Receive a weekly summary and discussion of the top papers of the week by leading researchers in the field.
Applications of artificial intelligence in clinical management, research and health administration: imaging perspectives with a focus on hemophilia.
In Expert review of hematology
INTRODUCTION :
AREAS COVERED :
EXPERT OPINION :
Doria Andrea S
2023-Mar-20
Artificial Intelligence, arthropathy, augmentation, hemophilia, imaging, joints, machine learning, radiogenomics, regulations
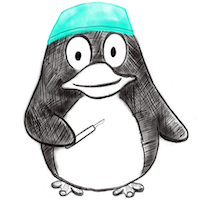
Quantifying and Improving the Performance of Speech Recognition Systems on Dysphonic Speech.
In Otolaryngology--head and neck surgery : official journal of American Academy of Otolaryngology-Head and Neck Surgery
OBJECTIVE :
STUDY DESIGN :
SETTING :
METHODS :
RESULTS :
CONCLUSION :
Hidalgo Lopez Julio C, Sandeep Shelly, Wright MaKayla, Wandell Grace M, Law Anthony B
2023-Jan-24
artificial intelligence, dysphonia, laryngology, transfer learning, voice
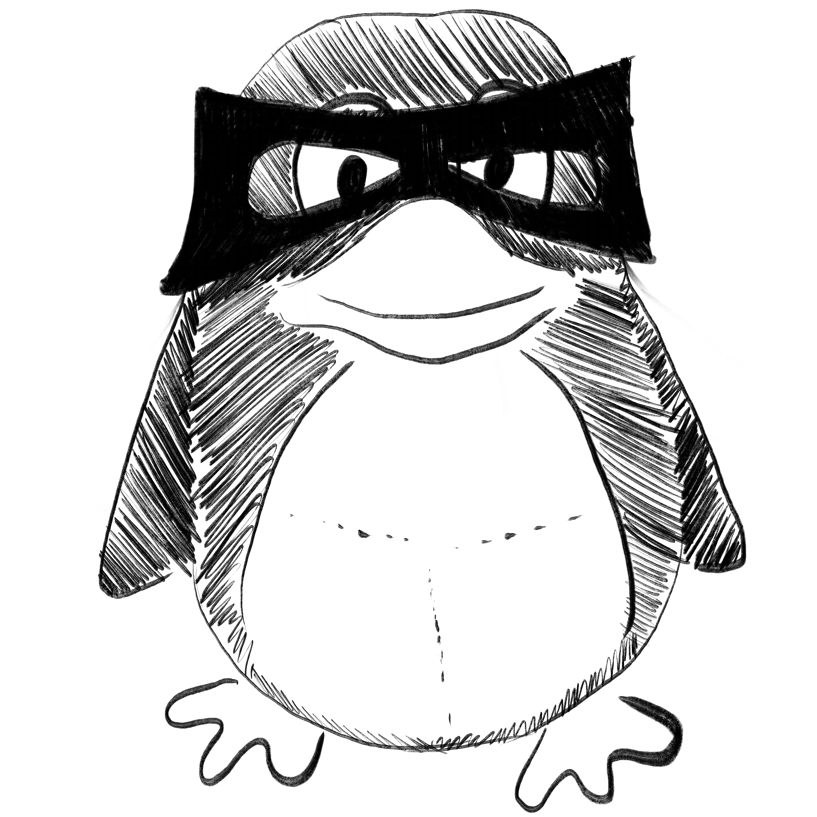
Intelligent Cubic-Designed Piezoelectric Node (iCUPE) with Simultaneous Sensing and Energy Harvesting Ability toward Self-Sustained Artificial Intelligence of Things (AIoT).
In ACS nano ; h5-index 203.0
Huang Manjuan, Zhu Minglu, Feng Xiaowei, Zhang Zixuan, Tang Tianyi, Guo Xinge, Chen Tao, Liu Huicong, Sun Lining, Lee Chengkuo
2023-Mar-20
artificial intelligence of things (AIoT), machine learning, piezoelectric generator, self-powered sensor, status monitoring
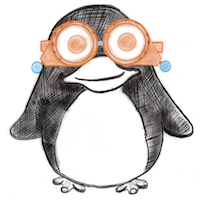
Analysis of risk and protective factors associated with retinal nerve fiber layer defect in a Chinese adult population.
In International journal of ophthalmology
AIM :
METHODS :
RESULTS :
CONCLUSION :
Wang Ye-Nan, Wang Ya-Xing, Zhou Jin-Qiong, Wan Qian-Qian, Fang Li-Jian, Wang Hai-Wei, Yang Jing-Yan, Dong Li, Wang Jin-Yuan, Yang Xuan, Yan Yan-Ni, Wang Qian, Wu Shou-Ling, Chen Shuo-Hua, Zhu Jing-Yuan, Wei Wen-Bin, Jonas Jost B
2023
age, axial length, central anterior chamber depth, diabetes mellitus, hypertension, retinal nerve fiber layer, retinal nerve fiber layer defect, visual acuity
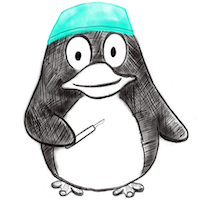
Objective Pharyngeal Phenotyping in Obstructive Sleep Apnea With High-Resolution Manometry.
In Otolaryngology--head and neck surgery : official journal of American Academy of Otolaryngology-Head and Neck Surgery
OBJECTIVE :
STUDY DESIGN :
SETTING :
METHODS :
RESULTS :
CONCLUSION :
Kent David T, Scott William C, Ye Cheng, Fabbri Daniel
2023-Jan-29
drug-induced sleep endoscopy, high-resolution manometry, obstructive sleep apnea, sleep surgery
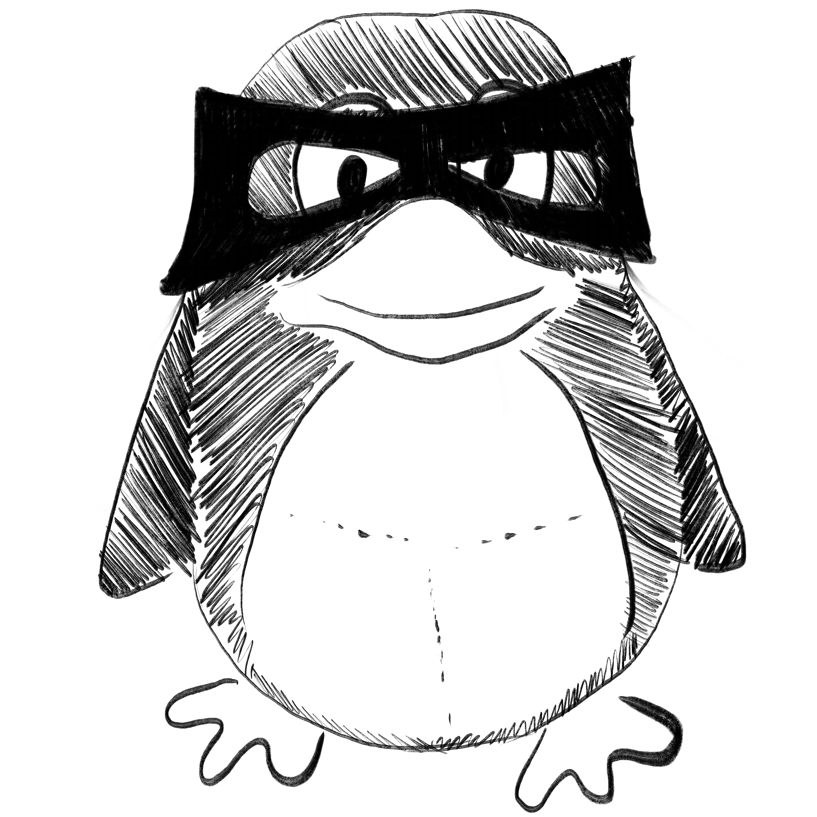
Movement Optimization for a Cyborg Cockroach in a Bounded Space Incorporating Machine Learning.
In Cyborg and bionic systems (Washington, D.C.)
Ariyanto Mochammad, Refat Chowdhury Mohammad Masum, Hirao Kazuyoshi, Morishima Keisuke
2023
Weekly Summary
Receive a weekly summary and discussion of the top papers of the week by leading researchers in the field.