Receive a weekly summary and discussion of the top papers of the week by leading researchers in the field.
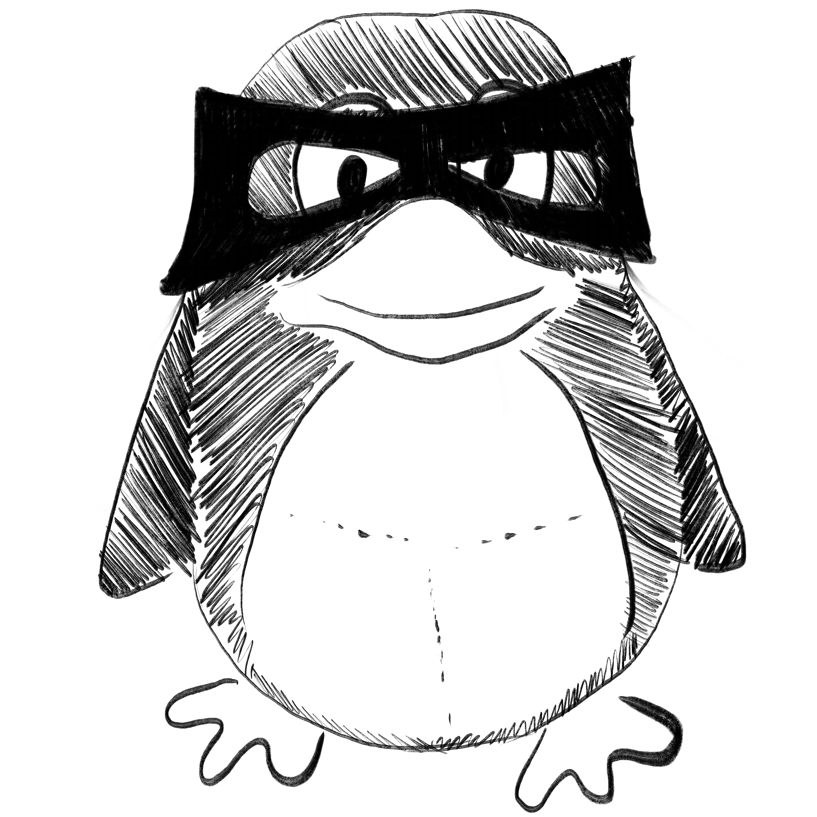
The effect of socially evaluated multitasking stress on typing rhythms.
In Psychophysiology
Wetherell Mark A, Lau Shing-Hon, Maxion Roy A
2023-Mar-20
Individual differences, cardiovascular, heart rate variability, motor control
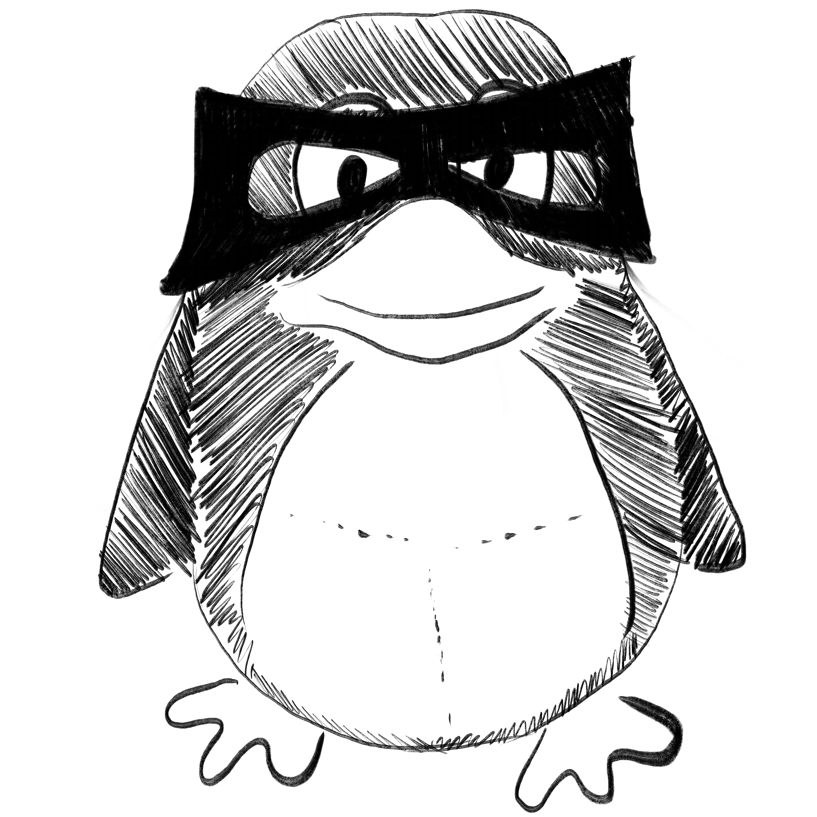
The intelligent experience inheritance system for Traditional Chinese Medicine.
In Journal of evidence-based medicine
Ren Xue, Guo Yan, Wang Heyuan, Gao Xiang, Chen Wei, Wang Tengjiao
2023-Mar-20
Traditional Chinese Medicine, artificial intelligence, pediatric asthma
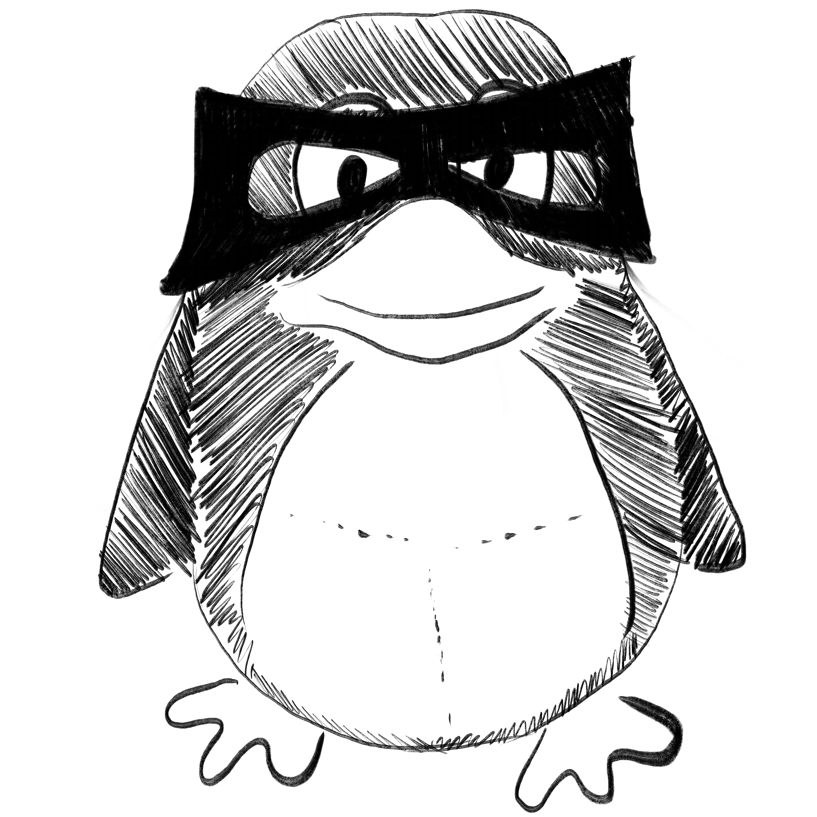
Construction accident prevention: A systematic review of machine learning approaches.
In Work (Reading, Mass.)
BACKGROUND :
OBJECTIVE :
METHODS :
RESULTS :
CONCLUSION :
Cavalcanti Marília, Lessa Luciano, Vasconcelos Bianca M
2023-Mar-13
Construction industry, algorithms, machine , safety management
Comprehensive transcriptomic analysis to identify biological and clinical differences in cholangiocarcinoma.
In Cancer medicine
BACKGROUND :
METHODS :
RESULTS :
CONCLUSIONS :
Silvestri Marco, Nghia Vu Trung, Nichetti Federico, Niger Monica, Di Cosimo Serena, De Braud Filippo, Pruneri Giancarlo, Pawitan Yudi, Calza Stefano, Cappelletti Vera
2023-Mar-20
bioinformatics, cholangiocarcinoma, next generation sequencing, transcriptomics, tumor-infiltrating immune cells
Everyday Driving and Plasma Biomarkers in Alzheimer's Disease: Leveraging Artificial Intelligence to Expand Our Diagnostic Toolkit.
In Journal of Alzheimer's disease : JAD
BACKGROUND :
OBJECTIVE :
METHODS :
RESULTS :
CONCLUSION :
Bayat Sayeh, Roe Catherine M, Schindler Suzanne, Murphy Samantha A, Doherty Jason M, Johnson Ann M, Walker Alexis, Ances Beau M, Morris John C, Babulal Ganesh M
2023-Mar-13
Alzheimer’s disease, amyloid, artificial intelligence, naturalistic, plasma biomarkers
Predicting Conversion from Subjective Cognitive Decline to Mild Cognitive Impairment and Alzheimer's Disease Dementia Using Ensemble Machine Learning.
In Journal of Alzheimer's disease : JAD
BACKGROUND :
OBJECTIVE :
METHODS :
RESULTS :
CONCLUSION :
Dolcet-Negre Marta M, Imaz Aguayo Laura, de Eulate Reyes García, Martí-Andrés Gloria, Matarrubia Marta Fernández, Domínguez Pablo, Fernández Seara Mará A, Riverol Mario
2023-Mar-13
Alzheimer’s disease, classification, machine learning, mild cognitive impairment, subjective cognitive decline
Weekly Summary
Receive a weekly summary and discussion of the top papers of the week by leading researchers in the field.