Receive a weekly summary and discussion of the top papers of the week by leading researchers in the field.
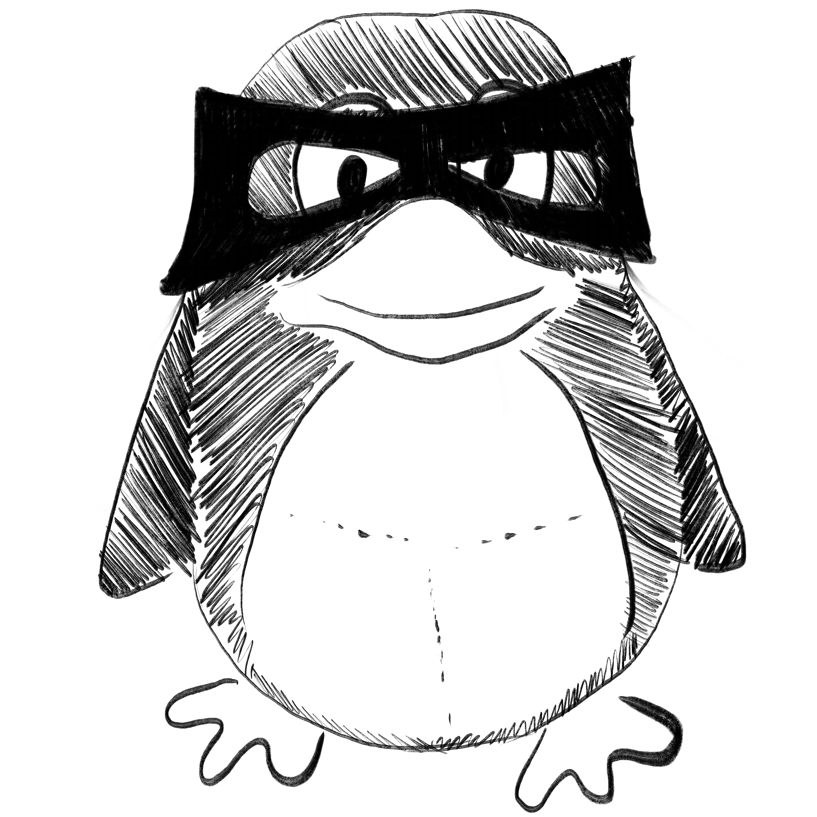
Comparison of Machine Learning Methods in the Study of Cancer Survivors' Return to Work: An Example of Breast Cancer Survivors with Work-Related Factors in the CONSTANCES Cohort.
In Journal of occupational rehabilitation
PURPOSE :
METHODS :
RESULTS :
CONCLUSION :
Badreau Marie, Fadel Marc, Roquelaure Yves, Bertin Mélanie, Rapicault Clémence, Gilbert Fabien, Porro Bertrand, Descatha Alexis
2023-Mar-20
Breast cancer, Machine learning, Methods, Prediction, Return to work, Survivors
Mechanisms influencing the factors of urban built environments and coronavirus disease 2019 at macroscopic and microscopic scales: The role of cities.
In Frontiers in public health
Zhang Longhao, Han Xin, Wu Jun, Wang Lei
2023
COVID-19, computer vision, deep learning, relevance, street view images, urban built environment
LIMITR: Leveraging Local Information for Medical Image-Text Representation
ArXiv Preprint
Gefen Dawidowicz, Elad Hirsch, Ayellet Tal
2023-03-21
A hybrid deep forest-based method for predicting synergistic drug combinations.
In Cell reports methods
Wu Lianlian, Gao Jie, Zhang Yixin, Sui Binsheng, Wen Yuqi, Wu Qingqiang, Liu Kunhong, He Song, Bo Xiaochen
2023-Feb-27
class imbalance, deep forest, deep learning, drug combination prediction, synergy
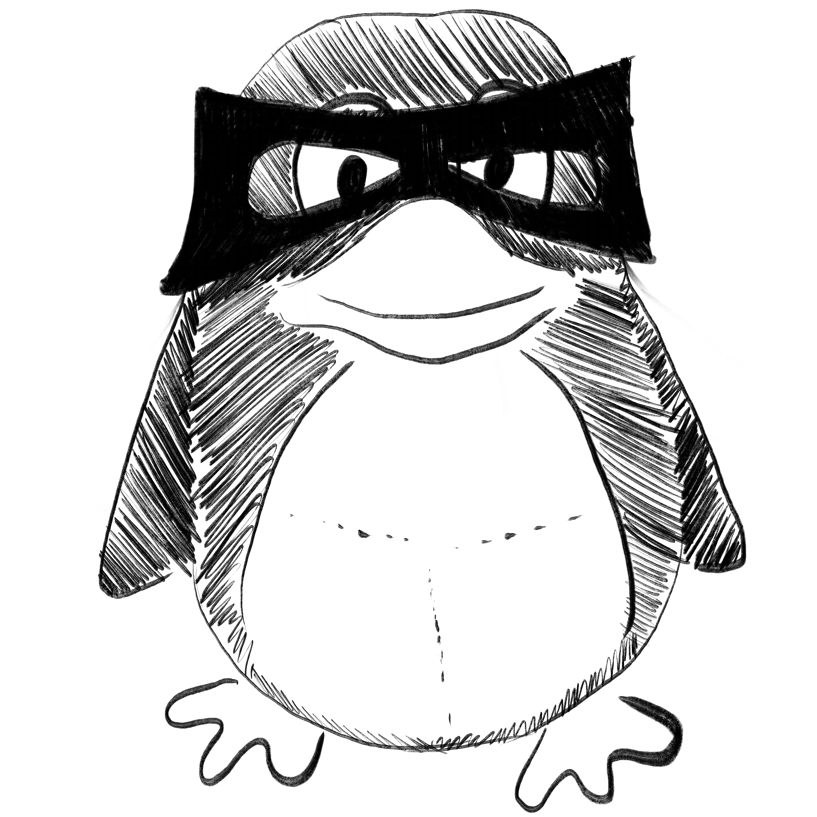
BOMA, a machine-learning framework for comparative gene expression analysis across brains and organoids.
In Cell reports methods
He Chenfeng, Kalafut Noah Cohen, Sandoval Soraya O, Risgaard Ryan, Sirois Carissa L, Yang Chen, Khullar Saniya, Suzuki Marin, Huang Xiang, Chang Qiang, Zhao Xinyu, Sousa Andre M M, Wang Daifeng
2023-Feb-27
cell-type functional genomics, comparative analysis of brains and organoids, developmental gene expression patterns, manifold alignment tool and webapp
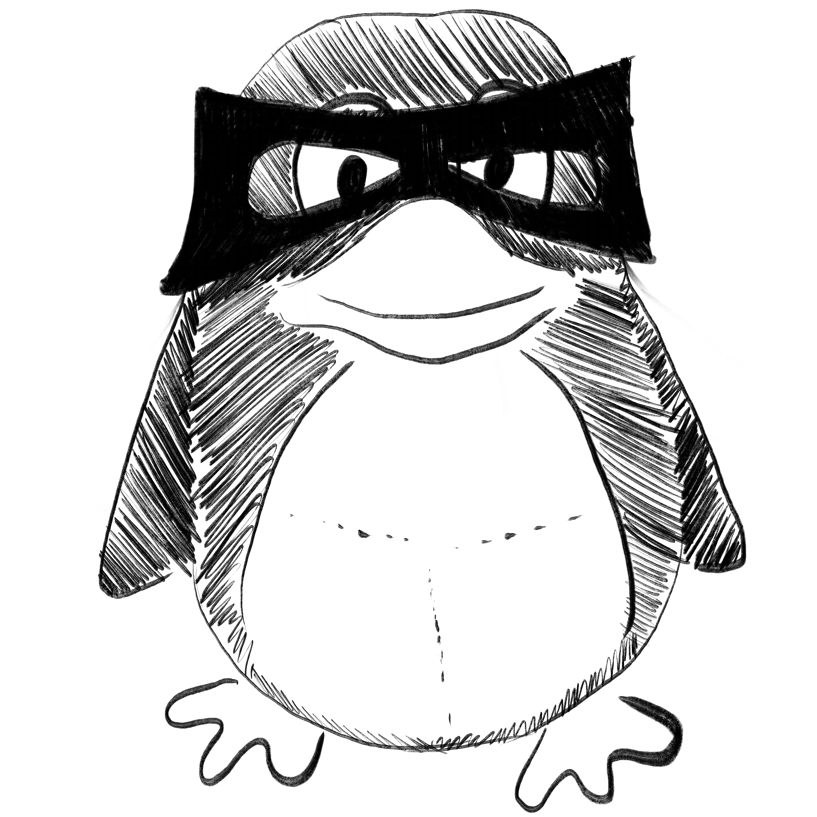
Exploring obesity, physical activity, and digital game addiction levels among adolescents: A study on machine learning-based prediction of digital game addiction.
In Frontiers in psychology ; h5-index 92.0
Gülü Mehmet, Yagin Fatma Hilal, Gocer Ishak, Yapici Hakan, Ayyildiz Erdem, Clemente Filipe Manuel, Ardigò Luca Paolo, Zadeh Ali Khosravi, Prieto-González Pablo, Nobari Hadi
2023
addiction, body mass index, children, obesity, physical inactivity, sedentary behaviors
Weekly Summary
Receive a weekly summary and discussion of the top papers of the week by leading researchers in the field.