Receive a weekly summary and discussion of the top papers of the week by leading researchers in the field.
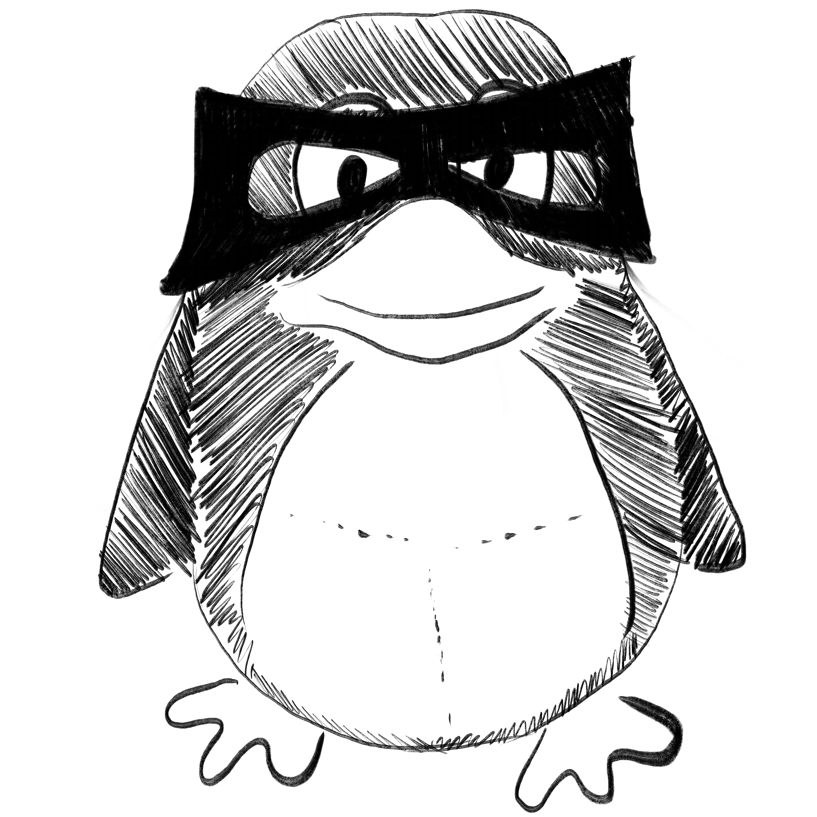
Improved fully convolutional neuron networks on small retinal vessel segmentation using local phase as attention.
In Frontiers in medicine
Kuang Xihe, Xu Xiayu, Fang Leyuan, Kozegar Ehsan, Chen Huachao, Sun Yue, Huang Fan, Tan Tao
2023
local phase, orientation scores, retinal vessel, segmentation, unsupervised enhancement
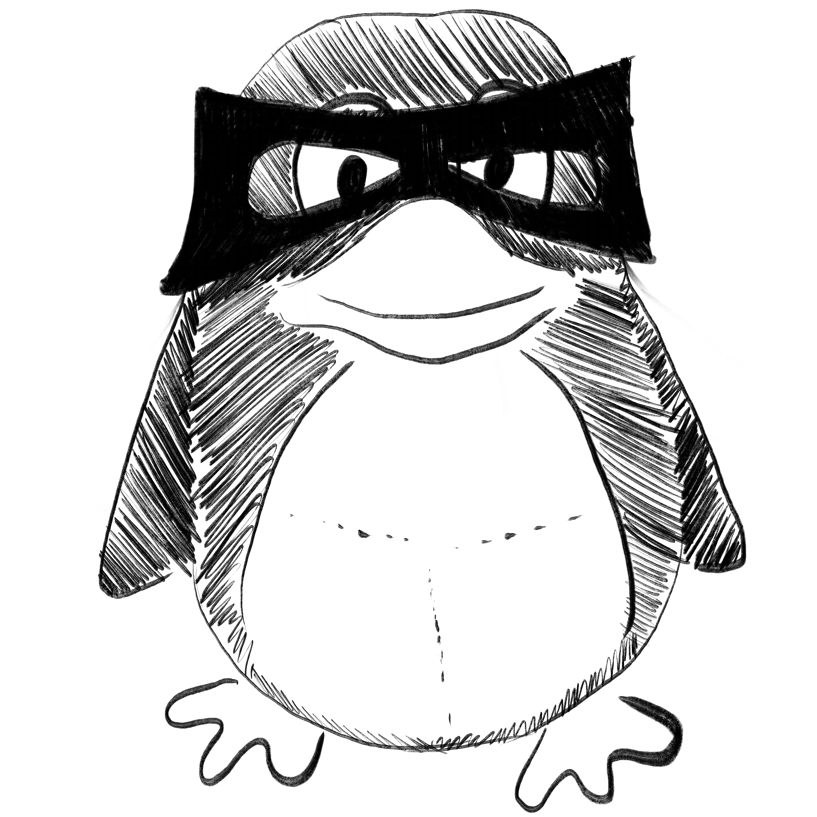
Energy-based analog neural network framework.
In Frontiers in computational neuroscience
Watfa Mohamed, Garcia-Ortiz Alberto, Sassatelli Gilles
2023
SPICE, analog, energy-based models, equilibrium propagation, framework, mixed-signal, neural networks
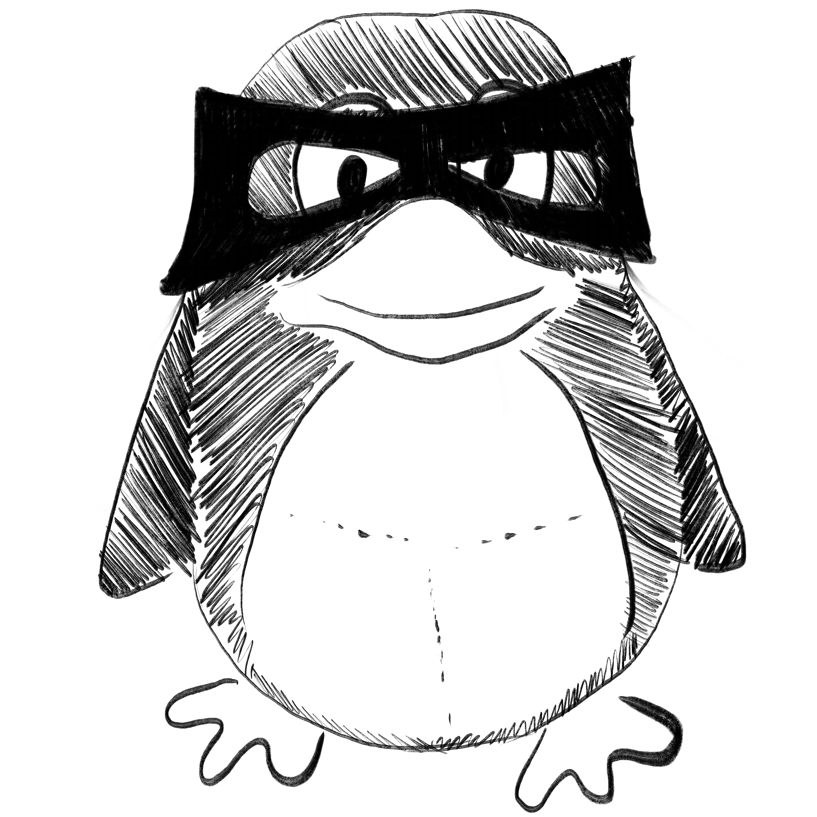
Identifying the challenges and opportunities of PCOS awareness month by analysing its global digital impact.
In Frontiers in endocrinology ; h5-index 55.0
BACKGROUND AND OBJECTIVE :
METHODS :
RESULTS :
CONCLUSION :
Malhotra Kashish, Pan Carina Synn Cuen, Davitadze Meri, Kempegowda Punith
2023
PCOS, PCOS awareness month, collaboration, global equity, mental health, polycystic ovary syndrome, social media
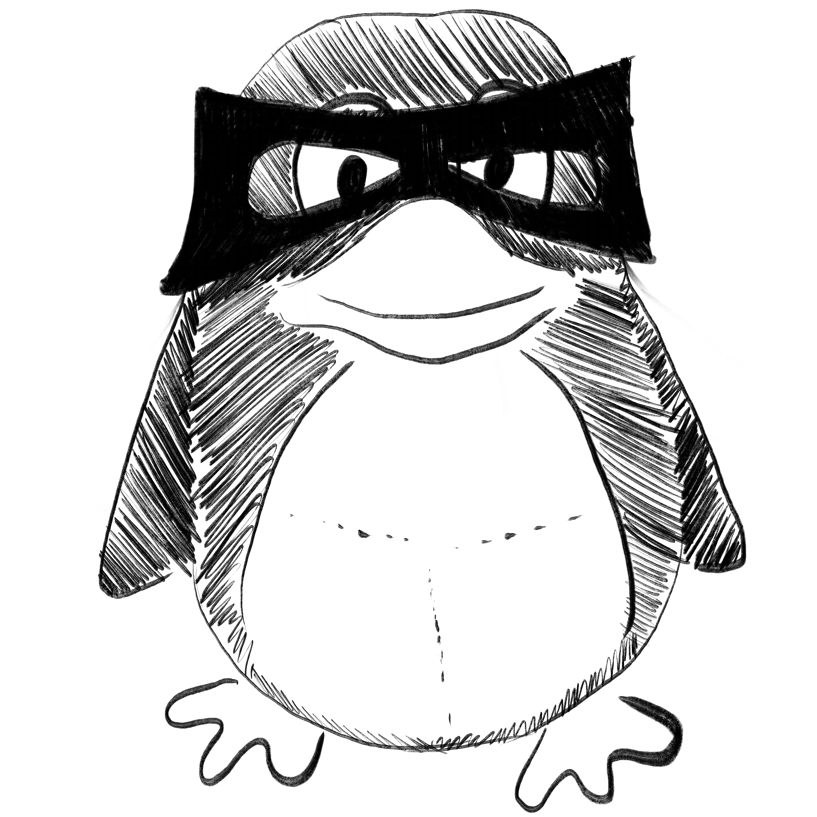
Microwave-assisted valorization and characterization of Citrus limetta peel waste into pectin as a perspective food additive.
In Journal of food science and technology
Sharma Poonam, Osama Khwaja, Varjani Sunita, Farooqui Alvina, Younis Kaiser
2023-Apr
Eco-friendly, Food additive, Genetic algorithm, Microwave-assisted extraction, Pectin, Support vector regression
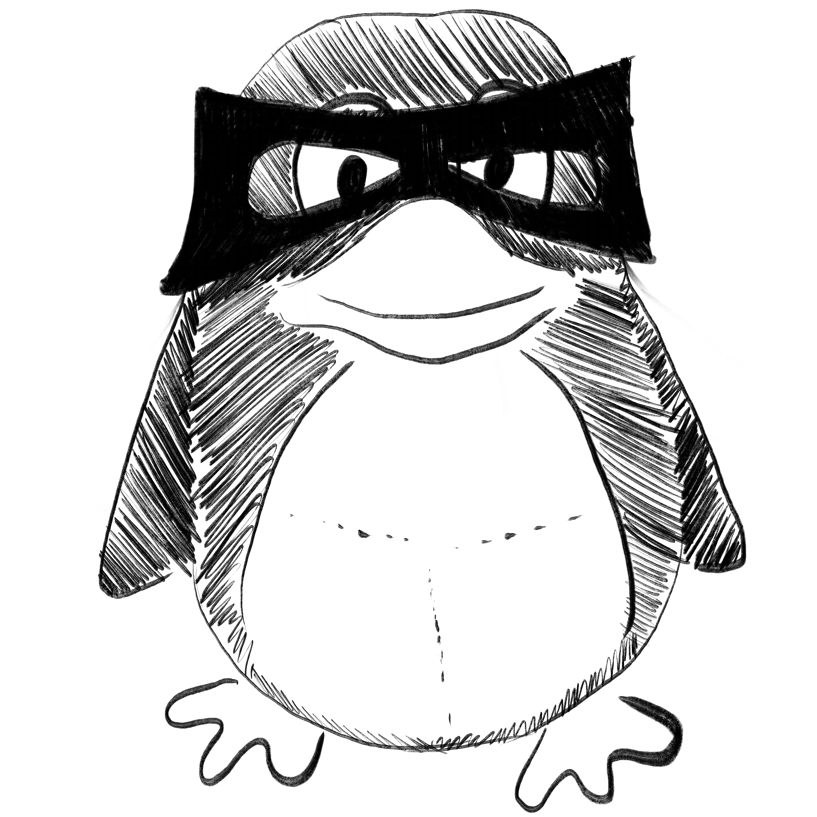
Blood RNA alternative splicing events as diagnostic biomarkers for infectious disease.
In Cell reports methods
Zhang Zijun, Sauerwald Natalie, Cappuccio Antonio, Ramos Irene, Nair Venugopalan D, Nudelman German, Zaslavsky Elena, Ge Yongchao, Gaitas Angelo, Ren Hui, Brockman Joel, Geis Jennifer, Ramalingam Naveen, King David, McClain Micah T, Woods Christopher W, Henao Ricardo, Burke Thomas W, Tsalik Ephraim L, Goforth Carl W, Lizewski Rhonda A, Lizewski Stephen E, Weir Dawn L, Letizia Andrew G, Sealfon Stuart C, Troyanskaya Olga G
2023-Feb-27
RNA splicing, SARS-CoV-2, diagnostic biomarker, host response assays, infectious disease, viral infection
Machine learning approaches to predict drug efficacy and toxicity in oncology.
In Cell reports methods
Badwan Bara A, Liaropoulos Gerry, Kyrodimos Efthymios, Skaltsas Dimitrios, Tsirigos Aristotelis, Gorgoulis Vassilis G
2023-Feb-27
artificial intelligence, drug discovery, drug response, machine learning
Weekly Summary
Receive a weekly summary and discussion of the top papers of the week by leading researchers in the field.