Receive a weekly summary and discussion of the top papers of the week by leading researchers in the field.
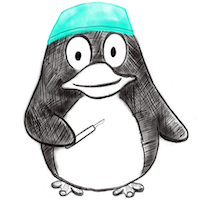
Machine learning prediction algorithm for in-hospital mortality following body contouring.
In Plastic and reconstructive surgery ; h5-index 62.0
BACKGROUND :
METHODS :
RESULTS :
CONCLUSIONS :
Peng Chi, Yang Fan, Jian Yu, Peng Liwei, Zhang Chenxu, Chen Chenxin, Lin Zhen, Li Yuejun, He Jia, Jin Zhichao
2023-Mar-21
Does deep learning software improve the consistency and performance of radiologists with various levels of experience in assessing bi-parametric prostate MRI?
In Insights into imaging
OBJECTIVE :
METHODS :
RESULTS :
CONCLUSIONS :
Arslan Aydan, Alis Deniz, Erdemli Servet, Seker Mustafa Ege, Zeybel Gokberk, Sirolu Sabri, Kurtcan Serpil, Karaarslan Ercan
2023-Mar-20
Deep learning, Magnetic resonance imaging, Prostate cancer
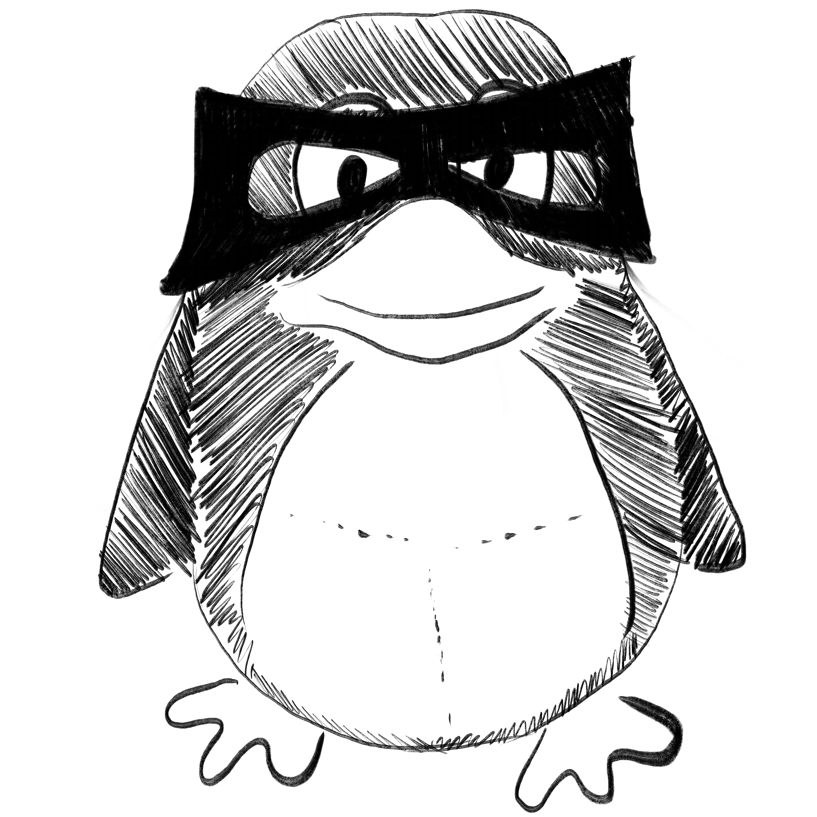
ASmiR: a machine learning framework for prediction of abiotic stress-specific miRNAs in plants.
In Functional & integrative genomics
Pradhan Upendra Kumar, Meher Prabina Kumar, Naha Sanchita, Rao Atmakuri Ramakrishna, Kumar Upendra, Pal Soumen, Gupta Ajit
2023-Mar-20
Abiotic stress, Computational biology, Deep learning, Machine learning, miRNAs
A cardiologist's guide to machine learning in cardiovascular disease prognosis prediction.
In Basic research in cardiology
Kresoja Karl-Patrik, Unterhuber Matthias, Wachter Rolf, Thiele Holger, Lurz Philipp
2023-Mar-20
Arrhythmia, Artificial intelligence, Atherosclerosis, Genetics, Heart failure, Machine learning
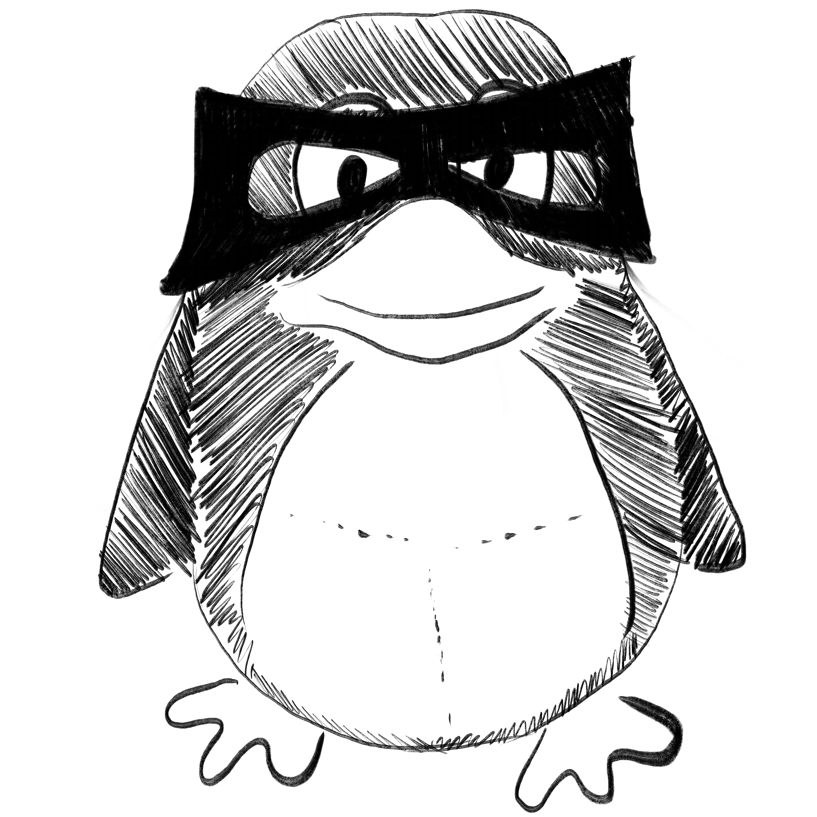
Artificial intelligence to predict outcomes of head and neck radiotherapy.
In Clinical and translational radiation oncology
Bang Chulmin, Bernard Galaad, Le William T, Lalonde Arthur, Kadoury Samuel, Bahig Houda
2023-Mar
ADASYN, adaptive synthetic sampling, AI, artificial intelligence, ANN, artificial neural network, AUC, Area Under the ROC Curve, Artificial intelligence, BMI, body mass index, C-Index, concordance index, CART, Classification and Regression Tree, CBCT, cone-beam computed tomography, CIFE, conditional informax feature extraction, CNN, convolutional neural network, CRT, chemoradiation, CT, computed tomography, Cancer outcomes, DL, deep learning, DM, distant metastasis, DSC, Dice Similarity Coefficient, DSS, clinical decision support systems, DT, Decision Tree, DVH, Dose-volume histogram, GANs, Generative Adversarial Networks, GB, Gradient boosting, GPU, graphical process units, HNC, head and neck cancer, HPV, human papillomavirus, HR, hazard ratio, Head and neck cancer, IAMB, incremental association Markov blanket, IBDM, image based data mining, IBMs, image biomarkers, IMRT, intensity-modulated RT, KNN, k nearest neighbor, LLR, Local linear forest, LR, logistic regression, LRR, loco-regional recurrence, MIFS, mutual information based feature selection, ML, machine learning, MRI, Magnetic resonance imaging, MRMR, Minimum redundancy feature selection, Machine learning, N-MLTR, Neural Multi-Task Logistic Regression, NPC, nasopharynx, NTCP, Normal Tissue Complication Probability, OPC, oropharyngeal cancer, ORN, osteoradionecrosis, OS, overall survival, PCA, Principal component analysis, PET, Positron emission tomography, PG, parotid glands, PLR, Positive likelihood ratio, PM, pharyngeal mucosa, PTV, Planning target volumes, PreSANet, deep preprocessor module and self-attention, Predictive modeling, QUANTEC, Quantitative Analyses of Normal Tissue Effects in the Clinic, RF, random forest, RFC, random forest classifier, RFS, recurrence free survival, RLR, Rigid logistic regression, RRF, Regularized random forest, RSF, random survival forest, RT, radiotherapy, RTLI, radiation-induced temporal lobe injury, Radiomic, SDM, shared decision making, SMG, submandibular glands, SMOTE, synthetic minority over-sampling technique, STIC, sticky saliva, SVC, support vector classifier, SVM, support vector machine, XGBoost, extreme gradient boosting
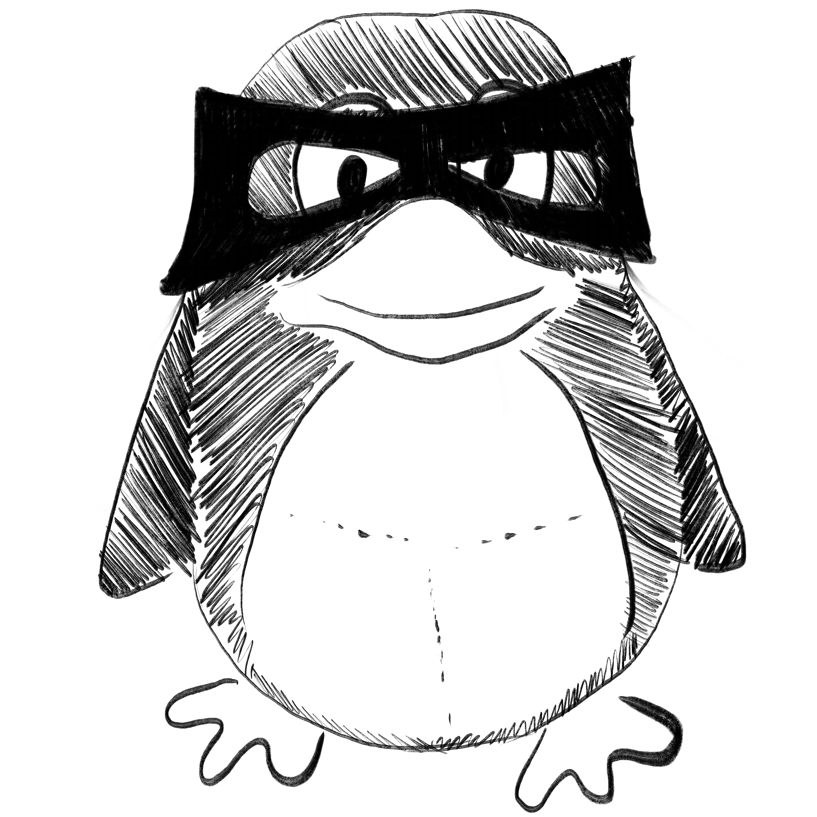
Machine learning-based immune prognostic model and ceRNA network construction for lung adenocarcinoma.
In Journal of cancer research and clinical oncology
PURPOSE :
METHODS :
RESULTS :
CONCLUSION :
He Xiaoqian, Su Ying, Liu Pei, Chen Cheng, Chen Chen, Guan Haoqin, Lv Xiaoyi, Guo Wenjia
2023-Mar-20
Immune prognostic model, Lung adenocarcinoma, SVM-RFE, WGCNA, ceRNA
Weekly Summary
Receive a weekly summary and discussion of the top papers of the week by leading researchers in the field.