Receive a weekly summary and discussion of the top papers of the week by leading researchers in the field.
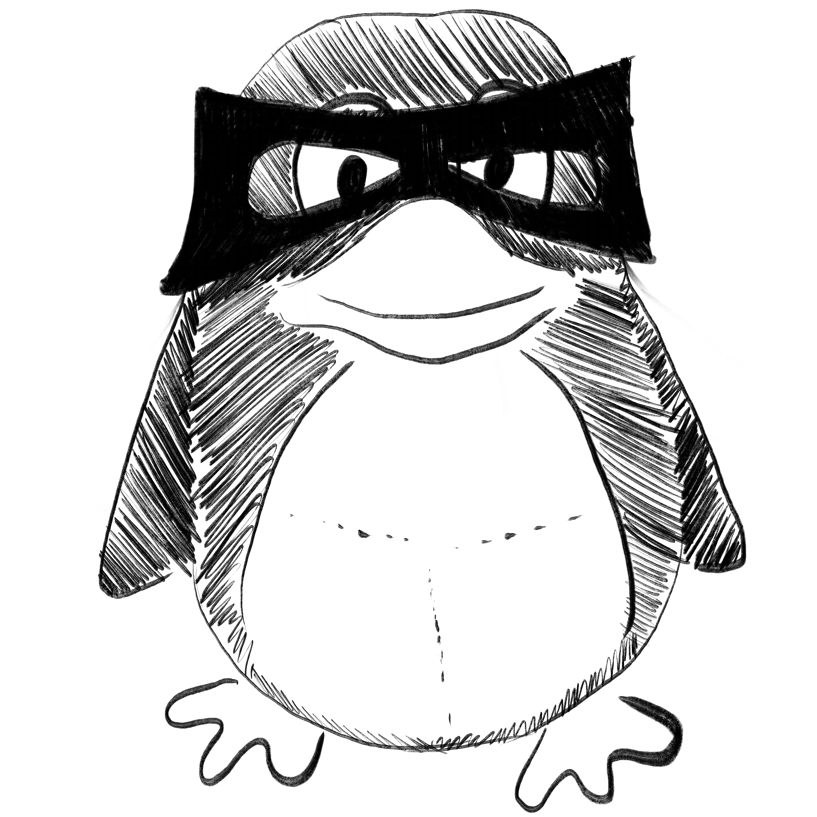
Mitigating climate and health impact of small-scale kiln industry using multi-spectral classifier and deep learning
Tackling Climate Change with Machine Learning workshop at ICLR 2023
Usman Nazir, Murtaza Taj, Momin Uppal, Sara Khalid
2023-03-21
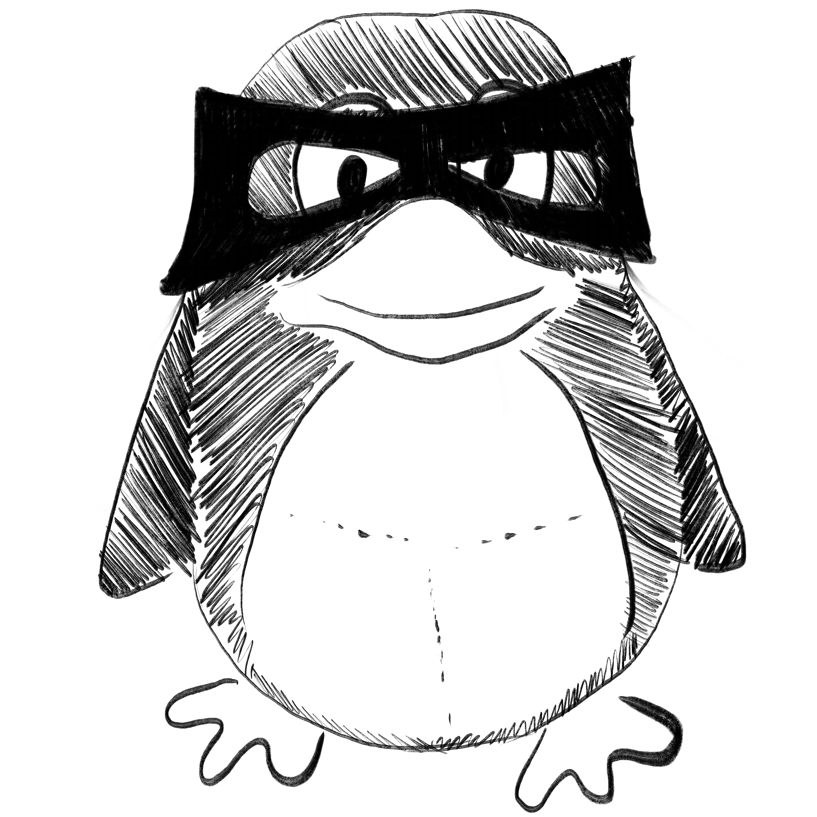
ALDH2 as a potential stem cell-related biomarker in lung adenocarcinoma: Comprehensive multi-omics analysis.
In Computational and structural biotechnology journal
Tran Thi-Oanh, Vo Thanh Hoa, Lam Luu Ho Thanh, Le Nguyen Quoc Khanh
2023
4-HNE, 4-Hydroxynonenal, AJCC, American Joint Committee On Cancer, ALDH, Aldehyde Dehydrogenase, Aldehyde Dehydrogenase 2, CGI, Cpg Island, CPTAC, Clinical Proteomic Tumor Analysis Consortium, CSCs, Cancer Stem Cells, Cancer stem cells, DNA methylation, Gene expression, IHC, Immunohistochemical, LCSCs, Liver Cancer Stem Cells, LUAD, Lung Adenocarcinoma, Lung adenocarcinoma, MAPK, Mitogen-Activated Protein Kinase, MDA, Malondialdehyde, NSCLC, Non-Small Cell Lung Cancer, OS, Overall Survival, Protein expression, ROS, Reactive Oxygen Species, SCLC, Small Cell Lung Cancer, Survival analysis, TCGA, The Cancer Genome Atlas, TMT, Tandem Mass Tags, TNM, Tumor-Node-Metastasis, UICC, International Union For Cancer Control, XRCC1, X-Ray Repair Cross-Complementing Protein 1
Computed tomography-based COVID-19 triage through a deep neural network using mask-weighted global average pooling.
In Frontiers in cellular and infection microbiology ; h5-index 53.0
BACKGROUND :
METHODS :
RESULTS :
CONCLUSIONS :
Zhang Hong-Tao, Sun Ze-Yu, Zhou Juan, Gao Shen, Dong Jing-Hui, Liu Yuan, Bai Xu, Ma Jin-Lin, Li Ming, Li Guang, Cai Jian-Ming, Sheng Fu-Geng
2023
artificial intelligence, computed tomography (CT), coronavirus disease 2019 (COVID-19), deep learning, global average pooling (GAP)
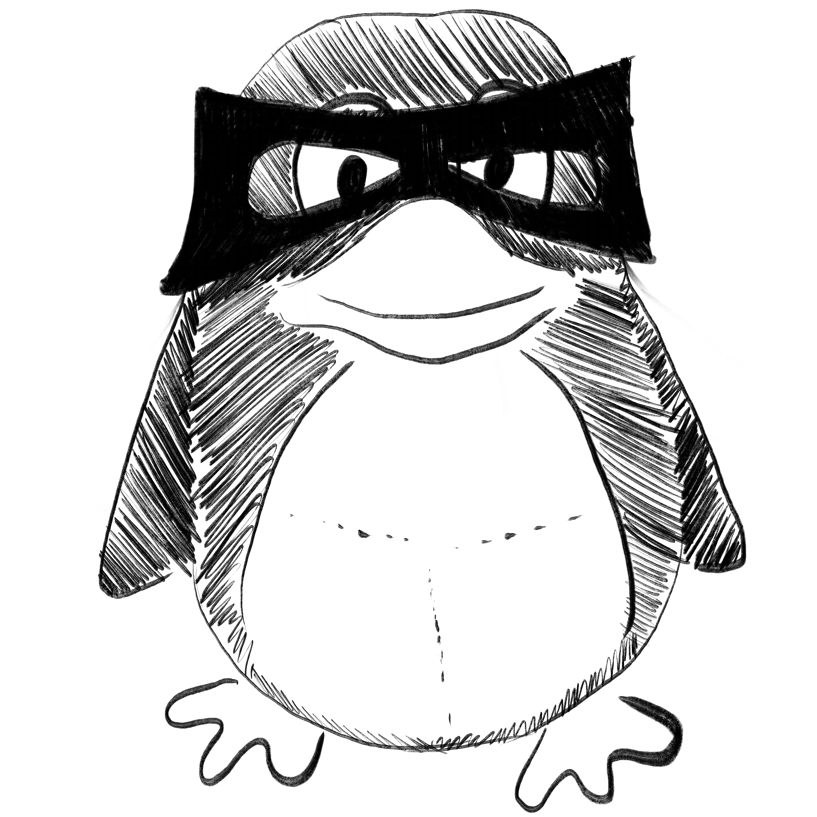
Applying Blockchain Technology in Network Public Opinion Risk Management System in Big Data Environment.
In Computational intelligence and neuroscience
Luo Zhenqing, Zhang Cheng
2023
Prediction of red blood cell transfusion after orthopedic surgery using an interpretable machine learning framework.
In Frontiers in surgery
OBJECTIVE :
METHODS :
RESULTS :
CONCLUSION :
Chen Yifeng, Cai Xiaoyu, Cao Zicheng, Lin Jie, Huang Wenyu, Zhuang Yuan, Xiao Lehan, Guan Xiaozhen, Wang Ying, Xia Xingqiu, Jiao Feng, Du Xiangjun, Jiang Guozhi, Wang Deqing
2023
RBC transfusion, interpretability, machine learning, orthopedic surgery, prediction model
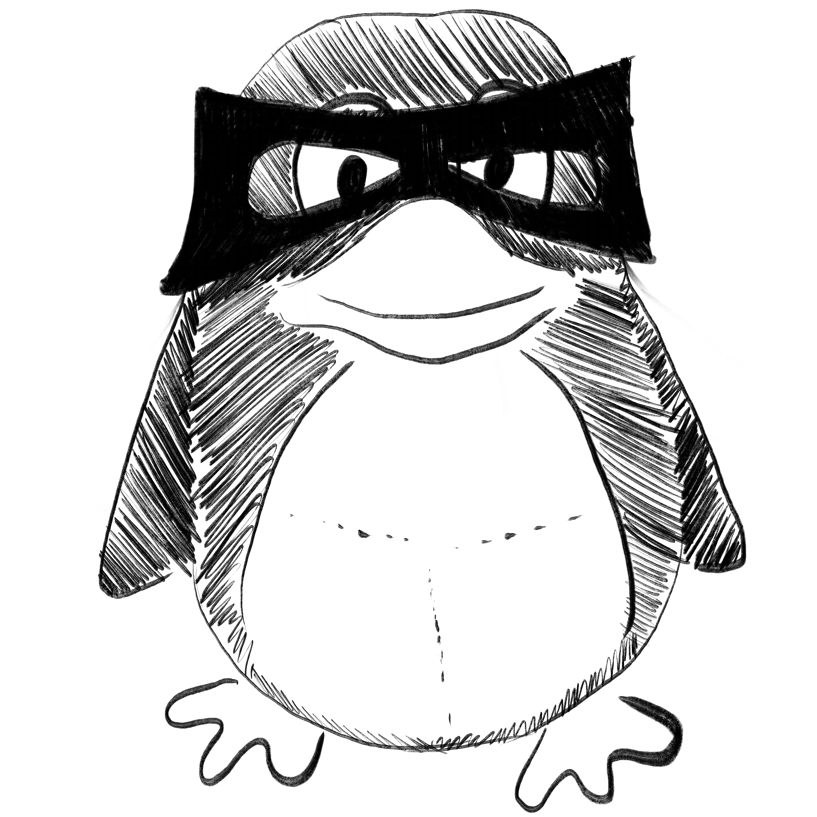
Field oriented control dataset of a 3-phase permanent magnet synchronous motor.
In Data in brief
Nustes Juan Camilo, Pau Danilo Pietro, Gruosso Giambattista
2023-Apr
ID control, Motor control, Neural networks, Simulink
Weekly Summary
Receive a weekly summary and discussion of the top papers of the week by leading researchers in the field.