Receive a weekly summary and discussion of the top papers of the week by leading researchers in the field.
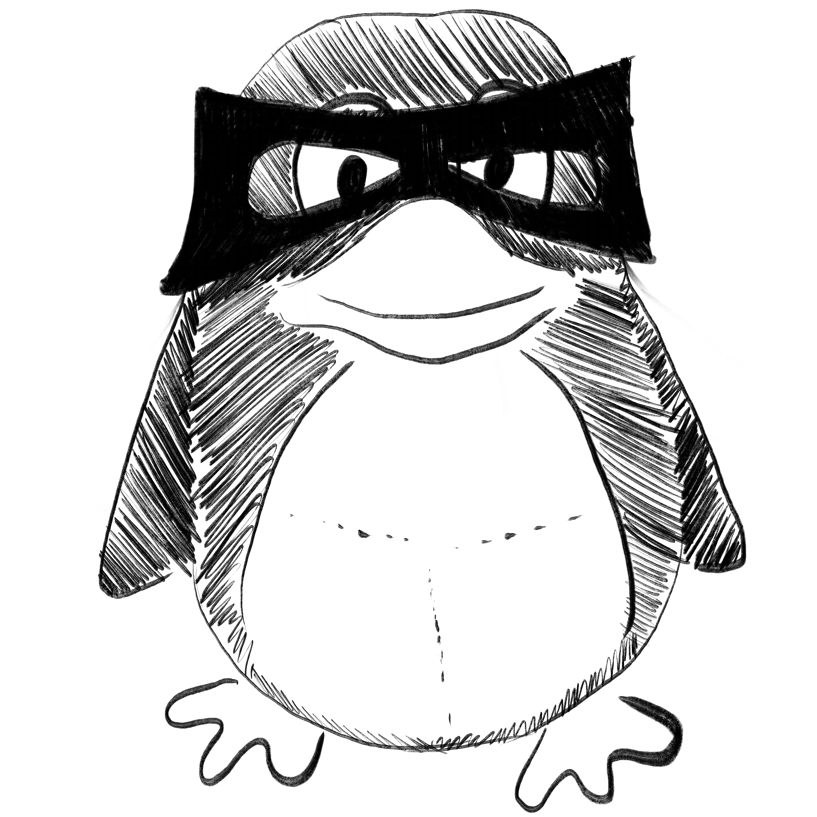
Prediction of postpartum hemorrhage using traditional statistical analysis and a machine learning approach.
In AJOG global reports
BACKGROUND :
OBJECTIVE :
STUDY DESIGN :
RESULTS :
CONCLUSION :
Mehrnoush Vahid, Ranjbar Amene, Farashah Mohammadsadegh Vahidi, Darsareh Fatemeh, Shekari Mitra, Jahromi Malihe Shirzadfard
2023-May
analysis, machine learning, postpartum hemorrhage, risk factors
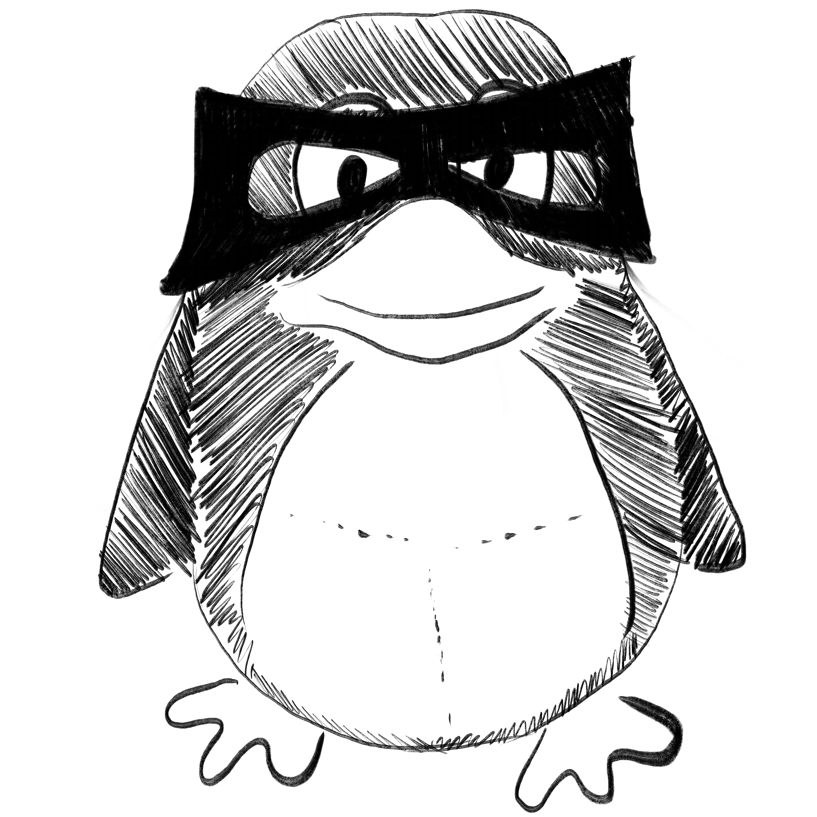
Methods of performance analysis in women's Australian football: a scoping review.
In PeerJ
BACKGROUND :
METHODOLOGY :
RESULTS :
CONCLUSIONS :
van der Vegt Braedan R, Gepp Adrian, Keogh Justin W L, Farley Jessica B
2023
AFLW, Australian Football, Data analysis, Female athlete, Game actions, Performance analysis, Running demands
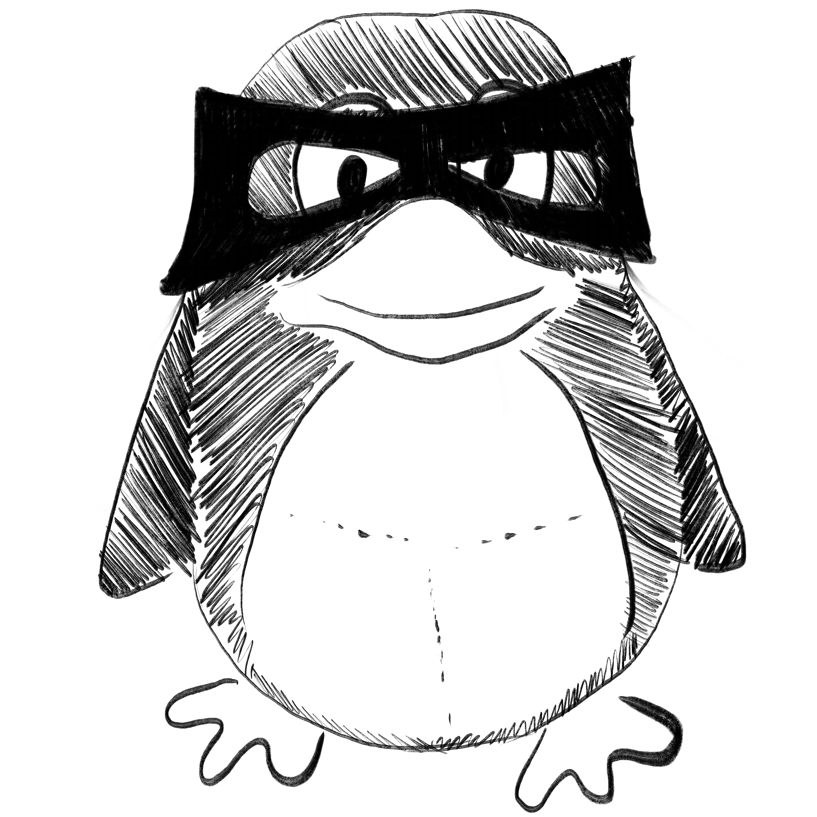
Sleep electroencephalography biomarkers of cognition in obstructive sleep apnea.
In Journal of sleep research
Gu Yusing, Gagnon Jean-François, Kaminska Marta
2023-Mar-20
K-complex, cyclic alternating pattern, odds ratio product, quantitative electroencephalography, sleep spindles, slow-wave activity
Improving Augmented Reality Through Deep Learning: Real-time Instrument Delineation in Robotic Renal Surgery.
In European urology ; h5-index 128.0
De Backer Pieter, Van Praet Charles, Simoens Jente, Peraire Lores Maria, Creemers Heleen, Mestdagh Kenzo, Allaeys Charlotte, Vermijs Saar, Piazza Pietro, Mottaran Angelo, Bravi Carlo A, Paciotti Marco, Sarchi Luca, Farinha Rui, Puliatti Stefano, Cisternino Francesco, Ferraguti Federica, Debbaut Charlotte, De Naeyer Geert, Decaestecker Karel, Mottrie Alexandre
2023-Mar-18
Augmented reality, Deep learning, Instrument segmentation, Kidney transplantation, Partial nephrectomy, Real time, Renal cell carcinoma, Robotic surgery, Three-dimensional models
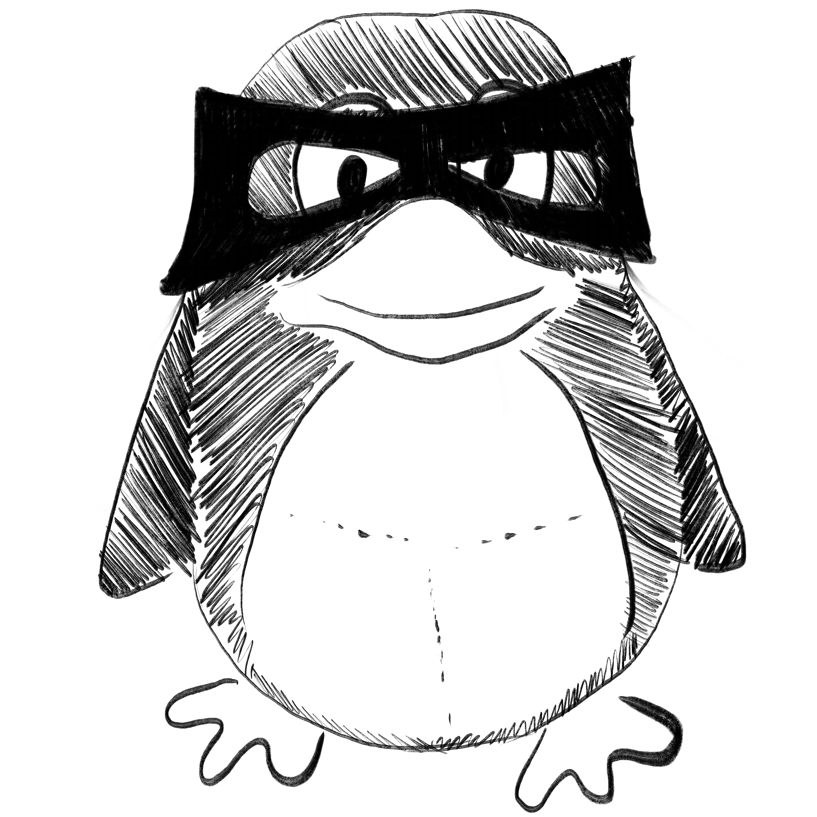
Sleep electroencephalography biomarkers of cognition in obstructive sleep apnea.
In Journal of sleep research
Gu Yusing, Gagnon Jean-François, Kaminska Marta
2023-Mar-20
K-complex, cyclic alternating pattern, odds ratio product, quantitative electroencephalography, sleep spindles, slow-wave activity
Improving Augmented Reality Through Deep Learning: Real-time Instrument Delineation in Robotic Renal Surgery.
In European urology ; h5-index 128.0
De Backer Pieter, Van Praet Charles, Simoens Jente, Peraire Lores Maria, Creemers Heleen, Mestdagh Kenzo, Allaeys Charlotte, Vermijs Saar, Piazza Pietro, Mottaran Angelo, Bravi Carlo A, Paciotti Marco, Sarchi Luca, Farinha Rui, Puliatti Stefano, Cisternino Francesco, Ferraguti Federica, Debbaut Charlotte, De Naeyer Geert, Decaestecker Karel, Mottrie Alexandre
2023-Mar-18
Augmented reality, Deep learning, Instrument segmentation, Kidney transplantation, Partial nephrectomy, Real time, Renal cell carcinoma, Robotic surgery, Three-dimensional models
Weekly Summary
Receive a weekly summary and discussion of the top papers of the week by leading researchers in the field.