Receive a weekly summary and discussion of the top papers of the week by leading researchers in the field.
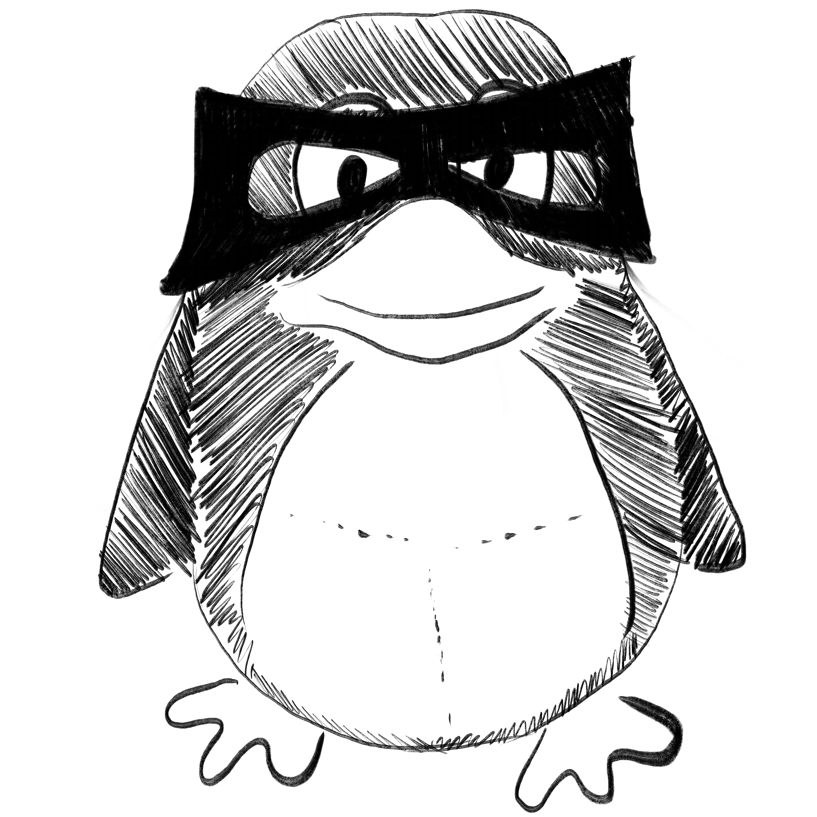
DeepMiceTL: a deep transfer learning based prediction of mice cardiac conduction diseases using early electrocardiograms.
In Briefings in bioinformatics
Liao Ying, Xiang Yisha, Zheng Mingjie, Wang Jun
2023-Mar-18
Bayesian optimization, cardiac conduction disease, deep transfer learning, electrocardiogram (ECG), mouse model
Overinterpretation of findings in machine learning prediction model studies in oncology: a systematic review.
In Journal of clinical epidemiology ; h5-index 60.0
BACKGROUND :
STUDY DESIGN AND SETTING :
RESULTS :
CONCLUSION :
Dhiman Paula, Ma Jie, Andaur Navarro Constanza L, Speich Benjamin, Bullock Garrett, Damen Johanna Aa, Hooft Lotty, Kirtley Shona, Riley Richard D, Van Calster Ben, Moons Karel Gm, Collins Gary S
2023-Mar-17
machine learning, prediction model, spin
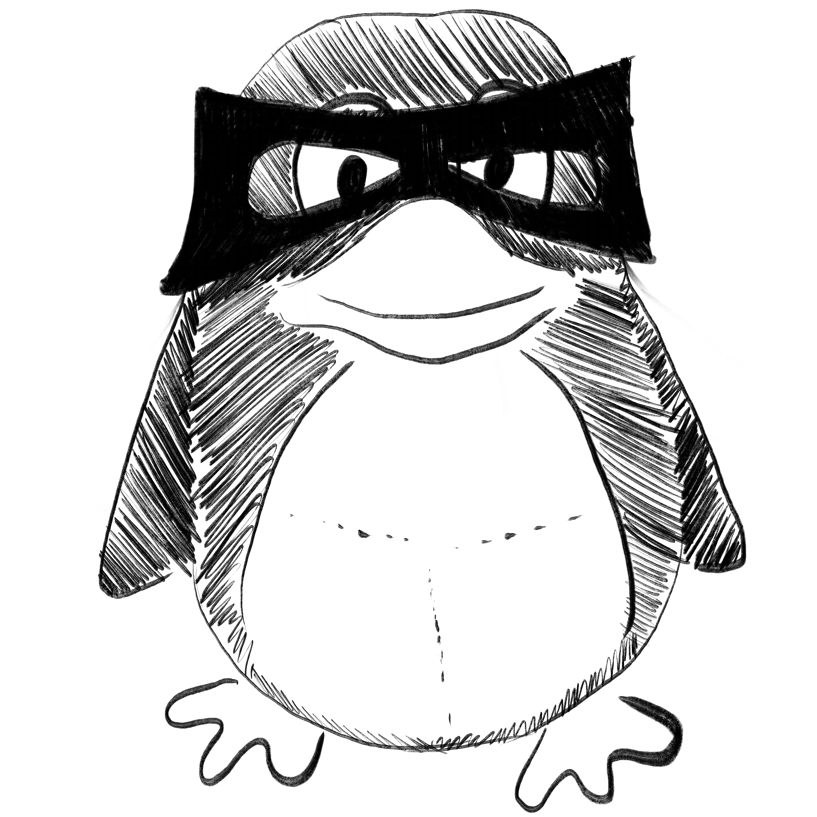
Utilizing Shared Frailty with the Cox Proportional Hazards Regression: Post Discharge Survival Analysis of CHF Patients.
In Journal of biomedical informatics ; h5-index 55.0
Ben-Assuli Ofir, Ramon-Gonen Roni, Heart Tsipi, Jacobi Arie, Klempfner Robert
2023-Mar-17
Cox proportional hazards regression, congestive heart failure, shared frailty, statistical methods, survival analysis
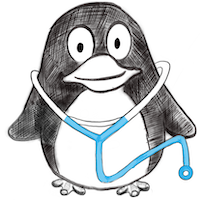
Representing and Utilizing Clinical Textual Data for Real World Studies: An OHDSI Approach.
In Journal of biomedical informatics ; h5-index 55.0
Keloth Vipina K, Banda Juan M, Gurley Michael, Heider Paul M, Kennedy Georgina, Liu Hongfang, Liu Feifan, Miller Timothy, Natarajan Karthik, V Patterson Olga, Peng Yifan, Raja Kalpana, Reeves Ruth M, Rouhizadeh Masoud, Shi Jianlin, Wang Xiaoyan, Wang Yanshan, Wei Wei-Qi, Williams Andrew E, Zhang Rui, Belenkaya Rimma, Reich Christian, Blacketer Clair, Ryan Patrick, Hripcsak George, Elhadad NoƩmie, Xu Hua
2023-Mar-17
Electronic health records, Natural language processing, Real-world study
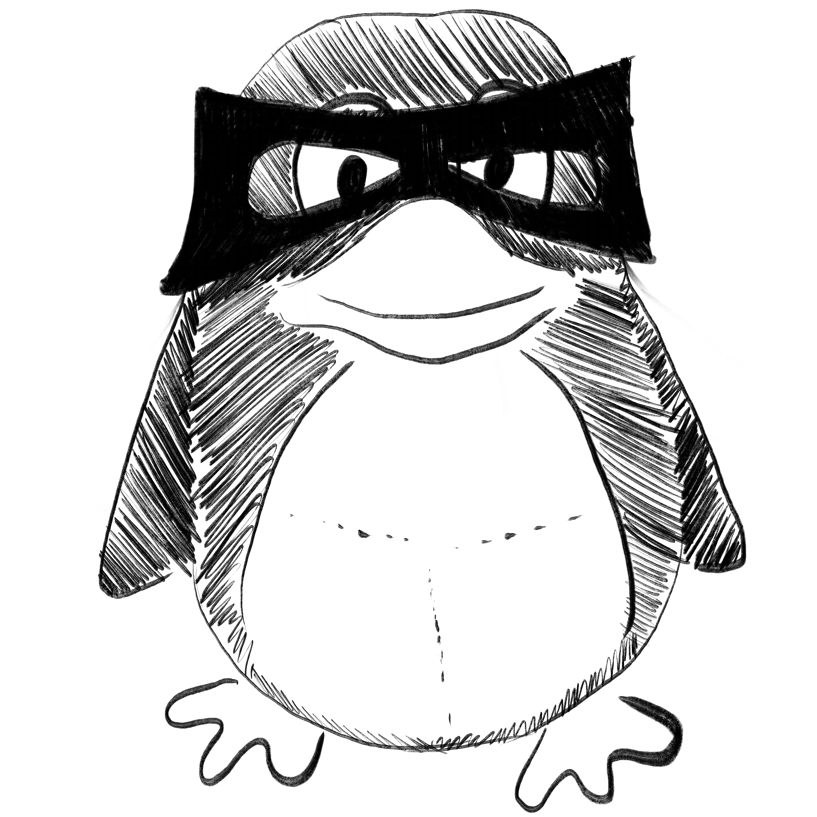
A deep learning-based novel approach to generate continuous daily stream nitrate concentration for nitrate data-sparse watersheds.
In The Science of the total environment
Saha Gourab, Rahmani Farshid, Shen Chaopeng, Li Li, Raj Cibin
2023-Mar-17
C-Q relationship, Deep learning, LSTM, Machine learning, Stream nitrate modeling, Water quality
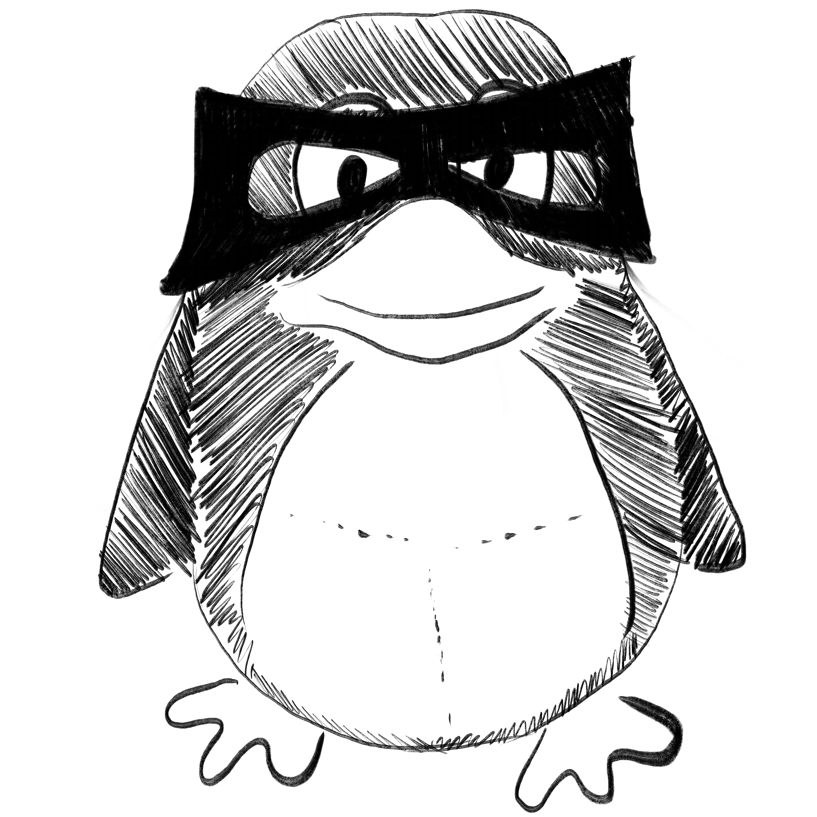
Assessment of removal rate coefficient in vertical flow constructed wetland employing machine learning for low organic loaded systems.
In Bioresource technology
Soti Abhishek, Singh Saurabh, Verma Vishesh, Mohan Kulshreshtha Niha, Brighu Urmila, Kalbar Pradip, Bhushan Gupta Akhilendra
2023-Mar-17
Kikuth kinetic approach, low organic loading vertical flow constructed wetland, remediation efficiency, removal rate coefficient
Weekly Summary
Receive a weekly summary and discussion of the top papers of the week by leading researchers in the field.