Receive a weekly summary and discussion of the top papers of the week by leading researchers in the field.
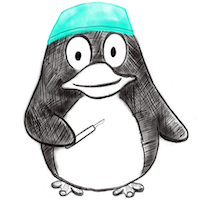
Artificial Intelligence for Automated Implant Identification in Knee Arthroplasty: A Multicenter External Validation Study Exceeding 3.5 Million Plain Radiographs.
In The Journal of arthroplasty ; h5-index 65.0
BACKGROUND :
METHODS :
RESULTS :
CONCLUSION :
Karnuta Jaret M, Shaikh Hashim J F, Murphy Michael P, Brown Nicholas M, Pearle Andrew D, Nawabi Danyal H, Chen Antonia F, Ramkumar Prem N
2023-Mar-18
artificial intelligence, implant identification, knee arthroplasty, machine learning, revision arthroplasty
Serum Immune Markers and Transition to Psychosis in Individuals at Clinical High Risk.
In Brain, behavior, and immunity
Mondelli Valeria, Blackman Graham, Kempton Matthew J, Pollak Thomas A, Iyegbe Conrad, Valmaggia Lucia R, Amminger Paul, Barrantes-Vidal Neus, Bressan Rodrigo, van der Gaag Mark, de Haan Lieuwe, Krebs Marie-Odile, Nordentoft Merete, Ruhrmann Stephan, Riecher-Rössler Anita, Rutten Bart P F, Sachs Gabriele, Koutsouleris Nikolaos, McGuire Philip
2023-Mar-18
VEGF, clinical high risk, cytokines, immune markers, inflammation, interlukin-6, psychosis, transition
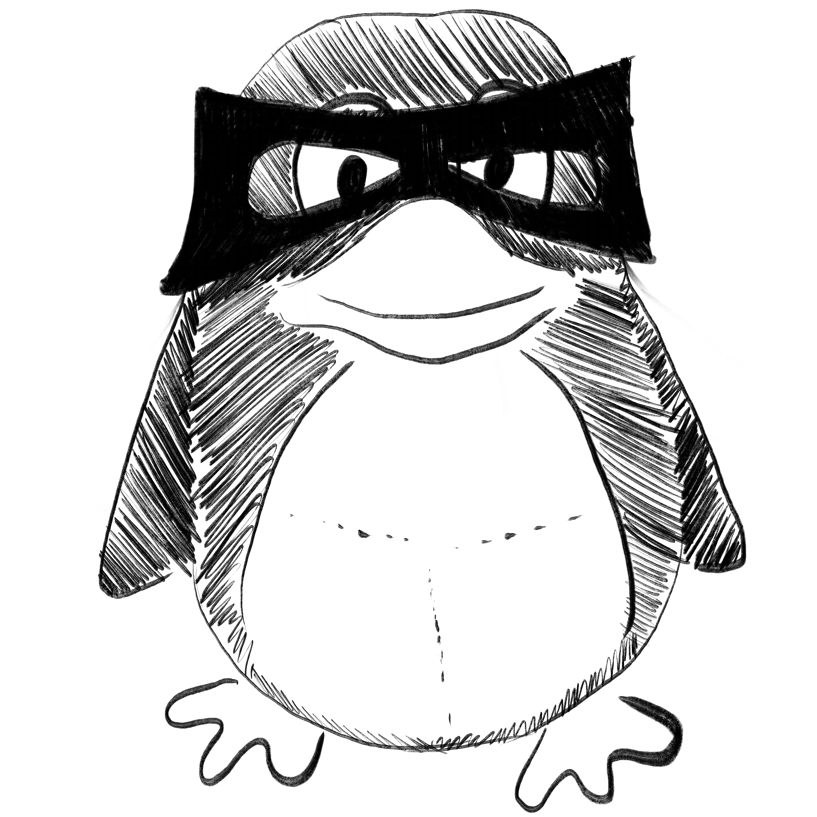
Identification of potentially contaminated areas of soil microplastic based on machine learning: A case study in Taihu Lake region, China.
In The Science of the total environment
Qiu Yifei, Zhou Shenglu, Zhang Chuchu, Qin Wendong, Lv Chengxiang, Zou Mengmeng
2023-Mar-18
Microplastic, Potential risk, Source–sink, Spatial correlation analysis
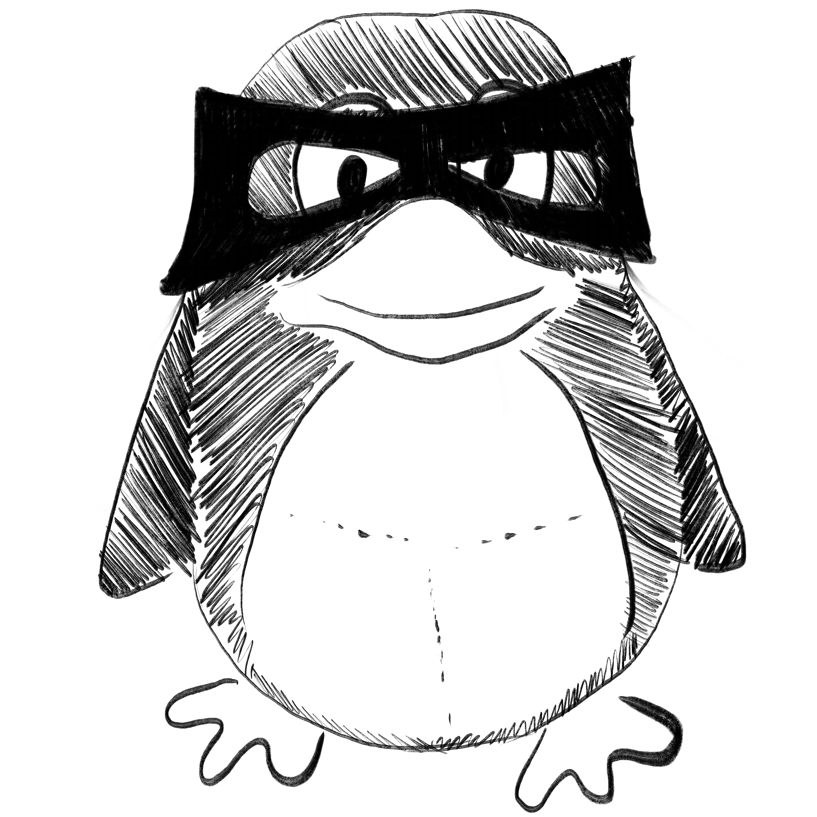
Improving predictions of shale wettability using advanced machine learning techniques and nature-inspired methods: Implications for carbon capture utilization and storage.
In The Science of the total environment
Zhang Hemeng, Thanh Hung Vo, Rahimi Mohammad, Al-Mudhafar Watheq J, Tangparitkul Suparit, Zhang Tao, Dai Zhenxue, Ashraf Umar
2023-Mar-18
Artificial intelligence, CO(2) capture, Carbon storage, Contact angle measurement, Deep learning, Wettability behavior
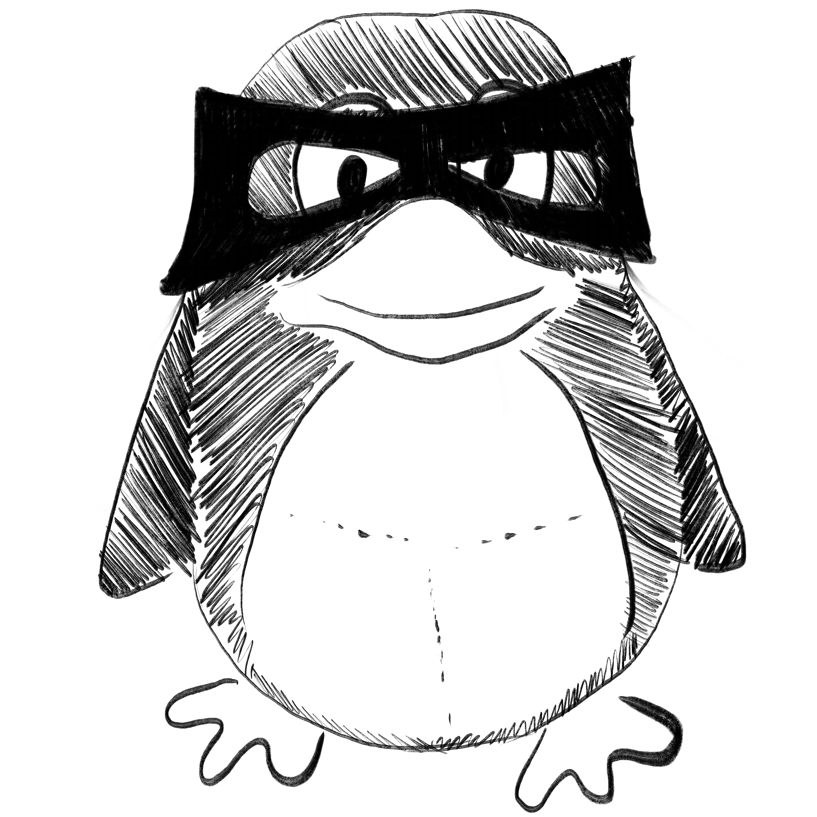
Calving prediction with continuous measurement of subcutaneous tissue glucose concentration in pregnant cows.
In Theriogenology ; h5-index 37.0
Wakatsuki Takuji, Nakamura Tsukasa, Ishii Ayumi, Konishi Kanta, Okubo Michiko, Souma Kousaku, Hirayama Hiroki
2023-Mar-13
Calving, Calving prediction systems, Cows, Dystocia, Subcutaneous tissue glucose concentration, Wearable sensors
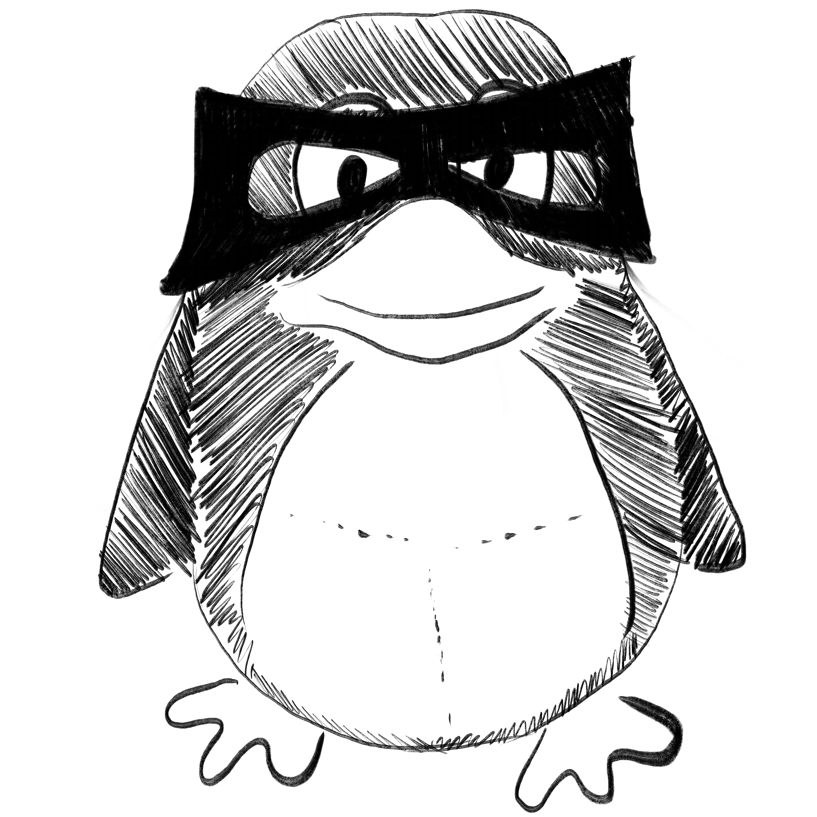
Step-adaptive sound guidance enhances locomotor-respiratory coupling in novice female runners: A proof-of-concept study.
In Frontiers in sports and active living
INTRODUCTION :
METHODS :
RESULTS :
DISCUSSION :
Harbour Eric, van Rheden Vincent, Schwameder Hermann, Finkenzeller Thomas
2023
breathing strategies, breathing techniques, entrainment, locomotor-respiratory coupling, running, synchronization
Weekly Summary
Receive a weekly summary and discussion of the top papers of the week by leading researchers in the field.