Receive a weekly summary and discussion of the top papers of the week by leading researchers in the field.
An AI-enabled research support tool for the classification system of COVID-19.
In Frontiers in public health
Tiwari Arti, Bhattacharjee Kamanasish, Pant Millie, Srivastava Shilpa, Snasel Vaclav
2023
Artificial Intelligence, COVID-19, bi-directional LSTM, classification, long short-term memory
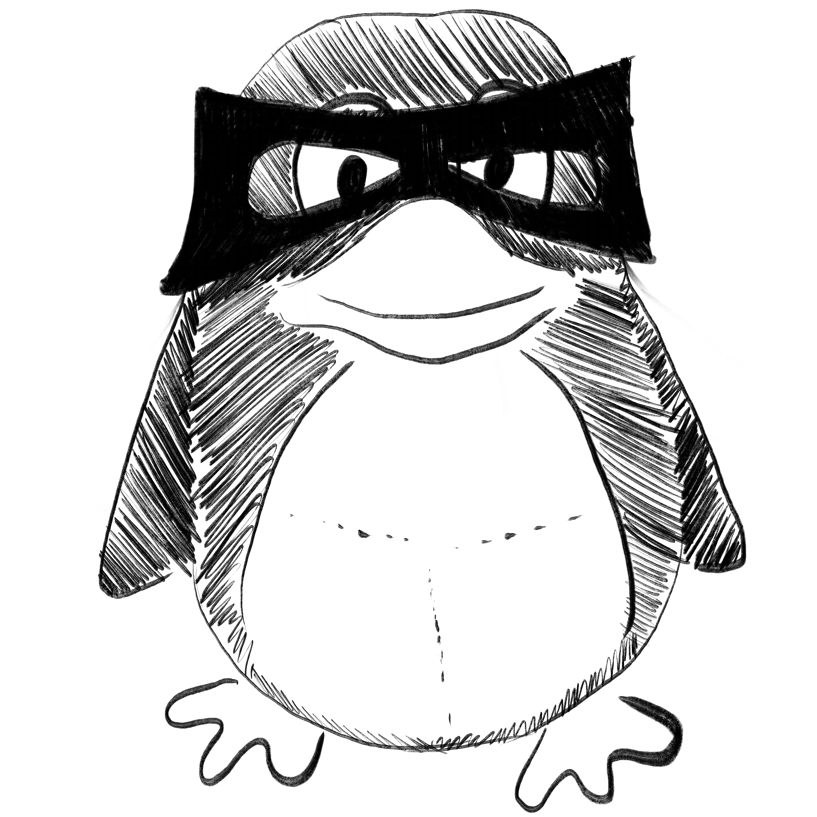
Large AI Models in Health Informatics: Applications, Challenges, and the Future
ArXiv Preprint
Jianing Qiu, Lin Li, Jiankai Sun, Jiachuan Peng, Peilun Shi, Ruiyang Zhang, Yinzhao Dong, Kyle Lam, Frank P. -W. Lo, Bo Xiao, Wu Yuan, Dong Xu, Benny Lo
2023-03-21
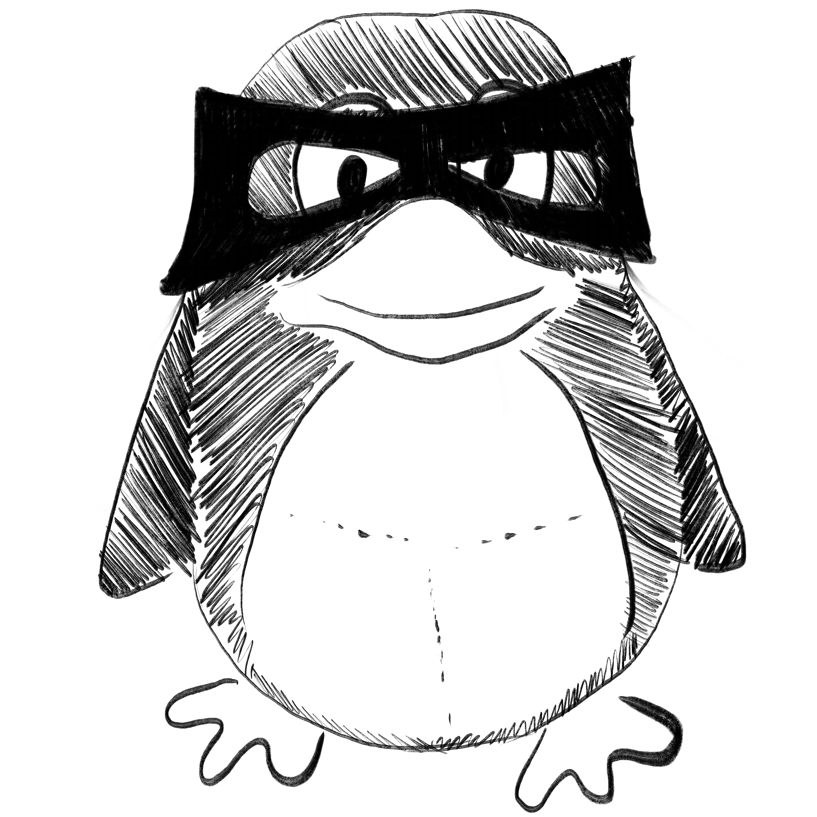
Clinical Biomarkers and Prediction Models for Poststroke Epilepsy: Have We Settled the Scores Yet?
In Neurology. Clinical practice
Yonas Amen S, Meschia James F, Feyissa Anteneh M
2023-Apr
Prognosis and Characterization of Microenvironment in Cervical Cancer Influenced by Fatty Acid Metabolism-Related Genes.
In Journal of oncology
Zhou Yanjun, Zhu Jiahao, Gu Mengxuan, Gu Ke
2023
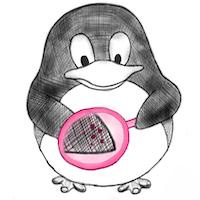
Towards AI-driven longevity research: An overview.
In Frontiers in aging
Marino Nicola, Putignano Guido, Cappilli Simone, Chersoni Emmanuele, Santuccione Antonella, Calabrese Giuliana, Bischof Evelyne, Vanhaelen Quentin, Zhavoronkov Alex, Scarano Bryan, Mazzotta Alessandro D, Santus Enrico
2023
artificial intelligence, biomarkers, deep aging clock, feature selection, longevity medicine, machine learning
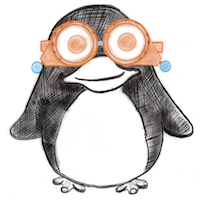
Using deep leaning models to detect ophthalmic diseases: A comparative study.
In Frontiers in medicine
PURPOSE :
METHODS :
RESULTS :
CONCLUSION :
Li Zhixi, Guo Xinxing, Zhang Jian, Liu Xing, Chang Robert, He Mingguang
2023
age-related macular degeneration, deep learning, diabetic retinopathy, fundus photograph, glaucomatous optic neuropathy
Weekly Summary
Receive a weekly summary and discussion of the top papers of the week by leading researchers in the field.