Receive a weekly summary and discussion of the top papers of the week by leading researchers in the field.
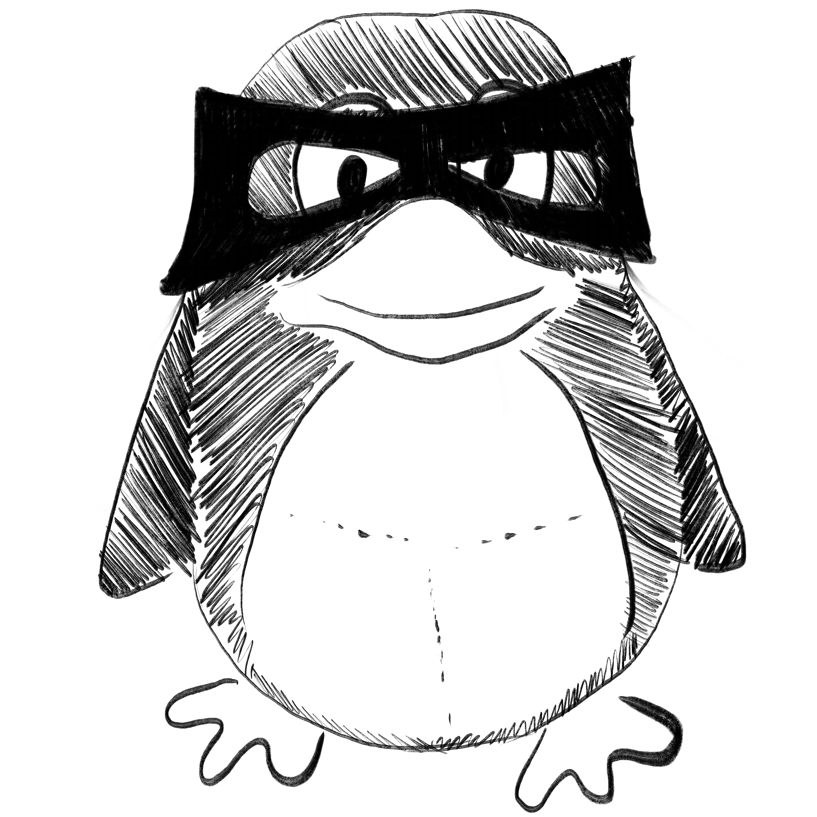
Development of maize plant dataset for intelligent recognition and weed control.
In Data in brief
Olaniyi Olayemi Mikail, Salaudeen Muhammadu Tajudeen, Daniya Emmanuel, Abdullahi Ibrahim Mohammed, Folorunso Taliha Abiodun, Bala Jibril Abdullahi, Nuhu Bello Kontagora, Adedigba Adeyinka Peace, Oluwole Blessing Israel, Bankole Abdullah Oreoluwa, Macarthy Odunayo Moses
2023-Apr
Autonomous robot, Herbicides, Maize images, Precision agriculture
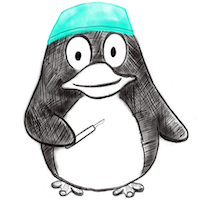
Real-time administration of indocyanine green in combination with computer vision and artificial intelligence for the identification and delineation of colorectal liver metastases.
In Surgery open science
INTRODUCTION :
METHODS :
RESULTS :
CONCLUSION :
Hardy Niall P, Epperlein Jonathan P, Dalli Jeffrey, Robertson William, Liddy Richard, Aird John J, Mulligan Niall, Neary Peter M, McEntee Gerard P, Conneely John B, Cahill Ronan A
2023-Mar
Artificial intelligence, Colorectal liver metastases, Fluorescence guided surgery, Fluorescence quantification, Indocyanine green, Liver surgery
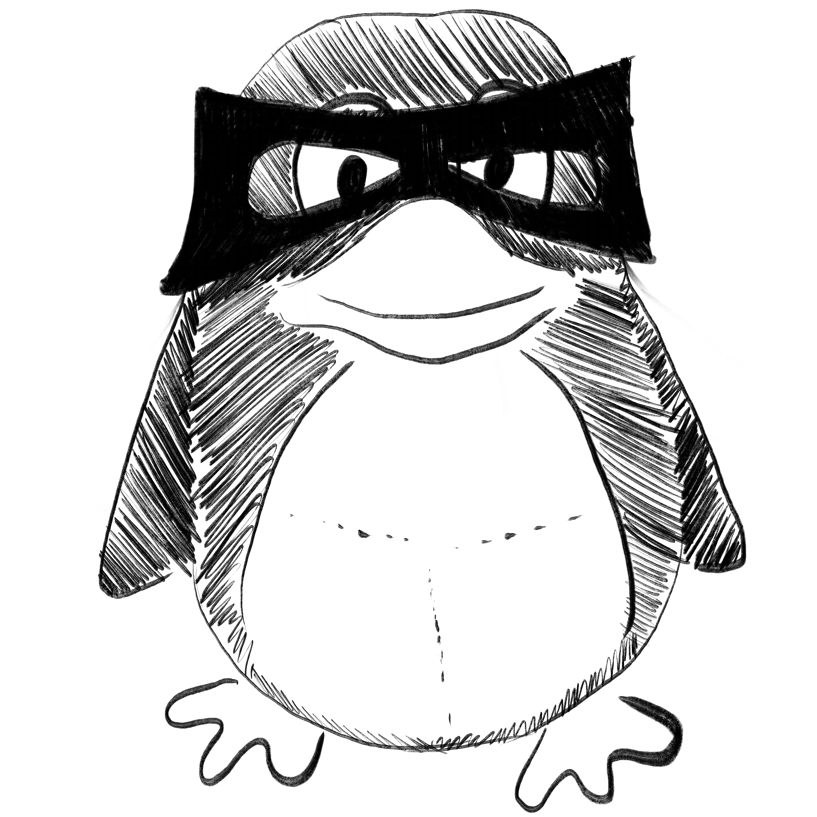
Characterization of chromatin accessibility patterns in different mouse cell types using machine learning methods at single-cell resolution.
In Frontiers in genetics ; h5-index 62.0
Xu Yaochen, Huang FeiMing, Guo Wei, Feng KaiYan, Zhu Lin, Zeng Zhenbing, Huang Tao, Cai Yu-Dong
2023
biomarker genes, chromatin accessibility, chromatin heterogeneity, machine learning, mouse cell type, single-cell resolution
Identification of immune biomarkers associated with basement membranes in idiopathic pulmonary fibrosis and their pan-cancer analysis.
In Frontiers in genetics ; h5-index 62.0
Fu Chenkun, Chen Lina, Cheng Yiju, Yang Wenting, Zhu Honglan, Wu Xiao, Cai Banruo
2023
basement membrane, idiopathic pulmonary fibrosis, immune, interstitial lung disease, pan-cancer
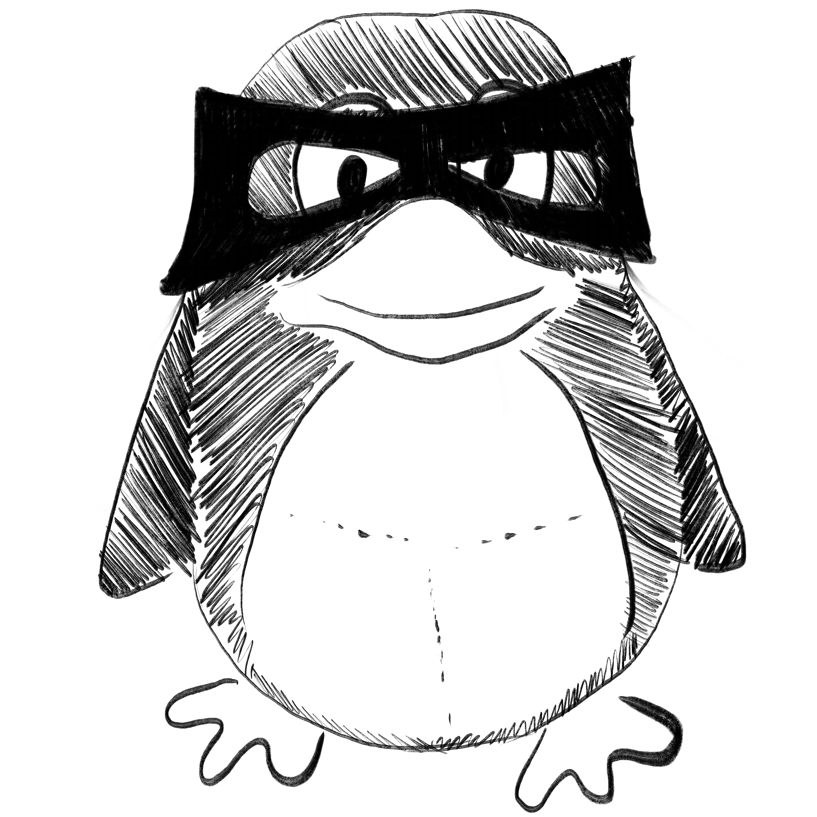
Towards automated video-based assessment of dystonia in dyskinetic cerebral palsy: A novel approach using markerless motion tracking and machine learning.
In Frontiers in robotics and AI
Haberfehlner Helga, van de Ven Shankara S, van der Burg Sven A, Huber Florian, Georgievska Sonja, Aleo Ignazio, Harlaar Jaap, Bonouvrié Laura A, van der Krogt Marjolein M, Buizer Annemieke I
2023
cerebral palsy, human pose estimation, machine learning, markerless skeleton tracking, motion capture, movement disorders
Multi-omics to predict acute radiation esophagitis in patients with lung cancer treated with intensity-modulated radiation therapy.
In European journal of medical research
PURPOSE :
METHODS :
RESULTS :
CONCLUSIONS :
Zheng Xiaoli, Guo Wei, Wang Yunhan, Zhang Jiang, Zhang Yuanpeng, Cheng Chen, Teng Xinzhi, Lam Saikit, Zhou Ta, Ma Zongrui, Liu Ruining, Wu Hui, Ge Hong, Cai Jing, Li Bing
2023-Mar-19
Acute radiation esophagitis, Dosiomics, Lung cancer, Machine learning, Radiomics, Radiotherapy
Weekly Summary
Receive a weekly summary and discussion of the top papers of the week by leading researchers in the field.