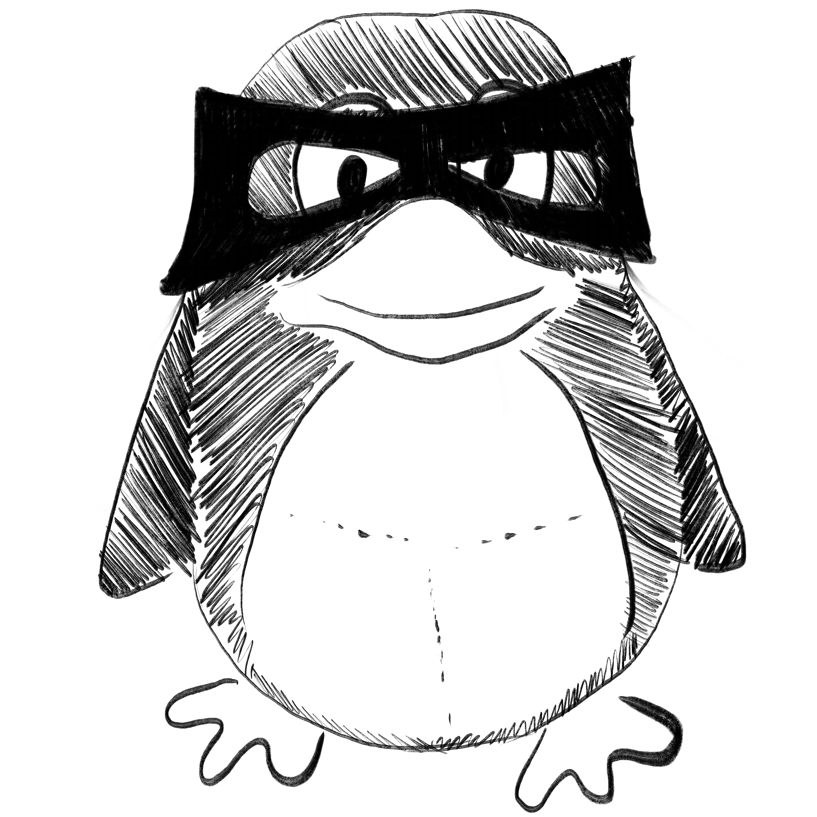
Fully nondestructive analysis of capsaicinoids electrochemistry data with deep neural network enables portable system.
In Food chemistry
Xing Zheng, Jiang Ying, Zogona Daniel, Wu Ting, Xu Xiaoyun
2023-Mar-07
Capsaicinoids, Deep learning, Portable platform, Quantitative, Sensor arrays
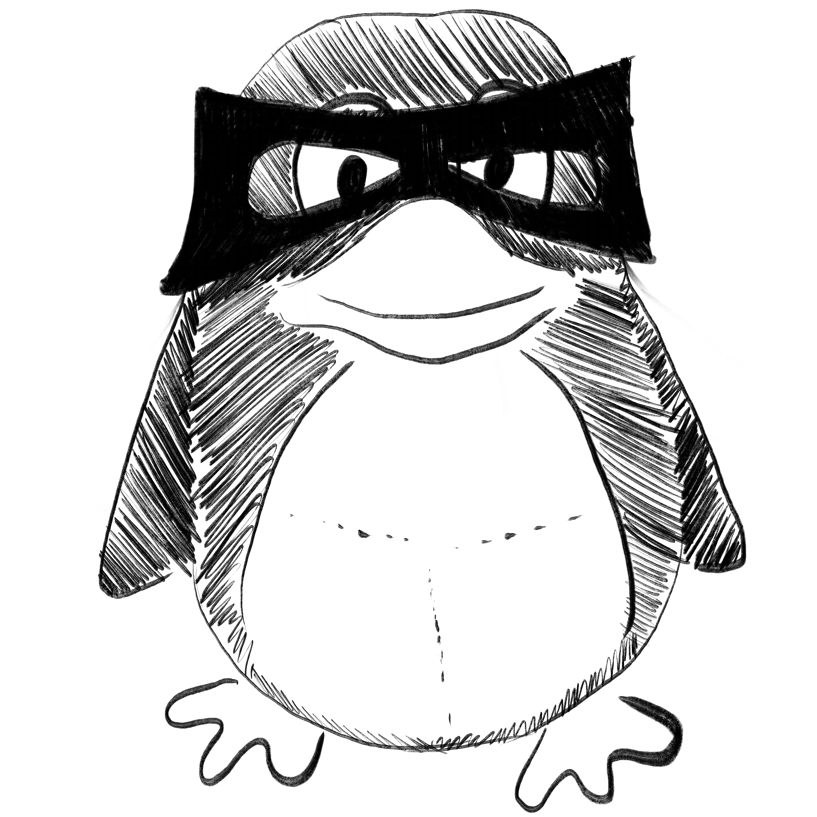
Global synchronization of complex-valued neural networks with unbounded time-varying delays.
In Neural networks : the official journal of the International Neural Network Society
Sheng Yin, Gong Haoyu, Zeng Zhigang
2023-Mar-06
Complex-valued neural networks, Global synchronization, Unbounded time-varying delays
Artificial intelligence for secondary prevention of myocardial infarction: A qualitative study of patient and health professional perspectives.
In International journal of medical informatics ; h5-index 49.0
BACKGROUND :
PURPOSE :
PROCEDURE :
FINDINGS :
CONCLUSIONS :
Pelly Melissa, Fatehi Farhad, Liew Danny, Verdejo-Garcia Antonio
2023-Mar-14
Artificial intelligence, Co-design, Myocardial infarction, Perspectives, Qualitative study, Secondary prevention
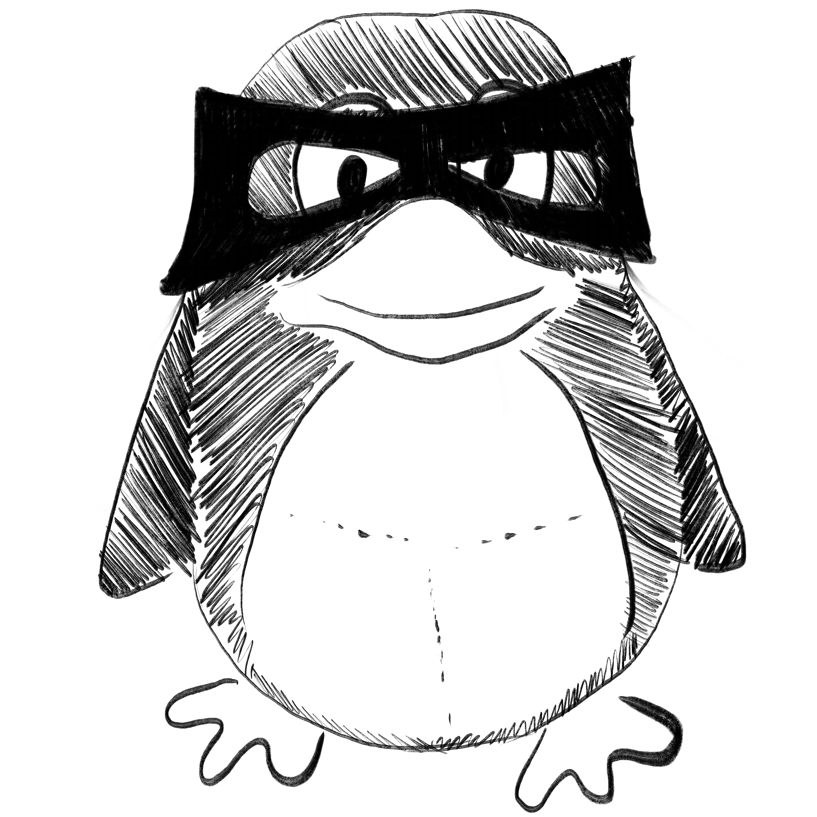
Deep learning pose estimation for multi-cattle lameness detection.
In Scientific reports ; h5-index 158.0
Barney Shaun, Dlay Satnam, Crowe Andrew, Kyriazakis Ilias, Leach Matthew
2023-Mar-18
Cascaded Latent Diffusion Models for High-Resolution Chest X-ray Synthesis
ArXiv Preprint
Tobias Weber, Michael Ingrisch, Bernd Bischl, David Rügamer
2023-03-20
Weekly Summary
Receive a weekly summary and discussion of the top papers of the week by leading researchers in the field.