Receive a weekly summary and discussion of the top papers of the week by leading researchers in the field.
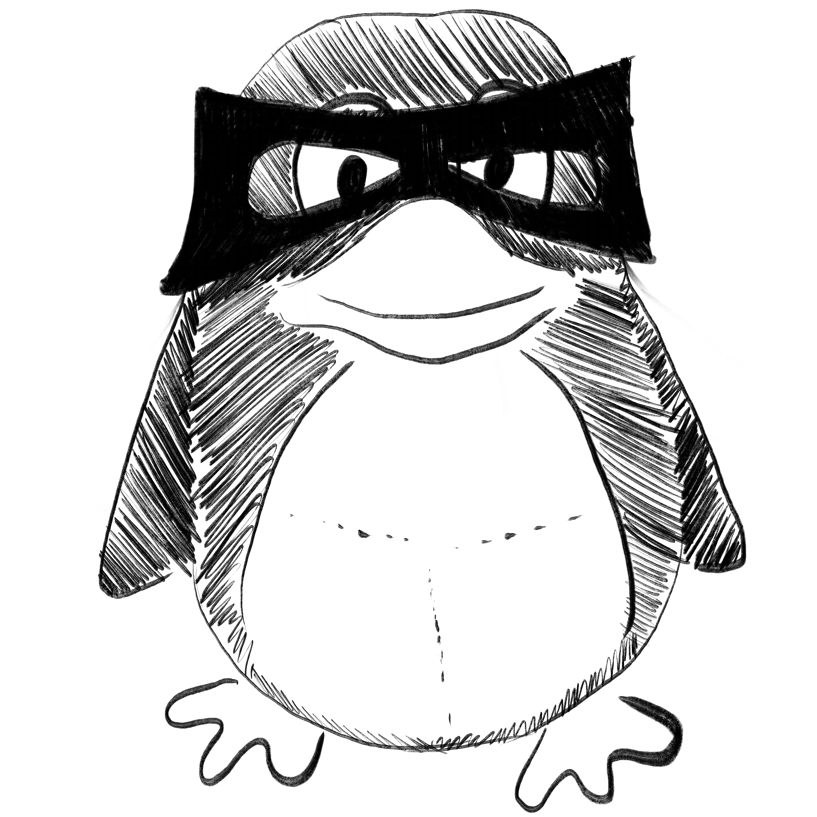
Machine learning and density functional theory simulation of the electronic structural properties for novel quaternary semiconductors.
In Physical chemistry chemical physics : PCCP
Gao Mengwei, Cai Bo, Liu Gaoyu, Xu Lili, Zhang Shengli, Zeng Haibo
2023-Mar-20
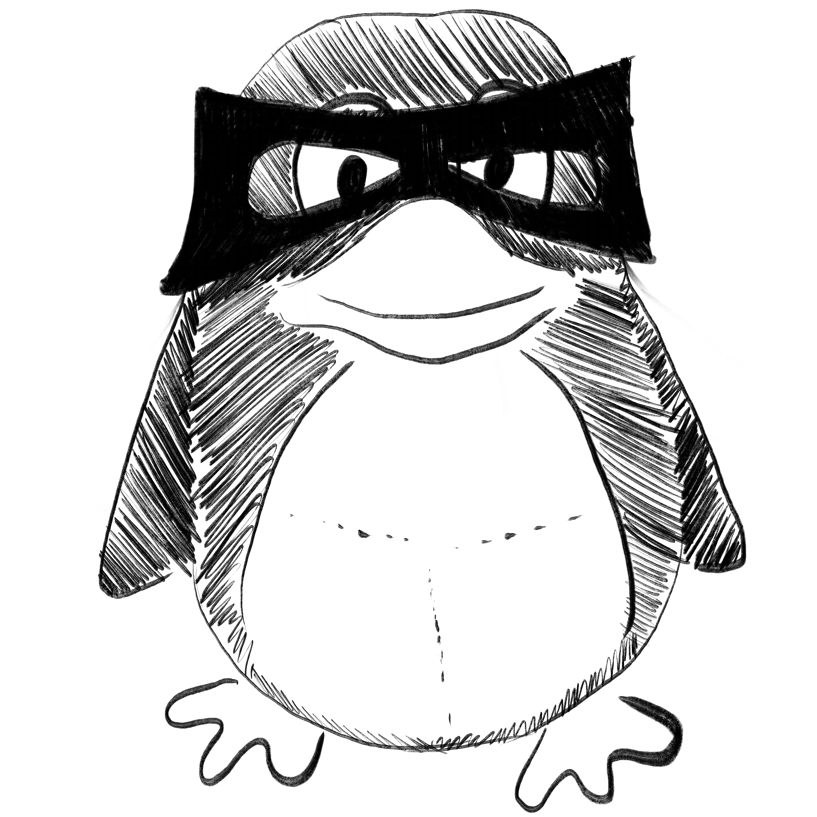
Are emerging technologies unlocking the potential of sustainable practices in the context of a net-zero economy? An analysis of driving forces.
In Environmental science and pollution research international
Agrawal Rohit, Priyadarshinee Pragati, Kumar Anil, Luthra Sunil, Garza-Reyes Jose Arturo, Kadyan Sneha
2023-Mar-18
Circular economy, Drivers, Emerging technologies, Net-zero economy, Pythagorean fuzzy DEMATEL, Sustainable practices
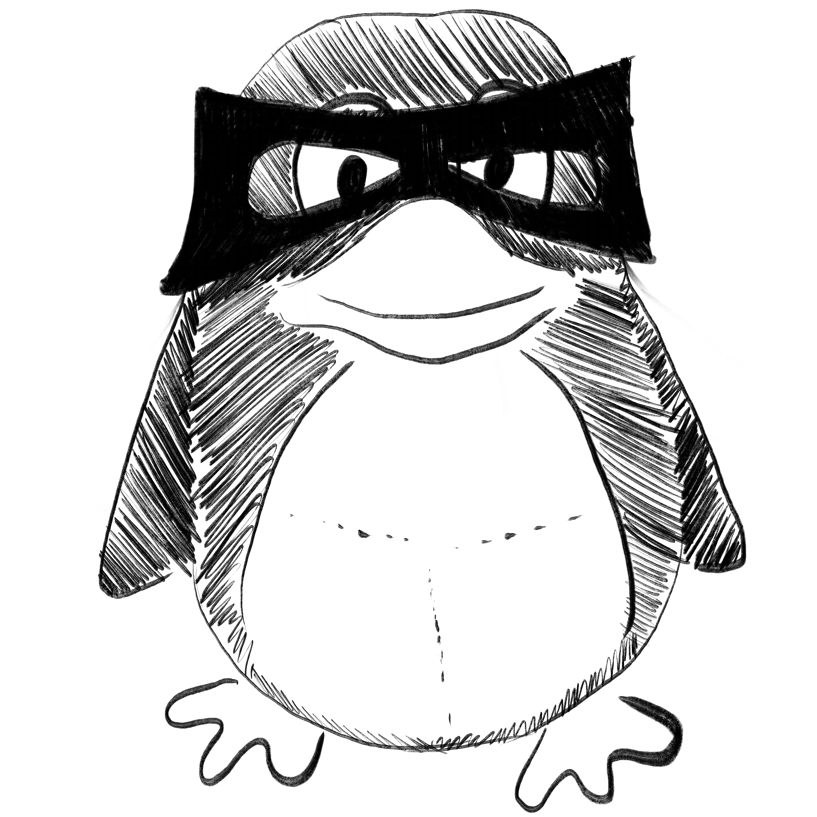
Optimization of biocementation responses by artificial neural network and random forest in comparison to response surface methodology.
In Environmental science and pollution research international
Pacheco Vinicius Luiz, Bragagnolo Lucimara, Dalla Rosa Francisco, Thomé Antonio
2023-Mar-18
Artificial neural networks, Cross-validation, MICP, Random forest, Response surface method
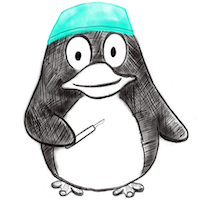
Semantic segmentation of surgical hyperspectral images under geometric domain shifts
ArXiv Preprint
Jan Sellner, Silvia Seidlitz, Alexander Studier-Fischer, Alessandro Motta, Berkin Özdemir, Beat Peter Müller-Stich, Felix Nickel, Lena Maier-Hein
2023-03-20
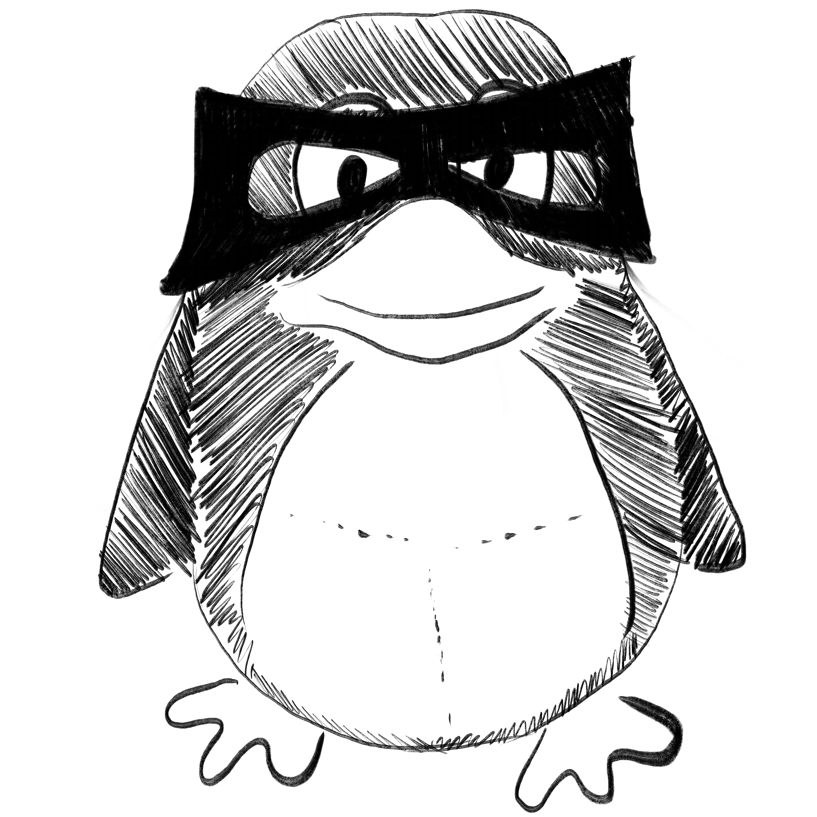
Lightweight saliency detection method for real-time localization of livestock meat bones.
In Scientific reports ; h5-index 158.0
Xu Tao, Zhao Weishuo, Cai Lei, Shi Xiaoli, Wang Xinfa
2023-Mar-18
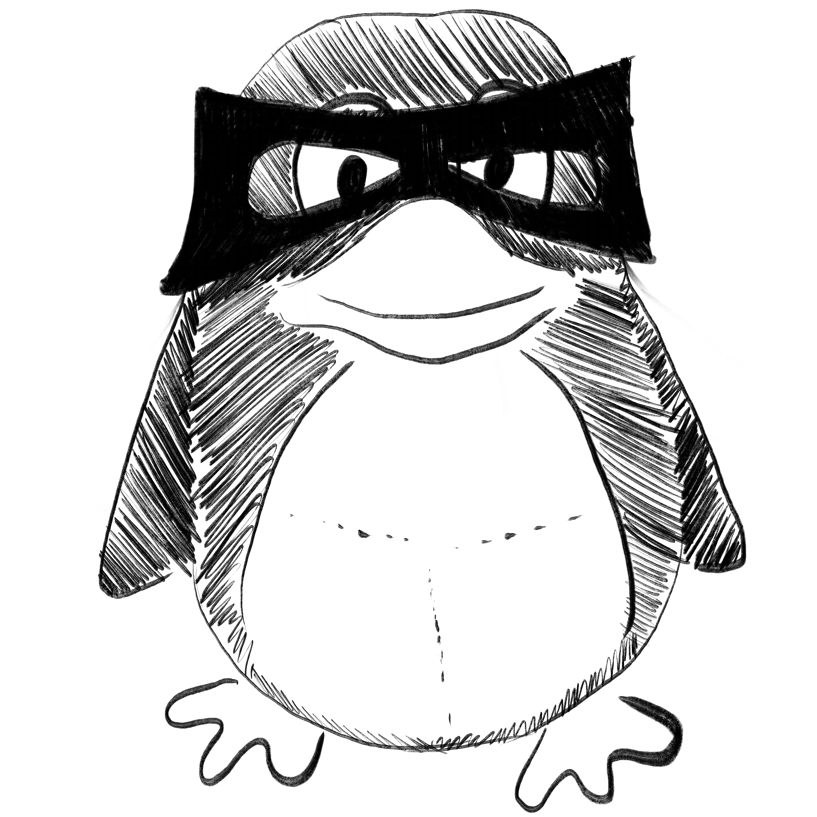
Agent-based Simulation for Online Mental Health Matching
ArXiv Preprint
Yuhan Liu, Anna Fang, Glen Moriarty, Robert Kraut, Haiyi Zhu
2023-03-20
Weekly Summary
Receive a weekly summary and discussion of the top papers of the week by leading researchers in the field.